Experience error-free AI audio transcription that's faster and cheaper than human transcription and includes speaker recognition by default! (Get started for free)
Neural Cellular Automata New Frontiers in Adversarial Reprogramming for AI Systems
Neural Cellular Automata New Frontiers in Adversarial Reprogramming for AI Systems - Neural Cellular Automata Fundamentals and Deep Learning Integration
Neural Cellular Automata (NCA) stand out as a hybrid approach, seamlessly blending the established framework of cellular automata with the potent capabilities of deep learning. This fusion empowers NCAs to dynamically learn complex update rules, broadening their applicability to a wider range of datasets and tasks. The integration of deep learning has also revealed a conceptual link between NCAs and other neural architectures like graph neural networks. This connection hints at the possibility of inheriting desirable traits like robustness from these related approaches. The push for efficient and scalable computing solutions, driven by advancements in deep learning, has stimulated the development of novel hardware implementations such as Photonic Neural Cellular Automata. This further emphasizes the potential of NCAs to transform computational paradigms. Beyond their theoretical underpinnings, NCAs are showcasing a valuable aptitude for handling complex tasks, particularly in the realm of self-organizing control systems. This suggests that their potential applications are expanding beyond mere mathematical modeling and into a wider array of practical domains.
Neural Cellular Automata (NCA) blend the established framework of cellular automata with the adaptive capabilities of deep learning. This hybrid approach offers the possibility of developing systems that exhibit emergent behaviors and self-organization in ways that go beyond the limitations of traditional CA, which are bound by predefined rules. The ability of NCAs to learn complex update rules via deep learning methods is a key advancement, broadening their applicability to a wider range of datasets and tasks. Interestingly, NCAs share a conceptual link with graph neural networks, potentially implying a similar degree of robustness.
This intersection of deep learning and cellular automata has accelerated the search for more powerful and efficient computational hardware. Photonics has emerged as a promising candidate for developing novel hardware like Photonic Neural Cellular Automata, driven by the ever-growing demands of complex deep learning applications. The core concept of cellular automata, drawing inspiration from how biological cells interact, remains a fascinating area of study. Recent findings reveal a relationship between cellular automata and Lukasiewicz logic, which suggests that deep neural networks could potentially be used to learn the underlying logic that governs the behavior of CAs.
It's intriguing that researchers are exploring the parallels between cellular automata and convolutional neural networks, particularly in their ability to model and represent dynamic systems. NCAs are built on iterative local rule applications, which result in complex global behavior. This suggests a substantial potential for modeling dynamical systems. We often conceptualize NCAs as grids of cells where some are "dead" or empty, while others, designated as "growing", can transition to a "mature" state based on defined circumstances. The ability to design these transition rules, and the emergent behavior this produces, makes NCAs potentially useful in the design of self-organized control systems across a wide range of applications. There's a lot of open questions here. We need to better understand how the choice of the underlying lattice design and the complexity of the rule space will impact performance and limit the scope of applicability.
Neural Cellular Automata New Frontiers in Adversarial Reprogramming for AI Systems - Adversarial Cell Injection Techniques in NCA Grids
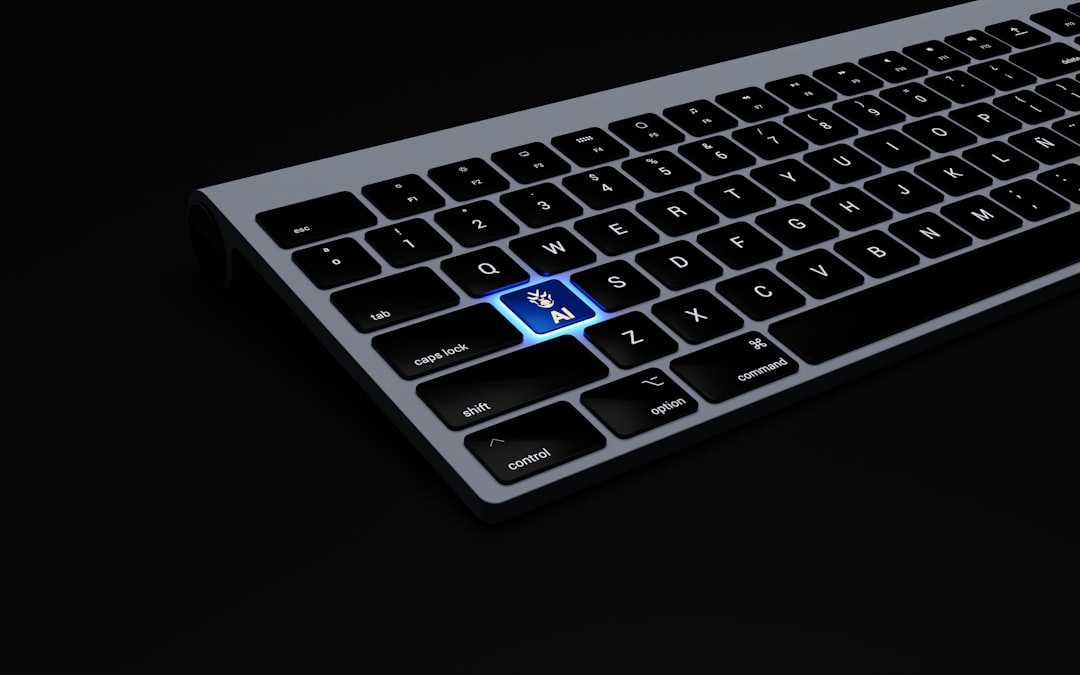
Adversarial cell injection techniques within NCA grids expose potential weaknesses in AI systems, showcasing how targeted manipulations can disrupt their emergent behaviors. By strategically introducing a small number of adversarial cells or modifying the overall grid state, researchers have shown that the robustness of NCA models can vary considerably depending on the specific attack. This research also shines a light on the development of attention-based NCAs like ViTCA, which enhance pattern formation and global coordination but also introduce new avenues for adversarial attacks. Examining the impact of these adversarial patterns on NCA systems provides critical insights into their resilience and the potential for unwanted reprogramming. Ultimately, a deeper understanding of how adversarial techniques can alter the behavior of NCAs helps us refine their design and implement safeguards against manipulation. The findings emphasize the importance of considering these vulnerabilities in the development of robust and dependable AI systems. It is still early days in this field, but it does raise the specter of security implications that are not fully understood.
Neural Cellular Automata (NCA) show promise in modeling complex systems through the interaction of simple local rules, but their susceptibility to adversarial manipulation is a growing concern. Injecting just a few strategically placed "adversarial cells" into the grid can dramatically alter the system's behavior, even in pre-trained models. This highlights a potential weakness in their robustness, especially in applications where reliability is crucial.
A new wave of NCAs, inspired by Transformer architectures, utilizes attention mechanisms to enhance their capabilities. Vision Transformer Cellular Automata (ViTCA) is a prime example, incorporating a localized yet globally aware self-attention scheme. However, even these advanced approaches seem to inherit the same vulnerabilities to adversarial cell injection.
The ability of cells to adapt their orientation, as seen in Growing Isotropic Neural Cellular Automata, introduces further complexity into these systems, which might both help and hinder their resistance to malicious manipulation. It seems that adversarial reprogramming techniques, when applied to NCAs, have a significant effect on experimental outcomes. The success or failure of an adversarial attack can vary greatly depending on the type of pattern introduced into the grid.
This kind of adversarial behaviour suggests that the attacking agent itself might also suffer a reduction in its own robustness when faced with certain injection patterns. This creates an intriguing dynamic where both the NCA and the adversarial technique have potential vulnerabilities and strengths in relation to one another.
This raises a lot of questions about the integrity and reliability of NCAs, as they can learn biased behaviours if the adversarial cells are skillfully placed within the grid. In this context, the study of adversarial reprogramming in NCAs is shedding light on how AI systems can be manipulated and their functionality altered through precise interventions.
It's notable that the ruleset defining the interaction between cells plays a significant role in how easily they are influenced by adversarial injections. The application of these rules, which drive the cell's iterative updates, ultimately produces the complex global behaviour of the system. It's intriguing that injecting adversarial cells can trigger unpredictable responses, including chaotic behavior, which highlights a limitation in traditional cellular automata frameworks.
This research also hints that the dimension of the grid and the time-dependent nature of cell interactions play crucial roles in how an NCA responds to adversarial patterns. The dimensionality of the grid seems to either exacerbate or dampen the effects of adversarial inputs, which is worth further study.
Injecting adversarial cells during specific stages of an NCA's operations can have vastly different effects on the system compared to injecting them at other points in time. Sometimes, it leads to system instability or failure, while in other scenarios it can create positive outcomes.
This research on adversarial injection points towards a significant gap in current machine learning practices—the lack of emphasis on incorporating adversarial robustness into the training phase. If we're going to develop NCA models for use in real-world applications, we need to explore methods for improving their resilience to these kinds of attacks during their development stage.
Neural Cellular Automata New Frontiers in Adversarial Reprogramming for AI Systems - Self-Organizing Properties Mimicking Biological Processes
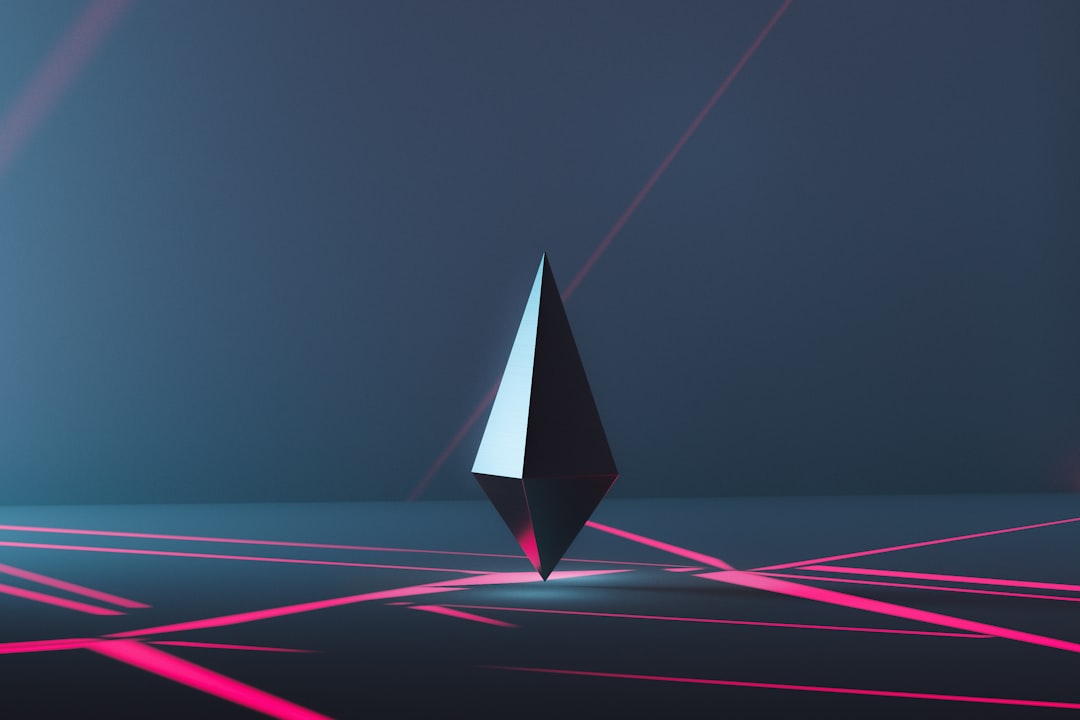
The capacity of systems to spontaneously self-organize, mirroring biological processes like embryogenesis, is a core principle being explored within the field of Neural Cellular Automata (NCA). NCAs demonstrate the ability to develop complex structures from simple starting points, much like biological systems form intricate patterns during growth. This inherent self-organizational behavior presents a powerful avenue for developing AI systems capable of generating structures and behaviors that are difficult to program explicitly. However, this same characteristic also presents a challenge. The unpredictable nature of NCA growth processes makes it difficult to fully control and anticipate the outputs of these systems.
The potential for adversarial manipulation adds another layer of complexity to this field. The ability to inject or alter grid states in a way that subtly redirects the self-organizing process is a major concern. Researchers are exploring various techniques that attempt to reprogram the behaviors of these systems through targeted interventions. These explorations highlight a critical need to enhance the robustness of NCAs, as unpredictable behavior and vulnerabilities to outside interference can severely impact the reliability of NCA-based applications.
While the ability to mimic biological self-organization holds incredible potential for advancements in AI, the challenges associated with unpredictable behavior and adversarial vulnerabilities must be addressed to build truly trustworthy and reliable systems in domains where outcomes are critical. This research pushes towards a future where the design and implementation of NCAs consider these inherent traits and vulnerabilities, ultimately paving the way for their safe and impactful use across diverse applications.
Self-organizing systems, like those seen during the development of embryos, exhibit the ability to form complex structures—segments, spirals, stripes, and spots—through cell-to-cell coordination. Neural Cellular Automata (NCA) models are inspired by this biological process, and they've shown an intriguing capability to develop artificial cells into complex structures, including images and even functional machines. However, while NCAs offer a robust computational approach, their inherent uncontrollability during the growth process presents a challenge in managing the final output.
Researchers have begun to explore different kinds of adversarial attacks on NCAs, two of which are injecting adversarial cells into the grid of a pretrained model or subtly adjusting the global state of the cells within that grid. There's also an emerging approach to designing self-organizing systems based on behavior, rather than a strictly structural focus. This new way of thinking is analogous to how morphogen gradients guide cell differentiation in biological systems. Furthermore, differentiable programming optimization techniques are now being used to learn how agents within self-organizing systems should behave. This opens up an interesting avenue for combining machine learning and developmental biology.
Interestingly, self-organization is not limited to embryological processes, it is also found in the mature tissues of adult organisms. This highlights the fundamental importance of self-organization in a wide range of biological systems. NCAs are end-to-end differentiable, meaning that we can optimize the neural networks within them for specific tasks related to self-organization. This characteristic makes them attractive for further investigation. The development of artificial neural network-based cellular automata that can evolve over time seems to hold a lot of potential. It is still early days, but this field could provide a promising direction for future research.
Over the past couple of decades, we've begun to see various systems that display these self-organizing qualities in different domains, which shows us that we're gaining a deeper and more nuanced understanding of this intriguing phenomenon. It will be interesting to see how this field develops further in coming years.
Neural Cellular Automata New Frontiers in Adversarial Reprogramming for AI Systems - Transformer-Based Architectures Enhancing NCA Robustness
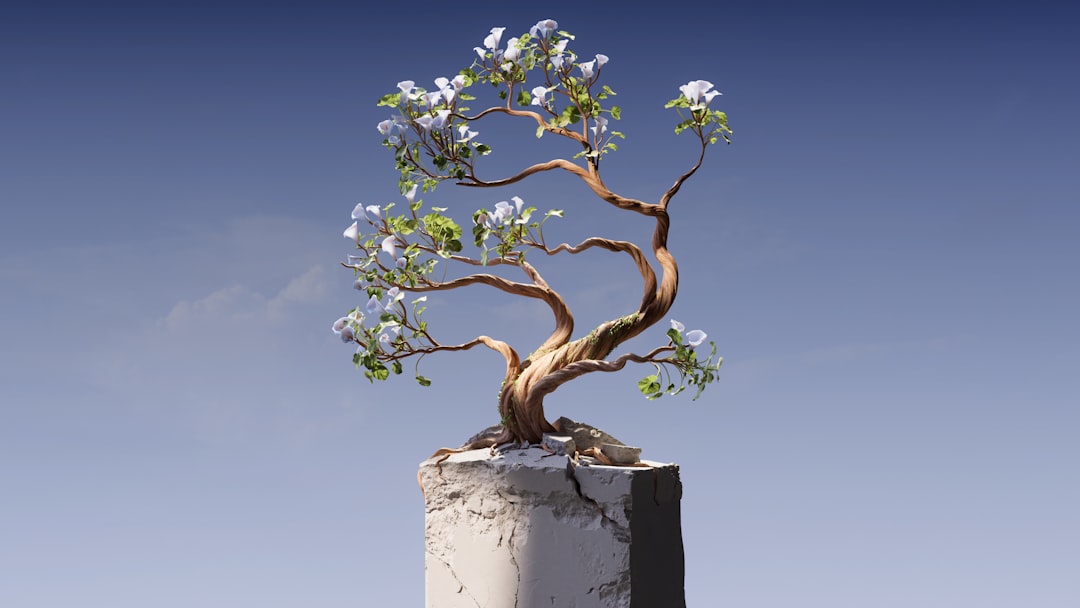
The combination of Transformer architectures with Neural Cellular Automata (NCA) is a notable step forward in bolstering the robustness of these models. By incorporating attention mechanisms, these designs allow NCAs to not only excel at handling local interactions but also improve their ability to recognize global connections within data. This in turn leads to greater adaptability across a variety of tasks. The creation of architectures like Vision Transformer Cellular Automata (ViTCA) exemplifies how attention-based enhancements can lessen the impact of things like noise and improve the models' ability to remove it. However, it's important to recognize that while these innovations enhance the NCA framework, they don't eliminate existing vulnerabilities to adversarial attacks. This suggests that as these architectures become more intricate, the importance of building strong defenses against manipulation also increases. This exploration of NCAs augmented by Transformers sheds light on new possibilities in the area of adversarial reprogramming and underscores the essential need for reliable and high-performing AI systems. While the improvements are significant, this area of research is still in its early phases and open questions remain regarding optimal methods for achieving and evaluating robustness against future adversarial attacks.
Neural Cellular Automata (NCA), initially conceived as a lightweight architecture modeling local cell interactions, have seen significant advancements through the integration of transformer-based architectures. These architectures, widely used in image classification and natural language processing, introduce self-attention mechanisms into NCAs. This allows the model to consider interactions across the entire grid rather than just locally, which may enhance the ability to adapt to various situations. However, the exact impact on robustness seems to vary greatly depending on the type of attack and how the grid is set up. Predicting the performance of transformer-based NCAs in different contexts becomes difficult due to this unpredictable variability.
Interestingly, these transformer-inspired NCAs can exhibit emergent behaviors mimicking aspects of natural phenomena, like flocks of birds or the growth of biological organisms. This makes them attractive for modeling complex dynamic systems using computational methods that surpass what's possible with traditional techniques. But, it also introduces a problem: the attention mechanism used to capture interactions across the whole grid can cause cells within the grid to favor interactions with distant cells over more nearby ones. This can lead to counterintuitive or unexpected behaviors, particularly when NCAs are under attack.
Similar to more conventional deep learning models, NCAs that incorporate transformer architectures have vulnerabilities to adversarial manipulation. This means that it's possible to interfere with these systems through clever techniques. This can be problematic for situations where reliability is paramount. Also, the size of the grid used in these systems has a major impact on both how the system behaves naturally and its resilience to attacks. Higher-dimensional grids can either amplify or reduce the impact of adversarial actions, making it hard to choose the best setup for optimal performance.
A connection between morphogen gradients in biology and gradient-based optimization in machine learning offers a new angle for improving NCAs. This perspective proposes that focusing on tweaking NCA parameters with an eye towards controlling behavior, rather than rigidly controlling structure, could lead to better self-organization. Also, the timing of adversarial attacks can heavily influence the outcome. Attacking NCAs during certain points in their operation can cause chaos or complete failure, while other attacks may have a positive impact. This leads us to think that more complex models are needed that can handle adversarial events at any point in the process.
The increasing role of differentiable programming within NCA optimization has enabled fine-tuning of cell behaviors within a given environment. This opens up new possibilities for adapting NCAs to perform better in diverse real-world tasks. However, the very unpredictability inherent in emergent behaviors in NCAs creates a challenge when it comes to making sure the system is secure. The difficulty lies in designing strong protection against adversarial tactics, especially when system reliability is critical. This challenge needs to be carefully considered if we want to apply these kinds of NCAs in situations where failure is not an option.
Neural Cellular Automata New Frontiers in Adversarial Reprogramming for AI Systems - NCA Performance in Pattern Reproduction Under Adversarial Conditions
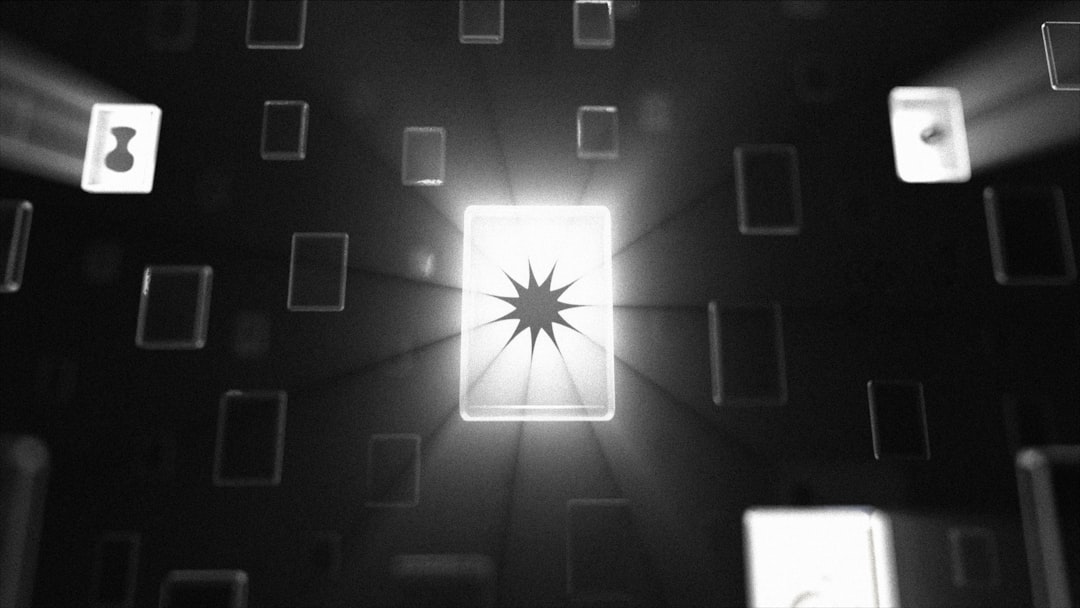
Neural Cellular Automata (NCAs) show potential in generating intricate patterns, even when faced with adversarial interference. This ability stems from their design, where local cell interactions lead to complex global behavior. This characteristic contributes to their generalizability and resistance to noise, but it also exposes them to adversarial techniques, such as strategically introducing malicious cells or modifying the overall state of the cellular grid. While variations like isotropic NCAs strive to reduce complexities like cell orientation, which could potentially increase their robustness, the full extent of their effectiveness in these situations is still unclear. This uncertainty raises questions regarding their dependability in real-world settings that necessitate robust security. The complex interplay of emergent behavior and adversarial attacks highlights the need for further research into enhancing the resilience of NCA models and developing countermeasures against targeted attacks. It's crucial to understand their strengths and limitations to apply them effectively in scenarios where their reliability is paramount.
Neural Cellular Automata (NCA) have shown a fascinating ability to mirror biological processes like pattern formation during embryonic development. This capability highlights their potential to model complex behaviors and structures from a set of basic rules. It's intriguing to think how such models can help us gain a deeper understanding of developmental biology, itself a complex field with many open questions.
However, the introduction of adversarial techniques, specifically cell injection, into NCA grids has revealed a vulnerability in these systems. We've found that even small changes to the grid, introducing just a few "adversarial cells," can significantly alter an NCA's behavior. This calls into question the robustness of NCA models, especially in applications where reliability is paramount. It appears that the current design methodologies may not be sufficient to mitigate this type of attack.
Interestingly, the grid's dimensionality plays a crucial role in determining how it responds to adversarial injections. We've observed that in higher-dimensional grids, the effects of adversarial attacks are either amplified or diminished. This variability suggests that the way we design the underlying grid structure is a major factor in ensuring the NCA's reliability. It seems that there's a lot we still need to learn about optimizing grid structure for optimal robustness.
One of the more unexpected observations from these experiments is the appearance of chaotic behaviors in response to certain adversarial cell injection patterns. It appears that injecting cells at specific times during an NCA's operation can create situations where the system behaves unpredictably. This creates a significant problem when it comes to ensuring that the system can operate reliably under attack.
The integration of transformer-based architectures, as seen in Vision Transformer Cellular Automata (ViTCA), has been an important step forward in addressing some of the weaknesses in earlier NCA implementations. By employing attention mechanisms, these new designs can handle both local and global connections within the data. These enhancements seem to improve performance on several benchmarks, reducing the impact of noise, for example. However, the concern remains that even with these improvements, NCAs are still susceptible to adversarial attacks. The greater complexity of these newer architectures just means that we need to be even more mindful of developing ways to protect against manipulations.
The combination of complexity and potential vulnerability in NCAs reveals a bit of a paradox. As these models grow more complex and feature-rich, their presumed robustness seems to decline. This implies that there is a need to rethink how we measure the robustness of these systems. In many applications, the systems we build are required to maintain stable performance over time, but the intrinsic nature of NCAs, especially the unpredictable behaviors they exhibit, complicates this requirement.
We've also seen that differentiable programming techniques allow us to tune the behavior of NCAs more precisely, tailoring them to specific tasks. This creates a continuous process of optimization and adaptation, which is quite remarkable. But this very dynamism might also introduce hidden vulnerabilities. The continuous evolution of NCAs can lead to unexpected interactions that can compromise their security.
A fascinating finding is that NCAs can mimic swarm intelligence, such as flocking patterns seen in nature. This makes them particularly appealing for modeling complex behaviors. However, we must keep in mind that it also leads to questions about how well we can control and predict the behaviors these systems might produce. This could be problematic if these systems are deployed in critical applications.
When NCAs are subjected to adversarial attacks, the balance between local and global interactions takes on an added importance. Some configurations seem to lead to the model's favoring of interactions with distant cells over local ones, leading to a potential breakdown of coherence in the system. This specific type of challenge in the design of NCAs requires targeted attention during the training phase to ensure model stability.
By studying how adversarial cells impact the behavior of NCAs, we can gain valuable insights into not just the vulnerabilities of these models but also into how we can improve them. This pursuit highlights the dual challenge researchers face: We must simultaneously find ways to bolster the security of these systems while ensuring that they retain their powerful emergent capabilities for useful practical applications. This is a challenge that will require innovative solutions as we move forward in developing more complex AI systems.
Neural Cellular Automata New Frontiers in Adversarial Reprogramming for AI Systems - Dynamic Environments Fostering Coexistence of Original and New CA Models
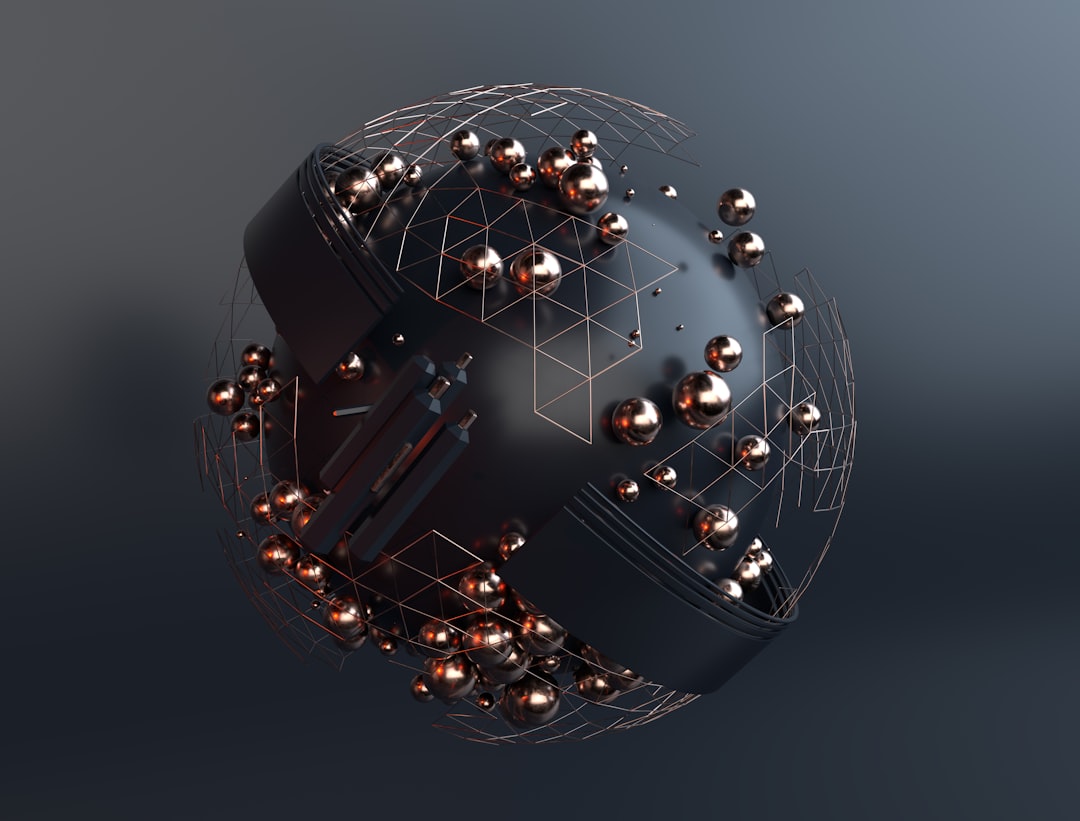
Dynamic environments serve as a critical testing ground for the interplay between established Cellular Automata (CA) models and the newer Neural Cellular Automata (NCA) paradigms. Within these environments, original CA rules and the adaptive capabilities of NCAs can coexist and influence one another, fostering the development of innovative computational approaches. The integration of deep learning into NCAs allows them to learn intricate update rules, leading to increased adaptability and applicability across a broader range of tasks. However, this adaptability comes with inherent challenges. The dynamic and often unpredictable emergent behaviors of NCAs in complex settings pose a significant hurdle for understanding and controlling their outputs.
The research into how original CA principles and advanced NCA features interact in dynamic environments is a relatively new area of study. The findings will likely offer valuable insights into improving the robustness of these models for practical applications. At the same time, it highlights the potential vulnerability of NCAs to adversarial manipulation. The ability to inject malicious data into the systems or modify their overall states has raised serious questions about their reliability. Ultimately, researchers strive to leverage the strengths of both CA and NCA models while actively addressing their vulnerabilities. This will ensure that as these systems become more sophisticated and complex, their behavior remains predictable and secure within dynamic environments.
Cellular automata (CA) models, with their foundational role in simulating complex systems, have found a new life with the advent of Neural Cellular Automata (NCA). This fusion of established CA principles with the learning power of deep learning opens a unique avenue to model dynamic systems. However, the emergent behavior inherent in NCAs, while fascinating, also poses challenges for control and prediction. One such challenge stems from the unpredictable nature of these emergent patterns, making it difficult to reliably achieve desired outcomes, particularly in scenarios where robust performance is crucial.
One area of considerable interest and concern is the vulnerability of NCAs to adversarial manipulations. Injecting just a few strategically placed cells into the grid can dramatically alter the behavior of these models, even those that have been extensively pre-trained. This vulnerability highlights a key concern about robustness, particularly in systems requiring high reliability. The dimensionality of the NCA grid itself can play a significant role in either amplifying or mitigating these attacks. For example, higher-dimensional grids can lead to either a heightened sensitivity or a more resilient response to adversarial inputs. Further research in this area is needed to guide the design of robust NCAs in different applications.
Furthermore, we've discovered that certain adversarial cell injection patterns can result in unpredictable, or even chaotic behavior. This suggests that maintaining stability in the face of an attack is a major concern. It is encouraging that incorporating transformer-based architectures into NCAs, as seen in ViTCA, has shown promise in enhancing robustness, particularly in terms of handling global connections within the grid. However, the increased complexity introduces new challenges for security and robustness.
Just as biological systems exhibit self-organizing characteristics in processes like embryonic development, NCAs can model this type of behavior. However, their inherent uncontrollability during growth can lead to unexpected and often unpredictable outcomes, which can make it challenging to design NCAs for use in situations requiring specific and reliably predictable outcomes.
Another intriguing avenue comes from drawing parallels between biological morphogen gradients and machine learning's gradient-based optimization methods. This perspective proposes that focusing on fine-tuning the behaviors of NCAs rather than simply their structure could provide a better approach to self-organization. NCAs can also model swarm intelligence observed in nature, for instance, flocking patterns. While this capability is exciting for exploring complex behaviors, it further complicates our ability to predict and control these systems, particularly in critical applications.
Finally, the ongoing application of differentiable programming in NCA optimization allows for continuous adaptation and refinement. This constant fine-tuning of cell behaviors enables NCAs to be tailored to perform optimally in specific settings. But this flexibility and adaptability also comes at a cost. The continuous evolution of NCAs during their operational phase increases the risk of introducing vulnerabilities that could be exploited, highlighting the challenge of striking a balance between system optimization and security. Balancing these dynamic interactions, particularly in the face of adversarial actions, demands thoughtful consideration in the design and training phase to ensure NCAs are robust enough for the wide range of tasks they have the potential to address.
In conclusion, the field of NCA is rapidly evolving, showcasing immense potential for addressing complex challenges in diverse areas. However, as with any advanced technology, the need for a comprehensive understanding of their vulnerabilities, alongside ongoing research efforts, is paramount. This is especially crucial for developing secure and reliable systems that can be used in settings demanding a high degree of reliability and safety. It's an exciting area of research and it will be interesting to see where it leads in the future.
Experience error-free AI audio transcription that's faster and cheaper than human transcription and includes speaker recognition by default! (Get started for free)
More Posts from transcribethis.io: