Experience error-free AI audio transcription that's faster and cheaper than human transcription and includes speaker recognition by default! (Get started for free)
How Video File Size Compression Affects Transcription Accuracy in 2024
How Video File Size Compression Affects Transcription Accuracy in 2024 - The impact of lossy compression on speech recognition
Lossy compression techniques, which permanently eliminate audio data to reduce file sizes, significantly impact the performance of speech recognition systems. These methods can achieve impressive compression rates, sometimes as high as 10 to 1, but this comes at a cost: noticeable degradation in audio quality. This deterioration can make it more challenging for automatic speech recognition (ASR) systems to accurately interpret the spoken words.
The impact of lossy compression isn't uniform across all codecs. Different methods of compression affect transcription accuracy in varying ways, underscoring the need to carefully consider the codec when aiming for high-quality transcription. Recent research suggests that generative compression approaches might provide a promising alternative. These techniques enable considerable file size reduction with minimal perceived loss in audio quality as judged by machine learning models, suggesting a potential avenue for improving the robustness of transcriptions in compressed audio.
Looking ahead, the ongoing pursuit of lossless or near-lossless compression algorithms could potentially eliminate the negative effects of lossy compression altogether. This may pave the way for more accurate and reliable speech recognition in the future, particularly in contexts where audio compression is unavoidable.
Lossy compression methods, such as MP3 or AAC, achieve substantial file size reductions by discarding certain audio data. This can unfortunately eliminate frequency components essential for clear speech recognition, particularly in situations with background noise or competing sounds. Even subtle changes, like a 10% decrease in the audio sampling rate, can introduce distortions that confuse speech recognition algorithms, ultimately leading to a higher number of errors and misunderstandings of the spoken words.
The effect of compression isn't universal across all languages. Languages that rely heavily on tone or pitch, for instance, might be disproportionately affected as the compression process might fail to preserve the nuanced pitch patterns crucial for their meaning. Moreover, some lossy compression formats emphasize certain audio characteristics over speech, inadvertently boosting background noise to the detriment of the desired speech. This can hinder the speech recognition system's ability to accurately identify and process the intended spoken content.
There's also evidence that compression can cause certain speech sounds to blend together, leading to a loss of unique characteristics needed for accurate transcription. It can even create masking effects, where loud sounds obscure quieter speech, hindering the clarity of crucial phonetic cues used for accurate transcription. The way audio compression alters the temporal resolution of audio, a vital component for differentiating rapid changes in speech and noise, further complicates the issue. This issue highlights an interesting difference: how humans perceive compressed audio might be different than how speech recognition algorithms interpret it, thus presenting more difficulties for AI models trained on compressed audio data.
We've seen that real-time speech recognition systems, like those used in online meetings or video conferencing, experience performance drops when presented with compressed audio streams, especially when methods like dynamic range compression are used in addition to lossy formats. It seems that the degree of compression is pivotal. Overly aggressive compression introduces distortion and artifacts that not only impact the quality of audio but can also make it more challenging to effectively train machine learning models designed for speech recognition tasks. The interplay between compression choice and the learning process of these algorithms is something that warrants ongoing investigation.
How Video File Size Compression Affects Transcription Accuracy in 2024 - Optimal bitrate for maintaining transcription quality
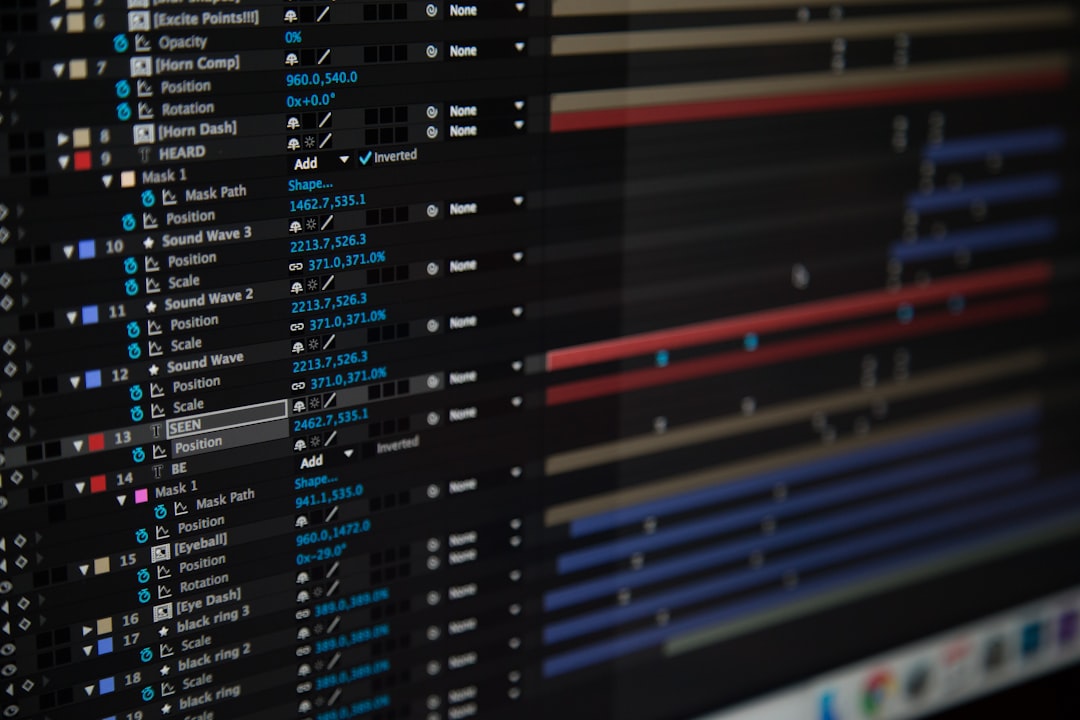
The quality of a video's transcription hinges on the bitrate used during encoding. A higher bitrate means more data is included per second, potentially leading to better audio fidelity and ultimately, improved transcription accuracy. Finding the sweet spot between file size and audio quality is key. For a standard 1080p video at 30 frames per second, a bitrate around 4000 kilobits per second (Kbps) is often a good starting point. However, lower bitrates, while decreasing file sizes, can compromise the nuances of audio. These losses can lead to audio artifacts and a higher likelihood of errors during transcription.
Optimal bitrate selection involves a balancing act. Factors like the resolution, frame rate, and complexity of the content all play a part in determining the ideal bitrate for a specific video. It's a delicate interplay between the desire for manageable file sizes and the need for clear, undistorted audio that allows for accurate transcription. Overlooking this critical step can negatively impact the quality of automated transcriptions, highlighting its significance in the video creation process.
The quality of audio transcription is closely tied to the bitrate of the video or audio file. Research indicates that maintaining a bitrate of at least 128 kbps for audio significantly helps transcription accuracy, as it preserves the necessary audio detail for speech recognition systems (SRS) to operate effectively. Falling below this threshold can result in a noticeable reduction in clarity, making transcription more difficult.
The connection between bitrate and transcription quality isn't a simple linear relationship. A small increase in bitrate can yield a disproportionately larger improvement in clarity, especially in complex audio environments with background noise. This non-linear behavior is a noteworthy aspect of audio encoding.
Interestingly, some high-performance audio codecs, like Opus, feature variable bitrate capabilities. They dynamically adjust the bitrate in real-time based on the complexity of the audio. This characteristic allows them to maintain quality during sections with dense speech while adapting to quieter segments without excessive data usage.
SRS may struggle with specific frequency ranges that are vital for recognizing distinct sounds (phonemes). Maintaining a balanced frequency response, usually attainable with higher bitrates, is essential for accurately capturing these subtle sound details.
It's also intriguing that some older codecs like Speex were designed to prioritize human speech frequencies. This kind of specialization might make them perform better for ASR even at lower bitrates, suggesting a potentially valuable area for improvement in transcription.
Higher bitrates also reduce the appearance of compression artifacts. At very low bitrates, transcriptions often suffer from a "smearing" effect where successive sounds become distorted and harder to distinguish, hindering accurate transcription.
The nuances of certain languages or dialects, especially those with complex tonal variations, often require higher bitrates to maintain their specific characteristics. This implies that a flexible approach to bitrate selection based on the language being transcribed may be beneficial.
Furthermore, the audio sample rate can interact with bitrate to impact transcription quality. A higher sample rate (like 48 kHz instead of 44.1 kHz) combined with an optimal bitrate can capture finer audio details crucial for accurate transcription.
Emerging research explores the use of machine learning methods to dynamically optimize bitrate during live transcription. This innovative approach could lead to a better balance between quality and file size in real-time applications.
The type of content being transcribed also has a substantial effect. Transcription of complex topics with technical terminology or dense speech may require a higher bitrate compared to casual conversations. This suggests that a flexible strategy for managing bitrate, allowing it to adapt to varying transcription needs, may be desirable.
How Video File Size Compression Affects Transcription Accuracy in 2024 - How 32kbps compression affects transcription results
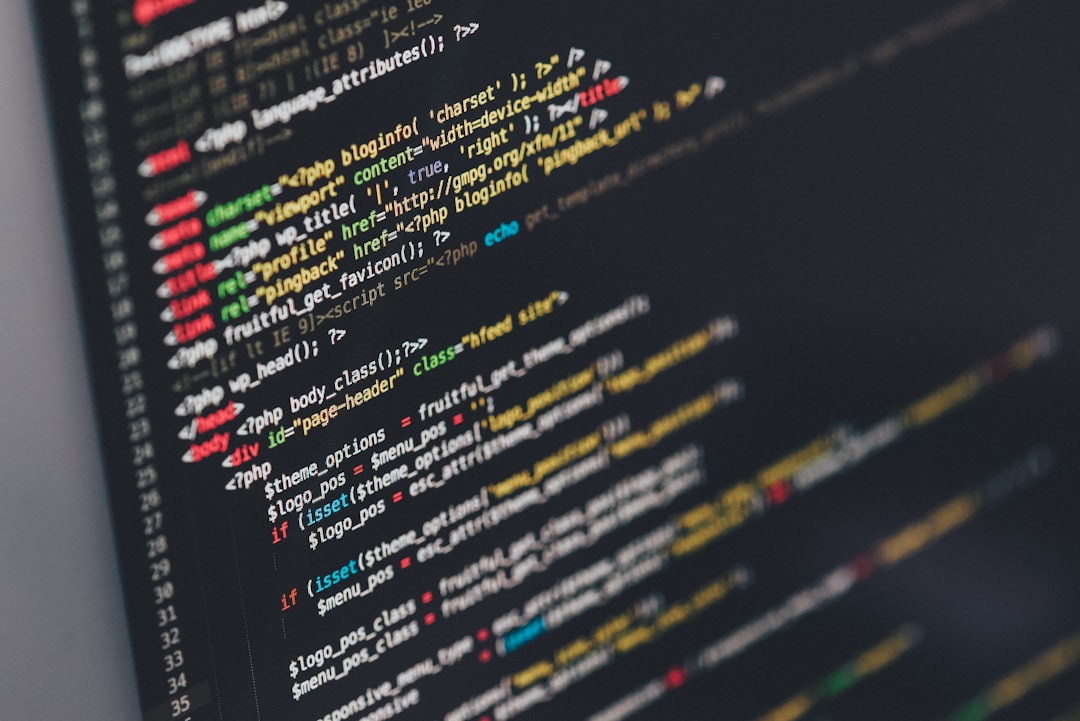
When audio is compressed down to 32kbps, the transcription accuracy suffers considerably. This is largely due to the nature of lossy compression, which inevitably discards audio information to achieve smaller file sizes. Unfortunately, this reduction in data can eliminate subtle but crucial aspects of speech, making it harder for systems designed to understand human language to do their job properly. Things like specific frequencies essential to discerning different sounds are often lost at this low level. Furthermore, it can lead to the introduction of distortions and blurring, effectively making it more difficult to differentiate between individual speech sounds. For better accuracy in transcriptions, aiming for at least 64kbps is typically recommended, as that level maintains a more comprehensive level of audio detail that is helpful for speech recognition. This knowledge is vital for anyone relying on transcriptions of compressed video or audio.
When audio is compressed down to 32 kbps, we start to see a noticeable impact on transcription accuracy. This is primarily due to the nature of lossy compression, where certain audio information is discarded to reduce file size. One key issue is the tendency to prioritize more prominent frequencies, potentially resulting in the loss of finer phonetic details crucial for accurate transcription, particularly in complex soundscapes.
We often observe that compressed audio at this rate can cause speech sounds to blur together, a phenomenon sometimes referred to as "smearing." This can obscure unique phonetic elements that are essential for speech recognition systems to differentiate words accurately.
Counterintuitively, 32 kbps compression can actually increase the prominence of background noise. This can be problematic because it can mask quieter or softer speech, creating a challenge for accurate transcription in noisy environments.
Furthermore, some languages rely heavily on distinct frequency patterns for meaning. If these frequency patterns get distorted by 32 kbps compression, it can make transcription significantly more difficult, especially for tonal languages. It's important to remember that while some audio at this bitrate may still be intelligible to humans, it introduces subtle distortion, especially during rapid speech, leading to potentially higher errors during automated transcription.
A significant problem with very low bitrates like 32 kbps is that they often result in the loss of critical sound frequencies. These frequencies, which are often filtered out because they're deemed less "important," are in fact crucial for conveying subtle nuances in speech. This makes it harder for speech recognition algorithms to fully understand a speaker's intent.
Individual vocal characteristics, including accents or speech patterns, can be lost at this level of compression. This means speech recognition models struggle to adapt to different speaking styles, leading to a decline in accuracy.
We've also found that transcription services can experience delays when processing highly compressed audio. This is likely because speech recognition algorithms find it more challenging to process and understand lower quality audio in real time.
Training machine learning models on this low-quality data can lead to models that don't perform well when presented with higher quality audio. This can create a disconnect where a system's ability to transcribe is dependent on a specific bitrate level, limiting the system's broader usefulness.
It's also worth considering that instead of a fixed bitrate like 32 kbps, a more adaptive approach might be beneficial. Using a variable bitrate, where the compression level changes based on the audio complexity, could be a solution to mitigate some of these negative effects. This is an intriguing avenue of exploration in improving the robustness and accuracy of transcription in the future.
How Video File Size Compression Affects Transcription Accuracy in 2024 - ProRes format advantages and limitations for transcription
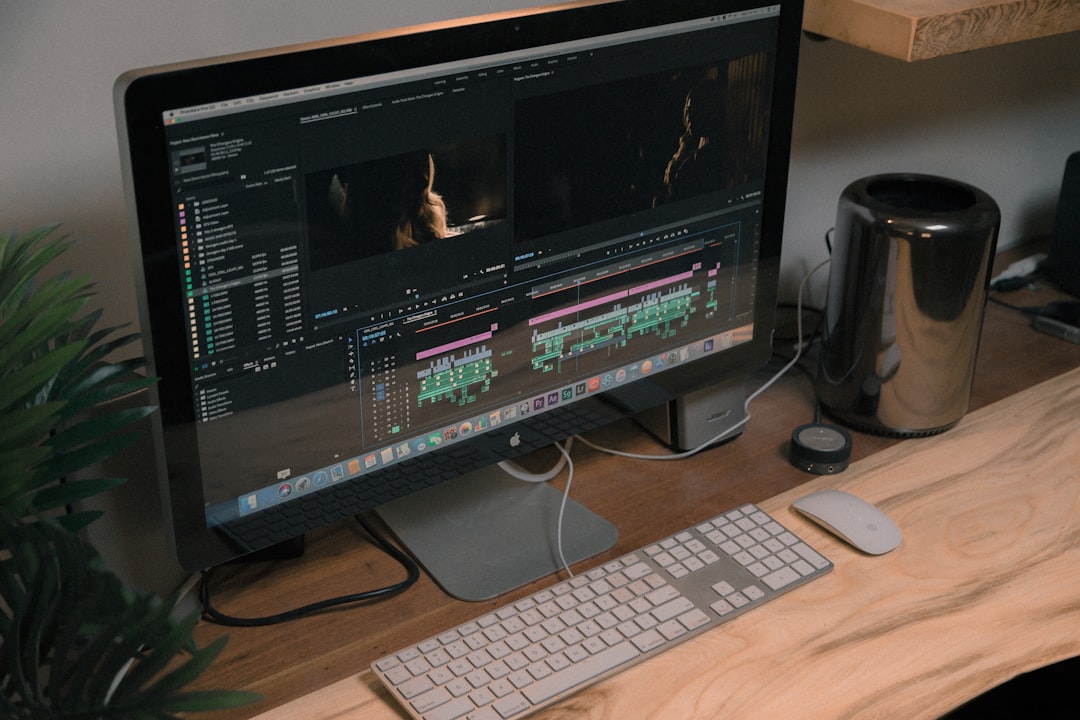
ProRes, a high-quality video format designed for professional editing, holds certain advantages for transcription due to its emphasis on audio fidelity. Its intraframe compression, which processes each frame individually, maintains a higher level of audio quality during editing, beneficial for accurate speech recognition. Essentially, the audio information isn't significantly degraded during the video encoding process, resulting in a cleaner audio signal for transcription. This approach is in contrast to many other codecs which compromise audio quality to achieve smaller file sizes.
However, ProRes's commitment to audio fidelity and visual detail comes at a cost: its files are substantially larger than other formats like H.264 or H.265. This can pose issues for storage, especially when working with long videos or numerous files. Depending on the resources and workflows in place, managing ProRes files can be cumbersome. Additionally, bandwidth requirements for transferring these files could create delays and impact efficiency for projects that rely on online collaboration or cloud-based transcription platforms.
While ProRes undeniably produces high-quality audio that is beneficial for transcription, the large file sizes might be a deterring factor for certain users. It becomes a trade-off between the advantages of preserving detailed audio for transcription and the resource requirements for managing these files. In essence, the value of using ProRes for transcription depends on the specific circumstances, and the user should weigh the impact of file sizes against the importance of maximizing audio fidelity for the task.
Apple's ProRes format, introduced in 2007, is a high-quality video compression method primarily designed for post-production workflows. It employs intraframe compression, meaning each frame is encoded independently, leading to higher quality during video editing compared to formats that rely on interframe compression, such as H.264 or H.265. This is partly due to ProRes' ability to support up to 12-bit color, resulting in a significantly wider range of colors and dynamic range compared to standard 8-bit video. This higher bit depth carries over into audio, offering a richer representation of sound that can benefit speech recognition.
However, these advantages come with a trade-off: ProRes files are significantly larger than those produced by lossy formats. For instance, ProRes 422 files are roughly 174 times larger than files encoded in the HEVC format, while ProRes 4444 files are about 411 times larger. This creates challenges in situations where storage capacity or bandwidth is limited. This fact could potentially slow down transcription workflows, especially when processing large volumes of video data.
ProRes RAW, introduced in 2018, extends ProRes compression to raw video images captured by certain cameras. It offers variants like ProRes RAW HQ that provide a compromise between quality and data rate. The data rates for ProRes RAW HQ fall between ProRes 422 HQ and ProRes 444, allowing filmmakers a degree of control over video file sizes while maintaining quality suitable for professional applications.
In the context of transcription, the benefits of ProRes' quality become apparent. Maintaining higher audio fidelity in ProRes files helps speech recognition systems perform better, as more audio detail remains preserved compared to heavily compressed formats. For example, features such as multi-channel audio that many compressed formats may not support can benefit the separation of specific audio cues for transcription. However, the high fidelity of ProRes comes with a greater demand for computing resources. Transcription systems that need to process ProRes files in real-time might struggle due to the increased processing load compared to formats with lower computational requirements.
Despite its size limitations, ProRes offers consistent quality across different resolutions and bitrates, making it a reliable format for professional video productions where high-quality transcription is a priority. The format's suitability for handling nuanced audio characteristics, like pitch and tone variations, can be particularly beneficial when transcribing tonal languages. Although technically lossy, the quality degradation in ProRes is often considered near-lossless. But the lack of features like variable bitrates found in codecs like Opus forces users to carefully balance file size and quality beforehand. There's no automated adaptation to content complexity, which might need to be considered when choosing a compression format for transcribing various types of content.
In summary, ProRes format provides considerable benefits for video editing and audio quality, but it demands greater computational and storage resources compared to many other codecs. Its ability to retain high-fidelity audio data offers a substantial advantage for accurate transcription, particularly for languages with rich phonetic variations, but users must be prepared for the associated file size increase and processing requirements.
How Video File Size Compression Affects Transcription Accuracy in 2024 - Comparing human and automated transcription accuracy rates
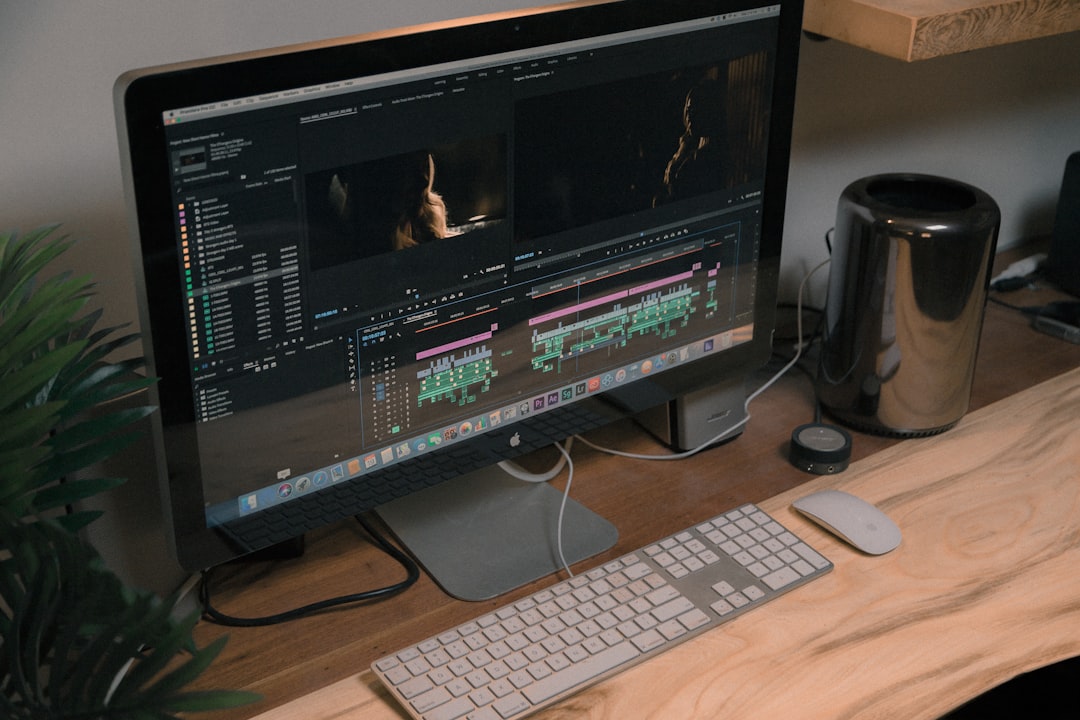
Human transcribers typically achieve accuracy rates close to 99%, significantly surpassing the 80% to 90% accuracy range often seen in automated transcription services. This higher accuracy is a direct result of the human ability to understand context, nuances of language, and deal with complex audio situations. While human accuracy is a major advantage, automated services excel in speed. They can generate transcripts in a matter of minutes, while human transcription can take substantially longer, especially for longer or more complex audio.
The choice between automated or human transcription comes down to prioritizing specific project needs. Budget limitations, the complexity of the audio, and desired accuracy levels all play a role. For simpler projects where speed and cost are more important than absolute accuracy, automated services can be a practical solution. On the other hand, projects requiring greater accuracy, like those needing specific formatting, might benefit more from human transcription or hybrid approaches.
Hybrid approaches, combining automated and human efforts, seem to provide a balanced approach. Automated systems handle the initial transcription, and humans refine the output, potentially leading to a higher quality final product than either alone. This becomes especially important when audio compression can reduce the clarity of audio, causing automated systems to struggle to understand and accurately interpret the spoken words. Understanding how video compression affects transcription quality becomes key to deciding if a hybrid approach or a solely human approach is needed to get the results desired.
When comparing human and automated transcription accuracy, it's evident that humans consistently outperform most automated systems. Human transcribers, in ideal settings, can achieve accuracy rates close to 99%, while automated services typically fall within the 80% to 90% range. This discrepancy stems from the human ability to leverage a deeper understanding of language, including context, nuances, and variations like sarcasm or idioms, which can be difficult for automated systems to interpret.
Humans are naturally equipped to adapt to accents and dialects, a challenge for automated systems that often require specialized training for each variation. This makes human transcribers more versatile when dealing with diverse speech patterns. Furthermore, the kinds of errors made differ significantly. Humans might make occasional typos, while automated systems frequently produce phonetic errors, misinterpreting similar-sounding words, which can lead to semantic mismatches within the transcribed text.
Another critical point is the ability to manage complex audio environments. While humans can readily navigate overlapping speech or background noise, automated systems often falter when faced with such challenging audio conditions. Although human transcription usually necessitates significantly more time than automated methods, requiring a time investment that is likely five times longer than the audio duration, automated systems deliver a swift turnaround, prioritizing speed over absolute accuracy.
The performance of automated systems is also intricately linked to the quality and quantity of data used during training. If the training set lacks sufficient variety in accents, speaking styles, or audio conditions, the system's ability to adapt to new audio inputs will be significantly reduced. This limitation contrasts with humans, who continuously learn and adapt to a wide array of speech characteristics.
In real-time transcriptions, humans can immediately address errors or misinterpretations, providing a more dynamic and accurate transcription experience. This agility often surpasses automated systems, which usually require post-processing to rectify errors after the transcription is complete. Humans also excel at understanding and preserving commonly used phrases, which automated systems often struggle to recognize unless specifically trained on those phrases.
Additionally, humans effectively preserve the intended meaning of figurative language like metaphors and similes, which automated systems frequently misinterpret as literal expressions, ultimately skewing the transcribed text.
Automated transcription systems have undeniably become valuable tools across numerous applications, offering cost-effectiveness and fast turnaround times. Yet, their limitations when navigating the complexity and nuances of spoken language underscore the continuing value of human transcribers in scenarios demanding precision and a nuanced understanding of language.
How Video File Size Compression Affects Transcription Accuracy in 2024 - Audio file formats and their effects on transcription precision
The precision of automated transcription in 2024 is strongly tied to the characteristics of the audio file format used. Popular choices like MP3 and WAV have different impacts on the transcription process. MP3s, while convenient due to their compact size achieved through lossy compression, sacrifice audio quality. This can negatively impact transcription accuracy, especially when dealing with already difficult audio like speech with background noise or accents. On the other hand, lossless formats like WAV and FLAC retain the full audio information, providing a cleaner signal for the transcription software to analyze. This can be highly beneficial when seeking high accuracy, especially in situations where the audio may be complex or challenging. As video formats continue to develop, the selection of the appropriate audio format and how it's compressed will become even more important for ensuring optimal transcription results. It's a choice that should be carefully considered when striving for the best possible transcription output.
1. The selection of an audio file format has a significant impact on how well transcription systems perform. Formats like WAV and FLAC, which are uncompressed, offer much cleaner audio compared to compressed formats like MP3, leading to more accurate transcriptions. This is because these uncompressed formats maintain the integrity of the original audio signal, without any data loss.
2. Audio files with lower bitrates, particularly those below 128kbps, can lead to the merging of overlapping sounds, creating a challenge for speech recognition systems. These systems rely on distinguishing between separate phonetic elements, and the loss of clarity caused by low bitrates can hinder their ability to do so effectively.
3. When audio is encoded using a codec that applies a high compression ratio, the resulting file might include artifacts and distortion. This distortion can confuse the machine learning models used for speech recognition as they are quite sensitive to audio quality and fidelity. The presence of such artifacts introduces unintended alterations into the audio that negatively impact the transcription output.
4. Interestingly, some audio compression techniques use a method called perceptual encoding. This approach prioritizes the frequencies that humans are less likely to notice, while discarding other frequencies. Although seemingly efficient in terms of data reduction, this can obscure crucial phonetic information, which is particularly detrimental for the accurate transcription of languages that rely on subtle sound patterns and distinctions.
5. Research suggests that processing audio files compressed with lossy formats can take longer compared to lossless formats. This is likely because the algorithms struggle to decipher the compromised audio signals, resulting in a need for more post-processing corrections to achieve satisfactory results. This adds to the complexity and time involved in generating accurate transcripts.
6. It's interesting that not all audio formats handle background noise in the same way. Certain formats might unintentionally amplify ambient sounds, which can easily mask spoken words and impede accurate transcription. This issue is particularly relevant in environments with multiple speakers or significant background noise, where capturing individual speech can be challenging.
7. The sample rate of an audio file also influences transcription accuracy. While 44.1kHz is the standard for audio CDs and most music recordings, higher sampling rates might be needed for capturing detailed audio recordings, which are often crucial in specialized fields such as medical or scientific contexts. These higher rates capture finer details in the sound waves, which help provide better source audio for transcription.
8. The variations in compression techniques can have a unique effect on different speakers and languages. For instance, tonal languages rely on specific frequency patterns to convey meaning accurately. If these frequencies are lost during compression, transcription errors are likely to increase significantly. So understanding how different compression choices affect different kinds of languages is important for transcription accuracy.
9. Recent developments in audio coding have led to improvements in techniques like the Opus codec, which supports variable bitrates in real-time. This dynamic adaptation offers exciting possibilities for maintaining audio quality in challenging environments where speech recognition is needed. Adapting to the conditions can make the transcription results better in environments that are constantly changing.
10. While file formats that use high compression ratios offer considerable storage space advantages, the resulting compromise in transcription accuracy raises some serious questions about their long-term viability in professional contexts where accurate transcription is paramount. In scenarios where precision is essential, perhaps higher fidelity formats might be more suitable. The balance between data size reduction and accuracy is an important consideration for choosing the best approach.
Experience error-free AI audio transcription that's faster and cheaper than human transcription and includes speaker recognition by default! (Get started for free)
More Posts from transcribethis.io: