Experience error-free AI audio transcription that's faster and cheaper than human transcription and includes speaker recognition by default! (Get started for free)
New Frontiers in Voice AI Beyond Text-Based Models
New Frontiers in Voice AI Beyond Text-Based Models - Real-time Multimodal Processing in Voice AI
Real-time multimodal processing signifies a significant leap forward in voice AI, moving beyond the limitations of traditional text-based models. By incorporating various data types—audio, visual cues, and textual information—voice AI systems can gain a deeper understanding of context and user intent. This leads to more fluid and natural interactions, a boon for applications like voice cloning, podcast creation, and audiobook production. Imagine voice-cloned narrators that respond dynamically to the story or listeners seamlessly adjusting audiobook playback based on visual cues.
However, developing genuinely effective real-time multimodal interactions is a complex and ongoing endeavor. While current implementations are demonstrating progress, we’re still far from seamlessly blending various sensory inputs into a unified understanding for the AI. The path forward necessitates a focus on innovative research to optimize the integration and processing of multimodal data streams. If successful, we can expect to see a dramatic shift in how humans interact with AI systems, transitioning towards a more intuitive and natural experience. This would truly unlock a new frontier in voice AI.
The integration of multiple sensory inputs—like voice, visuals, and even gestures—is pushing voice AI beyond its traditional text-based confines. We're seeing systems that can process and react to this multifaceted data in real-time, paving the way for more sophisticated voice interfaces in applications such as conversational agents. OpenAI's work, for example, extends their Chat Completions API to encompass audio, making interactions more dynamic. Similarly, models like Llama 32 exhibit impressive capabilities by intertwining voice input with the interpretation of text or images. This multisensory approach is further evidenced in Microsoft's Azure services, specifically with GPT-4o and the AI Studio, which offer robust audio handling for real-time conversation and the analysis of speech features respectively. ChatGPT's evolving functionality, including conversational voice interactions, showcases this trend. However, these developments are not without their challenges. The new multimodal versions of ChatGPT, employing separate models for audio transcription, text processing, and output generation, illustrate the intricate nature of these systems and the hurdles in achieving a seamless, integrated experience. Essentially, these recent strides in voice AI signify a departure from the more simplistic, command-based assistants like Siri or Alexa. While we're still working towards achieving truly advanced multimodal experiences, the future certainly seems to lie in building AI systems that can interact with users on a far richer and more contextual level. This shift holds immense potential for creating innovative audio experiences – from improved audiobook production that better captures emotional nuances to generating more dynamic and interactive podcast content through the inclusion of soundscapes and real-time translation. The ability to understand a wider range of cues and adapt accordingly will make voice AI a significantly more valuable tool, pushing the boundaries of creativity and accessibility across a wide variety of applications.
New Frontiers in Voice AI Beyond Text-Based Models - Advancements in Emotive Voice Synthesis from Brief Samples
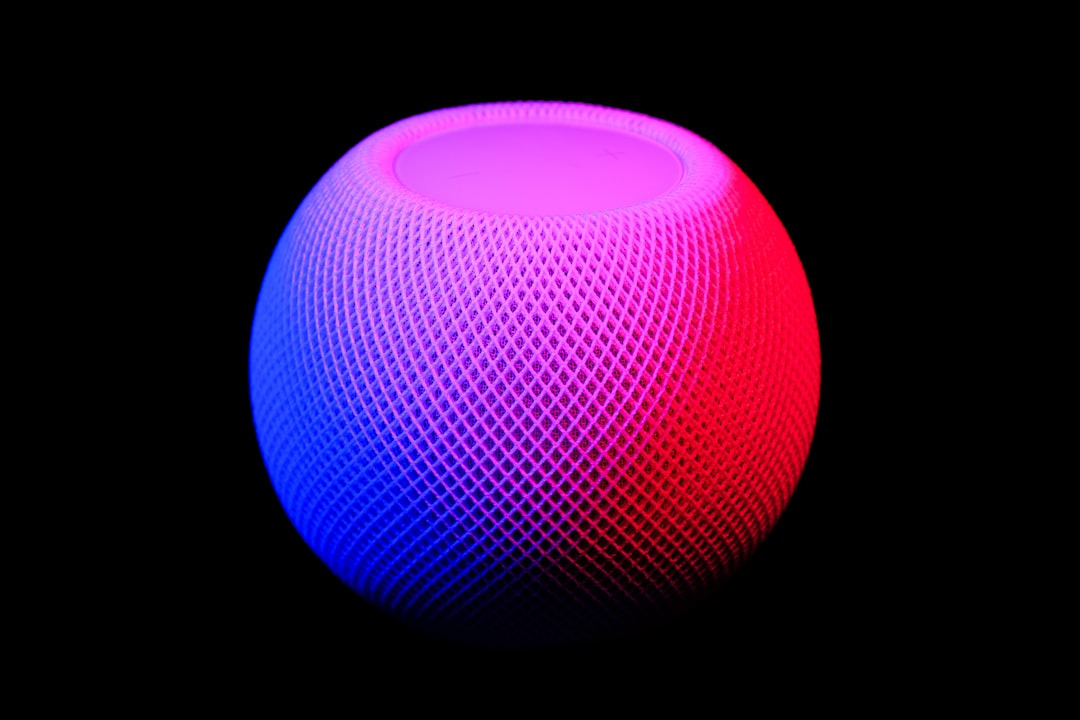
New Frontiers in Voice AI Beyond Text-Based Models - Flow Matching Techniques Revolutionizing Speech Generation
Flow matching is revolutionizing how AI generates speech, pushing the boundaries of what's possible in voice AI beyond the limitations of older, text-based models. These techniques leverage vast amounts of speech data to learn intricate patterns, resulting in significantly improved speech synthesis. Models like Voicebox and SpeechFlow are prime examples, showcasing the ability to produce high-quality, natural-sounding speech, achieving what traditional approaches often struggled with.
This advancement goes beyond just synthesizing speech. Flow matching can enhance tasks like voice conversion, enabling the transformation of a person's voice into another, and speech infilling, where missing sections of audio can be intelligently recreated based on context. Prior methods typically suffered from limitations in scale and adaptability, restricting the diversity of generated voices. However, with access to enormous quantities of speech data, these newer AI models achieve a degree of naturalness and flexibility previously unattainable. The implications for applications such as podcast creation, voice cloning, and audiobook productions are substantial, with the potential to deliver more realistic, compelling, and accessible audio experiences.
As voice AI matures, flow matching techniques hold great promise in fostering more seamless and emotive interactions with AI. While it's still early days, these advances open the door to a future where voice interactions feel more natural and less robotic.
Flow matching presents a novel approach to speech generation, allowing AI models to dynamically adjust the rhythm, tone, and emphasis of spoken words. This is achieved by training models on massive datasets, like the 60,000 hours used in SpeechFlow, which enables them to learn the intricate interplay between text and its corresponding audio representation. This approach goes beyond basic text-to-speech by aiming to replicate the nuanced emotional expression conveyed through human speech, making voice cloning and audio content production more realistic and engaging.
Essentially, flow matching allows AI models to "feel" the rhythm of the content and adapt accordingly. The success of this method hinges on its ability to capture the link between text and its emotional expression – the variations in intonation, speed, and volume that help communicate feelings. This is evident in systems like Voicebox, a non-autoregressive flow-matching model designed for "infilling" missing speech sections based on audio context and textual cues. It's intriguing to consider how psycholinguistic principles are leveraged in this context, particularly given the human brain's propensity to readily process varied speech patterns over monotonous ones.
While the field of voice synthesis has seen an evolution in methods, from basic text-to-speech to more intricate voice conversion, flow matching distinguishes itself by focusing on dynamic adaptation. This adaptive quality shines through in voice cloning applications, where it could potentially preserve the unique qualities of a speaker's voice while adapting to various contexts and emotional states. Furthermore, this method's capabilities extend beyond basic synthesis; it offers potential for influencing how generated audio interacts with environmental context, like background sounds.
However, there are still limitations and open questions surrounding these techniques. While they hold promise for enhancing the listener experience in diverse fields such as audiobooks and podcasts, they must continue to overcome challenges in replicating truly natural-sounding speech. The "uncanny valley" remains a potential hurdle, underscoring the need for researchers to refine their approaches to ensure that the generated speech sounds believable and engaging, rather than robotic or artificial. Researchers are investigating the integration of flow matching with other AI techniques, such as neural control systems, with the aim of creating hyper-realistic audiobooks, where voice actors effectively mimic human emotions and intentions.
The application of flow matching is broadening, extending into post-production audio engineering where it could be used to manipulate recorded voices more efficiently. As this technology advances, it will become increasingly crucial for creating richer, more nuanced audio experiences in fields like audiobook production and interactive podcasting. The ability for voice AI to respond and adjust based on the narrative flow, emotional cues, and environmental sounds presents an intriguing area for future research and development. These techniques are not merely enhancing voice synthesis but are pushing the boundaries of how we experience audio, bridging the gap between human and AI-generated voices, and creating new avenues for creativity and accessibility.
New Frontiers in Voice AI Beyond Text-Based Models - Integration of Professional Voice Acting in AI Models
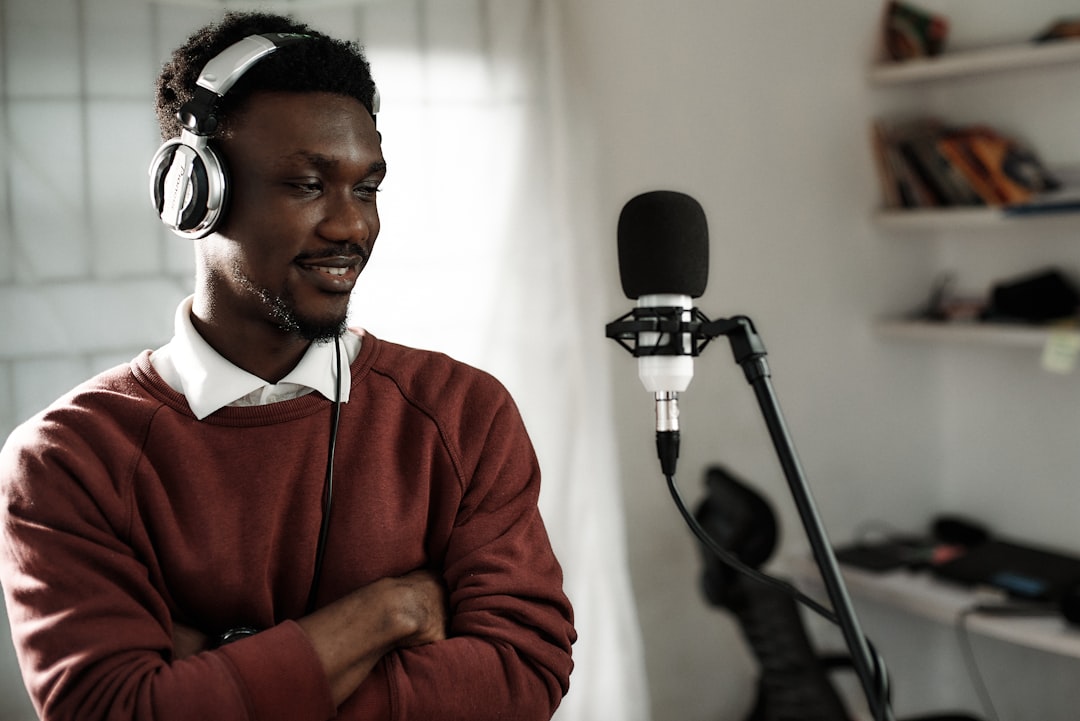
The integration of professional voice actors into AI models represents a significant shift in voice technology, impacting how audio content is produced and experienced. We are witnessing a growing partnership between voice actors and AI developers, resulting in sophisticated AI models that combine the expressive capabilities of human voices with the technical advantages of AI. This collaboration yields richer and more nuanced audio experiences across diverse fields, such as audiobook narration, which can now capture a wider spectrum of human emotions, and podcast production, where AI might enable adaptive features based on listener preferences. Nevertheless, the potential for AI-generated voices to sound unnatural, falling into the so-called "uncanny valley", remains a key obstacle. Continued research and development efforts are vital for refining AI voice models to achieve seamless and truly engaging audio. Furthermore, as new techniques like flow matching become increasingly popular, they hold promise for creating even more compelling and realistic voice interactions, ultimately pushing the boundaries of voice artistry.
The integration of professional voice actors into AI models offers a compelling avenue for enhancing the naturalness and emotional depth of synthesized speech. This is particularly valuable in applications like audiobook and podcast production where authentic emotional expression is crucial for audience engagement. By incorporating the vast range of vocal nuances exhibited by human actors, AI models can learn to replicate subtle emotional inflections, pauses, and breath patterns that contribute to a more believable listening experience. This data-driven approach allows for the creation of audio outputs that are not just technically proficient but also emotionally resonant, effectively minimizing the “uncanny valley” effect that can often be associated with synthetic voices.
Furthermore, professional voice actors bring a wealth of experience in delivering performances across various genres and styles. This allows AI models to develop a more nuanced understanding of how voice adapts to context. Consequently, AI can dynamically alter the tone and pace of a synthetic voice based on the story's flow, character development, or even listener preferences. Imagine an audiobook where the narrator's tone subtly shifts to reflect the emotional weight of a scene or a podcast where the AI-driven host seamlessly adjusts the speech pattern based on audience interaction.
However, the integration of professional voice actors also presents interesting research challenges. For instance, developing algorithms capable of capturing the complexities of human emotion and translating them into compelling voice performances is a continuous area of investigation. Additionally, maintaining a balance between the individuality of professional voice actors and the inherent limitations of AI's ability to completely replicate a specific human vocal performance is another interesting area. Moreover, the ethical considerations of how AI-generated voices might be used to replicate or mimic specific individuals raises important questions that warrant careful thought.
Despite these challenges, the prospect of blending human artistry with AI's capabilities is exciting. We're seeing advancements in AI's capacity for cross-linguistic versatility, enabling natural-sounding multilingual audio outputs. In the future, this technology could facilitate the development of adaptive algorithms that tailor voice delivery based on listener preferences, enriching the storytelling experience in a personalized way. The potential for creating a truly captivating and human-like audio experience through the integration of professional voice acting and AI holds great promise for diverse fields, especially those focused on storytelling and interactive content generation.
New Frontiers in Voice AI Beyond Text-Based Models - Neural Style Transfer Applications in Voice Cloning
Neural style transfer, initially explored in image processing, is now being adapted for voice cloning. This means AI can not only mimic the words of a speaker but also capture their unique emotional tone and vocal characteristics. This opens up exciting possibilities for enriching synthesized audio in areas like audiobooks and podcasts.
By combining neural style transfer with the power of deep learning, we can extract key phonetic features from even short voice samples, constructing comprehensive voice models. These models can then generate audio that retains the speaker's identity throughout longer narratives, a valuable feature for audiobook productions.
Recent research extends this by using musical elements and environmental sounds as influence layers within the voice cloning process, effectively merging the speaker's voice with the surrounding audio context. This could enhance the immersive quality of stories in podcasts or audiobooks, creating a richer auditory experience.
Interestingly, researchers have discovered that neural style transfer in voice cloning can also reduce the computational demands of these models. This happens because the method can efficiently capture complex voice characteristics without the need for enormous datasets. This increased efficiency could enable smaller-scale audio projects to leverage high-quality voice synthesis without massive resource investments.
Looking ahead, neural style transfer could lead to more personalized audio experiences. We might see systems that adapt the emotional tone and pacing of a narrator based on listener preferences or demographic information. This ability to personalize the voice could improve audience engagement with the content.
Imagine an interactive podcast where AI-powered voice cloning generates dynamic audio responses from characters based on listener input. This real-time response, mirroring audience engagement, could amplify emotional cues and make the experience more interactive and engaging.
Researchers are also exploring how neural style transfer can work in tandem with reinforcement learning. The goal is to create voice models that learn and adapt their style over time, based on user interactions. This adaptive feature could pave the way for audiobooks that evolve their narration style according to individual reader preferences.
However, one ongoing challenge is balancing stylistic changes with maintaining clear and intelligible speech. The goal is to introduce expressive qualities without compromising the listener's ability to understand the content. This delicate balance is a focus of ongoing research.
Beyond enhancing emotional expression, neural style transfer could also be used to adapt voices to different dialects and accents. This would broaden the appeal of audio content, allowing creators to reach a wider audience. Audiobooks could then incorporate regional accents, improving accessibility and relatability.
Finally, this technology could lead to "live" audio editing capabilities. Content creators might be able to adjust narrations in real-time, responding to audience engagement and seamlessly changing styles or tones without significant post-production effort. This could be a game-changer for interactive audio content.
New Frontiers in Voice AI Beyond Text-Based Models - Transformer-based Generation for Creative Audio Outputs
Transformer-based architectures have become increasingly important in audio generation, showing promise in capturing intricate aspects of human speech, like emotional nuance. This ability to fine-tune the tone and delivery of synthesized speech holds incredible potential for applications like audiobooks and podcasts, where a compelling narrative depends on evoking the appropriate emotions. We're seeing advancements in techniques like flow matching, which can dynamically adjust features like rhythm, intonation, and emphasis in real time, making AI-generated narrators more responsive to the content they're delivering. It's quite remarkable that tools like neural style transfer can generate sophisticated voice models with less data, making quality voice synthesis accessible even for smaller projects.
The integration of environmental sounds alongside voice cloning is another exciting avenue of research. It suggests the potential for audio narratives that place the narrator's voice within a rich soundscape, adding a new dimension of immersion for the listener. Future applications might even offer tailored audio experiences, adapting the emotional tone and speed based on listener preferences or demographics. We could potentially witness a future where audiobooks and podcasts evolve their narration dynamically based on listener reactions, leading to a truly interactive audio experience.
The development of AI models that learn and refine their voice styles over time through reinforcement learning represents another fascinating research direction. This approach could lead to audiobooks that adapt their narration based on individual preferences, personalizing the experience further. Interestingly, there's ongoing research into extending voice cloning to encompass different dialects and accents, potentially increasing the reach and relatability of audio content across a wider spectrum of listeners. Furthermore, reducing the "uncanny valley" effect – that slightly off-putting sensation when a synthetic voice is too close to human but not quite right – is crucial. By carefully using high-quality recordings from professional voice actors, we're pushing for more natural-sounding AI voices that avoid a robotic or artificial quality.
Looking ahead, innovations could even revolutionize audio editing, potentially providing real-time capabilities for adjusting narrations while recording. This would allow content creators to quickly respond to audience reactions and adjust the narrative's voice tone or style instantly, streamlining the audio production process. Overall, the application of transformer-based generation models and these related developments present a fascinating landscape for research, promising to change how we create and experience audio, especially in fields like audiobook production, voice cloning, and podcasting. The future of voice AI seems poised to become increasingly sophisticated, blurring the lines between human and AI-generated audio. While there's still work to be done, it's clear that AI's impact on the audio landscape is only just beginning.
Experience error-free AI audio transcription that's faster and cheaper than human transcription and includes speaker recognition by default! (Get started for free)
More Posts from transcribethis.io: