Experience error-free AI audio transcription that's faster and cheaper than human transcription and includes speaker recognition by default! (Get started for free)
AI Upscaling Meets Audio Exploring Meta's Audiobox for Enhanced Video Sound Quality
AI Upscaling Meets Audio Exploring Meta's Audiobox for Enhanced Video Sound Quality - Meta's Audiobox Unveils Natural Language Audio Generation
Meta's Audiobox is a new AI model designed to generate audio from text and voice prompts. It can create everything from voices to sound effects, catering to a wide array of audio production scenarios. The model's performance is notable, achieving strong results in text-to-speech and text-to-sound tasks when compared to other models. A key feature is its use of Bespoke Solvers, a technology that drastically speeds up audio creation compared to traditional methods. Furthermore, Audiobox offers flexible control over audio styles through natural language prompts, in addition to traditional voice prompts. This allows users to finely tune the output audio for specific needs. The model is built for adaptability, allowing developers to customize it for different applications. While still in testing, Audiobox has demonstrated the ability to seamlessly blend speech and sound generation, a feature particularly promising for video productions looking to improve audio quality. This, in turn, hints at a future where a more holistic approach to audio creation might be possible.
Meta's recently unveiled Audiobox is a foundational AI model built for audio creation. It's quite impressive in its ability to generate both speech and sound effects based on text instructions and even voice inputs. The model's flexibility allows for a wide range of applications, suggesting it could be useful for tailoring audio to specific contexts.
Audiobox's performance, as measured by standard benchmarks, is noteworthy. For example, its text-to-speech (TTS) capabilities demonstrate a high level of accuracy in replicating human speech. Similarly, in generating sounds based on text descriptions, it achieves a respectable level of performance. The developers have introduced something called "Bespoke Solvers", which significantly speeds up the audio generation process compared to traditional methods, potentially making it a more efficient tool for audio production.
Audiobox comes in a few specialized versions, focusing either on speech or broader sound generation, indicating a modular approach to developing its capabilities. What's particularly interesting is the ability to combine natural language instructions with voice prompts to influence the generated audio, giving creators more precise control over style and speech characteristics. Furthermore, the model's architecture allows it to be adapted for a range of audio generation tasks.
It seems Meta is committed to making Audiobox accessible. It's already open for public testing, allowing researchers and potentially creative users to interact with it. This move provides valuable feedback for refining the technology. Essentially, Audiobox aims to seamlessly integrate sound and speech generation, moving beyond simply generating voice to a more unified approach to audio creation.
The long-term implications of Audiobox for content creation, especially video content where audio quality plays a crucial role, are potentially profound. However, like any advanced AI system, there are limitations to overcome. There's still room for improvement in addressing diverse accents and dialects. Ongoing development will likely focus on refining these aspects to make the generated audio even more natural and versatile.
AI Upscaling Meets Audio Exploring Meta's Audiobox for Enhanced Video Sound Quality - Realistic Soundscape Creation for Video Enhancement
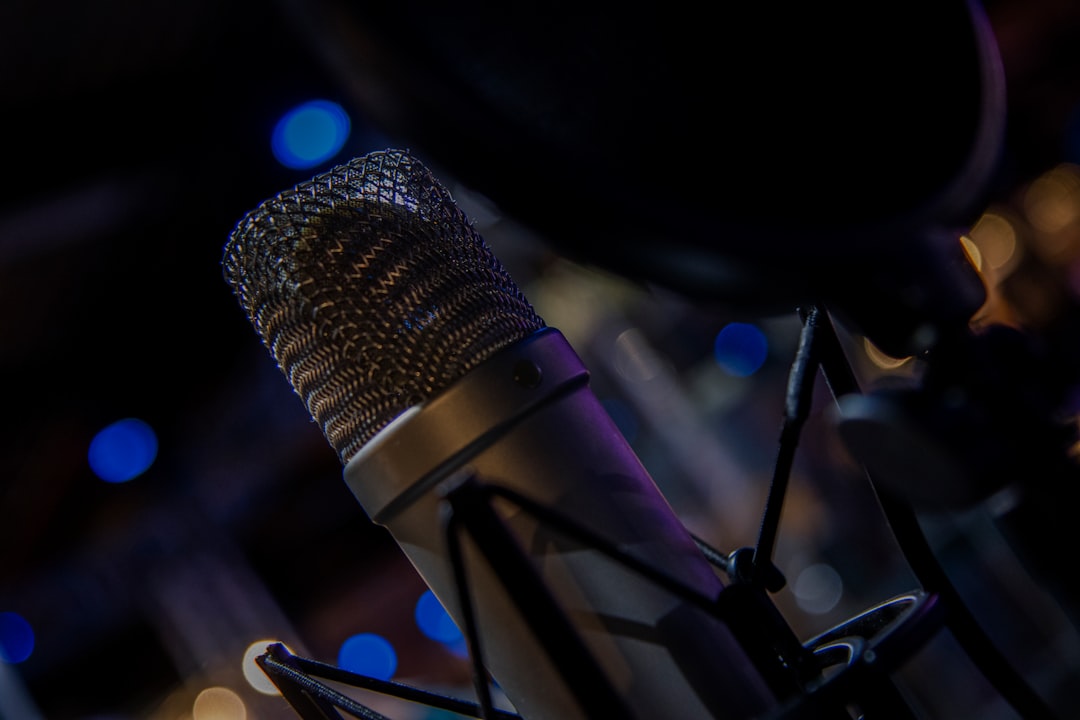
Improving the audio in videos is becoming increasingly important, and AI is playing a key role in this development. Tools like Meta's Audiobox are now capable of creating incredibly realistic soundscapes that go beyond simple background noise. This opens up possibilities for a much more immersive viewing experience, as the audio can be perfectly synchronized with the visuals, creating a more believable and engaging world within the video.
The ability to generate specific sounds or ambient noises with AI empowers creators to shape the viewer's perception and emotional response. Imagine enhancing a historical documentary with the authentic sounds of a bustling marketplace or adding the roar of a crowd to a sports highlight reel. While these AI-driven improvements to audio are promising, they also raise questions. Can these technologies truly replicate the nuances of human speech and diverse accents across various languages?
The ongoing evolution of AI-powered audio generation presents a fascinating intersection of creativity and technology. While the potential for more captivating and realistic video experiences is clear, the path to fully leveraging the potential of AI-generated soundscapes will likely involve continuous refinement and addressing certain limitations. It's important to critically evaluate both the opportunities and challenges this development presents as the technology matures.
Creating realistic soundscapes is vital for enhancing the impact of video content. Our brains don't just process sound based on its loudness and pitch; we interpret it within a context, making soundscapes crucial for establishing mood and atmosphere. Techniques like binaural audio, which uses two microphones to mimic how we hear in 3D, can make a video's sound environment more immersive and believable.
The Doppler effect, the change in pitch as a sound source moves towards or away from us, can be simulated to add a layer of realism, particularly during action sequences where movement is prominent. Psychoacoustics, the study of how we perceive sound, is a valuable tool for sound designers to manipulate things like volume and pitch to evoke specific emotional responses.
It's been shown that even ambient sounds, things like wind or background chatter, can dramatically improve the sense of realism in a video. Including carefully crafted ambient noise can help to pull viewers into the world of the video, adding depth to the overall experience.
Meta's Audiobox, which uses machine learning techniques, is a promising example of AI tackling audio generation. It learns from massive audio datasets, resulting in high-quality and contextually appropriate audio output. Neural network models within Audiobox are continually being refined and are able to adapt to produce sound that fits seamlessly with visual elements.
Interestingly, studies suggest that the careful design of a soundscape can actually influence how a viewer interprets a story. This highlights the critical role sound design has in affecting narrative perception. Sound localization techniques, such as adjusting sound levels and timing, can create the illusion of sound coming from specific locations within a scene. This is particularly beneficial in enhancing the viewer's understanding of the spatial relationships between various parts of the video.
Specific sound effects play a significant role in realism. The crunch of footsteps on gravel or the rustle of leaves—these can significantly enhance the authenticity of a video, often engaging viewers subconsciously and building tension. Audiobox's capacity to allow real-time adjustments and user feedback establishes a dynamic creative environment for audio enhancement. This continuous refinement process offers a potential pathway towards unprecedented levels of audio accuracy and relevance, particularly within video enhancement workflows. While exciting, there are many challenges and limitations in making truly realistic soundscapes. However, there is a lot of potential for AI to significantly push the boundaries of soundscape creation for video.
AI Upscaling Meets Audio Exploring Meta's Audiobox for Enhanced Video Sound Quality - Unified Approach Integrates Speech and Sound Generation
Meta's Audiobox presents a novel approach to audio creation by combining speech and sound generation into a single framework. This unified system allows users to describe the desired audio output using natural language, leading to the creation of rich and detailed soundscapes. A key aspect of Audiobox is its ability to bridge the gap between traditional text-to-speech and broader sound generation. It leverages a technique called "flowmatching" to offer increased control over the audio output, enabling fine-tuning of various aspects of sound. Moreover, Audiobox's ability to disentangle speech control gives creators the capacity to tailor generated voices with specific accents, emotional tones, and intonations. This feature, coupled with natural language prompting, gives users fine-grained control over the nuances of generated speech. The integration of speech and sound within a single model could significantly impact fields like video production, potentially revolutionizing how audio is produced and incorporated into various media formats. While promising, it remains to be seen how well this new approach will translate into the complex and nuanced demands of real-world audio production.
Meta's Audiobox presents an intriguing approach to audio generation, unifying speech and sound creation within a single framework. This unified model is built on the idea that treating speech and sound effects as distinct elements isn't ideal, and that blending them allows for more coherent and realistic audio outcomes, especially beneficial for enhancing video content.
The model's architecture allows for real-time adjustments to audio traits. This adaptive quality fosters a dynamic interaction between the user and the system, enabling a more responsive approach to sound design and refining the output audio. The potential impact extends beyond video; we can envision Audiobox's influence on gaming and virtual reality, enabling context-aware soundscapes that respond to events within those interactive spaces.
Audiobox aims to reproduce the fine nuances of human speech, capturing tonal shifts and emotional cues. This level of detail is exciting as it hints at future potential in animated productions and video games, hopefully creating interactions that feel more genuine to viewers. Utilizing principles from psychoacoustics, Audiobox strives to generate audio environments that elicit specific emotional reactions in listeners. This is potentially powerful, offering content creators another avenue to influence viewer engagement through auditory triggers.
The model's training leverages a broad collection of audio data, covering diverse sounds, voices, and contexts. This diverse learning process is essential for generating audio that's not just realistic but also relevant to the story or situation being portrayed. Furthermore, the model has incorporated sound localization, allowing for the realistic impression of audio coming from different points within a scene. This fosters a sense of depth and immersion in viewers.
While promising, Audiobox still faces hurdles in its ability to handle the subtleties of diverse accents and dialects. This indicates areas where language processing technologies can be improved. Audiobox utilizes neural synthesis methods to craft not only speech but a range of sound effects, responding to both text and voice input prompts. This combined input offers a more user-friendly experience for creators.
The incorporation of AI into sound generation raises questions about the evolution of the sound design profession. As AI assumes more of the creative burden, the role of human sound designers may change, perhaps shifting towards higher-level creative direction while leveraging the speed and efficiency of machine-driven production. This evolving creative collaboration will certainly impact the entire sound design landscape. It's exciting to speculate on this emerging intersection of human ingenuity and AI-powered sound creation.
AI Upscaling Meets Audio Exploring Meta's Audiobox for Enhanced Video Sound Quality - Audiobox Achieves High Scores in TTS and Text-to-Sound Tasks
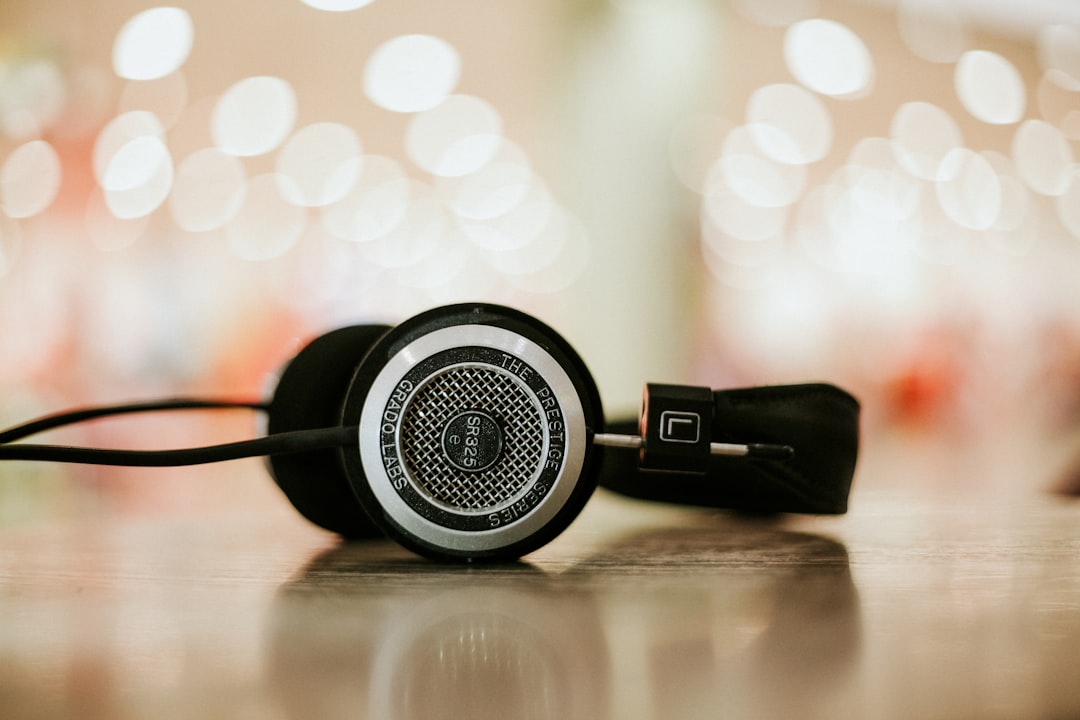
Meta's Audiobox stands out in the field of AI-driven audio generation, demonstrating impressive results in tasks like converting text to speech (TTS) and generating sounds based on written descriptions. It's scored well on standard tests, showing a strong ability to produce human-like speech in TTS scenarios and convincingly creating sounds from text instructions. A key part of Audiobox is its use of bespoke solvers, which significantly speed up the process of audio creation compared to traditional techniques—a speed increase of over 25 times. This speed advantage, along with the ability to finely control the audio output using text prompts, gives Audiobox the potential to create highly customized soundscapes. The model's unified approach aims to combine speech and sound generation, suggesting it could revolutionize audio production in a variety of settings. However, Audiobox still faces challenges in replicating the complexities of real-world sounds, including handling different accents and nuances in emotional expression. It remains to be seen how effectively it can handle these details moving forward.
Meta's Audiobox, a research model focused on audio generation, has demonstrated strong performance in a variety of tasks, particularly in text-to-speech (TTS) and generating sounds based on text descriptions. It achieves impressive results on established benchmarks, suggesting that it's quite good at creating audio that sounds similar to human speech.
One of the interesting features of Audiobox is the use of Bespoke Solvers, a technology that significantly speeds up the audio generation process. This speed improvement could potentially revolutionize how audio is produced, particularly in media workflows where time is critical. Audiobox isn't limited to simple voice generation. It can understand and respond to natural language prompts, which allows for a lot more control over the output audio. Users can specify not just what sound they want but also the desired emotional tone, creating audio that is more nuanced and contextually relevant.
The model incorporates a method called flowmatching which gives creators a good deal of flexibility in adjusting the dynamics of the generated audio, including its timing, intensity, and overall tonal characteristics. The ability to influence the emotional tone of generated speech hints at potential applications in gaming and animation, where more realistic and emotionally engaging interactions are desired.
Audiobox attempts to overcome some of the challenges in traditional audio creation by combining speech and sound generation into a single system. By not treating these elements as separate entities, the goal is to create more coherent and natural-sounding audio. The researchers have trained the model on a wide variety of audio data, covering a wide range of sound sources, voices, and contexts, aiming to ensure the generated audio is diverse and appropriate. It's also capable of simulating sound coming from different parts of a scene, improving the sense of space for listeners.
In the future, it will be interesting to see how AI models like Audiobox continue to evolve and reshape sound design workflows. While users have the capability to make adjustments in real-time, it will be important to see how well Audiobox adapts to subtle variations like accents and dialects. As the technology matures, it's likely that the roles of human sound designers will evolve, possibly focusing more on creative direction and conceptualization, rather than the technical aspects of audio generation. All in all, Audiobox presents a promising approach to audio generation, particularly for video content, although the extent to which it truly addresses the nuances of real-world audio production remains to be seen.
AI Upscaling Meets Audio Exploring Meta's Audiobox for Enhanced Video Sound Quality - Bespoke Solvers Accelerate Audio Generation Process
Meta's Audiobox relies on a novel approach called Bespoke Solvers to significantly speed up audio generation. These solvers dramatically cut the time it takes to create audio, improving efficiency by over 25 times compared to traditional methods. This allows Audiobox to produce high-quality audio, including voices and sound effects, much faster. This speed is particularly helpful when creating audio that needs to align with specific needs or styles. While the solvers enhance control over audio output and foster rich, detailed sounds, it's still uncertain how well Audiobox handles a wide range of accents and emotional expressions in speech. Moving forward, the ability of Audiobox to capture the subtleties of human speech and other complex audio phenomena will be vital for its widespread adoption and impact on the audio creation landscape. The success of Audiobox ultimately hinges on how well it addresses these remaining challenges, and whether it can truly revolutionize the standards of audio production in various fields.
Meta's Audiobox utilizes a novel approach to speed up audio generation by integrating what they call "Bespoke Solvers." This innovation leads to a substantial increase in speed, over 25 times faster than standard methods. It's interesting to see how these custom solvers impact audio production pipelines.
Audiobox also utilizes a technique referred to as "flowmatching," which allows for precise control over the nuances of audio. Users can adjust factors such as timing and intensity with more accuracy than previously possible, allowing for greater control over the final audio output's tonal characteristics.
One of the key aspects of Audiobox is its unified approach to audio generation. Rather than treating speech and sound effects as separate processes, the model combines them into a single framework. The idea seems to be that this holistic approach could lead to audio that's more naturally integrated with visual content.
Furthermore, Audiobox continually learns and adapts based on a huge amount of audio data. It can generate audio that's contextually appropriate for different situations and emotions, which is a key factor in realistic audio creation. This adaptive learning ability is what makes the technology adaptable to various applications and audio creation scenarios.
The capability to infuse generated speech with emotional nuance is intriguing. It suggests that it could potentially improve the realism of interactions in animated content or video games by making the audio output feel more genuine.
Audiobox leverages natural language instructions as input. This means that users can guide the audio generation process using simple language, making the model more accessible to a wider range of creators without needing extensive technical skills.
The inclusion of sound localization techniques enhances the sense of immersion for listeners. The system can create the illusion that sounds are coming from various positions within a scene, adding depth and realism to soundscapes.
The breadth of training data is clearly important to the model's success. Audiobox was trained on a wide array of audio, including various voices, contexts, and sound effects. This allows the model to generate audio that's suited to a diversity of situations, attempting to capture the complexity of real-world audio environments.
However, despite the progress, Audiobox faces limitations in its ability to reproduce the subtlety of accents and emotional expressions within speech. This points to opportunities for improvement in language processing within the model.
As AI-powered audio generation tools like Audiobox continue to evolve, it's likely the role of sound designers will also shift. They may move towards roles that emphasize creative direction and oversight, relying on these tools to handle the technical aspects of audio creation. It's a fascinating time to consider the changing landscape of sound design as AI technologies become increasingly sophisticated.
AI Upscaling Meets Audio Exploring Meta's Audiobox for Enhanced Video Sound Quality - Disentangled Speech Control Enables Customized Voice Manipulation
Meta's Audiobox incorporates a feature called "Disentangled Speech Control," which represents a notable development in the field of customized voice manipulation. This technique allows for the separation of different components within speech, like the content of what's being said and the speaker's characteristics. This means that developers and creators can now fine-tune the voice generated by the AI model to a remarkable degree, including aspects like accent, emotional tone, and inflection. The potential for crafting highly individualized voice experiences across various fields, such as video editing or interactive media, is significant.
While this level of control is undeniably impressive, it's important to acknowledge that the ability to completely replicate the richness and subtle variations present in human speech is still a work in progress. Challenges remain in fully capturing the nuances of diverse accents, dialects, and emotional expression. Further development is needed to perfect AI's ability to mimic these complex aspects of human communication. The pursuit of such advanced control over voice generation holds promise for creating more immersive and expressive audio experiences that closely align with the intricacies of human speech.
Meta's Audiobox employs a technique called flowmatching, allowing for precise control over the generated audio's characteristics like timing, intensity, and tone. This control offers more nuanced audio outputs, which can be crucial for achieving desired moods or effects. It's intriguing how effectively this technique allows for refining audio.
One of the interesting features in Audiobox is what's called "disentangled speech control". This lets users independently modify aspects of generated speech like accents and emotional tones, which is helpful for aligning the audio output with specific characters or narrative elements. It raises the question of whether it can handle all the subtle variations in how humans speak.
Audiobox is built for real-time adjustments, creating an interactive feedback loop. Creators can refine the audio on the fly based on instant feedback. This real-time refinement could streamline the audio production process, but it remains to be seen if it truly addresses the challenges of audio creation.
The core of Audiobox lies in advanced neural networks trained on a diverse range of audio data. This adaptability is crucial for ensuring the system can produce contextually relevant and accurate audio in various situations and scenarios. However, its reliance on such a large dataset could potentially be a point of concern in regards to the source of that data and bias that might be present.
A key feature of Audiobox is its attempt to combine speech generation with the creation of sound effects within a single system. This unified approach suggests the potential for more harmonious and coherent audio outputs, particularly beneficial for video and interactive media. Though whether this approach is genuinely superior remains to be investigated more thoroughly.
A noticeable feature is Audiobox's use of natural language for input. This contrasts with many traditional audio production tools which often require technical expertise, potentially democratizing audio creation. While this is a promising direction, it raises questions about whether the language model underlying this natural language input could introduce unexpected biases in the generated audio.
The Audiobox model incorporates sound localization features to mimic how sounds are perceived in three dimensions. This approach helps enhance the realism and immersiveness of the audio output for viewers. Though, how well the model truly reproduces the complex ways we perceive sound within a space could be further scrutinized.
To design audio that evokes particular emotions in the listener, Audiobox relies on psychoacoustic principles. This scientifically informed approach has the potential to significantly enhance viewer engagement. However, it's unclear the extent to which it can consistently generate a desired emotion in various listeners.
Audiobox employs bespoke solvers to dramatically accelerate audio creation compared to conventional techniques. This speed improvement is a significant step forward, potentially revolutionizing audio production workflows and saving substantial time. But it remains unclear how this might impact the overall quality of audio or the fidelity of the generated sounds.
While Audiobox has numerous capabilities, replicating the subtle variations in human speech, particularly diverse accents and nuances of emotion, still poses a challenge. It's crucial that developers address these challenges to maximize the model's effectiveness across diverse use cases. Whether these challenges can be completely addressed is not clear at this time, but is a crucial point that should be actively investigated going forward.
Experience error-free AI audio transcription that's faster and cheaper than human transcription and includes speaker recognition by default! (Get started for free)
More Posts from transcribethis.io: