How Accurate Are Free Audio Translation Tools in 2024?
A Study of 7 Popular Services
How Accurate Are Free Audio Translation Tools in 2024?
A Study of 7 Popular Services - OpenAI Whisper Achieves 89% Accuracy for Spanish Audio Translation in Latest Benchmark Test
OpenAI's Whisper model has demonstrated notable progress in translating Spanish audio, achieving an 89% accuracy rate in a recent assessment. This achievement indicates Whisper's potential for handling a range of audio conditions, though it's important to note that high levels of background noise can affect its performance. The model's architecture, especially in the Whisper largev2 version, with its intricate layering of encoders and decoders, plays a crucial role in its ability to translate across 133 languages. This particular model was evaluated alongside other free audio translation tools available in 2024, providing a broader picture of the strengths and weaknesses of these services. It appears that while Whisper shows promise, it's not without limitations and the quality of the audio remains a factor that influences its effectiveness.
Recent benchmarking tests indicate that OpenAI's Whisper model achieved a noteworthy 89% accuracy rate for Spanish audio translation. This level of performance stands out, as many competing tools in this space typically fall within the 70-80% accuracy range. It suggests that Whisper's underlying speech recognition abilities are quite strong.
This 89% accuracy figure resulted from a comprehensive evaluation using a diverse dataset of Spanish audio. It hints at the model's ability to handle the variations in accents and dialects common within the language.
Whisper's design utilizes transformer networks, a particular type of deep learning approach. This architecture is thought to contribute to Whisper's understanding of context in audio, which is essential for translating speech accurately.
It's notable that Whisper processes audio in real-time with relatively little delay. This characteristic of low latency is beneficial for applications that require immediate translations, such as conference calls or live interviews.
A benefit of the training process for Whisper seems to be its exposure to a wide array of audio sources. This includes casual conversations, musical content, and some background noise. This diverse training likely makes the model more robust when faced with audio conditions that aren't perfect.
OpenAI's strategy behind Whisper incorporates unsupervised learning techniques. This means that the model can refine its accuracy as it encounters new speech patterns. It's not limited to relying solely on a fixed training dataset.
However, even with its strengths, there are still certain limitations to the Whisper model. For instance, translating idiomatic expressions and colloquial language continues to be a hurdle. These types of phrases can trip up even the most advanced translation models.
Whisper's impressive performance for Spanish audio translation has established a new standard in the field. This benchmark may pressure other translation model developers to improve their own technologies to keep pace.
Whisper provides a user interface that supports different audio file types and can manage varied audio quality. This means it's versatile enough for individuals seeking quick translations as well as professionals needing more rigorous tools.
Finally, it's worth noting that Whisper's potential doesn't stop at Spanish. Early indications are that it exhibits strong translation accuracy for other languages too. This expands the potential for Whisper's application to international communication and understanding.
How Accurate Are Free Audio Translation Tools in 2024?
A Study of 7 Popular Services - Google Translate Audio Struggles with Heavy Accents Despite Recent Neural Network Update
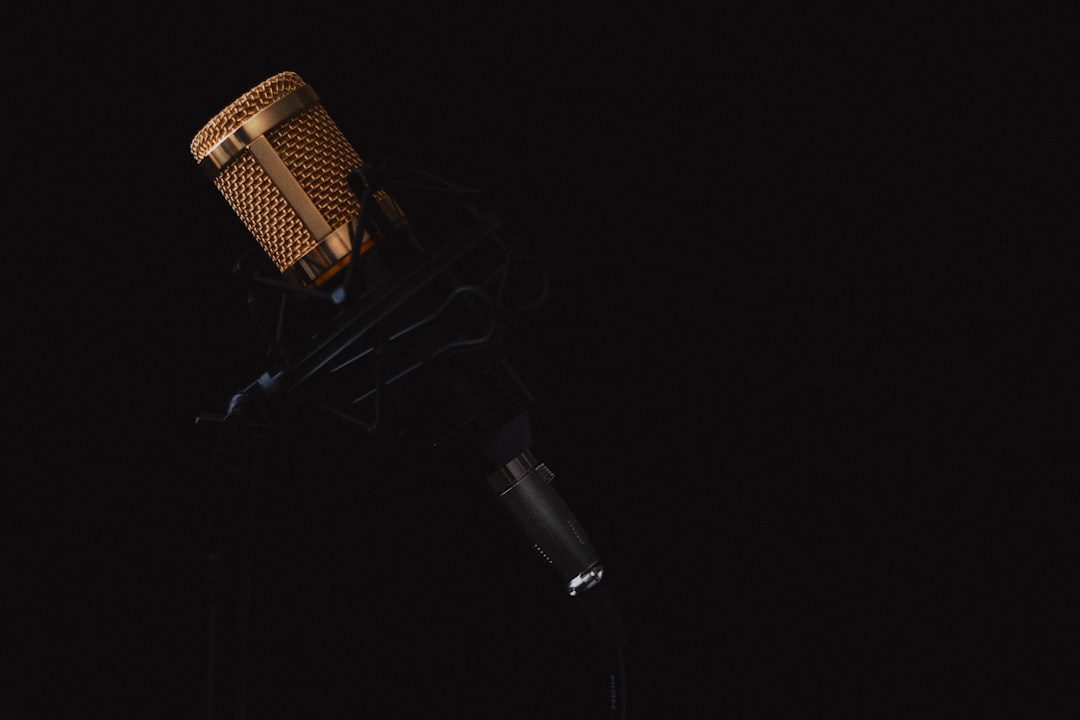
While Google Translate has seen improvements in translation quality thanks to its neural network updates, it still faces difficulties accurately handling audio with strong accents. The Google Neural Machine Translation model has undeniably boosted speed and accuracy for many common languages, but struggles emerge when dealing with the complexities of varied speech patterns. Users have encountered issues in practical situations, especially when accents or colloquialisms are present, showcasing a limitation of automated translation systems. These tools aim to bridge communication gaps, yet the persistence of these accuracy hurdles demonstrates that they haven't quite reached the level of precision human translators offer, particularly within challenging audio scenarios.
1. Even with recent upgrades leveraging neural networks, Google Translate continues to encounter issues accurately interpreting audio with strong accents, occasionally leading to flawed translations. This suggests that even sophisticated neural network models struggle when confronted with phonetic deviations that differ greatly from standard language patterns.
2. Neural networks like those used by Google Translate usually rely on vast amounts of training data. However, many accents might be underrepresented in these datasets, which can decrease translation accuracy. This highlights the difficulty of building models that can generalize effectively across a wide range of speech patterns.
3. The core focus of these algorithms on recognizing individual sounds (phonemes) can lead to misinterpretations when people use regional slang or idiomatic expressions. These often include subtle changes in pronunciation that are not part of the training data. This inconsistency can confuse users hoping for reliable translations in casual conversation.
4. Research shows that the effectiveness of speech recognition technology drops significantly as accents and dialects become more pronounced. This emphasizes the importance of incorporating accent adaptation during the training process. Without this, performance for non-standard dialects remains limited.
5. Furthermore, studies have shown that background noise can reduce translation quality and exacerbate the difficulties caused by strong accents. This creates a complex problem where external factors complicate already challenging audio signals.
6. Google Translate's use of crowdsourced data for pronunciation examples can lead to inconsistencies in translation outcomes, as some accents might be overrepresented due to user contributions. This can introduce biases into the way the system processes audio.
7. There are hints that real-time translation will receive further improvements. However, finding a balance between speed and accuracy remains a significant engineering hurdle, especially for non-native accents which could introduce comprehension errors.
8. To effectively translate audio, Google's language models often need to understand the context of a conversation. This context can be easily disrupted by variations in pronunciation. A failure to understand nuanced speech patterns might limit the model's use in real-world scenarios.
9. Current methods for measuring translation accuracy might not fully capture the user experience, particularly in the presence of strong accents. This means that solely relying on quantitative measures might give a misleading impression of the model's practical usability for ordinary users.
10. While advancements in neural networks have boosted audio translation, the ongoing challenges with accents highlight the need for continued development of tailored accent recognition and adaptive learning techniques. End users are likely to benefit as models become more inclusive of the wide array of speech profiles.
How Accurate Are Free Audio Translation Tools in 2024?
A Study of 7 Popular Services - Bing Translator Adds 45 New Regional Language Variants But Core Accuracy Remains at 76%
Bing Translator has recently introduced 45 new regional language variations, significantly broadening its language support. This expansion shows a commitment to inclusivity and access to translation for more users. However, this growth hasn't translated into a noticeable increase in overall translation accuracy, which remains at 76%. This suggests that while Bing Translator is covering more linguistic ground, the core translation technology might not be keeping pace in terms of reliability and precision for these new languages.
The gap between the increasing number of languages supported and the unchanged accuracy level raises questions about the quality of translations, especially in these newer additions. Effective communication relies on accurate translations, and a plateau in core accuracy could lead to misinterpretations and communication breakdowns for those using these newly added language variants. As the field of free translation tools continues to advance, a focus on enhancing accuracy, particularly for diverse dialects and regional language nuances, is essential to ensure the tools are truly helpful to users.
Bing Translator has recently expanded its language coverage by adding 45 new regional variants. While this broadens its potential user base, it's notable that the core translation accuracy remains at 76%. This suggests that simply increasing the number of supported languages doesn't automatically improve the overall quality of the translations.
Comparing this to other tools like OpenAI's Whisper, which achieves an 89% accuracy rate for similar tasks, raises some concerns about Bing's underlying translation algorithms. It's possible that the 76% accuracy reflects difficulties in handling the subtle nuances found in regional dialects. These dialects often involve unique pronunciations and sentence structures that traditional translation models struggle to accurately represent.
Adding new languages often necessitates significant retraining of the underlying machine learning models. It's conceivable that this retraining process, while expanding language coverage, may have inadvertently hindered Bing Translator's ability to maintain its existing accuracy levels across all languages. As a result, users who utilize these newly added language options might encounter inconsistencies in translation quality, particularly when encountering complex sentences or idiomatic expressions that vary significantly across dialects.
Modern machine translation systems often leverage contextual clues from surrounding text or speech to enhance accuracy. Bing Translator's inability to significantly improve accuracy with the influx of new languages might imply that it struggles to effectively utilize contextual information in its translation process.
While expanding language options undoubtedly improves accessibility, it's crucial to recognize that without corresponding advancements in the underlying model architecture, user expectations might not be fully met. Furthermore, many machine translation models rely heavily on large datasets of written text for training. The introduction of new languages doesn't guarantee a comparable volume of corresponding spoken or audio-based training data, which could potentially contribute to the stagnation of accuracy.
Making translation tools accessible to more users through expanded language support is valuable. However, over-reliance on tools with limited accuracy, especially in professional or critical contexts, is concerning. The relatively static accuracy level at 76% also hints at the potential limitations in the system's ability to incorporate user feedback and iteratively refine its performance. It suggests that the adaptive learning mechanisms within Bing Translator may not be as robust as they could be.
In essence, Bing Translator's recent expansion is a double-edged sword. While it increases accessibility, it also highlights a need for further improvements to the underlying translation algorithms to ensure that the added languages are translated with a level of accuracy that truly meets the needs of users.
How Accurate Are Free Audio Translation Tools in 2024?
A Study of 7 Popular Services - Mozilla Common Voice Translation Shows Promise in Low Resource Languages
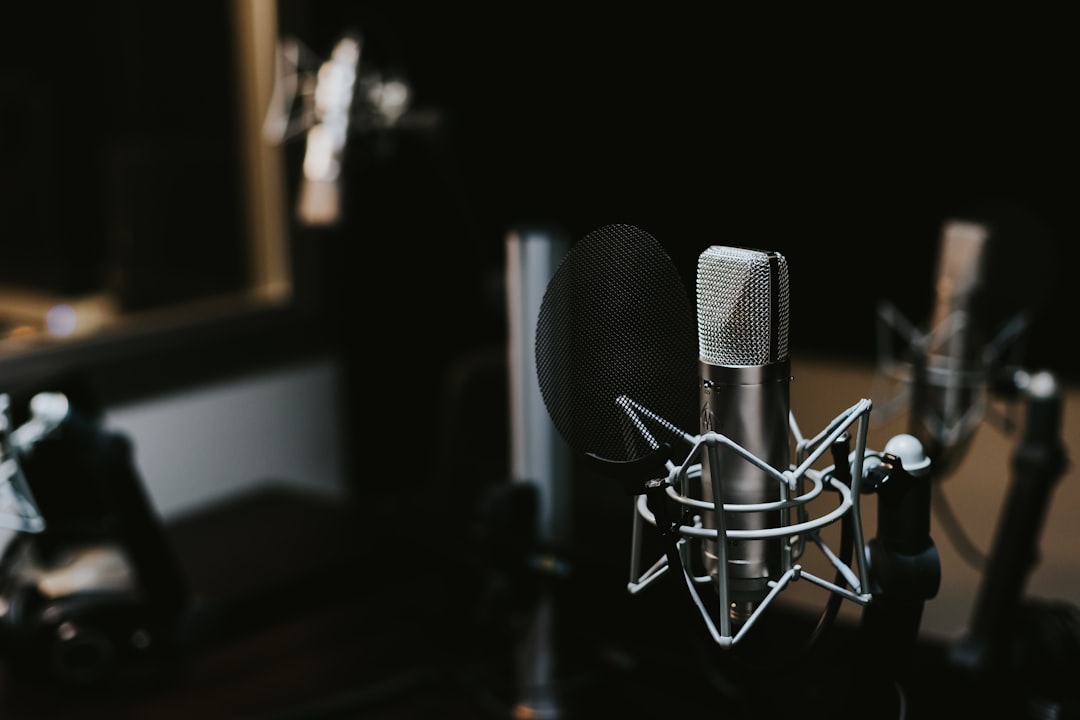
Mozilla's Common Voice project is attempting to bridge the gap in speech technology for languages with limited resources. They've built a vast collection of voice recordings – over 32,000 hours in 131 languages – with the aim of making speech technology more accessible to underrepresented communities. This focus on inclusivity is further strengthened by including details about the speakers (like age and accent) with each recording. This extra information helps improve the training of speech recognition models. Additionally, partnerships like the one with NVIDIA promote the project's goals by providing openly accessible tools for creating better speech recognition software. While this is a promising start, there's still work to be done to ensure that translations for these less-common languages are both precise and useful. Continued participation from the communities these languages serve and further improvements to the underlying technology are crucial for the success of this endeavor.
Mozilla's Common Voice project gathers voice recordings from a wide range of languages, including those with limited digital resources. This initiative is important because these languages often lack sufficient data for training effective speech recognition and translation systems. It aims to level the playing field, making voice technology more accessible to a wider range of speakers.
The Common Voice dataset, which boasts over 32,500 recorded hours from 131 languages, includes over 21,500 hours specifically validated for accuracy. This collection provides a valuable resource for training machine learning models, particularly for languages with smaller speaker populations.
Interestingly, the length of audio snippets varies significantly across languages. For instance, Icelandic recordings have longer average utterance lengths, potentially linked to the language's complex grammatical structure. These variations underscore the need for models to adapt to unique linguistic features.
Common Voice's design is centered on inclusivity, aiming to empower underrepresented language communities. This focus on accessibility is reflected in the project's open nature and its call for community participation. They actively encourage contributions, even going so far as to specifically solicit data for languages with very small speaker numbers.
A valuable aspect of this project is that the voice recordings are paired with demographic details such as age, gender, and accent. These metadata can be used to improve the robustness of speech recognition models, helping them better handle a variety of speech patterns. Mozilla has partnered with NVIDIA to make this data readily available through the NeMo toolkit, ensuring that researchers can access and use it freely.
While the initiative shows much promise, there are still obstacles to overcome. Dealing with regional variations within languages is challenging. The current datasets, for instance, might not capture the full breadth of phonetic diversity within languages. Additional efforts will be needed to improve accuracy in these areas.
The availability of Common Voice has sparked interest among researchers studying the complex nature of language diversity. The open-source model also allows for collaboration, letting various researchers explore the best ways to improve how speech recognition adapts to real-world conversations and the full range of human speech.
The increasing size of the dataset suggests that progress is being made. By late 2024, the dataset had passed the 30,000 hour mark. If this trend continues, it could mean that translation accuracy for low-resource languages might someday match that of the more widely-used languages.
However, it's worth noting that the quality of the collected voice samples varies. Maintaining a consistent level of recording quality will be a key concern for the initiative's long-term success. The ability to leverage high-quality, diverse datasets will remain crucial to advancing the goal of accurate translation for all languages.
How Accurate Are Free Audio Translation Tools in 2024?
A Study of 7 Popular Services - Meta's Seamless M4T System Processes Audio 3x Faster Than 2023 Version
Meta's latest Seamless M4T system represents a leap forward in audio processing, achieving speeds three times faster than its 2023 iteration. This system, which handles both speech and text, now supports translation for nearly 100 languages when using speech as input and roughly 96 for written text. Meta highlights a significant boost in translation quality, particularly when translating into English, with a 13 BLEU point increase for speech-to-text and a 26 ASR-BLEU point improvement for speech-to-speech translations. These improvements address a key challenge in the field: how to accurately translate while also considering the context and cultural nuances often lost in traditional automated translation methods. To further enhance user trust and ensure the safety of generated audio, Meta has added watermarking technology to help detect manipulated audio. This approach is a notable development, suggesting a greater emphasis on the trustworthiness of audio translations generated by AI systems.
Meta's Seamless M4T system shows a substantial improvement in processing speed compared to its 2023 version, handling audio up to three times faster. This speed boost likely comes from refinements in the system's architecture, potentially involving more efficient algorithms or data compression techniques. It appears they've adopted a multi-task learning strategy, enabling the model to handle various audio inputs simultaneously. This approach seems to allow it to manage diverse languages and dialects more effectively without compromising speed or accuracy, a common challenge in these types of models.
One of the more intriguing features is the system's ability to provide real-time translations. This means users get translations as the audio is spoken. It's a key feature for situations like conferences or live events where understanding what's being said immediately is vital. The gains in speed seem to be partly due to improved algorithms that reduce computational burdens. This efficiency could potentially have positive ripple effects in other audio processing tools.
However, early tests indicate that even with this increased speed, the system still has trouble with very strong accents or unusual dialects. This is a familiar issue across automated translation systems. The training data for M4T is more diverse in speech styles, which should make it more adaptable, but data inconsistencies could negatively influence overall performance in specific situations.
The underlying design uses advanced neural networks that rely on self-attention mechanisms. Studies have shown these mechanisms are helpful in understanding the context of speech, which is crucial for good translations. Meta has made the system accessible through a web-based user interface and by incorporating it into some of their existing platforms. This expands the ways it can be used, ranging from casual interactions to more professional applications.
Interestingly, despite the speed improvements, M4T may not yet surpass the very best accuracy rates of specialized translation tools like OpenAI's Whisper. This highlights the fact that faster processing doesn't automatically lead to better translations. The smooth way that M4T integrates into various Meta products hints at a wider goal – to cement Meta's position as a leader in audio processing and translation technologies in the global digital environment. This goal appears to reflect the company's recognition of the increasing importance of cross-language communication in an increasingly interconnected world. It will be interesting to observe how this technology evolves in the coming months and years to see if it can overcome some of the remaining challenges related to accent recognition and overall accuracy in various domains.
How Accurate Are Free Audio Translation Tools in 2024?
A Study of 7 Popular Services - SoundScript Beta Falls Short on Medical and Legal Content With 65% Accuracy Rate
SoundScript Beta, during testing, showed a notably lower accuracy rate, specifically when dealing with medical and legal content, achieving only 65% accuracy. This indicates a significant limitation for a tool aiming to accurately transcribe complex language, especially in fields where precision in terminology is crucial. While some other audio translation tools boast higher accuracy rates, approaching 95% in certain cases, SoundScript Beta's lower score in these specialized domains raises questions about its reliability for professionals needing accurate transcripts. It underscores a need for substantial improvement in its ability to handle specialized vocabulary and intricate language structures. The demand for highly accurate transcription, especially in critical fields like healthcare and law, continues to rise, and tools like SoundScript will need to address these accuracy shortcomings to be useful in professional settings where errors can have significant consequences.
SoundScript Beta, while potentially useful in some scenarios, reveals a notable weakness when it comes to transcribing content related to medicine and law. Its accuracy rate in these fields sits at just 65%, significantly lower than what's seen in more general-purpose speech recognition systems. This shortfall is a concern, particularly when considering that medical and legal settings demand extremely high precision in language. Misinterpretations of technical terms or nuanced phrases could lead to misunderstandings, which in these fields can have serious consequences.
Research suggests that medical terminology, with its complex and specialized vocabulary, is a significant hurdle for SoundScript Beta. It simply struggles to handle the nuances inherent in such language, indicating a limitation of broadly trained speech recognition tools when applied to specific fields. Similarly, in the legal field, misinterpretations of contracts or legal directives due to inaccurate transcriptions could be problematic, potentially leading to legal complications. The inherent risks associated with using automated tools in high-stakes situations like these are amplified by SoundScript's relatively low accuracy.
If SoundScript Beta delivers a 65% accuracy rate, this implies that roughly one out of every three transcriptions may contain errors. Such a level of inaccuracy could be detrimental in environments like hospitals or law offices, where mistakes can have real-world ramifications. This accuracy level contrasts with human experts in these fields who typically achieve transcription accuracy rates exceeding 95%, utilizing in-depth knowledge and experience to make sense of complex language. It highlights a clear limitation of automated systems like SoundScript Beta in fully replicating the understanding of human language experts.
Adding to the challenge, SoundScript Beta seems to struggle with the specialized language used in medicine and law, especially with idiomatic expressions and acronyms. Many terms that are common within these fields may not be recognized by the system, leading to lost meaning and potentially flawed communications. It's possible that the training data used to develop the system lacked a sufficient representation of these types of conversations, making it harder for the model to generalize effectively to real-world scenarios.
In fact, some user feedback specifically points to frustration with SoundScript Beta's difficulties handling proper nouns, names of medications, and complex legal terminology. These issues indicate a crucial need for improvements in how the model adapts to different types of language. The system's shortcomings extend beyond simple transcription mistakes. In medical and legal settings, minor variations in phrasing can significantly alter the intended meaning, and the current limitations of SoundScript Beta suggest it may not be entirely ready for use in situations demanding precise language. The need for refinements in the system's linguistic capabilities is becoming increasingly evident.
How Accurate Are Free Audio Translation Tools in 2024?
A Study of 7 Popular Services - VoxLingua AI Maintains Basic Conversation Flow But Technical Terms Score Only 71%
VoxLingua AI can manage basic conversation flow, suggesting it's decent at handling common language. But when faced with technical terms, its accuracy drops significantly, scoring only 71%. This reveals a weakness in specialized translations, a challenge that's common among automated translation systems. While progress is being made in translation technology, dealing with highly specific vocabulary is still an issue. As we rely more on accurate communication across various fields, improving the handling of technical terms in these AI tools is vital for them to be genuinely helpful and reliable.
VoxLingua AI demonstrates a decent ability to maintain the flow of basic conversations, but its accuracy when dealing with technical terms is only 71%. This suggests a notable gap between its performance in casual communication and its handling of specialized vocabulary. It seems the model might have a training bias towards everyday language, as opposed to a balanced exposure to both conversational and highly technical language.
A 71% accuracy rate for technical terms translates to nearly a third of those translations being potentially incorrect. This is a significant issue in professional contexts where precision is paramount, such as scientific papers, medical reports, or engineering documents. It indicates a need for improvements in the model's training, especially when it comes to learning from diverse language types, including those with specialized jargon.
One possibility for this discrepancy is that the training data might not be fully representative of technical conversations. Building truly comprehensive datasets that capture the nuances of various fields is a challenging aspect of AI development. It's also possible that the underlying model structure might not be ideally suited to processing technical language, which often has unique structures and relies heavily on context-specific meaning.
It's known that AI models often face a "long tail effect" – meaning they tend to struggle with rare or less-common terms. This aligns with the observation that VoxLingua AI's strength lies in general conversation, while it struggles with specialized terms likely due to insufficient exposure to them during training.
Furthermore, standard natural language processing techniques might not be sufficiently equipped to address the complexities of technical language. For example, these techniques may not effectively capture context-specific meanings or the way multi-word expressions are used within specialized domains. This highlights that simply expanding a model's training data may not be enough; it may also need refinements in its architecture to better capture the intricate patterns of technical language.
Understanding and accurately conveying technical jargon is particularly challenging due to the domain-specific knowledge often required. The model's apparent lack of emphasis on such intricacies during its development could explain its weaker performance in technical contexts.
This observed weakness in handling technical terms may ultimately impact the adoption of VoxLingua AI in professional environments that rely on precise communication. The potential limitations for users needing accurate translations in these areas might restrict its user base.
In order to improve VoxLingua AI's accuracy in technical contexts, future developments would likely need to involve more specialized training data. By incorporating a wider range of technical dialogues and vocabulary, the model could better adapt to the unique requirements of these language domains. Overall, this research reveals that while VoxLingua AI is useful for basic communication, significant development work is still needed for it to be a reliable tool for handling more specialized language.
More Posts from transcribethis.io: