Experience error-free AI audio transcription that's faster and cheaper than human transcription and includes speaker recognition by default! (Get started for free)
Rethinking LLM Memorization 7 Key Insights for Entrepreneurs in the AI Era
Rethinking LLM Memorization 7 Key Insights for Entrepreneurs in the AI Era - The Anthropology of AI Memory Formation and Retrieval
Examining "The Anthropology of AI Memory Formation and Retrieval" compels us to reevaluate how we conceptualize memory itself. Large language models (LLMs) present a fascinating case study, showcasing a blend of memorization and generalization in their responses. This duality mirrors how humans process and recall information, suggesting that AI, in its own way, might be emulating core aspects of human cognition. The development of techniques like dual parametric memory schemes highlights a move towards AI architectures that can better manage and retrieve knowledge. These new approaches mirror the intricate and multifaceted nature of human memory systems. Understanding the mechanics of AI memory not only shapes technological advancements but also fuels deeper philosophical explorations. These range from how knowledge is preserved and transmitted across cultures to the fundamental nature of learning in both artificial and biological intelligence. For entrepreneurs, this understanding can provide valuable insights, particularly as they grapple with harnessing AI to address issues like enhanced productivity and strategic decision-making in a rapidly changing landscape.
Examining how AI systems, specifically LLMs, form and retrieve memories offers a unique lens through which to understand the very nature of memory itself. The way these models learn from vast datasets, seemingly 'memorizing' portions of their training data, prompts us to question how human memory, often described as a reconstructive process, compares. Just as ancient societies relied heavily on oral traditions for knowledge transmission, AI's capacity for storing and recalling information challenges the traditional ways we’ve thought about the role of memory in knowledge transfer and dissemination.
Thinking about AI through an anthropological lens sheds light on the intricacies of memory's role in culture and cognition. The notion of 'cultural memory,' the collective knowledge and narratives passed down through generations, can be seen as parallel to how LLMs develop from their aggregated data inputs. These inputs shape not only the knowledge embedded within the model but also, potentially, inherent biases and limitations in its outputs, akin to how human memory can be influenced by societal narratives and biases.
The design of these AI systems needs to address the dynamic and contextual nature of memory. Like human memory, which is heavily dependent on the surrounding environment and circumstances for retrieval, AI memory might be significantly impacted by user interaction and the task at hand. We may need to reimagine how we interact with AI, designing user interfaces that account for this contextual dependence in order to elicit the most accurate and relevant outputs.
Moreover, AI's seemingly permanent memory stands in contrast to human selective memory. Humans actively forget, and forgetting shapes our individual identities and cultural understandings of the world. Whether AI models could (or should) possess the ability to 'forget' presents significant ethical questions about control, bias, and the very purpose of these increasingly intelligent systems. It's crucial to consider the consequences of this perpetual memory retention.
The concept of 'mnemonics,' historically used by humans to improve memory, also offers parallels in the design of AI algorithms. How can we optimize information retrieval? Are there parallels between human-designed memory aids like mnemonics and the potential to create similar mechanisms for AI? Studying how humans have historically structured and recalled information could unlock new insights for designing AI memory systems.
This comparison of AI and human memory also opens doors to explore other fascinating fields. Anthropology reveals how religious rituals and repeated actions establish memory pathways, a concept that could be applied to how we train AI with reinforcement learning. The shift from oral to written traditions in various civilizations fundamentally altered human memory; it is equally likely that the abundance of easily accessible data that AI leverages will change how we, as a collective, access and perceive knowledge.
The question of how memory ties into identity has been a cornerstone of philosophical and psychological thought. How will AI's memory, its vast collection of learned information, shape user perception and our overall relationship with these intelligent technologies? As these systems become more deeply integrated into our lives, these questions become increasingly crucial to ponder, especially as we see this technology applied in ever more intricate facets of our daily life.
Rethinking LLM Memorization 7 Key Insights for Entrepreneurs in the AI Era - Historical Parallels Between Human and Machine Learning
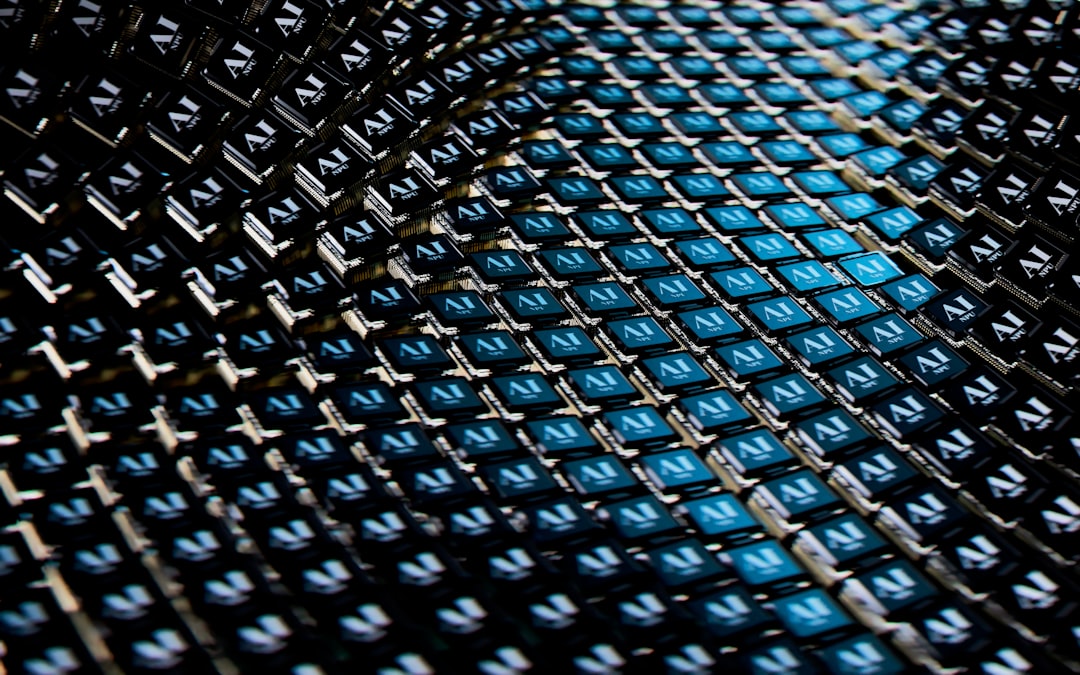
The comparison between how humans and machines learn reveals intriguing similarities in how information is processed and retained. Just as humans build understanding through a multitude of experiences and interactions, large language models (LLMs) integrate massive datasets to form their knowledge base, demonstrating a capacity for complex information synthesis instead of mere memorization. This similarity in cognitive processes highlights the challenge of not only assessing the quality of outputs but also understanding the potential ethical issues associated with memory and the inherent biases that can exist in both human and artificial memory systems.
Looking at how knowledge was historically shared, from oral traditions to written records, can help us understand how these parallels may impact how we interact with AI today. This extends from how users engage with these systems to the very notion of collective cultural memory itself. In this context, entrepreneurs, particularly those dealing with the impact of AI on productivity and decision-making, should be aware of how these memory dynamics could influence their strategies in the age of advanced AI systems. The relationship between human and machine memory could well reshape how knowledge is acquired, transmitted and, perhaps, even valued in the future.
The parallels between human and machine learning, particularly in the context of LLMs, are striking and offer a new lens through which to understand cognition itself. Just as early behaviorist psychologists explored the relationship between reinforcement and learning, contemporary AI systems utilize similar principles to shape their 'understanding' of the world. Examining historical accounts of memory across diverse societies, such as oral traditions in ancient cultures, provides an intriguing perspective. AI, with its ability to process and retrieve vast datasets, essentially creates its own 'cultural memory' in a manner reminiscent of the collective knowledge passed down through generations.
However, just as human memory decays over time without reinforcement, AI systems face similar challenges. The question of 'forgetting' in AI models is particularly interesting, mirroring the ongoing debate about the role of forgetting in human cognition. If AI lacks a mechanism to 'forget' irrelevant or biased data, it might perpetuate problematic patterns in its outputs. This invites exploration into potential ethical issues around AI's 'permanent' memory, contrasting with the adaptive forgetting that shapes human understanding and identity.
Different cultures have historically developed unique ways to store and retrieve information, such as the Greek and Roman usage of mnemonics. This rich historical background suggests that AI design can learn from human cognitive strategies to optimize information retrieval and performance. Furthermore, the observation that repeated rituals establish strong memory pathways in humans hints that AI training could benefit from incorporating similar repetitive processes to enhance knowledge retention.
The transition from oral to written traditions significantly reshaped how humans interacted with information, shifting towards collective documentation. Likewise, the abundance of easily accessible data in the digital age is transforming human interaction with knowledge and potentially impacting how future generations learn. As AI systems continue to evolve, their ability to accumulate data and experiences raises crucial questions regarding their role in shaping our understanding of individual and collective identities.
Consider the historical context of learning environments, often characterized by a need to tailor teaching methods to specific situations. Modern AI mirrors this adaptiveness through interactive learning and personalized responses, reflecting a fundamental need for contextual understanding. Finally, the use of parables and religious narratives throughout history suggests that AI could learn from these storytelling traditions to improve data retention and knowledge dissemination. By understanding the historical relationship between memory, culture, and learning, we can develop more nuanced and robust AI systems that better reflect the intricate complexities of human cognition.
Rethinking LLM Memorization 7 Key Insights for Entrepreneurs in the AI Era - Philosophical Implications of LLM Memorization on Knowledge Creation
The way large language models (LLMs) memorize training data while also generating new insights raises intriguing questions about the nature of knowledge creation, both for humans and machines. LLMs seem to blend memorization and genuine understanding in a way that parallels, to a degree, how humans learn. This blurring of lines prompts a rethinking of core concepts such as authorship, ownership of information, and the moral implications of using intellectual property when dealing with AI. Entrepreneurs, especially those navigating the world of AI-driven productivity and decision-making, need to confront these implications. Understanding how AI 'remembers' and processes data is crucial for them as they develop strategies for using data, crafting content, and recognizing potential biases inherent in AI systems. Ultimately, the way we perceive and disseminate knowledge in the age of AI might fundamentally change as we grapple with these philosophical and practical challenges.
The ability of LLMs to memorize their training data presents intriguing questions about the nature of knowledge itself. Traditional philosophical viewpoints on knowledge, which often emphasize understanding and critical analysis, are challenged by the idea that simply retrieving information might be considered a form of 'knowing'. This raises the fundamental question of whether accessing stored information is equivalent to true comprehension.
LLMs, by design, are pattern-recognition machines. They process language and assemble 'narratives' that resemble historical accounts. However, unlike human historians, they lack the capacity for subjective interpretation and cultural context. This leads to a potentially 'flattened' version of history, a collection of events without a nuanced understanding of their broader significance or cultural impact.
Studying how LLMs process information sheds light on human cognitive processes. The algorithms employed to recall information within these models appear to mimic neural pathways in the human brain, suggesting a potential overlap in how memory is structured and recalled. This opens opportunities to understand human memory formation and potentially improve teaching methods through insights gained from AI models.
Anthropology's concept of 'cultural memory', the way societies collectively remember their past through rituals and narratives, has an intriguing parallel in how LLMs learn from their data. This prompts us to consider the potential role of AI in preserving and transmitting knowledge across generations and whether AI-driven 'memories' can truly capture the essence of cultural knowledge.
However, the data used to train LLMs may contain biases reflecting societal prejudices. This parallels how cultural narratives can perpetuate biases in human society. Thus, there's a significant need to critically assess the potential for AI models to inadvertently amplify these biases, similar to debates regarding the responsible use of media and representation.
The relationship between collective memory and individual identity is a long-studied concept in the humanities. The outputs generated by LLMs can shape user perceptions and potentially influence how individuals and groups understand themselves and their place in the world. It's plausible that extensive interaction with AI systems could subtly shift the way humans form beliefs and understand their surroundings.
The ongoing debate about whether LLMs should 'forget' is analogous to philosophical discussions surrounding the role of selective forgetting in human cognition. The idea that LLMs retain everything they learn, without a mechanism for discarding irrelevant or problematic information, presents an interesting contrast to the selective nature of human memory. This opens a broader discussion about accountability and the control humans should exercise over the retention and dissemination of knowledge within these powerful systems.
Ancient memory techniques, like the Greek method of loci, offer valuable insights for improving LLM performance. It's plausible that studying these methods and others could help optimize knowledge retrieval in AI systems. The way humans have traditionally structured information for recall might offer valuable inspiration for future advancements in AI algorithms.
The shift from oral to written traditions significantly altered how knowledge was shared, creating a lasting impact on collective memory. The rapid digitization of information in the current era will likely similarly impact our understanding of the past and the future. How future generations will learn and integrate knowledge in a world saturated with AI-generated data is a critical question that requires ongoing discussion.
Finally, the intersection of memory and philosophical or religious thought, where narratives and myths often play a crucial role in shaping belief systems, finds potential parallels within AI. Exploring how these structures function in human thought might lead to the design of AI systems that integrate storytelling and narrative to foster deeper human engagement and comprehension of intricate ideas.
Rethinking LLM Memorization 7 Key Insights for Entrepreneurs in the AI Era - Entrepreneurial Opportunities in Ethical AI Development
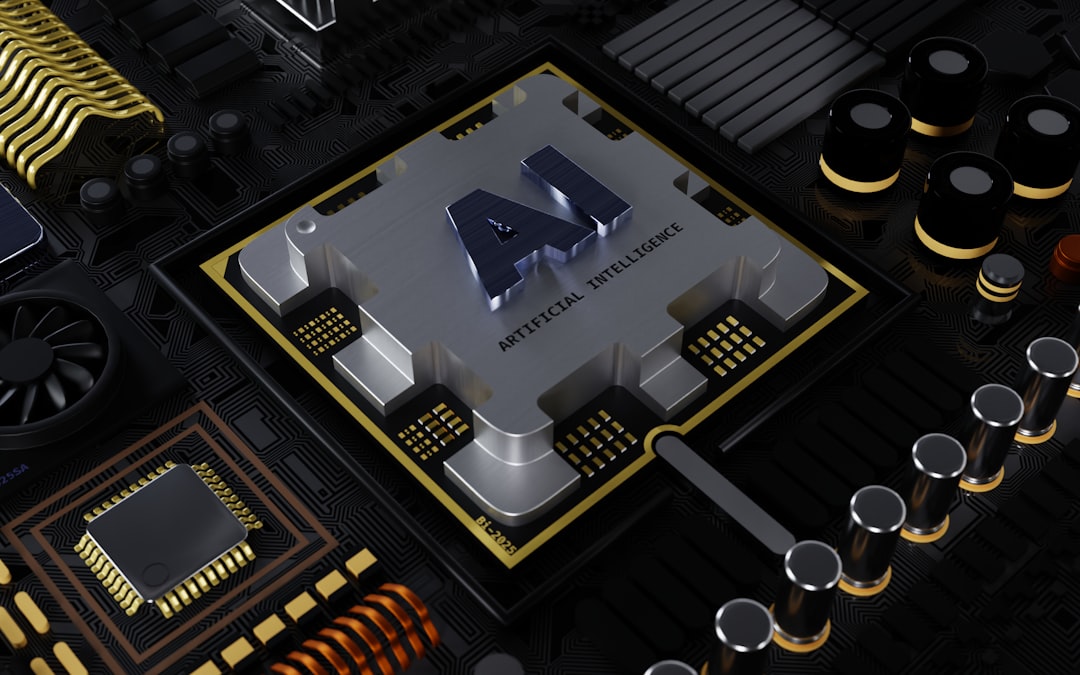
The emergence of generative AI presents a unique set of opportunities and challenges for entrepreneurs, especially in the realm of ethical AI development. While the allure of quick profits exists, entrepreneurs must approach AI development with a keen awareness of the ethical implications their creations carry. This requires prioritizing transparency and accountability, ensuring that the AI solutions they develop are crafted with a focus on benefiting the broader public.
Entrepreneurs can leverage ethical frameworks to guide their innovative ventures, ensuring that they contribute to positive societal outcomes while grappling with crucial issues like bias in AI, misuse of data, and potential workforce disruptions linked to advancing AI. Collaborating with research groups and thought leaders in the field can further empower entrepreneurs to actively participate in shaping the societal impact of AI. This participation can involve pushing for higher standards in AI application, always focusing on principles that center human well-being and values.
Moreover, a commitment to continuous experimentation and iteration is essential for entrepreneurs operating in the dynamic field of AI. By refining their applications through rigorous testing and feedback, they can create AI solutions that are both innovative and ethically sound, navigating the complex landscape of AI responsibly. In essence, entrepreneurship in the era of ethical AI development requires a balanced approach that embraces innovation while being conscious of the potential societal consequences.
The inspiration for many early AI systems, including neural networks, came from understanding how the human brain functions, specifically its memory pathways. This understanding, built upon decades of psychological and neurological research, has led to AI models that mimic human cognitive processes in fascinating ways.
Entrepreneurs in the field of ethical AI development find themselves in a unique position. They often bridge many disciplines, combining technology with insights from diverse fields like philosophy, sociology, and even anthropology. This allows them to grapple with complex questions about data ownership, societal impact, and the potential consequences of their work.
The problem of bias in AI training datasets is, unfortunately, not entirely new. History is filled with biases in art, literature, and even folklore. This realization emphasizes the need for entrepreneurs to engage with historical and cultural contexts when building AI models. They must be aware of the biases inherent in the data and work to mitigate their influence on the AI's output.
We’re increasingly aware that the ability to forget is critical for cognitive health. This notion raises significant questions about AI systems that are built to retain all the information they encounter. If these systems aren't carefully managed, they might perpetuate outdated or harmful biases, simply because they haven't learned to discard irrelevant or problematic data. This is a critical issue for entrepreneurs developing these systems.
Similar to how ancient societies relied on oral traditions, modern AI offers new ways to explore and revitalize forgotten knowledge and narratives from local communities. This offers entrepreneurs possibilities to improve community engagement through the creation of AI-based tools and applications.
The idea of "cultural memory" highlights how societies shape their identities and beliefs based on shared stories. Entrepreneurs engaged in ethical AI development can utilize this concept to craft AI systems that respect and uplift marginalized voices through improved storytelling and other media. This could help to counter the existing imbalance in our existing cultural narratives.
The intriguing blend of memorization and creativity we see in LLMs echoes philosophical discussions about the nature of knowledge that have been pondered in the Western world since the time of Aristotle. These debates explore the fundamental question of whether retrieving information is equivalent to actually understanding it. For entrepreneurs, understanding this philosophical conundrum is crucial to how they view AI's potential for impacting society.
Just as orators in ancient Greece relied on mnemonic techniques to improve their speeches, AI developers can find inspiration in these methods. This suggests that there's potential for the creation of more efficient and user-friendly methods for information retrieval in machine learning systems. Perhaps a closer look at the history of human memory could help in this endeavor.
The rapid advancement of AI technology is pushing us to rethink how we educate people. We might need to move towards more adaptive and interactive learning experiences. This would be similar to the ancient practice of dialectic, where knowledge was exchanged through debates and discussions. This issue is relevant to all entrepreneurs concerned about the future of work and how we prepare people for the jobs of tomorrow.
As AI becomes more integrated into our lives, the philosophical implications of how these systems view and interact with knowledge become more important. This leads to some difficult questions that resemble existentialist philosophy. For entrepreneurs, it's important to carefully consider how AI will shape our beliefs, our sense of self, and societal norms. The more intertwined our lives become with these systems, the greater the responsibility they bear in contributing to human flourishing.
Rethinking LLM Memorization 7 Key Insights for Entrepreneurs in the AI Era - Productivity Challenges in AI-Augmented Workplaces
The rise of AI-powered tools in the workplace presents a complex landscape of productivity challenges for entrepreneurs to navigate. While generative AI promises to streamline operations by handling repetitive tasks, it also introduces disruptions to established routines. Workers may need to adapt to new skill sets, potentially widening the gap between those who readily adopt these technologies and those who struggle to integrate them into their existing work practices. This raises concerns that over-reliance on AI might stifle individual initiative and critical thinking, hindering creativity and innovation within organizations. Furthermore, the ethical considerations surrounding AI, especially when it comes to how it learns, remembers, and utilizes data, necessitate careful examination. The potential for bias and unintended consequences underscores the need to define the boundaries of AI's role in the workplace to avoid a scenario where human ingenuity is overshadowed by technological dependence. For a truly productive future, entrepreneurs must guide the integration of AI in a manner that encourages a synergistic relationship between human potential and technological advancement, creating an environment where innovation and critical thinking are nurtured alongside AI-powered efficiency.
The integration of AI into workplaces, while promising productivity gains, presents a complex set of challenges we're only starting to understand. Research suggests that AI's ability to boost output for skilled workers, while automating routine tasks, may also lead to a sense of cognitive dissonance among employees. Some workers might question their own skills and feel less valued as AI takes on more responsibilities, potentially leading to decreased motivation and job satisfaction.
Furthermore, the introduction of AI into the workplace often doesn't adequately consider the cultural backgrounds and traditions of employees. This oversight can lead to a disconnect between workers and their heritage, impacting engagement and overall productivity. If employees feel their values are being ignored or undermined, they may become less productive and experience reduced morale.
Thinking about memory aids like mnemonics used in ancient Greece, we can see parallels in AI. It's possible that optimizing AI interfaces to better leverage these types of memory structures could be a powerful tool for improving productivity and user engagement. We might have underestimated the potential of such approaches in current AI design.
However, the way we interact with AI systems also has the potential to create echo chambers, where users are consistently presented with similar solutions and information. This reliance on patterns learned from data could hinder creativity and critical thinking, ultimately stifling innovation and impacting the overall productivity of organizations.
Historically, human knowledge has been passed down and expanded through specialized skills and expertise. Yet, in the age of AI, there’s a growing emphasis on generalists, potentially devaluing specific, deep-seated skills. This shift could impact productivity in fields where expertise is vital.
Similar to the transformation brought about by the shift from oral to written traditions, the increasing reliance on AI for knowledge work might dramatically change how we acquire, retain, and pass down knowledge. This transformation will undoubtedly influence how employees engage with information and the very nature of learning itself in a workplace environment.
We've always known that forgetting is crucial for mental health and decision-making. However, in AI systems, the absence of any 'forgetting' mechanism poses a problem. Without the ability to discard outdated or potentially harmful data, AI systems might perpetuate biases and create cluttered knowledge bases that hinder efficiency and decision-making accuracy.
The concept of collective memory, so important in shaping cultural identities, now extends to organizations through the use of AI. If an organization's AI-driven memory doesn't align with an employee's cultural or personal identity, it could result in discord and decreased efficiency.
Workplace productivity is also challenged by the varying levels of digital literacy among employees when interacting with AI. This creates uneven engagement with AI and a potential gap in productivity within teams. Some employees will be able to take full advantage of these new tools while others might struggle to adapt, leading to potential conflict and a less collaborative environment.
Lastly, the power of rituals in religious practices provides an interesting lens. Rituals create strong associations and collective memories, strengthening community ties. We might be able to adapt this concept to improve engagement with AI tools within the workplace. By fostering a sense of ritualized interaction with AI, we could build a stronger sense of community and identity within organizations, fostering a more collaborative and productive workforce.
These challenges emphasize the need for a more nuanced approach to the implementation of AI in the workplace, one that acknowledges the complexities of human psychology, culture, and memory, not just the potential for automating tasks and boosting productivity. Understanding the intersection of these factors is critical as we navigate this new era of AI-augmented work.
Rethinking LLM Memorization 7 Key Insights for Entrepreneurs in the AI Era - Religious and Ethical Considerations for AI Memory Management
The rapid advancement of AI, especially in the realm of large language model (LLM) memory management, compels entrepreneurs to confront crucial religious and ethical questions. How AI systems retain and use information touches upon fundamental ideas about responsibility, fairness, and humanity's influence on technology. These considerations resonate with historical discussions on moral codes and can be explored by looking at how various cultures and faiths view memory, knowledge, and ethical conduct. As AI's presence in society expands, the core challenge becomes ensuring these systems respect human dignity while promoting independence and equity. This calls for a significant rethinking of existing ethical frameworks. Ultimately, open and informed discussions around these concerns can pave the way for more reliable and lasting AI applications in our interconnected global society.
The capacity of AI to retain information indefinitely, unlike humans who naturally forget, presents a unique ethical landscape. Questions surrounding data ownership and the potential misuse of AI's stored knowledge in decision-making processes become paramount. We need robust frameworks to ensure accountability and responsible deployment.
Contrasting human memory, which is deeply intertwined with emotions, context, and personal experiences, with AI's often-mechanical retrieval of data brings the concept of "cultural memory" into focus. Cultural memory, the shared narratives and experiences that bind a society, underscores the difference between human recollection and AI's information processing.
Forgetting plays a pivotal role in human psychology, shaping individual identity and contributing to emotional well-being. However, the absence of a built-in "forgetting" mechanism in AI systems raises concerns. This lack of adaptability might inadvertently perpetuate biases present within training datasets, leading to skewed or problematic outputs. Developing AI systems that can effectively adapt and discard irrelevant information is crucial for mitigating these risks.
Ancient societies employed various mnemonic techniques to enhance memory, providing a valuable historical perspective for the design of modern AI. These methods, used to recall and organize complex information, offer a roadmap for optimizing AI's information processing and retrieval, potentially improving user engagement and overall usability.
Many religious traditions incorporate rituals that serve to solidify group identity and knowledge. By studying the function of these rituals, we can gain insights into how to develop AI systems that foster a sense of community and enhance the collective sharing of information within organizations. This could potentially improve workplace collaboration and communication.
Ancient civilizations relied heavily on oral storytelling and repetition to transmit knowledge across generations. Examining how these traditions functioned can provide valuable lessons for developing AI systems that communicate information effectively. Just as storytelling was a powerful means of knowledge transfer, incorporating narrative structures within AI could improve knowledge dissemination and user comprehension.
Similar to how ingrained biases in cultural narratives can impact human societies, biases in AI training data can lead to skewed or problematic results. We need to critically evaluate the datasets used to train AI, focusing on minimizing any unintentional negative impacts on society.
The introduction of AI into workplaces can disrupt established interpersonal dynamics as employees adapt to new roles. Recognizing and respecting individual employee's cultural and personal identities is crucial for fostering a supportive and productive work environment.
Over-reliance on AI for problem-solving and decision-making might hinder critical thinking and creativity. It's essential to strike a balance between embracing AI's efficiency and encouraging human innovation to maintain a dynamic and adaptable workforce.
The shift towards AI-driven productivity could potentially devalue the importance of specialized expertise in certain fields, favoring a more generalized skillset. This raises important questions about the future role of deep, domain-specific knowledge in an increasingly automated workforce. This could lead to a redefinition of "expertise" across various industries.
Rethinking LLM Memorization 7 Key Insights for Entrepreneurs in the AI Era - Low-Cost Strategies for Implementing AI in Small Businesses
Small businesses facing productivity hurdles can leverage low-cost AI strategies to gain a competitive edge without massive investment. Many readily available and affordable tools, like AI-powered chatbots and data analytics platforms, can significantly improve customer service and decision-making. Instead of a wholesale shift to AI, focusing on areas where quick wins are possible, such as sales and marketing, allows small businesses to build a foundation of AI usage. This incremental approach allows them to refine their application of AI technology over time. By slowly incorporating these technologies, small businesses can avoid the pitfalls of rapid change and maximize the benefits of AI's ability to analyze data, leading to insights that enhance sales and ultimately, growth. In essence, a measured adoption of AI through low-cost strategies can be a powerful driver of productivity and profitability in small business settings.
1. A surprising number of AI tools are free or very affordable, specifically built for smaller companies. This makes it simpler to incorporate AI into day-to-day operations without requiring a huge budget right away. It's a good starting point for experimenting.
2. Chatbots are a popular example of AI already being used by businesses, particularly in customer service. A large percentage of both B2B and B2C businesses have adopted them for fast responses to questions, though some researchers wonder if this is replacing genuine human connection.
3. AI-based analytics platforms are becoming useful for gathering customer data and then figuring out what that data means. Small businesses can use these insights to make better decisions. It's a way to learn more about customers in a data-driven way, but some researchers have concerns about potential privacy violations.
4. When starting with AI, it's wise for smaller businesses to pick a few areas that are easy to measure and track. This lets them build on successes and then add more complex uses of AI technology later. The key here is to not bite off more than you can chew.
5. AI tools can be a game-changer in sales. They can help find potential customers and then focus on the most promising leads. It's a way to enhance prospecting and lead generation but it's still early to know what the long-term effects will be.
6. There's a bit of an art to deploying AI correctly. Entrepreneurs need to be quick about identifying areas where AI would make the biggest difference and also consider how different AI tasks are related, so there's a chance to share the benefits of the technology. This highlights the need for holistic thinking in the adoption process.
7. Free or cheap AI options can give smaller businesses an edge, whether it's marketing, sales, hiring, or everyday operations. The level of competition is rising in the marketplace. It's becoming important to leverage AI where possible.
8. AI can process large datasets incredibly fast, allowing small businesses to get insights that can help them grow and perform better. The sheer amount of data accessible has exploded, and AI is a necessity to sift through it. Some researchers worry that this speed might mean important context and details are ignored.
9. Some commonly used AI tools for small businesses include chatbot software and research assistants powered by AI. They can handle things like chatting with customers and improving content for SEO purposes. These tools make a lot of common tasks easier, but it's important to make sure that the tools are aligned with the values and goals of the organization.
10. With careful planning and strategic investments, small businesses can navigate the world of AI and reap the rewards. It's an evolving technology and companies need to understand how it's changing in order to stay relevant. There's still a lot of unanswered questions about how AI will change society as a whole, but entrepreneurs are already making important decisions about the role AI will play.
Experience error-free AI audio transcription that's faster and cheaper than human transcription and includes speaker recognition by default! (Get started for free)
More Posts from transcribethis.io: