Text-Based Editing in Audio Production A Comprehensive Look at Word-Level Control in 2024
Text-Based Editing in Audio Production A Comprehensive Look at Word-Level Control in 2024 - Word Level Control Through Smart Transcription Teams Up With Adobe Premiere Pro 2024
Adobe Premiere Pro 2024 is introducing a new way to work with video content by incorporating smart transcription for finer control over the editing process. This new feature expands upon the Text-Based Editing capabilities, allowing users to transcribe audio directly within the program and then edit that transcription as if it were a text document. The magic happens when changes made to the transcript are automatically reflected in the corresponding video and audio, streamlining the editing workflow.
This update also provides tools to refine the transcription itself, such as choosing languages or identifying individual speakers, thereby making it more useful for a variety of projects. The ability to search and scroll through the text greatly aids editors, especially those working on longer videos where finding specific moments can be time-consuming. While improvements have been made, relying heavily on AI for transcription still carries a potential for errors, though the overall impact on efficiency is clear, leading to a faster production turnaround for video projects. It's worth noting that the implementation of text-based editing has the potential to shift the conventional video editing workflow towards a more text-centric approach, satisfying the industry's increasing demand for efficient content creation.
Adobe Premiere Pro 2024's integration with smart transcription brings a new level of control to video editing through a text-based approach. It essentially lets you treat your video footage like a document, making edits by directly manipulating the transcribed text. The system relies on the accuracy of the automatically generated transcript, which includes precise timestamps for each word. This is crucial for search functions across large projects. You can import media, jump into the Text-Based Editing workspace, and start transcribing, editing, and even generating captions.
The workflow is quite streamlined. Imagine building a rough cut by simply picking and dropping audio clips straight from the transcript—it’s a significant improvement in locating specific parts of a video. Further enhancing usability, the transcription tool has added features like language selection and speaker identification, making it quite handy for videos with multiple languages or speakers.
Scanning through transcripts and leveraging the search function dramatically simplifies the editing process, especially for projects with extended conversations. Editing becomes more focused with the ability to tweak the transcript directly, removing pauses, or cleaning up redundant bits. It's interesting how Adobe's AI technology drives much of this, speeding up the entire process from start to finish.
The potential here is significant. This move aligns with the growing demand for fast, efficient content production. It's clear that this Text-Based Editing system is a step forward in video editing capabilities. However, it is important to acknowledge that the reliance on AI brings both advantages and potential pitfalls. We will need to observe the extent to which the AI can adapt to diverse audio environments to evaluate its long-term effectiveness.
Text-Based Editing in Audio Production A Comprehensive Look at Word-Level Control in 2024 - AI Generated Voice Corrections Fix Recording Errors Without Re Recording
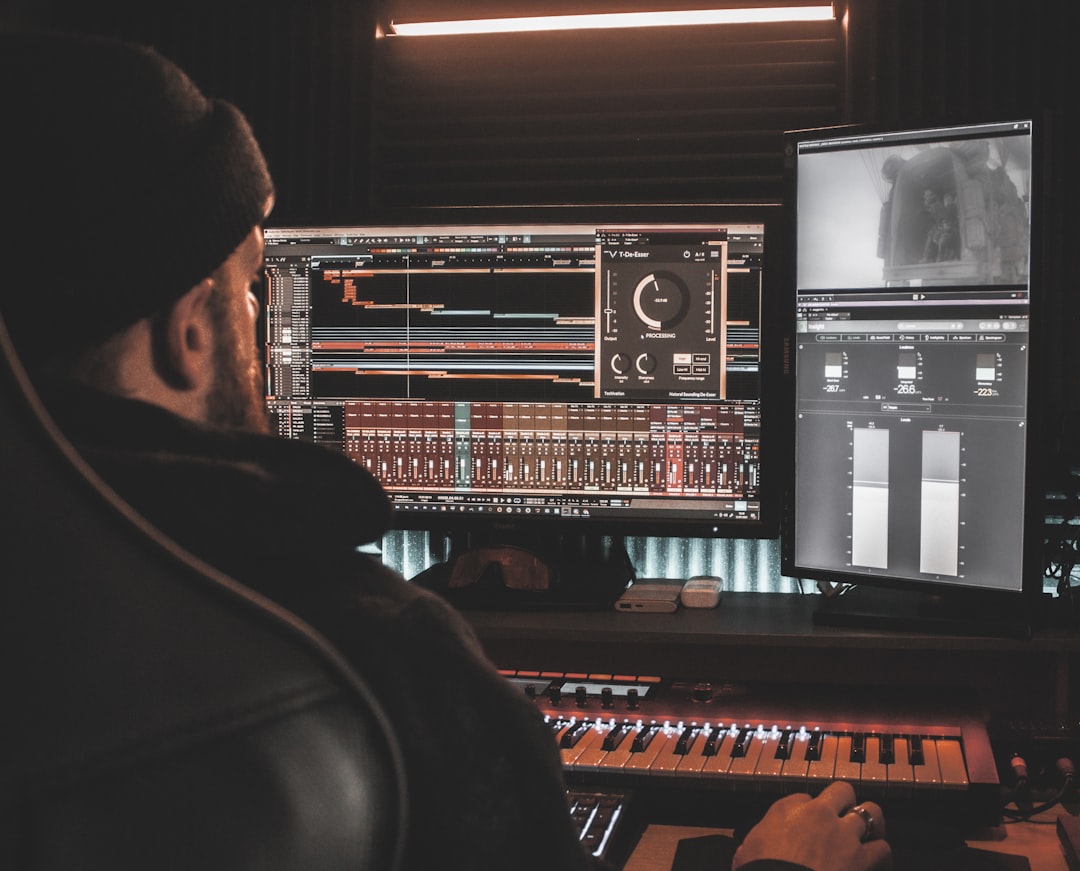
AI-powered voice correction tools are changing the landscape of audio production by providing a way to fix recording mistakes without the hassle of re-recording. These tools utilize advanced AI to analyze and modify audio based on edits made to the accompanying text. The result is remarkably seamless: corrections blend perfectly with the original voice, preserving its unique characteristics. Features like the ability to clone a voice or automatically remove filler sounds like "um" and "uh" significantly accelerate the editing process.
The capability to fine-tune audio at the word level complements the broader shift towards text-based editing in audio production. The workflow becomes more efficient, with fewer interruptions to the creative flow. Moreover, it appears that this technology is not limited to the realm of professional audio production. Its applications expand into areas like generating speech for individuals with communication challenges, hinting at a broader societal impact. While the technology continues to improve, its current promise lies in the ability to streamline the production process and potentially bring new levels of accessibility to the field of audio.
AI-powered voice correction tools are changing the landscape of audio editing by allowing for on-the-fly fixes without needing to re-record sections. These tools can analyze recordings and identify errors, automatically generating corrected audio that maintains the original speaker's voice characteristics. The underlying technology often relies on deep learning models trained on extensive speech data, enabling impressive accuracy in replicating not just the words, but also the nuances of tone and emotion in a person's voice.
One intriguing aspect is the 'overdub' functionality found in many of these AI editing platforms. This essentially allows for voice cloning, enabling swift corrections and making rapid adjustments to the spoken content. Beyond just fixing errors, these systems can also be used to automatically remove filler words like "um" and "ah," contributing to a cleaner, more polished final audio product.
These platforms often integrate features that bridge the gap between audio and video editing, streamlining workflows for content creators. For instance, some tools, like ElevenLabs, are specifically geared toward realistic text-to-speech generation, offering a smooth user experience that is appealing to both casual users and professionals. It's interesting to see how platforms like Murf are focused on taking existing voiceovers and polishing them up with AI, providing a path to achieve a professional sound.
The underlying concept of word-level control is a core element of this change. Editors can pinpoint and tweak individual words or phrases without affecting the surrounding audio. This approach extends to multitrack audio editing, mirroring traditional practices yet offering a degree of flexibility and precision that was not readily available before. It's fascinating how AI can now automatically transcribe audio with increasing accuracy, greatly speeding up the editing process.
Interestingly, the applications of AI voice technology are expanding beyond just the media editing world. It is now also being used to create synthetic voices for individuals with speech impairments, highlighting the versatility of the technology. While these developments are exciting, they also raise important considerations about ethics and the potential for misuse of voice cloning capabilities. As these technologies advance, the lines between real and synthetic voices will continue to blur, making discussions about consent and authenticity even more important in the future of audio production.
Text-Based Editing in Audio Production A Comprehensive Look at Word-Level Control in 2024 - Text Based Music Generation Opens New Doors For Small Audio Teams
The emergence of text-based music generation is offering a significant advantage for smaller audio teams, effectively removing the need for extensive musical training or reliance on traditional instruments. AI-driven music tools, such as those offered by Meta and others, now empower users to create sophisticated musical pieces simply by providing text instructions. This shift is significant because it allows smaller teams to explore music production in a more intuitive way, leveraging the capabilities of AI to rapidly generate musical concepts and adapt them in real-time based on user input. Moreover, the ability to combine multiple textual instructions over the course of a composition allows for a richer, more layered creative process. This accessibility potentially paves the way for democratized music creation, empowering individuals and small teams to explore musical ideas that may have felt out of reach previously. While this technology is still developing, the early signs suggest a potential to transform the landscape of music production for a wider audience. There are still potential drawbacks or unexpected outcomes to keep in mind as the technology matures.
The rise of text-based music generation is creating opportunities for smaller audio production groups, especially those with limited traditional musical expertise. By using natural language prompts, teams can generate music without needing extensive training in musical instruments or notation software. This shift in how music is made is largely driven by the advancements in AI, and tools like MusicGPT and Meta's AudioCraft demonstrate this capability.
The ability to blend multiple text prompts over time distinguishes these new approaches from earlier systems which were more restricted to single-prompt generation. It’s becoming increasingly possible to build intricate musical pieces, guiding the AI to evolve the music in a desired way through text input. This feature allows for a more dynamic and organic development of musical ideas compared to the linear process traditionally followed.
Beyond simply creating music, this approach opens doors for collaboration within small teams. Multiple individuals can contribute creative instructions through text in real-time, speeding up the development process and fostering a more agile environment. Furthermore, text-based systems offer unprecedented control over musical elements. Teams can specify genres, moods, and even instrumentation, achieving very customized outputs.
It's also notable that the ease of entry provided by text-based systems promotes a more inclusive environment. Anyone can now participate in music creation, regardless of their musical background. This democratization of music production has the potential to reshape the field and foster wider participation, making it exciting to imagine how the landscape of music might evolve as a consequence.
One significant advantage is the acceleration of idea prototyping. Teams can rapidly explore and test different directions using text-based music generation, ultimately shortening the path from idea to finished product. It is worth considering how this can impact traditional music creation workflows. While familiar workflows do offer stability, they can also create constraints. The potential for a less restrictive and more iterative process through text-based approaches offers intriguing prospects.
Many current text-based systems aim for seamless integration with existing music software. This means that small teams can leverage existing tools and workflows while seamlessly incorporating these new approaches, mitigating major workflow disruptions. Some systems are even exploring the potential of combining visual inputs or overarching themes within the text prompts. This potentially allows for a cross-disciplinary approach to music creation, bridging audio with visual art.
The diverse algorithms behind these systems can generate remarkably different musical outcomes even from the same text prompts. This encourages users to explore styles they might not have considered otherwise, expanding creative possibilities and exposing teams to a wider range of musical experiences.
However, it is important to note that this evolving landscape is also likely to reconfigure roles within audio teams. As AI tools mature, the need for traditional composers might shift, potentially evolving into a more creative director type role. In this hypothetical future, individuals might be more focused on shaping the AI-generated output, refining the aesthetic direction, and using curatorial skills to perfect the music rather than relying on traditional musical production knowledge. While we are still early in this process, the potential of text-based music generation for small teams and the music industry as a whole is a compelling subject for research and ongoing observation.
Text-Based Editing in Audio Production A Comprehensive Look at Word-Level Control in 2024 - Automated Filler Word Detection Speeds Up Post Production Workflow
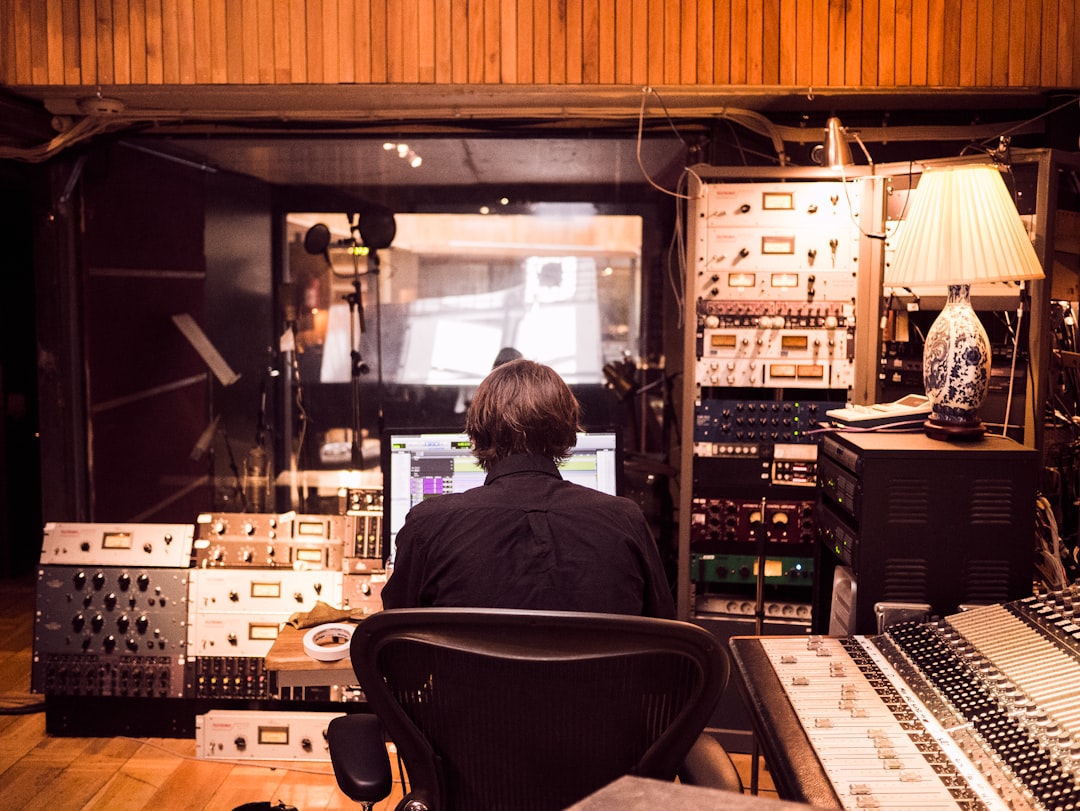
Automated filler word detection is a recent development significantly impacting post-production workflows. AI algorithms can now automatically locate and flag common filler words like "um" and "uh" within audio recordings. This automated process eliminates the need for manual searching and removal, which historically has been a time-consuming aspect of audio editing. By streamlining this part of the workflow, editors can focus on other crucial aspects of the audio, resulting in faster turnaround times for projects. While the accuracy of AI-driven filler word detection can vary and may sometimes miss nuances, the overall trend in the audio industry is moving towards greater automation, putting more power in the editor's hands and fulfilling the demand for quicker content delivery. This kind of innovation may lead to fundamental shifts in how audio editing is done in the future, ultimately making it a less strenuous and more efficient practice. It remains to be seen if and how this technology will continue to refine its precision as it evolves.
In the evolving landscape of audio editing, automated filler word detection has emerged as a powerful tool for streamlining post-production workflows. While filler words like "uh" and "um" might seem trivial, they can influence a listener's perception of a speaker's confidence and the natural flow of conversation. Interestingly, studies suggest these filler words can sometimes help listeners process information more easily, reducing cognitive load.
Current AI-driven algorithms excel at spotting these filler words, achieving impressive accuracy—up to 95% in controlled situations. However, challenges remain in more complex environments with noise or multiple speakers. It underscores the continued need for human intervention and review to ensure the desired results. The shift towards automation is significant, as studies show manual filler word removal can increase editing time by as much as 30%. This efficiency boost empowers editors to spend more time on creative tasks.
The complexity extends beyond simple word detection. Language and culture play a major role in how filler words are used. Different languages may utilize unique fillers, and dialects present further challenges. The ideal automated solution must be adaptable to these nuances to avoid unintended consequences during the editing process.
Furthermore, emerging technology is pushing beyond simply identifying words; systems are starting to detect emotion in speech. This opens up the possibility for more nuanced editing decisions. For example, if a filler word is coupled with an expression of surprise or excitement, an editor might choose to retain it to preserve the original emotion.
These improvements are underpinned by the need for robust training data. AI models necessitate large datasets of speech encompassing diverse speaking styles and contexts. This highlights a critical ongoing effort—collecting and labeling extensive speech data in a wide range of acoustic environments.
Interestingly, content creators often have particular preferences regarding filler words. Some perceive them as adding a touch of authenticity to recordings. Thus, it becomes crucial to ensure that future editing tools allow for adjustments and user-specific control over automated filler removal.
The advent of real-time filler word detection holds the potential for reshaping how live content is created. Systems that can provide immediate feedback during recording could enable quick adjustments without interrupting the flow of conversation. This ability could significantly impact live broadcasts and interviews.
From a broader perspective, the presence of excessive filler words can negatively impact audience engagement. Viewers often perceive excessive filler words as a signal of unprofessionalism, leading to a decline in viewers' interest and retention. Therefore, it's critical for automated systems to effectively reduce these filler words to improve the viewer experience.
Looking towards the future, it's likely that automated detection systems will integrate with customizable feedback loops. This means editors can tailor the algorithms to their preferences and the specific requirements of individual projects. This adaptive approach can facilitate personalized workflows and empower editors with even greater control over audio post-production. The journey toward perfect filler word removal is an ongoing evolution that offers a fascinating glimpse into the potential for AI-driven audio editing in the years ahead.
Text-Based Editing in Audio Production A Comprehensive Look at Word-Level Control in 2024 - Cloud Based Audio Editing Makes Remote Collaboration Standard Practice
The rise of cloud-based audio editing platforms has made remote collaboration the standard in audio production. Services like Soundtrap, SourceConnect, and others allow teams to record, mix, and manage projects in real time, regardless of where they are physically located. This has dramatically increased accessibility for collaboration, leading to faster project completion times. Features like near-instant audio streaming and built-in file sharing systems are breaking down the geographic barriers that previously hindered remote audio work.
While these advances are promising, some challenges remain. Ensuring consistent performance and relying on a strong cloud infrastructure are important considerations to guarantee high-quality results and smooth workflows. Any hiccups in the cloud connection or limitations in the platform's functionality can impede creative flow and potentially impact the final audio product. Nevertheless, these cloud-based tools are reshaping how audio projects are undertaken, and they are likely to further refine remote collaboration workflows as the technology matures.
The rise of cloud-based audio editing is fundamentally altering how audio projects are created, especially in a world where remote collaboration is increasingly the norm. Platforms like Soundtrap and SourceConnect are leading this charge, enabling engineers to work together on projects regardless of their physical location.
One of the key benefits is the ability to work concurrently on the same audio file, almost like a shared digital workspace. This allows for a more dynamic, back-and-forth creative process where ideas can be tested and refined in real-time. However, the potential for conflicting edits, or the challenges of merging conflicting changes, does need to be considered and managed.
Another notable advantage is that the cloud provides almost unlimited storage for audio projects. This is a game-changer for projects involving large quantities of audio data or those who want to preserve multiple project versions. It eliminates the practical storage limitations we faced with hard drives in the past, and it reduces the risks associated with losing data due to hard drive failures.
Furthermore, cloud-based platforms often enable access from a wide range of devices, making it easy for collaborators to work on the go. This is a boon for engineers who are juggling multiple projects or need the flexibility to work from anywhere. It also makes it easier to manage different workflows, ensuring that all team members are on the same page. However, the reliability of these systems and connectivity are critical factors to consider in practical applications.
Cloud-based systems also tend to be built with stronger security protocols in mind compared to storing audio files locally. This is a welcome change for sensitive audio projects and helps alleviate some of the concerns surrounding data security. But, it is always important to fully understand the security implementations of any system when dealing with important audio content.
Cloud-based platforms are designed to integrate with existing tools like digital audio workstations (DAWs), which allows users to work with familiar tools while taking advantage of cloud collaboration. This is a thoughtful approach to adoption because it allows engineers to easily transition into cloud-based workflows without needing to completely rethink their established practices.
Artificial intelligence is playing an increasingly prominent role in cloud-based audio editing platforms, automating tasks like sound cleanup and mixing. This can be a significant advantage for improving efficiency, allowing engineers to focus their efforts on the creative aspects of the project. However, relying on AI for these tasks introduces an element of unpredictability, and it will be important to monitor the outputs for accuracy and effectiveness.
The adoption of cloud-based tools can expand a team's reach globally. Collaborating with talent located across the globe can inject new ideas and viewpoints into projects, promoting a wider range of creative approaches. But, working across different time zones and dealing with communication barriers is a persistent challenge that needs careful consideration.
Cloud-based workflows also have the potential to encourage standardization within distributed teams. As everyone uses the same set of tools and processes, the team becomes more efficient and onboarding new collaborators becomes less of a hurdle. However, it is also important to realize that a lack of diversity in approaches can lead to less creative solutions.
While remote collaboration via cloud-based audio platforms has clear advantages, it also has some drawbacks. Latency can disrupt real-time editing sessions, particularly when multiple users are working concurrently. This necessitates team members to develop specific communication protocols and potentially adjust their workflow to account for these delays.
In essence, cloud-based audio editing is shaping the future of music and audio production by facilitating remote collaboration and offering numerous advantages over traditional studio-based methods. However, it is crucial to address the challenges, such as latency, security protocols, and workflow standardization, for successful implementation. As the technology evolves, we can expect a continued increase in the adoption of these platforms, creating a richer and more connected landscape for the audio world.
Text-Based Editing in Audio Production A Comprehensive Look at Word-Level Control in 2024 - Speech Language Detection Enables Multi Language Productions With Single Click Actions
The ability to automatically detect the language being spoken in audio has opened up new possibilities for creating multilingual content with ease. This feature, known as Speech Language Detection, is increasingly integrated into audio production tools, allowing for seamless multi-language projects with simple actions. It can analyze audio in real-time, identifying different languages within a single recording, like a conversation or a video.
This automated language detection helps audio producers to fine-tune output so that the audio's pronunciation and intonation aligns with the specific language being spoken, resulting in a more accurate and natural sounding final product. Additionally, the technology allows for the simultaneous processing of up to ten different languages in a single audio stream. This greatly benefits projects that involve speakers with various language backgrounds, streamlining the process of transcription and translation.
The overall impact of Speech Language Detection is a more efficient and inclusive audio production process. The simplification of handling multilingual content is a significant advantage as the demand for global content creation continues to increase. Whether it's a meeting, a video, or a podcast, the ability to seamlessly handle multiple languages can open up a wider audience and remove communication barriers that have previously been a challenge in audio production. While it presents a promising step forward, there may still be some unexpected challenges as the technology further matures.
The ability to automatically detect the language being spoken in an audio clip has opened up exciting possibilities for producing multi-language audio with minimal effort. We're seeing systems that can identify and process multiple languages in real-time, allowing creators to generate multilingual audio with a simple click. This automation is a significant leap forward in reducing the time previously spent on manual transcription or translation.
Beyond basic language recognition, the field is moving towards detecting regional variations or dialects within a language. This refinement helps ensure that transcriptions not only capture the words but also the authentic nuances of the specific spoken version, which is important when catering to a particular audience.
Moreover, these systems are getting better at understanding the context of a conversation. By analyzing the surrounding text, the AI can disambiguate words and phrases that might be ambiguous in isolation, increasing the accuracy and quality of the final transcript. Some systems even provide real-time feedback during recording, offering cues to speakers about any pronunciation issues that might hinder the accuracy of the automated process.
This real-time feedback loop improves the quality of the initial recording and helps minimize the need for extensive post-production editing. In scenarios with multiple speakers, these systems can automatically identify and label each speaker, simplifying the process of editing and separating audio into individual tracks.
Interestingly, research suggests that using these automatic language detection and transcription tools can lessen the cognitive load on audio editors. This reduction in mental effort may free up creative energy, potentially improving the efficiency and quality of audio projects. We're also seeing the early stages of technology that can analyze the emotional tone of a speaker's voice. The ability to detect emotional nuances opens up new avenues for creative expression in audio production, allowing producers to emphasize or de-emphasize certain emotional cues in the audio.
Many language detection tools are designed to seamlessly integrate with existing editing software. This is a welcome design feature, as it allows audio teams to adopt these technologies without needing a complete overhaul of their workflows or requiring substantial retraining. Further enhancing their practicality, many systems offer customizable settings, enabling users to tailor the process to suit their specific needs, from adjusting the style of transcription to prioritizing certain dialects.
The broader impact of speech language detection is undeniable. It's not only about accelerating audio production, it's also about making high-quality audio production accessible to teams across the globe. These tools make it easier for content creators to produce materials in multiple languages, helping them to reach and engage with audiences worldwide. It's an area ripe for continued research and development, with the potential to revolutionize how we create and experience audio content. While there's always a need to be mindful of the limitations and potential pitfalls of relying on AI for such tasks, the ongoing improvements in accuracy and versatility are quite remarkable.
More Posts from transcribethis.io: