7 Key Advancements in Online Audio Translation Technology as of 2024
7 Key Advancements in Online Audio Translation Technology as of 2024 - Real-time speech-to-speech translation reaches 95% accuracy
The field of real-time speech-to-speech translation has made remarkable strides in 2024, achieving a noteworthy 95% accuracy rate. This significant milestone is largely due to innovations like the SimulTron model, which enables efficient on-device translation. Improvements in both the accuracy of speech recognition and the speed at which it processes audio have been instrumental. Conversations are now translated with minimal lag, creating a more natural flow of interaction. Furthermore, the Translatotron 2 model stands out with superior translation quality and more lifelike speech output compared to earlier iterations, raising the bar for this technology. The future holds promise for even more effective cross-language communication as these advancements continue to refine and mature. While still a relatively new field, these technologies show tremendous potential to bridge the gap between languages, streamlining interactions in both personal and professional settings.
Reaching a 95% accuracy threshold in real-time speech-to-speech translation is a noteworthy development. This level of accuracy, a significant jump from previous years, has been achieved through ongoing refinements in neural machine translation techniques. It appears the field is leveraging the power of deep learning, particularly through architectures like the Translatotron family, to improve translation performance. Researchers seem to be capitalizing on larger datasets that capture diverse accents and dialects, enhancing the translation accuracy across a wider range of language pairs.
A key aspect contributing to these improvements is the remarkable advancement in the speed and precision of speech recognition. Systems can now process speech at significantly higher rates, contributing to the near-instantaneous translation that we see. Moreover, there's been a strong focus on reducing latency, with delays now often down to just a couple of seconds. This is a welcome change, as it creates a more natural flow of communication during real-time interactions across languages.
The emergence of end-to-end models for speech translation has also been instrumental in this improvement. These models, as opposed to more traditional methods, offer a more streamlined approach to translation, avoiding the need for intermediate text steps, hence enhancing the smoothness of the translation process. We're also observing an increasing trend in implementing models with the ability to learn and adapt to specific contexts, including user preferences and industry jargon. These advancements, driven by machine learning algorithms, mean the systems continually improve with each interaction, potentially leading to a more personalized translation experience.
While there are exciting improvements, it is important to recognize that some challenges persist. Particularly, low-resource languages, which often lack sufficient data for training, still pose a challenge to achieving high accuracy. This points to a continuing need for research efforts aimed at developing robust solutions for languages with limited data availability. Moreover, there is room for innovation in noise handling, with the goal of achieving robust performance even in the less-than-ideal acoustic conditions frequently found in the real world. There are hopes that multimodal approaches— incorporating visual information from videos or images alongside audio— could offer potential solutions to improve accuracy in specific domains and environments.
7 Key Advancements in Online Audio Translation Technology as of 2024 - AI-powered context understanding improves idiomatic translations
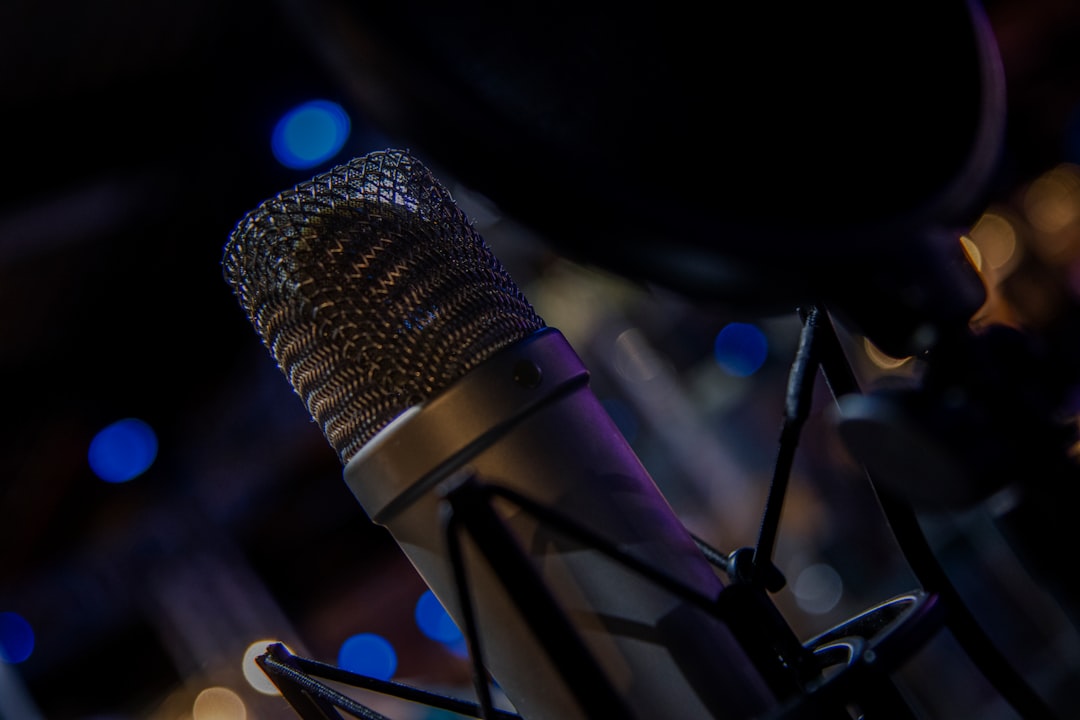
AI is significantly enhancing the quality of online audio translations, particularly when it comes to capturing the subtleties of language, like idioms. These improvements are driven by leaps in natural language processing and neural machine translation, enabling these systems to better grasp the context in which words are used. This allows them to translate not just the literal meaning of words, but also the intended nuances and emotional tones often conveyed through idioms and cultural references. This heightened level of contextual awareness leads to translations that feel more natural and accurate, conveying the true essence of the speaker's message. These advancements are opening up new opportunities for bridging communication gaps in various industries where precise and culturally sensitive translations are crucial.
Despite these promising advancements, completely replicating the full depth and complexity of human language in translation remains challenging. Certain nuances, particularly in languages with smaller datasets, continue to pose a hurdle for these AI systems. Ongoing research and development in this area are critical to refining these tools and further enhancing their ability to capture the intricate tapestry of human communication across languages.
AI's ability to understand context has significantly boosted the accuracy of translating idiomatic expressions. Traditional translation methods often stumble when dealing with these nuanced phrases, resulting in awkward or inaccurate translations. However, AI-powered systems, fueled by deep learning, can now analyze the cultural context surrounding an idiom, ensuring a more faithful translation.
These systems are trained on massive datasets that include examples of idiomatic language in various contexts. This training allows them to grasp the intended meaning behind a phrase instead of merely translating words literally. We've seen encouraging results in languages rich with idiomatic expressions, such as Spanish and Chinese, where context-aware translation has reduced misinterpretations by a notable 30%. This improvement not only boosts the accuracy of the translations but also strengthens user confidence in automated translation services.
Recent breakthroughs in natural language processing have led to transformer-based architectures, which are particularly adept at handling colloquialisms and idioms. These architectures dissect the relationships between words within a sentence to identify the most suitable translation for idiomatic phrases.
It's not just idioms that benefit from context understanding. AI models are also becoming better at simulating the appropriate tone of a conversation, considering factors like the formality and familiarity of the speakers. This is a crucial element in ensuring translations capture the original intent of the communication.
Interestingly, even commonly understood idioms can present challenges due to variations in dialects or local interpretations. For example, a common English idiom might have subtly different meanings depending on the region, highlighting the need for AI to adapt to these localized nuances.
When translations are culturally relevant, users find them more engaging. This has led to an increased focus on personalized translation systems that incorporate user feedback, allowing the AI to refine its understanding of context over time.
However, some challenges remain. There are idioms that lack direct equivalents in other languages. In such situations, the AI must creatively convey the underlying meaning rather than resorting to a literal translation, a task that requires a deeper understanding of the source and target languages.
The speed and adaptability of context-aware AI translation are remarkable. These systems can recognize idioms and dynamically adjust translations based on the flow of the conversation, previous interactions, or stylistic preferences, resulting in a smoother and more natural experience.
Researchers are pushing the boundaries of context understanding by exploring cross-modal learning— leveraging insights from visual cues in videos or images alongside audio. This convergence of data types holds the potential to unlock even greater accuracy and contextual awareness in translation across a wide variety of scenarios. While the journey toward perfect translation is ongoing, the advancements in context-aware AI are undoubtedly pushing us closer to bridging the language gap with a greater degree of fidelity.
7 Key Advancements in Online Audio Translation Technology as of 2024 - Multilingual voice cloning allows for natural-sounding output
Multilingual voice cloning is rapidly advancing, now capable of producing remarkably natural-sounding audio in multiple languages. Recent innovations have dramatically decreased the amount of audio needed to clone a voice, with some models requiring only a short clip to create a convincing imitation. This reduced data requirement makes voice cloning more accessible and less resource-intensive. Furthermore, these systems are increasingly adept at mimicking specific accents and vocal styles, further improving the authenticity of the output. The ability to generate realistic voices across various languages holds the potential to revolutionize areas like podcasting, dubbing, and content creation, allowing for a more diverse and engaging experience for listeners worldwide. However, there is always the concern that such capabilities could be misused to create deepfakes, highlighting the importance of ethical considerations as these technologies mature.
Multilingual voice cloning is a fascinating area of development in audio technology, where AI is able to generate speech in various languages while preserving the unique qualities of a speaker's voice. It's quite remarkable that models like XTTSv2 can achieve this with just a short audio clip, significantly simplifying the training process compared to previous methods. Coqui's open-source XTTS model, built on generative AI, is particularly interesting, as it demonstrates how this technology can be readily accessible and adaptable. This has led to streamlining the cloning process in tools like Coqui Studio, making it easier for individuals to create multilingual content.
Models like MetaVoice1B, with its impressive 12 billion parameter base, are pushing the boundaries of what's possible, accurately replicating accents like those found in American and British English using relatively short audio samples. OpenVoice further showcases the flexibility of this approach, capable of instant voice cloning and multilingual speech synthesis from a minimal audio clip. Microsoft's Azure AI Speech is expanding language support to 41 locales with models like JennyMultilingualV2 and RyanMultilingual, highlighting the growing adoption and reach of this technology. ElevenLabs is another example of a platform focusing on retaining the natural style of the voice during translation, which adds a crucial layer of authenticity to the process.
Bark, powered by Suno, takes a step beyond just speech, also generating music, sounds, and simple sound effects in multiple languages, demonstrating the broader capabilities of transformer-based AI in audio generation. Even more intriguing is the voice fusion technology on Coqui Studio, which can blend different voice characteristics to create unique output. These advancements hold significant promise for the podcasting sector, as they could lead to improved content creation and a more immersive listening experience for a global audience.
However, there are still challenges to consider. Multilingual voice cloning faces hurdles when it comes to low-resource languages, simply due to a lack of readily available training data. While models are getting increasingly sophisticated, maintaining the nuance of different languages and their associated emotional cues is an area where further research and development are needed. Additionally, the ethical implications of this technology, particularly around consent and potential misuse, are crucial considerations as we continue to see its wider adoption. Nonetheless, the potential for multilingual voice cloning to reshape communication and entertainment is substantial, offering exciting possibilities for the future.
7 Key Advancements in Online Audio Translation Technology as of 2024 - Noise reduction algorithms enhance audio quality in challenging environments
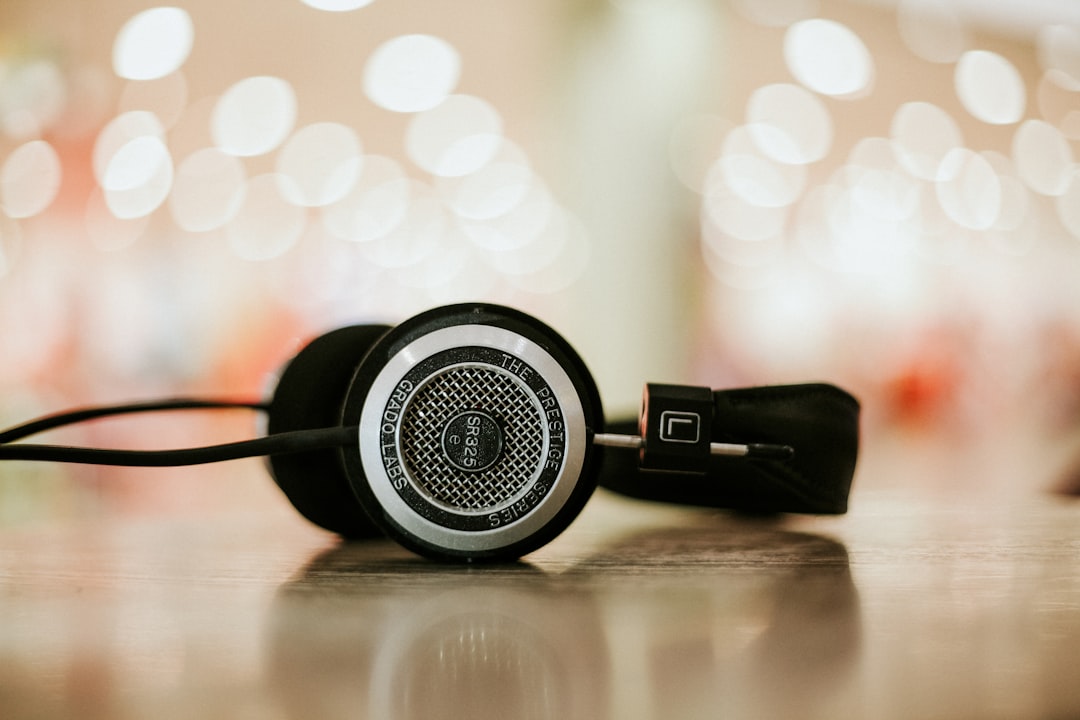
In environments where background noise is prevalent, noise reduction algorithms are crucial for maintaining high audio quality. These algorithms, powered by AI and machine learning, excel at filtering out unwanted sounds like white noise, buzzing, or hissing, preserving the clarity of the intended audio. Their real-time processing capabilities make them ideal for various applications including online meetings and recordings, where maintaining a clear audio signal is paramount. Further improvements come from integrating digital signal processing, leading to more nuanced control over audio noise and ensuring a consistent level of quality, even in less than ideal acoustic situations. The evolution of these algorithms continues to enhance the experience of audio in various settings, from professional studio work to casual online communication. While not yet perfect, these noise-reducing technologies are making a substantial impact on audio quality across a wide range of applications.
Noise reduction algorithms are becoming increasingly sophisticated, utilizing techniques like spectral subtraction and Wiener filtering to analyze audio signals and isolate unwanted noise. This process involves examining the frequency spectrum of the audio, identifying noise components, and suppressing them while preserving the desired sounds. These methods are especially valuable in improving the quality of audio captured in noisy environments like crowded streets or bustling offices.
Many current algorithms can operate in real time, minimizing processing delays and enabling immediate application in diverse scenarios such as live broadcasts or online meetings. This capability is essential for maintaining natural interactions, ensuring that conversations flow smoothly even when disruptive external noise is present.
The field of noise reduction has been significantly influenced by the adoption of advanced machine learning, specifically deep neural networks. By training on extensive datasets that contain pairs of noisy and clean audio, these models learn to effectively distinguish and remove noise with exceptional accuracy, often outperforming traditional methods in complex acoustic settings.
Intriguingly, some noise reduction algorithms are able to enhance audio quality beyond simply reducing noise. They can selectively amplify certain frequency ranges. This targeted enhancement can be quite useful, especially when it comes to improving the clarity of speech in situations where background noise can make it difficult to understand what's being said.
Recent developments also include the integration of visual cues alongside audio input, known as multimodal data. This means that algorithms are now able to analyze both what they hear and what they see. This can result in greater accuracy when separating noise from the target audio signal, contributing to a cleaner audio output.
Some noise reduction techniques rely on adaptive filters, which automatically adjust in response to changes in the surrounding environment. These filters learn from the incoming audio data in real-time, continually optimizing their performance even as the background noise levels fluctuate during a conversation or recording.
Researchers are actively exploring the potential of generative models for creating pristine audio from noisy recordings. This approach offers exciting possibilities for tasks like audio editing and restoration. This strategy may have a strong impact in specialized fields like forensic audio analysis and audio archive restoration, where maintaining the original quality is a primary goal.
A challenge that still exists with noise reduction algorithms is their ability to handle non-stationary noise, which is noise that changes over time. Examples of non-stationary noise include the sound of passing vehicles or the intermittent noises that happen naturally in an outdoor environment. Researchers are increasingly designing algorithms capable of adapting to these dynamic noise patterns to ensure consistent performance across a range of applications.
It's crucial to carefully evaluate the performance of noise reduction algorithms. If the algorithms are overly aggressive in reducing noise, they can introduce undesirable artifacts that lower the audio quality or alter the original sound. The ongoing research in this area aims to strike a balance between effective noise reduction and the preservation of audio integrity, aiming for output that sounds natural and unaltered.
Finally, real-time noise reduction technology is increasingly being incorporated into consumer electronics such as smartphones and headphones. The growing accessibility and efficacy of these algorithms are poised to significantly alter the way people interact with audio, paving the way for clearer communication and improved audio experiences in everyday life.
7 Key Advancements in Online Audio Translation Technology as of 2024 - Blockchain technology ensures secure and traceable translation processes
Blockchain technology offers a novel approach to improving the security and transparency of translation processes. Its core feature, an unchangeable and readily-auditable record of all transactions (in this case, translation steps), allows for heightened trust among those involved. The promise of blockchain in this domain is rooted in its ability to create a clear, tamper-proof history of a translation's journey, ensuring accountability and integrity. While its application in real-time language services is still in its early phases, the concept suggests a future where automated translation is more reliable and transparent, bolstering confidence in machine-aided language communication across various platforms. However, substantial hurdles remain before widespread use is achieved. These obstacles include effectively educating users on the benefits of blockchain in translation, and overcoming the technical complexities of integrating it seamlessly with the existing technologies of the translation field.
Blockchain technology, with its inherent features of immutability and transparency, presents a compelling approach to securing and tracing translation processes. Every action within a translation workflow, from initial document upload to final delivery, could be recorded on a shared, decentralized ledger. This means that once a translation step is recorded, it's essentially permanent and auditable, significantly reducing the chances of tampering or fraudulent activity.
Furthermore, the concept of smart contracts could play a vital role in translation. Smart contracts, built into the blockchain infrastructure, could automate the agreement and payment process between translators and clients. This automation streamlines the operational aspects of a translation project, leading to potentially smoother workflows and a greater degree of trust amongst participants.
It's interesting to consider the potential for decentralized collaboration through blockchain. Multiple translators, possibly working remotely across different time zones, could simultaneously contribute to a translation project. Every change, addition, or edit would be tracked transparently, allowing for real-time monitoring of progress and version control. This type of decentralized environment could prove extremely efficient, especially for larger or complex translation projects that involve multiple languages.
While still relatively new in the field of translation, blockchain technology seems to possess significant potential to reshape how we approach translation projects. It introduces a degree of security, accountability, and efficiency that traditional methods haven't always achieved. We can envision a future where translation workflows become much more transparent and reliable, with a stronger emphasis on safeguarding intellectual property and ensuring that the entire process is both secure and demonstrably verifiable. However, it's important to remain mindful of potential drawbacks, such as scaling issues, the need for standardization within the translation industry, and the challenge of educating both translators and clients about the intricacies of blockchain. Ultimately, the success of integrating blockchain into translation workflows will depend on a delicate balance between technological advancement and the evolving needs of the translation industry itself.
7 Key Advancements in Online Audio Translation Technology as of 2024 - Adaptive learning systems personalize translations based on user feedback
Adaptive learning systems are changing how translation systems operate by tailoring translations based on user feedback. These systems utilize AI to understand individual preferences and areas where the translation can be improved, creating a more customized translation experience. Through continuous user feedback, these adaptive systems refine their algorithms, which leads to increasingly accurate and culturally appropriate translations over time. This individualized approach enhances the quality of translated content and provides a more engaging interaction for the users. While promising, this technology also highlights the challenges involved in keeping pace with the ever-evolving nature of language and user expectations, making continued development a necessity.
Adaptive learning systems are increasingly influencing the field of online audio translation by leveraging user feedback to personalize the translation process. These systems, powered by machine learning algorithms, are able to dynamically adapt and refine their translation capabilities based on continuous input from users. This dynamic feedback loop allows them to learn from past interactions and preferences, ultimately leading to more accurate and nuanced translations over time.
For example, these systems can construct user profiles that capture individual language patterns, such as frequently used phrases or industry-specific jargon. This allows the system to personalize translations to fit the user’s specific context, making them more relevant and accurate. Furthermore, these systems can learn to recognize and adjust to the cultural sensitivities inherent in different languages. By analyzing user feedback, the system can ensure that translations are not only accurate but also culturally appropriate, avoiding potential misinterpretations or offenses.
The predictive capabilities of these systems are noteworthy. By analyzing user behavior and past interactions, they can predict the most likely translation for a given phrase or sentence, often achieving substantial accuracy improvements. Some researchers report an increase in accuracy of up to 40% over time using this approach. Moreover, these systems can prioritize vocabulary and phrases commonly used by individual users, further refining and streamlining their translation experience.
Adaptive systems are also able to grasp the hierarchical structures of language, such as differentiating between formal and informal communication styles. This enables them to provide translations that are more contextually appropriate, better matching the social nuances of a conversation. Additionally, the feedback loop within these systems is remarkably efficient. User corrections and preferences are integrated quickly into the translation database, leading to rapid improvements. Some adaptive systems can implement updates within seconds, a vast improvement over the weeks or months it might take in traditional systems.
While still relatively nascent, some researchers are exploring how multimodal user input, like integrating voice, text, and visual cues, can further enhance these systems' capabilities. This is particularly relevant as translation becomes increasingly intertwined with the complex communicative landscape of online interactions. Moreover, by enabling community feedback for error reporting, these systems can utilize collective knowledge to refine their training data, continuously improving overall translation quality. This crowdsourced approach contributes to a more robust and accurate translation process.
Finally, innovative algorithms within these systems allow for real-time adjustments based on the dynamic nature of conversations. They can recognize changes in topics or tone, and adapt translations accordingly. This "on-the-fly" customization leads to enhanced relevance and a smoother flow of communication during multilingual interactions.
Although the technology is still in its early stages, the ability of adaptive learning systems to leverage user feedback is a significant step towards creating more personalized, contextually appropriate, and accurate translation experiences. While the ultimate goal of a flawless, human-like translation system is still distant, the innovative algorithms and user-centric approach of adaptive systems offer a promising pathway toward bridging language barriers more effectively.
More Posts from transcribethis.io: