Experience error-free AI audio transcription that's faster and cheaper than human transcription and includes speaker recognition by default! (Get started for free)
The Evolution of Neural Text-to-Speech Advancements in Natural Prosody and Emotion
The Evolution of Neural Text-to-Speech Advancements in Natural Prosody and Emotion - Neural TTS Models Surpass Traditional Methods in Naturalness
The emergence of neural TTS models has revolutionized the field of speech synthesis, surpassing traditional methods in their ability to generate naturally sounding speech. Traditional techniques, often relying on concatenating pre-recorded speech snippets, frequently resulted in a robotic, artificial quality. Neural TTS, however, leverages deep learning architectures to produce a more fluid and lifelike auditory experience. These models can now better emulate the nuances of human speech, including variations in intonation and pitch. Further, techniques like factorized vector quantization have allowed researchers to break down speech into fundamental elements like content, prosody, and timbre, leading to even more refined and nuanced synthesis. This advancement not only enhances the quality of the synthesized speech but also contributes to faster processing speeds, facilitating real-time applications. The ability to manipulate speech features like prosody also paves the way for more interactive and user-friendly applications, pushing the boundaries of human-computer interaction.
Neural TTS has fundamentally shifted the landscape of speech synthesis by leveraging deep learning. Instead of stitching together pre-recorded audio segments like traditional methods, these models learn intricate patterns from vast datasets, encompassing diverse accents and speech styles. This allows them to generate speech that is not only natural-sounding but also carries a greater degree of expressiveness, often outperforming traditional methods in conveying emotional nuances and regional characteristics.
One notable advancement has been the incorporation of adversarial training. This technique enables the models to produce contextually appropriate speech that surpasses the rigid phonetic rules of older systems, resulting in outputs that are more fluid and coherent. Neural TTS models have also shown remarkable prowess in capturing subtle prosodic features, such as intonation and stress patterns, which contribute to a more engaging auditory experience.
However, the complexity of neural TTS comes at a cost. The models demand substantial computational resources both during training and deployment. This presents a challenge for practical applications, particularly in resource-constrained environments, where the efficiency of traditional techniques might be preferred. Nonetheless, recent work with architectures like WaveNet and Parallel WaveGAN has significantly improved the audio fidelity of neural TTS, bringing it closer to the quality of recorded human speech.
The evolution of neural TTS has also fostered a greater emphasis on user interaction. More recent models can adjust their output in real-time based on user input, dynamically adapting speech style and pacing, leading to more dynamic and engaging user experiences. Furthermore, these models can now generate speech that expresses a wider range of emotions with convincing authenticity, making them particularly suited for applications like educational and entertainment platforms.
The quality of neural TTS hinges heavily on the training data. Models trained on linguistically diverse datasets excel at producing speech that reflects a variety of cultural backgrounds. But, this highlights a major hurdle—ensuring that generated speech is free of bias and accurately reflects the full spectrum of human linguistic expression. The remarkable advancements in neural TTS have brought about ethical questions concerning the potential for voice impersonation and the psychological effects of synthetic voices on users, urging us to consider not only the technology itself but also its broader implications.
The Evolution of Neural Text-to-Speech Advancements in Natural Prosody and Emotion - Advancements in Prosody Control for Dynamic Speech Synthesis
Recent advancements in controlling prosody within dynamic speech synthesis represent a significant step forward in neural TTS. New approaches, like the ProsodyTTS model, are combining statistical modeling with neural networks to achieve a more granular control over speech characteristics, leading to increased precision in manipulation. However, many contemporary neural TTS systems still primarily rely on attention mechanisms, often lacking explicit methods for managing prosody during speech generation. Additionally, traditional emotional speech synthesis systems, heavily reliant on supervised learning methods that pair text with emotional speech, face limitations in capturing and expressing diverse emotional states.
The field is increasingly exploring interactive, multi-level techniques for prosody control in neural TTS models. This involves incorporating linguistic features like sentence structure and meaning into the synthesis process to improve the naturalness and expressiveness of synthetic speech. The hope is that these new methods will result in synthetic speech that sounds even more like natural human speech, capturing the full range of intonation and expression. While progress has been made, challenges remain in fully achieving the nuanced expressiveness and variability found in authentic human communication.
The quest for more natural-sounding speech synthesis has led to a surge in research focused on refining prosody control within neural TTS models. While attention-based mechanisms have become prevalent in end-to-end TTS, explicitly controlling the desired prosody during generation remains a challenge. Early attempts involved embedding global prosodic features from reference audio to influence synthesized speech, but these methods often lacked finesse.
The introduction of models like ProsodyTTS, trained with supervised auxiliary learning, has shown promise in improving generalization capabilities, but still faces limitations. The ability to model subtle melody, effectively mimicking the emotional nuances present in human speech, is a current focal point. Further, understanding and appropriately applying contextual cues from text, like punctuation and semantics, to dynamically adjust prosodic elements is crucial for making synthetic speech sound more natural and coherent.
Furthermore, differentiating between periodic and aperiodic speech elements is becoming increasingly important for creating more realistic variations in pitch and volume. The desire for dynamic speech also drives the development of multi-speaker synthesis, enabling single models to emulate a wider range of speaking styles and personalities. This could have significant impacts on creating engaging and believable interactions in dialogue systems. Beyond simply expressing explicit emotions, there's growing interest in teaching TTS models to interpret and express implicit emotions found in the context of the text. This includes subtle cues like sarcasm or disappointment, pushing the boundaries of creating authentic synthetic conversations.
Generative Adversarial Networks (GANs) have shown potential in refining prosody control. By pitting a generator against a discriminator, these models learn to produce speech with improved naturalness. Additionally, research now involves detecting and correcting instances where prosody doesn't align with the content being spoken, leading to a more harmonious and less jarring experience.
The goal of developing TTS systems that can seamlessly adapt to different languages while maintaining accurate emotional and contextual cues is also gaining momentum. This cross-linguistic prosody adaptation would pave the way for broader global adoption. Meanwhile, user-driven customization is gaining traction. Ideally, users will be able to specify the prosodic features they prefer, such as speech pace and tone, leading to more tailored and interactive experiences. Finally, researchers are investigating real-time prosody adjustment, allowing systems to react to user feedback or environmental factors, maintaining clarity and emotional resonance even in dynamic settings. This ability to adapt dynamically adds another layer of complexity to the field, and while advancements are being made, achieving truly fluid and adaptive prosody in neural TTS remains an active area of exploration.
The Evolution of Neural Text-to-Speech Advancements in Natural Prosody and Emotion - Emotion Detection and Generation in TTS Systems
The ability of TTS systems to detect and generate emotions is an area of growing research. Recent innovations, such as EmoCtrlTTS, demonstrate the potential for generating emotionally expressive speech. EmoCtrlTTS offers emotion-controllable zero-shot TTS, which can generate highly emotional speech along with non-verbal cues, leading to more lifelike speech synthesis. Efforts are also focused on Multimodal Emotion Recognition (MER) systems, aiming to incorporate various data sources like facial expressions and physiological signals to better understand and replicate human emotions. Furthermore, researchers are exploring the use of advanced machine learning techniques like convolutional neural networks and attention-based networks to refine emotion detection within text and speech. Transformer models, such as BERT, have also proven effective in analyzing textual context and identifying emotions within the text. Despite these advancements, the field faces challenges in capturing the full range of human emotional expression and ensuring the generated speech remains both contextually relevant and unbiased. The complexity and nuance of human emotions make it a difficult area for TTS to fully replicate.
Current efforts in neural TTS are starting to explore how computers can understand and replicate human emotions. This area, often called affective computing, relies on analyzing various aspects of input, like the tone and context of text, to recognize, interpret, and simulate human feelings.
However, accurately detecting emotions in text and then generating synthetic speech that reflects them is still quite challenging. Many TTS systems try to extract specific features like pitch, speed, and volume from audio to pinpoint emotion. But, relying solely on these acoustic cues can lead to misinterpretations. For example, a system might incorrectly infer happiness from a fast speaking rate, which can result in generated speech sounding out of place or artificial.
To address this, some TTS systems utilize emotion lexicons – basically, a dictionary where words or phrases are associated with particular emotional weights. This approach allows systems to tailor their output based on the detected sentiment of the text. But, the potential issue here is that any biases inherent in the training data might get reinforced and amplified by the system.
Interestingly, modern TTS systems have the capacity to weave together different emotional tones within a single output. This capability opens up the possibility of expressing more nuanced and multifaceted emotional states that are difficult to convey using just one emotional tone.
Beyond audio cues, researchers have observed that some neural TTS systems can even learn to interpret non-verbal clues, such as punctuation patterns and sentence structure, as subtle emotional indicators. These systems are beginning to leverage those subtle nuances in a way that contributes to a more expressive, and arguably more human-like, generated speech output.
Currently, there's a growing focus on using reinforcement learning to guide the generation of emotional speech. The goal is to create algorithms that receive feedback from users based on how they perceive the emotional quality of the synthetic speech. This feedback loop can help refine the models' ability to generate outputs that match users' expectations.
However, there are still many limitations. Synthesizing complex and nuanced emotions like sarcasm or irony remains a significant hurdle. It highlights the challenge of developing systems that truly understand and replicate the subtleties of human emotional expression, both verbal and non-verbal.
The performance of these systems is greatly impacted by the diversity and richness of the training datasets. Systems trained on a broader range of emotional expressions tend to generate more natural sounding outputs compared to those trained on smaller, less diverse datasets.
There is also the question of how listeners interpret different emotional cues in synthesized speech. It appears that certain combinations of pitch, speed, and other aspects of speech, which might be considered appropriate for expressing happiness, may not translate as effectively when conveying other emotions, potentially causing inconsistencies in how listeners interpret the synthesized speech.
Researchers are actively exploring how to build what we call 'emotion-aware' TTS systems. This could lead to applications in areas like therapy and education, where conveying emotional responsiveness through speech can have a positive effect on users. However, we also need to consider the ethical implications of these systems, such as the potential for emotional manipulation in future applications.
The Evolution of Neural Text-to-Speech Advancements in Natural Prosody and Emotion - Zero-Shot Cross-Lingual Emotion Transfer Techniques
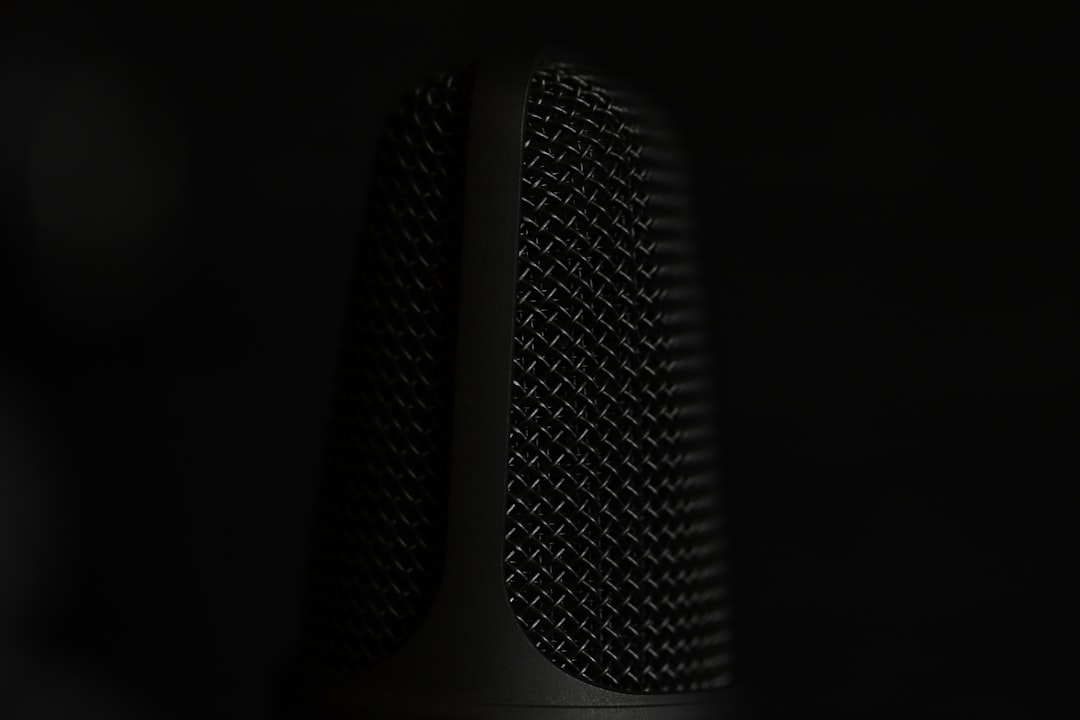
Zero-shot cross-lingual emotion transfer techniques introduce a novel approach to speech synthesis, tackling the complex task of conveying emotions across different languages without the need for training data specifically paired for each language. This method attempts to address challenges like unnatural accents that often arise when transferring emotions between languages. Promising developments, such as the DelightfulTTS architecture, strive to enhance the capabilities of zero-shot emotion transfer, though the intricate interplay of emotions within cross-lingual speech synthesis remains relatively under-investigated. Further, the inherent connections between speaker characteristics, emotional expressions, and language features make the creation of truly expressive systems across languages a considerable obstacle. As researchers continue to delve into robust training strategies and the implementation of emotional controls, the field moves towards generating more nuanced and genuine synthetic speech across multiple languages.
Zero-shot cross-lingual emotion transfer in TTS aims to generate emotional speech in a target language without needing specific training data for that language. The idea is to take what a model learns about emotions in one language (say, English) and apply it to another (like Spanish) by utilizing shared emotional representations across languages. These techniques rely on shared semantic spaces that link emotional cues between languages.
However, the success of this zero-shot transfer often hinges on the richness of the source language's data. Languages with ample resources tend to be better starting points for accurate emotional transfer when dealing with mixed-language scenarios. Intriguingly, sometimes these zero-shot methods can produce outputs that are more expressive than those from models trained explicitly for the target language. It seems they leverage the emotional depth of the source language in novel and inventive ways.
The major challenge here is the varying cultural contexts tied to emotions across languages. What might be a common emotional expression in one culture might not be easily understood or even appropriate in another. This means a generic emotional representation might not work seamlessly across linguistic boundaries.
Evaluating the accuracy of emotions in zero-shot transfers remains mostly reliant on human assessments. Automated metrics are still struggling to fully capture the subtle intricacies of emotional expression, making evaluations somewhat subjective. Research suggests that people's acceptance of synthetic emotional speech varies depending on their familiarity with how those emotions are typically expressed in their native language. This demonstrates the intricate link between emotion recognition and cultural context.
Researchers believe combining zero-shot techniques with various sensory inputs—facial expressions or gestures—could make synthetic speech more realistic and emotionally richer, potentially helping to bridge gaps in emotional comprehension. Current refinement efforts involve incorporating adversarial training to boost the authenticity of emotional speech outputs. This is a direct attempt to address the challenges of achieving accurate emotional fidelity across different languages.
As zero-shot cross-lingual emotion transfer gains more attention, ethical concerns arise. There's a potential for emotional manipulation and misuse in different applications, urging us to use it cautiously and consider its broader implications. It's a powerful technique, but needs careful consideration before it's widely deployed.
The Evolution of Neural Text-to-Speech Advancements in Natural Prosody and Emotion - End-to-End TTS Synthesis Improves Pitch and Rhythm
End-to-end TTS systems have shown a remarkable ability to improve the naturalness of synthesized speech, especially in terms of pitch and rhythm. By directly mapping text to speech waveforms, these models can better capture and represent the complex interplay of intonation and timing that is crucial for human-like speech. This is a significant step forward from earlier methods that often struggled to produce speech that sounded fluid and expressive. The capacity to learn these subtle prosodic details enables the generated speech to better reflect the rhythm and nuances of natural conversation.
Moreover, these systems can now incorporate emotional cues into the synthesized output. The models can adapt the speech characteristics in a manner that better reflects the intended emotion within the text, whether it's excitement, sadness, or any other human sentiment. This ability to create speech with an emotional dimension makes the experience far more engaging and immersive. However, achieving a truly convincing level of emotionality in synthetic speech remains a challenge. The complexity of human emotions and the vast range of ways they are expressed through language and voice make this a difficult goal to attain.
In essence, end-to-end TTS is helping to bridge the gap between robotic-sounding speech and genuine human communication. The advancements in modeling pitch and rhythm have made a tangible difference, making synthesized speech more appealing and believable. While still in development, the potential of end-to-end TTS to continue pushing the boundaries of natural and emotionally-rich speech synthesis is promising.
End-to-end TTS synthesis has shown remarkable progress in producing speech with more natural pitch and rhythm. By streamlining the process and eliminating separate modules for these aspects, we've seen more cohesive and lifelike speech. This is a significant advancement compared to the somewhat robotic quality of traditional concatenative and parametric systems.
Neural TTS models, like WaveRNN and FastSpeech, have been instrumental in this improvement. They directly generate audio waveforms, allowing finer control over aspects like pitch and rhythm. Moreover, training models on specific prosodic features, like stress and intonation, has improved their ability to convey emotional nuances, making the generated speech more expressive.
Furthermore, the ability of modern TTS systems to adjust pitch and rhythm in real-time, based on the context of the input, has significantly impacted the interaction with them. This dynamism makes the interaction feel more human-like, moving away from a purely mechanical interaction. It's an interesting development that allows us to better adapt to users' needs.
Interestingly, incorporating things like facial expressions and gestures into training datasets has shown promising results. It enhances the capacity of TTS systems to better align their speech with the way humans express emotion, making the generated output more realistic.
Additionally, end-to-end systems have excelled in transferring emotional aspects between languages. They can effectively leverage commonalities in sounds and emotional expression across different languages to produce natural-sounding speech. This has the potential to open up a wider range of applications and improve accessibility to users globally.
The integration of generative adversarial networks (GANs) in training has also refined pitch and rhythm control, yielding a more organic and smoother speech output. They are particularly useful in correcting instances where the generated speech has poor prosodic alignment.
Despite these advancements, perfect pitch and rhythm consistency remains a challenge, particularly when the model encounters unusual input or is not trained on sufficiently varied data. It's a constant challenge in the field.
We've also found that the diversity and quality of the training datasets directly influence the performance of end-to-end TTS in adjusting pitch and rhythm. Models trained on extensive and representative data outperform those trained on limited data, leading to concerns about bias potentially being introduced.
Finally, researchers are increasingly looking into ways to incorporate user feedback into the training process. It's hoped that through this real-time interaction we might be able to enhance pitch and rhythm control for different uses and individual user preferences. It's a promising field for personalized and improved voice synthesis.
The Evolution of Neural Text-to-Speech Advancements in Natural Prosody and Emotion - Deep Learning Algorithms Revolutionize Speech Quality from Text
Deep learning has revolutionized the field of text-to-speech (TTS) by enabling the production of significantly more natural-sounding speech. These advancements rely on neural network architectures, including recurrent and convolutional networks, which have enhanced the quality and articulation of synthetic voices. The ability to generate speech with a greater degree of natural prosody—including intonation, stress, and rhythm—has been a key development, leading to a more expressive and engaging auditory experience. TTS systems can now effectively convey emotional nuances within the synthesized speech, making the generated voice more human-like. However, the complexity of these deep learning models presents challenges, requiring significant computational resources for training and implementation. Additionally, ensuring access to a wide range of diverse training data is crucial for overcoming biases and producing a more representative spectrum of voices. Nonetheless, the continuing development of deep learning algorithms for TTS holds significant promise for future improvements in speech quality and personalization, enabling a greater breadth of applications across various domains.
Deep learning has significantly advanced the field of text-to-speech (TTS) by enabling the creation of more natural and human-like synthetic speech. The use of neural networks, especially recurrent neural networks (RNNs) and convolutional neural networks (CNNs), has been key to enhancing the quality and articulation of synthetic voices. Furthermore, deep learning techniques have improved the incorporation of prosody—intonation, stress, and rhythm—into TTS, leading to a smoother and more expressive listening experience.
One exciting frontier in TTS is the growing ability to synthesize speech that conveys various emotions. This is accomplished by training models to recognize and replicate the emotional nuances embedded within text. Novel models like WaveNet and Tacotron have been pivotal in this area by generating audio waveforms directly from text, avoiding the limitations of traditional approaches that stitch together pre-recorded speech segments. These 'end-to-end' systems are generally easier to train and deliver better results.
Researchers are exploring the potential of incorporating large pre-trained language models into TTS. The hope is that these models can provide a deeper understanding of the context and meaning within text, leading to even more sophisticated and nuanced speech output. The issue of diverse voices and accents is being addressed by increasing the range of data used to train models, making for a more personalized and inclusive experience.
Continued advancements in computing power and algorithm optimization are enabling the development of more intricate and powerful TTS models, further boosting the quality of generated speech. Neural TTS is increasingly finding applications in everyday technologies such as virtual assistants, audiobooks, and automated customer service systems, highlighting its rising importance and widespread use.
However, the complex nature of neural TTS also brings challenges. There's a growing awareness that biases present in the training data can have a negative impact on the quality and fairness of the resulting speech. It's crucial to consider these potential biases and work to mitigate them. Similarly, as synthetic voices become more convincingly human, there are ethical concerns regarding voice impersonation and the potential for malicious use of emotional manipulation in synthetic speech. As a community, we need to engage with these emerging ethical questions as the field advances.
Experience error-free AI audio transcription that's faster and cheaper than human transcription and includes speaker recognition by default! (Get started for free)
More Posts from transcribethis.io: