How Product Image Generators Are Adapting RAG Models for Enhanced E-commerce Visual Search
How Product Image Generators Are Adapting RAG Models for Enhanced E-commerce Visual Search - Pinterest Shopping Updates RAG Systems With Visual Similarity Feature December 2024
Pinterest's December 2024 update introduced a visual similarity feature aimed at improving its shopping platform. Now, users can search by image, a capability woven directly into the Pinterest experience. This allows users to find related items within a single image (or "Pin"), effectively making visual search a central part of how people interact with the platform. Behind the scenes, Pinterest has been refining its RAG (Retrieval-Augmented Generation) models. These models are designed to help users find the right products by pulling together relevant information, leading to more accurate and helpful search results. The integration of image-based search has seen substantial adoption, with millions of users now employing it. This highlights the feature's increasing role in how people shop online. Moreover, the Lens BETA expands visual search beyond just Pinterest's image library by enabling mobile phone-based searches, furthering the platform's goal of creating a seamless shopping experience across all its tools. While the technology shows promise, there's always the question of how Pinterest will maintain user privacy and ensure the visual similarity feature doesn't inadvertently lead to biased or unfair search results.
Pinterest, in its December 2024 update, has integrated a visual similarity feature into its shopping tools. This allows users to search for products simply by using an image, essentially broadening the scope of visual search within their platform. It seems Pinterest is banking on this functionality becoming a major driver of user engagement, particularly with its Chrome extension. The way this works involves their RAG systems, which are being tweaked to guide users towards appropriate product choices based on visual cues. This RAG approach allows for more dynamic information retrieval, resulting in responses that are more relevant to what the user sees.
The Lens BETA feature, which uses your phone camera, takes this a step further, extending visual search beyond just Pinterest's own image library. It's interesting that they are using this technology to try and predict future trends, which suggests Pinterest sees the future of e-commerce tied to visual search and understanding user behavior through these image interactions.
Their aim seems to be the creation of a seamless shopping experience across all their tools. The impressive scale of visual search users highlights how critical this feature is to Pinterest. Ultimately, Pinterest's improvements in RAG for visual search reflect the wider shift towards more adaptable AI models in online retail. This is a notable example of how language models are being used to enhance the shopping experience, but I also wonder how much further this can improve customer satisfaction and how it might impact things like product discovery in the long run.
How Product Image Generators Are Adapting RAG Models for Enhanced E-commerce Visual Search - Product Staging App Pixelcut Adds RAG Based Background Removal Tool
The Pixelcut product staging app has recently incorporated a RAG-based background removal tool, marking a step forward in how e-commerce product photos are created. This feature, powered by artificial intelligence, simplifies the process of isolating and enhancing product images, making it easier to produce eye-catching product visuals for online stores. The app's ease of use and high-quality outputs certainly make creating professional-looking photos faster and more accessible. However, as AI takes over a greater share of the image editing process, concerns arise about the potential impact on product authenticity and visual integrity.
Pixelcut, with its ability to quickly generate visually compelling photos, fits within the trend of visual content improving the overall e-commerce customer experience. But, as these automated tools become more common, ensuring the quality and creative integrity of product photos within the growing landscape of AI-enhanced imagery will remain a challenge for both online businesses and consumers. This development, while streamlining production, also highlights the growing tension between technology's efficiency and the need to maintain an authentic representation of products for potential buyers.
Pixelcut, a product staging application designed to streamline e-commerce visuals, has integrated a new background removal feature built upon Retrieval-Augmented Generation (RAG) models. The traditional approach to background removal often involved tedious manual work, but Pixelcut's AI-powered solution automates this process, making it much faster and easier. The use of RAG here seems promising for refining the accuracy of the segmentation—the process of separating the product from its background. It's interesting to see how the app leverages these newer models for image editing, because the clarity of the product image is a key factor in whether or not a shopper decides to buy. There is a growing body of evidence that shows higher quality photos significantly influence purchasing decisions.
The RAG integration is not simply about faster processing. It seems to suggest a move towards making the background removal more dynamic, potentially allowing the AI to understand the product and scene better. This could lead to more nuanced editing, especially in situations with complex lighting. This also aligns with the growing trend of personalized e-commerce experiences. As online shoppers become more discerning, the quality of product visuals has become increasingly important. In the past, background removal might rely on simpler techniques like color detection or edge finding, but RAG allows for a deeper understanding of the content. While it's still early days, this deeper contextual awareness should lead to a reduction of the common artifacts we often see with automated image editing.
It's also notable that a large segment of online shoppers—research suggests the number is nearing 80%—heavily rely on product visuals when making purchase decisions. This emphasizes the need for e-commerce retailers to invest in high-quality imagery, and tools like Pixelcut make this more accessible. Furthermore, the relationship between RAG and visual search is worth exploring. As AI in these apps gets better at understanding the context of product images, the experience for shoppers could significantly improve. They might be able to find exactly what they're looking for much more easily, leading to a boost in overall satisfaction. This connection could be key to enhancing the speed and efficacy of e-commerce discovery, especially in an environment where attention spans are short. This ability to create a more impactful and eye-catching product photo is critical to keeping people engaged in online retail. We can see this as part of a wider shift towards AI-driven visual experiences within the e-commerce industry, where product visualization and discovery are key differentiators. It'll be interesting to see how these tools evolve and how shoppers respond to these increasingly sophisticated and automated image editing capabilities.
How Product Image Generators Are Adapting RAG Models for Enhanced E-commerce Visual Search - Amazon Tests New RAG Architecture For Cross Category Product Discovery
Amazon is exploring a new way to help shoppers find products across different categories using a technology called Retrieval-Augmented Generation (RAG). Essentially, it's about making their search system smarter. RAG enhances the ability of AI systems to generate responses by incorporating external information. This means the system can go beyond just what's been programmed into it and find more relevant details from other sources when you search. Amazon is experimenting with using Amazon Kendra, a search tool that can find related information based on what you type in. This could lead to more useful results when you're browsing for something specific, especially if you aren't entirely sure what you're looking for or how it's categorized.
While still in the testing phase, this approach suggests a possible future where e-commerce is more intuitive and responsive to the way people search. It might change how we expect products to be displayed and described online, and how images play a role in making purchasing decisions. If it works as intended, this could be a notable shift for the industry as online retail continues to rely more heavily on AI and personalization to improve user experience.
Amazon is experimenting with a new Retrieval-Augmented Generation (RAG) architecture to improve how shoppers find products across different categories. It seems they're aiming to build a system that can connect product images to related items in a more dynamic and nuanced way. This could be a big deal for the customer experience, leading to more browsing and, hopefully, more sales.
It's interesting to think about how this RAG model can leverage visual information. Research suggests that using high-quality product images can significantly boost sales, which makes sense, because visually appealing items are more likely to capture someone's attention. I suspect Amazon's investment in RAG for visual search is related to this desire to boost conversion rates and possibly even compete better with other retailers.
This RAG system, by its design, can not only react to what customers are searching for but also adapt to broader shifts in buying habits. It seems to be designed to analyze image data at scale to ensure the suggestions it provides are relevant to current trends. This, I think, is important in keeping customers engaged, since their preferences can change over time.
There's some evidence suggesting a mix of AI-generated and traditional product photos can actually improve how well shoppers find what they're looking for. Maybe Amazon will start mixing these image types to offer more tailored shopping experiences. It's an interesting area that could help them understand how users interact with the site better.
One thing that RAG models seem good at is minimizing the common mistakes we often see with visual search. If RAG can improve the accuracy of the image data it analyzes, shoppers are likely to have more confidence in the results, and perhaps be more likely to purchase something if they believe it accurately represents what they'll receive.
It's becoming increasingly common for online shoppers to expect smarter product discovery features, and this RAG development shows Amazon is taking this seriously. The move towards a more visual approach to e-commerce is apparent, and Amazon seems to be positioning itself as a leader in this trend.
Interestingly, the majority of consumers seem to prefer images over plain text when shopping online, which underscores the growing need for better visual search tools. Amazon's investment in this area may be in direct response to this changing behavior of online shoppers.
RAG can process visual information at lightning speed, which is crucial for tailoring suggestions to what a user is currently doing on the platform. Imagine a system that updates what's being shown to you in real-time as you browse! This level of personalization might be one of the goals Amazon hopes to achieve through RAG.
Besides recommending products, AI is being integrated to help understand how shoppers feel about product images, which could become another piece in Amazon's understanding of customer behavior. This is an interesting area that could lead to more focused marketing efforts.
As the reliance on visuals in online retail grows, there's a chance that future RAG features like augmented reality (AR) could transform how shoppers experience product visualization. Imagine trying on clothes virtually, or seeing how furniture will fit in your home, all through Amazon. This may represent a new frontier in how we shop online.
How Product Image Generators Are Adapting RAG Models for Enhanced E-commerce Visual Search - Shopify Integrates Visual Search With RAG Models For Product Recommendations
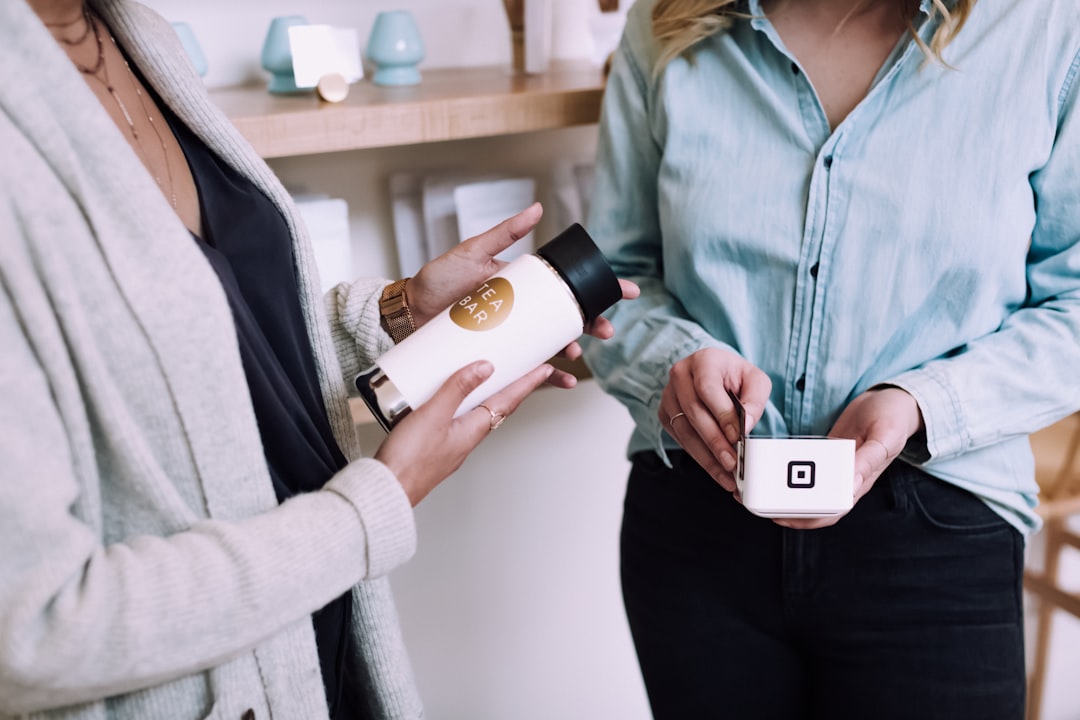
Shopify has integrated visual search into its product recommendations using Retrieval-Augmented Generation (RAG) models. This allows shoppers to upload images and quickly find similar products within the Shopify catalog. The idea is to make product discovery more intuitive, shifting away from traditional text-based searches. Since detailed product pages can contribute significantly to customer traffic, enhancing visual search becomes crucial for encouraging cross-selling and boosting sales conversions.
RAG models are helping Shopify's system leverage the power of large language models combined with real-time information retrieval to improve how product recommendations are generated. Essentially, the platform uses AI image recognition to identify visual elements like color and create descriptive tags, making products easier to find.
However, the ongoing challenge for any visual search system is how to quickly and accurately update its models with new product information. This is important to ensure the search results remain reliable and helpful. Shopify's Search & Discovery app also provides tools for merchants to refine the recommendations, allowing them to better control how products are presented to shoppers.
Ultimately, the goal is to predict a customer's desired style and present them with product suggestions that align with those preferences, making the overall shopping experience more personalized and enjoyable. The fashion, home decor, and accessory industries, where visual appeal significantly influences purchasing decisions, will likely benefit the most from these enhancements. But, as the technology matures, concerns about how well it maintains product authenticity and visual integrity will likely need to be addressed.
Shopify has integrated visual search into its platform using RAG models, aiming to improve how it recommends products. This AI-powered search lets customers find related items simply by uploading an image, making product discovery a lot more intuitive and faster. It's becoming increasingly clear that product detail pages are a major traffic driver for online stores, often accounting for up to half of customer visits. This means they play a key role in boosting sales through cross-selling and improving conversion rates. RAG models are interesting because they combine the power of language models with the ability to quickly access relevant information, and this is helping to change how product recommendations are generated.
The way this visual search system works involves AI-based image recognition to create descriptive tags about products based on visual aspects like color. One of the challenges for visual search is keeping these systems up-to-date as new products are added to a retailer's inventory. Maintaining accuracy and speed becomes tricky with a constantly changing pool of items. Thankfully, merchants have the ability to control how products are displayed and suggested through Shopify's Search & Discovery tools, giving them a degree of control over the recommendations. This all comes down to trying to anticipate what a shopper might want, using data to predict shopping intent and then suggesting more items in the same style.
The main idea behind this whole visual search concept is to tackle a weakness of traditional text-based search—it can sometimes be hard to find what you're looking for when relying on words alone. This visual approach seems to be a more natural way for some shoppers to interact with an online store. It's especially helpful in fields like fashion, home furnishings, and jewelry, where aesthetics are a crucial factor in buying decisions. There's a lot of room for improvement in this area, but it's clear that AI and visuals are playing a bigger role in the future of e-commerce. It will be fascinating to see how these systems evolve and how consumers continue to respond to these new search experiences. I am particularly curious about how future versions of RAG will be able to deal with subtle shifts in aesthetics and how they may be able to incorporate information like color palettes, composition, and lighting into their recommendations.
How Product Image Generators Are Adapting RAG Models for Enhanced E-commerce Visual Search - Adobe Scene Graph Improves Product Image Classification Through RAG Models
Adobe's Scene Graph, coupled with Retrieval-Augmented Generation (RAG) models, is changing how product images are categorized in online shopping. This approach excels at understanding the relationships between different parts of an image, which is important for creating and categorizing images more precisely. They use something called the SceneGenie model to convert written descriptions into a kind of visual map, a scene graph. This helps the AI to understand what's in the image and how it relates to the text.
Despite the improvements, accurately representing the various ways things look and how they interact within an image is a persistent challenge. It's a hurdle in effectively classifying the product images used in visual search. As e-commerce continues its shift towards a more visually-driven experience, Adobe's innovations could revolutionize how online stores manage and utilize product visuals for improved search functionality.
Adobe Scene Graph employs RAG (Retrieval-Augmented Generation) models to improve how product images are categorized by considering the relationships between different objects within a scene. This means the system goes beyond just recognizing individual items and starts to understand how they relate to each other visually, which can be useful for improving the accuracy of image search.
The SceneGenie model, for instance, takes text descriptions of a scene and converts them into a visual graph-like representation before generating the image. This clever approach helps ensure that the relationships depicted in the image align with the structure of the scene graph. This connection between graph structure and visual output relies heavily on the concept of scene layouts, which act as a sort of blueprint for the generated image. It's a way to bridge the gap between how we describe a scene using language and how that scene is visually represented.
While this idea of understanding the scene as a graph is powerful, it also brings new challenges. The visual appearance of objects and the relationships between them can vary drastically across images, requiring innovative methods to analyze those connections accurately. This has led to a lot of focus on developing approaches that can effectively reason about relationships within the image.
The use of diffusion models like those used in SceneGenie has undoubtedly helped increase the quality of generated images from text descriptions. However, it's still tough to reliably create images that accurately represent specific quantities of objects within a scene. Imagine trying to generate a scene with precisely three chairs and two lamps in the right locations—it's not always a straightforward task.
Graph Convolutional Networks are being used to overcome the issue of scene graph expansion. This task refers to the problem of filling in the missing information in the scene graph, specifically missing labels and the relationships between the objects. In other words, it's like trying to deduce the hidden connections in an image to ensure the AI has a complete picture of what it's looking at.
A major limitation for current scene graph generation efforts is the lack of variety in datasets. These datasets often don't fully capture the complex relationship between objects and their environments. This lack of diversity can impact how well object detection models can perform and could also limit the ability of these models to generalize to new and unseen situations.
The entire process of creating a scene graph and then using it to generate an image emphasizes how tricky it can be to translate the information stored in a graph to a visual representation. It's almost like having two separate languages you have to translate between.
Techniques like GANs and diffusion models have significantly impacted how well product image generators are able to perform. We're seeing significant improvements in image generation from text prompts, which opens up many new possibilities for e-commerce applications.
Furthermore, research is focusing on improving how product images are categorized. Incorporating prior knowledge into scene graph models is becoming a crucial part of this. This approach allows the AI to learn about the context of different products, potentially improving the quality of visual searches in the long run. This is an important trend to watch as visual search technologies continue to improve and potentially change how customers interact with online stores.
How Product Image Generators Are Adapting RAG Models for Enhanced E-commerce Visual Search - Wayfair Develops Custom RAG Model For Furniture Style Matching System
Wayfair has built a specialized RAG model specifically designed to improve how their furniture style matching system works. This means shoppers can now upload pictures of furniture and quickly find similar items within Wayfair's massive product library, which boasts over 8 million products. The RAG model makes the process smoother by automatically figuring out the most important parts of a user's image and essentially cropping it for them. This reduces the time spent searching and simplifies the overall shopping experience. The use of generative AI in the system also promises to improve the quality of images used for product recommendations, creating more realistic representations that are important for online sales. This focus on AI-driven visual search is part of a larger movement in e-commerce where retailers are using technology to make shopping easier and more enjoyable for customers. In fields like furniture sales, where the look and feel of a product are crucial to sales, this is a particularly important development.
Wayfair's development of a custom RAG model for furniture style matching is a fascinating example of how AI is being used to personalize the online shopping experience. It's a clever approach that tries to bridge the gap between what a customer might be looking for (based on an image) and what's available in Wayfair's massive inventory. The model combines textual and visual information, making it a multimodal system capable of understanding both the descriptive language users might employ and the visual cues present in an image. This multimodal approach could improve the relevance of search results, potentially leading to higher engagement and a smoother shopping experience.
The idea is to improve the accuracy of finding furniture styles that match what a customer is after. While this might sound simple, it's actually quite a difficult problem for AI to solve. Studies have shown that visually similar products can boost sales, so getting the matching aspect right is crucial. The quality of product images, which is becoming increasingly vital for consumers' purchasing decisions, also plays a big role in this system. Wayfair's approach suggests that they believe high-quality images, possibly enhanced by their own AI image generators, are essential to fostering consumer trust and enabling product discovery.
This new approach also impacts how products are tagged and described. The model can automatically assign accurate style tags to products, potentially saving Wayfair's product team, reportedly 700 strong, a substantial amount of time and effort usually spent with manual categorization. And the cool part is the RAG model is flexible. It can adapt to changes in the product catalog and user preferences in real-time, responding to the dynamism of the furniture market.
One interesting capability is the AI's ability to create virtual product staging. It can render 3D images of furniture within different settings, which allows shoppers to envision how a piece might look in their homes. This kind of feature can boost engagement and satisfaction, helping customers visualize their purchases better. They are also leveraging deep learning techniques to analyze customer interactions and improve the model's ability to make recommendations over time. It's like the AI is learning from each interaction, gradually gaining a deeper understanding of what consumers like and dislike.
It's not just about better product suggestions, though. The hope is this system will also help minimize customer returns, a major issue for online retailers. By ensuring more accurate style matches, Wayfair could potentially reduce the frequency of customers getting items that aren't quite what they envisioned. This innovative approach to visual search puts Wayfair in a better position to compete with other online furniture retailers, as consumers become increasingly reliant on visual cues when shopping. It will be interesting to see if other online retailers follow suit and adopt this technology, and whether consumers find it actually enhances their experience enough to be considered a major shift in how they interact with online furniture stores. The future of online shopping might be increasingly reliant on these kinds of AI-powered, visual search technologies, and Wayfair seems to be betting on this trend.
More Posts from transcribethis.io: