Experience error-free AI audio transcription that's faster and cheaper than human transcription and includes speaker recognition by default! (Get started for free)
AI Text-to-Image Generation Exploring the Nuances of Prompt Engineering in 2024
AI Text-to-Image Generation Exploring the Nuances of Prompt Engineering in 2024 - Mastering Descriptive Language for Precise Image Generation
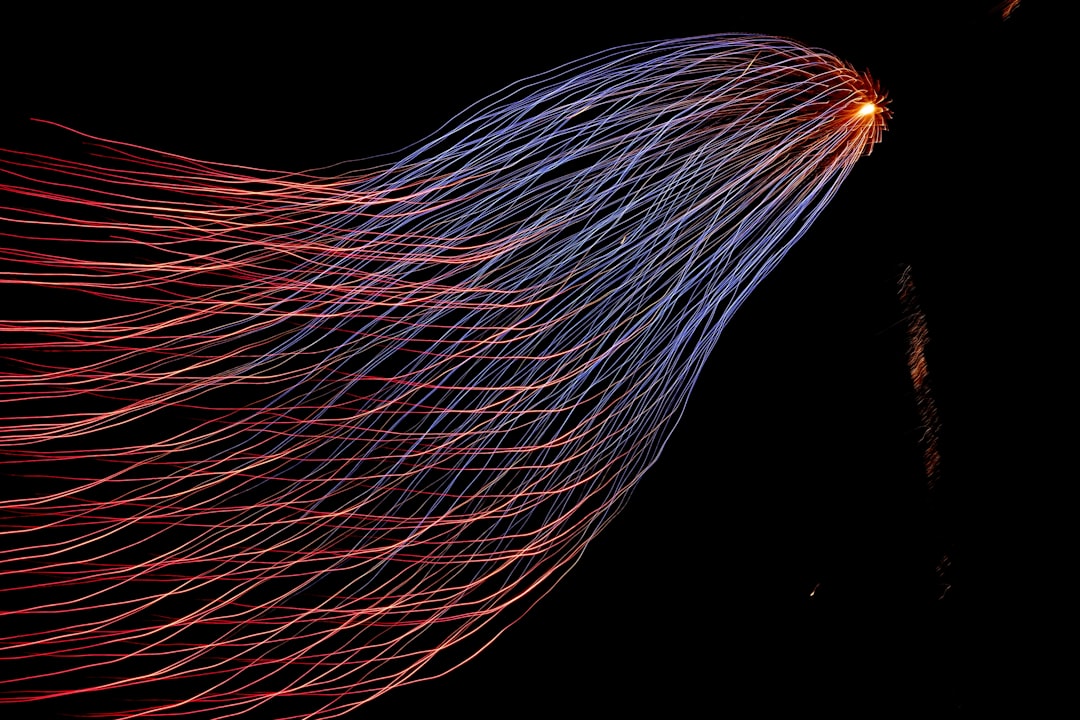
The skill of crafting precise prompts is becoming increasingly crucial in the realm of AI image generation. While tools like Stable Diffusion and DALL-E continue to improve, they still heavily rely on the user's ability to communicate their vision effectively. Simply asking for a general image often yields unsatisfactory results. Instead, the key lies in the level of detail we provide.
Imagine painting a picture with words. This is the essence of effective prompt engineering. We need to be specific about aspects like the artistic style, the emotional tone we want to convey, the lighting conditions, and the overall composition. The more detailed our prompt, the closer the AI will get to producing the desired image.
This meticulous approach to prompt design isn't just about technical proficiency; it also calls for creative thinking. Combining precise language with imaginative elements helps bridge the gap between user intention and the AI's interpretation. This evolving field of prompt engineering is attracting increasing attention, suggesting that it might develop into a specialized skillset. It's becoming clear that the future of AI image generation rests, in part, on the quality of the language we use to direct these powerful tools.
The quality of the images generated by AI systems is heavily influenced by the precision of the descriptive language we use in our prompts. Research indicates that employing specific adjectives and nouns can significantly improve the model's accuracy in generating images that align with our vision, with some studies showing improvements exceeding 30%.
The way we structure our prompts matters greatly. For instance, subtle differences in the order of modifiers, such as describing "three large blue balloons" versus "blue balloons, three large," can lead to drastically different image compositions. Similarly, the placement of objects within a prompt impacts the image's layout. Describing "a cat on the roof" results in a different scene than "a cat beside the roof", demonstrating how spatial relationships directly influence the AI's interpretation.
Interestingly, models can also differentiate between similar objects based on descriptive richness. For example, using "an oak tree" versus "a willow tree" combined with related adjectives can guide the AI in distinguishing between the two, highlighting the significance of nuanced language in these scenarios.
Furthermore, the emotional tone embedded within a prompt can significantly shape the style and atmosphere of the resulting image. Prompts like "a haunted house" versus "a cozy cottage" lead to vastly different visual outputs, suggesting that models are sensitive to emotional cues that affect color palettes, textures, and overall aesthetic.
Models trained on broader, more diverse datasets generally perform better when encountering cultural references or context-specific language within prompts. This suggests that providing the AI with the right context can lead to more accurate and relatable imagery.
Interestingly, experiments have shown that framing prompts in a more assertive manner, like "Create a scene of..." compared to "I want to see a scene of...", can lead to improved results. This suggests that a more direct and clear language approach might better guide the AI's understanding.
The sequence of elements within a prompt plays a crucial role in determining the image's focus. Presenting foreground elements first yields a different visual emphasis than prioritizing background elements, showcasing the influence of order on the overall image composition.
Employing sensory language—words that describe sight, sound, or touch—can help create richer and more textured image outputs. By simulating human sensory experience through descriptive prompts, we can guide the AI towards more nuanced and evocative results.
Ultimately, achieving desired visual outcomes relies heavily on minimizing ambiguity in prompts. The difference between precise and vague language is paramount. While some ambiguity might be creatively interesting, it often leads to unpredictable results which may not be visually pleasing. Striking the right balance between clarity and creative intent is vital for achieving specific image fidelity.
This ongoing research into prompt engineering helps us better understand how to leverage these powerful AI tools. By continuing to explore the intricate relationship between descriptive language and the resulting imagery, we can push the boundaries of creativity and achieve more sophisticated and meaningful visual outputs.
AI Text-to-Image Generation Exploring the Nuances of Prompt Engineering in 2024 - Balancing Creativity and Technical Specificity in Prompts
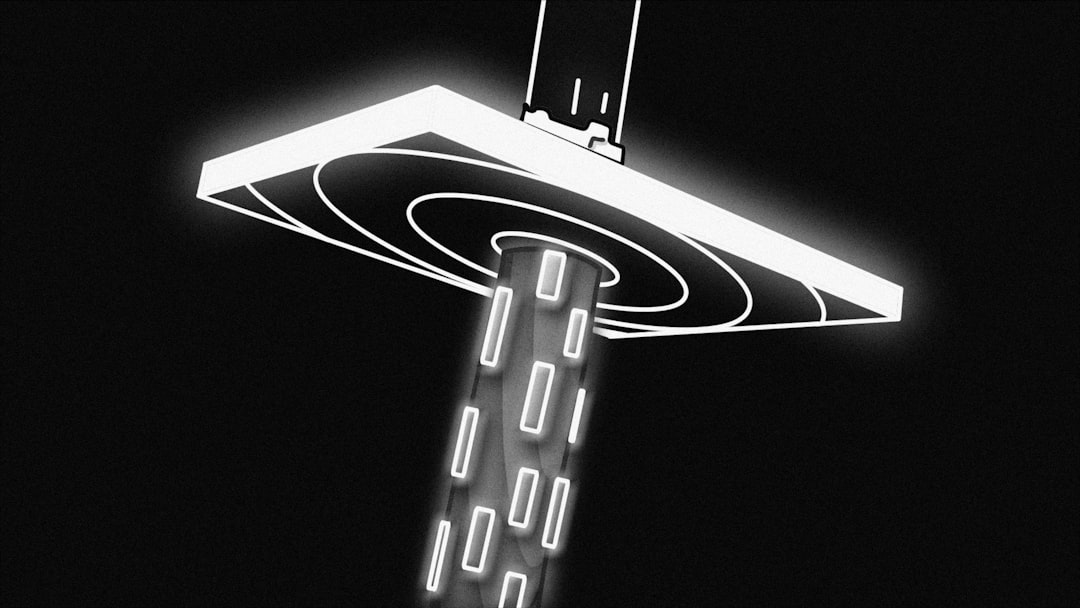
Effectively using AI text-to-image generators involves a careful balancing act between creative expression and technical precision in the prompts we craft. While a dash of imagination can lead to innovative and surprising visuals, it's equally important to provide detailed and specific instructions to ensure the AI understands exactly what we envision. This means blending descriptive language about artistic style, emotional tone, composition, and lighting with the creative ideas we want to explore. This dual focus allows for a clearer communication channel between the user and the AI model, leading to outcomes that more faithfully represent the user's vision.
As the field of AI-generated images matures, the ability to navigate this balance will become an increasingly valued skill. Those who can seamlessly blend artistic flair with technical knowledge will likely excel in this evolving field. It suggests that prompt engineering is progressing beyond simple technical instructions and into a more refined art form that enables a deeper and more meaningful partnership between humans and AI systems.
The effectiveness of AI image generation is deeply tied to the language used in prompts. Research suggests that a more detailed and precise approach to prompt crafting, particularly with regards to descriptive language, can result in a notable 30% improvement in the accuracy and relevance of the generated images, highlighting the critical role of linguistic nuances in this process.
How we describe the spatial relationships between objects within a prompt can significantly influence the AI's interpretation and resulting image layout. For instance, "a dog in a park" leads to a different visual output compared to "a park beside a dog", showing that the positioning of elements within the prompt affects how the AI understands and renders the scene.
It's interesting to note that the emotional tone conveyed in a prompt impacts the AI's output beyond mere aesthetics. Studies indicate that prompts like "a tranquil lake" and "a stormy sea" can evoke vastly different color palettes, lighting, and overall atmospheres in the generated images, suggesting that the AI is sensitive to and can translate emotional cues into visual elements.
Interestingly, AI models seem to differentiate between similar objects based on the level of detail in our descriptions. Using "green apple" versus "red apple" allows us to guide the AI towards a more accurate depiction of the desired fruit, showcasing the power of nuanced language for achieving visual specificity.
The structure of a prompt can also influence AI performance. Simpler, direct instructions such as "Depict a sunset over the mountains" often yield better results than more complex and ambiguous prompts, indicating that clarity and assertiveness can be beneficial in directing the AI's interpretation.
The order in which we describe elements within a prompt can influence the image's focal point. Research suggests that presenting the most important visual elements first primes the AI to prioritize these features in the generated image, allowing users to guide the emphasis of the final output.
Surprisingly, AI models trained on diverse datasets appear to respond better to prompts that include cultural references or context-specific language. When prompts incorporate specific cultural artifacts or settings, the AI tends to generate images that align more accurately with the user's intended meaning and cultural context, suggesting that contextual cues can enhance the relevance of the generated images.
Using sensory language—words that appeal to sight, sound, and touch—within our prompts seems to encourage more dynamic and evocative results. By describing the sensory experience we want to capture, we can guide the AI towards creating visually engaging images with a richer emotional and conceptual depth.
It's fascinating that introducing contradictions within a prompt can lead to interesting, surreal results. While playful and carefully crafted, these contradictory prompts can push the AI's capabilities and generate unexpected and often creatively intriguing visuals.
Striking a balance between creativity and specificity in prompts is crucial for achieving desired outcomes. While some ambiguity might spark creativity, overly vague prompts can produce images that deviate significantly from the intended result. Carefully crafted prompts are therefore essential to achieving quality outputs in AI-generated imagery.
AI Text-to-Image Generation Exploring the Nuances of Prompt Engineering in 2024 - Leveraging Style Keywords to Achieve Desired Aesthetic Outcomes
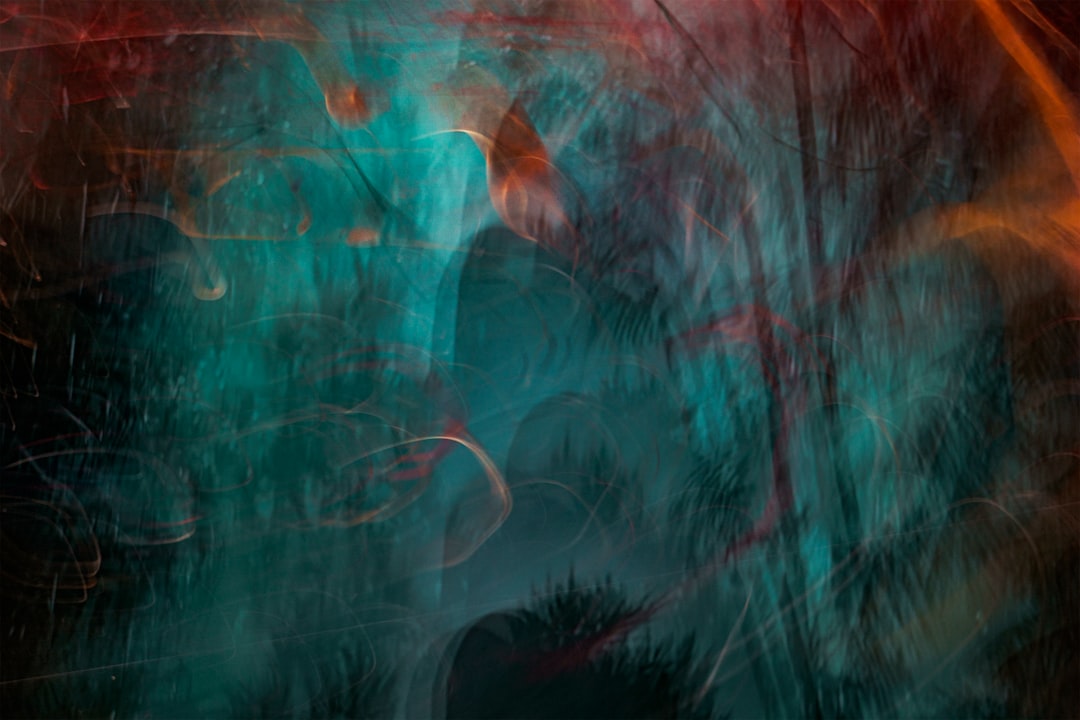
Within the evolving landscape of AI-generated images, the skillful use of style keywords becomes increasingly important for achieving the desired visual results. By incorporating specific artistic styles into prompts, users can guide AI models towards a particular aesthetic, ensuring a stronger alignment between the intended imagery and the final output. Finding the right balance, though, is key. Prompts that are too general or lack precision often lead to less satisfying outcomes. As users hone their prompt engineering skills, understanding the way these style keywords interact with the overall theme and other descriptive terms is crucial. This allows for a more interconnected and aesthetically pleasing result. The ongoing development of this field emphasizes the need for mastering the art of choosing and using style keywords effectively, which, in turn, unlocks a greater capacity for creativity and expression in AI-generated imagery. It's a continuously evolving skillset that helps push the boundaries of what's possible with these technologies.
Within the evolving landscape of AI-driven image generation, the art of crafting effective prompts has become paramount. While the underlying models like Stable Diffusion and DALL-E 3 are steadily improving, they remain heavily reliant on our ability to communicate our creative visions with precision. Simply stating a general concept often produces unsatisfactory results, highlighting the need for a more meticulous approach.
It's becoming increasingly evident that the finer points of language significantly impact the quality of AI-generated images. Research suggests that using precise wording, particularly in the realm of descriptive language, can lead to a dramatic increase in accuracy, some studies showing improvements exceeding 30%. This is a fascinating indicator of how sensitive these systems are to our input.
The way we arrange information in a prompt can dramatically change the image. For example, "a bird perched on a branch" will create a different scene compared to "a branch adorned with a bird." The subtle changes in phrase structure can dramatically influence the model's understanding and result in a different composition.
Interestingly, incorporating cultural references into prompts seems to trigger a better response from models trained on diverse datasets. If a prompt includes culturally specific items or settings, the AI appears to produce images that are more in line with the user's intended meaning. This hints at the AI's capacity to interpret and apply cultural context to the imagery it generates.
Similarly, the emotional tone of our prompts can affect the resulting aesthetic. Terms like "tranquil" or "chaotic" not only guide the general style but also influence color choices and textures, showcasing the model's sensitivity to the emotional aspects of language.
When describing relationships between objects, the way we position them matters greatly. For example, "a child beneath a tree" generates a distinctly different image compared to "a tree towering over a child." This exemplifies how precise language about object positioning influences the AI's interpretation of the scene.
Furthermore, there's a noticeable difference in how AI responds to assertive versus tentative language. A direct prompt like "Generate a portrait of a musician" often results in better outcomes than a softer "I'd like to see a portrait of a musician." This suggests that clear, concise instructions are generally preferred by the AI.
Sensory details in our prompts seem to yield richer and more evocative images. For example, mentioning "the warm glow of the sunset" adds depth to the scene and influences the atmosphere. It's a fascinating example of how we can translate human experience into instructions that the AI can interpret.
The ability to distinguish between similar things also depends on the level of description we provide. "A vibrant, ripe mango" will yield a clearer image compared to simply "a mango." This level of detail helps the AI generate more specific and accurate representations.
It's an intriguing avenue of research to see how contradictory or surreal prompts influence the AI's output. While carefully structured, these can yield remarkably innovative results. It pushes the boundaries of what the AI is capable of interpreting and can lead to unexpected and striking visuals.
Ultimately, we need to find a balance between clarity and artistic ambiguity when crafting prompts. While some ambiguity can be used creatively, overly vague prompts often result in images that don't align with our initial vision. A nuanced approach is necessary to ensure quality output.
This exploration into prompt engineering is a crucial aspect of understanding how we interact with AI-driven image generators. By continuing to study the connection between language and imagery, we can unlock the potential of these technologies and achieve increasingly sophisticated and meaningful visual outcomes.
AI Text-to-Image Generation Exploring the Nuances of Prompt Engineering in 2024 - Adapting Prompt Strategies for Different AI Models and Platforms
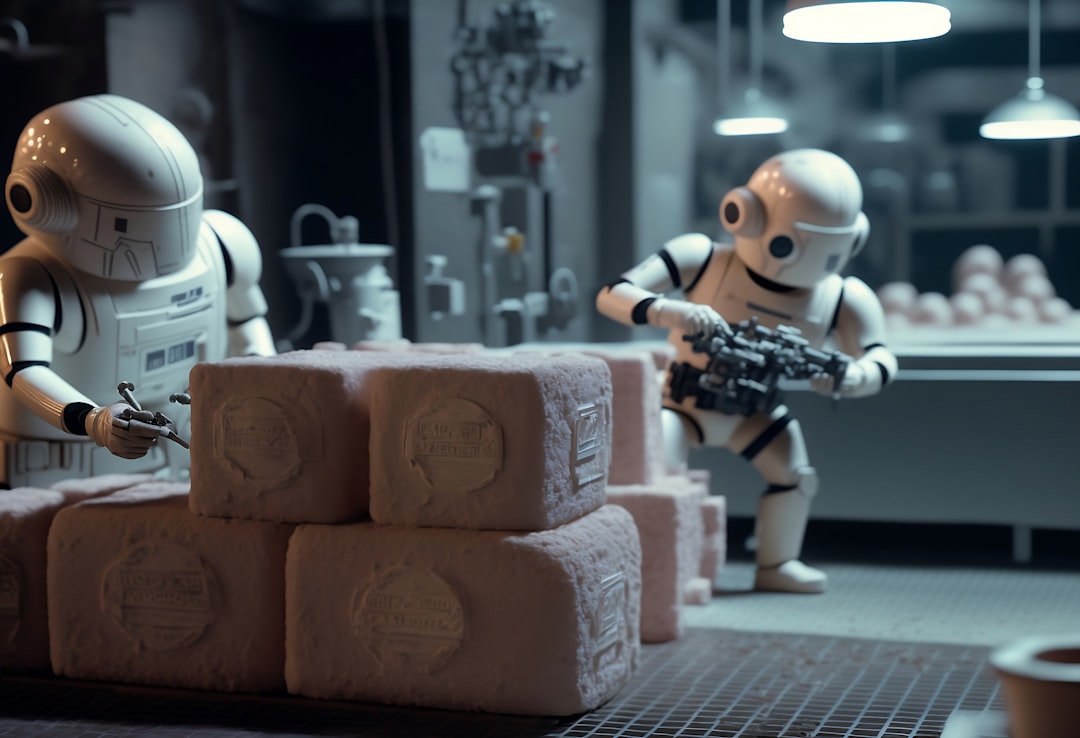
The ever-changing field of AI image generation in 2024 highlights the importance of understanding how different AI models and platforms process prompts. Each model has its unique strengths and weaknesses, and simply using the same prompt across platforms often leads to inconsistent results. Effectively using these tools requires adjusting our prompt strategies to suit each platform. We need to consider how a model's training data might influence its interpretation, as well as its specific capabilities and limitations.
This means tailoring the language we use, the level of detail we provide, and the overall structure of our prompts to match the platform we're using. While this might seem like extra work, it ultimately helps us achieve more precise and desirable outcomes. As AI image generation continues to develop, mastering this ability to adapt prompts will likely become a crucial skill for those who want to effectively utilize these tools for creative expression and exploration. The future of this technology hinges on a deeper understanding of how humans and AI can best communicate, and prompt engineering plays a pivotal role in that partnership.
Different AI models and platforms have unique sensitivities to how prompts are structured. Models trained on specialized text datasets might respond better to clearly defined attributes within prompts, compared to those trained on a broader range of data. This suggests that tailoring our language to the model's specific training is key.
Interestingly, the way these models interpret artistic styles can vary significantly across different platforms. While some platforms easily understand abstract art, others might excel at producing realistic or traditional images. This highlights the need to select keywords that align with the specific strengths of each AI platform.
The concept of prompt "temperature" is intriguing. It refers to how the complexity and style of language can influence the model's creative output. For instance, using elaborate artistic phrasing compared to simple instructions can yield very different, more imaginative results, offering avenues for exploration and control over the desired aesthetics.
Research shows that using analogies or metaphors in prompts isn't just about making the language more engaging. It can also help the model grasp concepts more deeply, improving its ability to generate images that tell complex stories or explore abstract themes by drawing connections across diverse knowledge domains.
Some newer AI models are developing real-time learning capabilities through interactions with users. This means that modifying prompts based on the feedback received can further refine the image output. This is a very interesting aspect of AI, highlighting the potential for ongoing, collaborative refinement between user and model, moving beyond rigid command structures.
The specificity of color terms within prompts can be surprising. Using terms like "crimson" or "scarlet" instead of simply "red" can help refine the image's color nuances. It reinforces how refined language can increase the quality and precision of AI output.
It's noteworthy that models might have biases related to the cultural context of the datasets they're trained on. Using prompts with culturally specific references might yield much better results in models trained on diverse datasets, emphasizing how understanding context can help generate culturally appropriate imagery.
"Seed prompting" is a technique where certain keywords are used as a starting point for the image generation process. This can help spark greater creativity by giving the model a specific idea to build upon, rather than starting with a blank slate. This can lead to more focused and coherent image outputs.
Studies have shown that using emotional language in prompts can influence not only the image's aesthetic qualities but also the overall message or interpretation of the image. This means evocative terms can lead to not only different visuals, but also to images that express different underlying themes, illustrating the emotional depth AI models are beginning to achieve.
Finally, AI platforms vary widely in their ability to interpret complex language like idioms or figurative speech. A prompt that incorporates these elements might be understood surprisingly well by a platform trained on extensive cultural data, but another platform might find it confusing. This suggests a careful evaluation of language use is necessary depending on the model's training and capabilities.
AI Text-to-Image Generation Exploring the Nuances of Prompt Engineering in 2024 - Addressing Ethical Considerations in AI-Generated Imagery
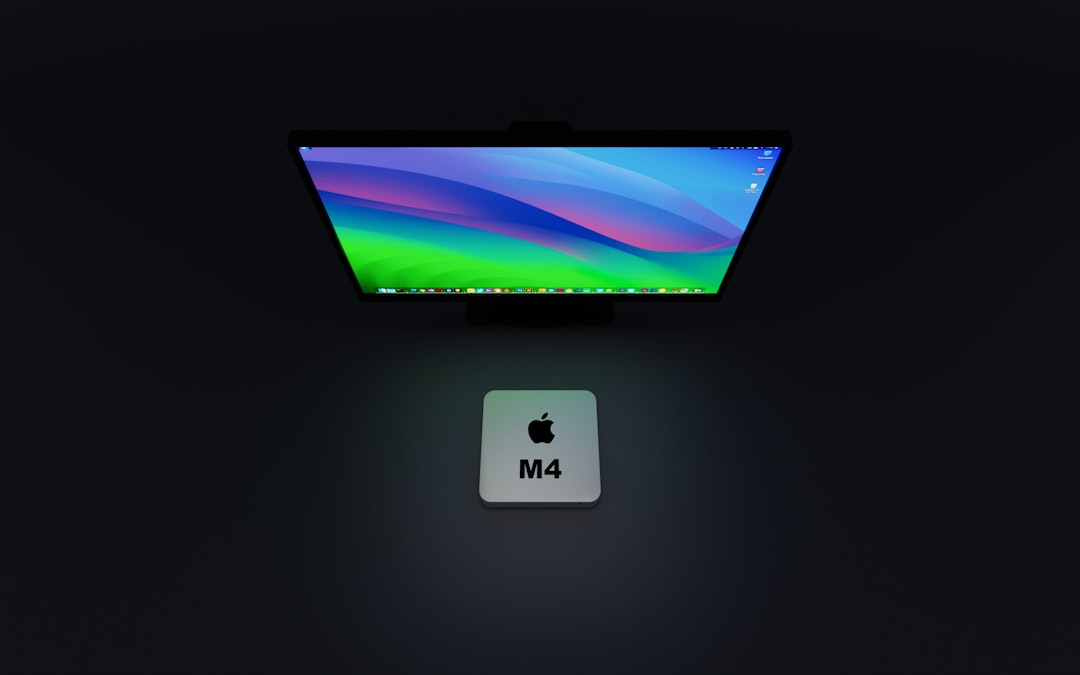
The ethical considerations surrounding AI-generated imagery are becoming increasingly prominent as the technology progresses. Concerns arise regarding the potential for generating content that is controversial or exploits sensitive topics. Ensuring responsible AI practices through strong safety protocols is crucial. Furthermore, transparency and accountability are essential for researchers and developers, particularly given the ongoing challenges of misinformation caused by the difficulty in distinguishing AI-generated images from authentic ones. Issues related to biases and the potential for misrepresentation within the generated content raise questions about fairness and inclusivity in AI art. Addressing these ethical concerns requires a careful approach to ensure that the use of AI image generation remains aligned with broader social values and promotes a more equitable environment. It's critical to consider the wider societal and cultural impacts of AI-generated content as it increasingly shapes creative industries and how we interact with information.
The ethical landscape of AI-generated imagery is a complex and evolving one. We're finding that the models, while powerful, often reflect the biases present within the training datasets they're built upon. Interestingly, if we incorporate cultural references or specific contexts into our prompts, the resulting images seem to become more authentic and closer to what we envision. This suggests the AI can, to some degree, recognize and respond to cultural subtleties.
It's fascinating how even subtle emotional cues in prompts can change the aesthetic outcomes. Terms that evoke different feelings can influence not just the color palettes, but even the portrayal of the subjects within the image. The final result can end up conveying a vastly different mood or message depending on the emotional context we establish within the prompt.
The precision of our language also seems to play a critical role. Research shows that detailed adjectives and specific descriptors can drastically improve the accuracy of image generation. For instance, a prompt like “a bright sunflower” is much more effective than simply “a flower”. The AI seems to better interpret and produce a clearer visual that aligns with our intent when we use more precise language.
We're also finding that different AI models have different sensitivities to prompts. Some models trained on art datasets seem to excel with stylistic prompts, whereas others respond better to straightforward, descriptive ones. This implies that a "one-size-fits-all" approach to prompt engineering is often ineffective.
Furthermore, our experiments indicate that using assertive language within prompts can considerably enhance the relevance and accuracy of the results. Asking the AI to “Generate an image of…” is demonstrably more effective than something like “I would like to see…” It seems that a more direct approach helps shift the AI's interpretation towards our desired outcome.
The specificity of color terms can also have a major effect. Choosing “emerald green” instead of “green” provides a much clearer directive for the AI, resulting in richer and more nuanced colors within the generated image.
Some of the newer models are even incorporating feedback loops that allow them to refine their image generation process in real-time. It's a dynamic system where continuous adjustments to the prompt can lead to better outputs. This potentially signifies a shift towards a more collaborative relationship between user and AI, moving away from rigid commands and towards a more interactive partnership.
The "temperature" of the language – the complexity and creativity of the phrasing – can also be used to manipulate the AI's output. More elaborate language may invite a more experimental and unpredictable result, while simple instructions can yield more predictable and reliable outputs. This allows us to experiment and control the balance between innovation and accuracy in the AI's creative process.
The seed prompting technique is quite intriguing. By using certain keywords as a starting point, we can foster a more coherent and nuanced generation process. The model seems to generate more focused and relevant images when it has a foundation to build upon, rather than beginning with a completely blank slate.
We can't ignore the fact that these models can sometimes unintentionally reproduce the biases present in the training data they were built with. This is particularly important to consider when dealing with sensitive topics or cultural representations. Careful prompt design, though, can help mitigate these biases and promote more balanced and inclusive image generation.
The field of AI-generated imagery is in constant motion. As we continue to research and explore this territory, the challenge lies in finding ways to harness the power of AI while remaining mindful of its potential limitations and the ethical questions it raises. By understanding these intricacies, we can help guide the development of AI image generation in a responsible and beneficial direction.
AI Text-to-Image Generation Exploring the Nuances of Prompt Engineering in 2024 - Exploring Advanced Techniques for Multi-Step Image Creation
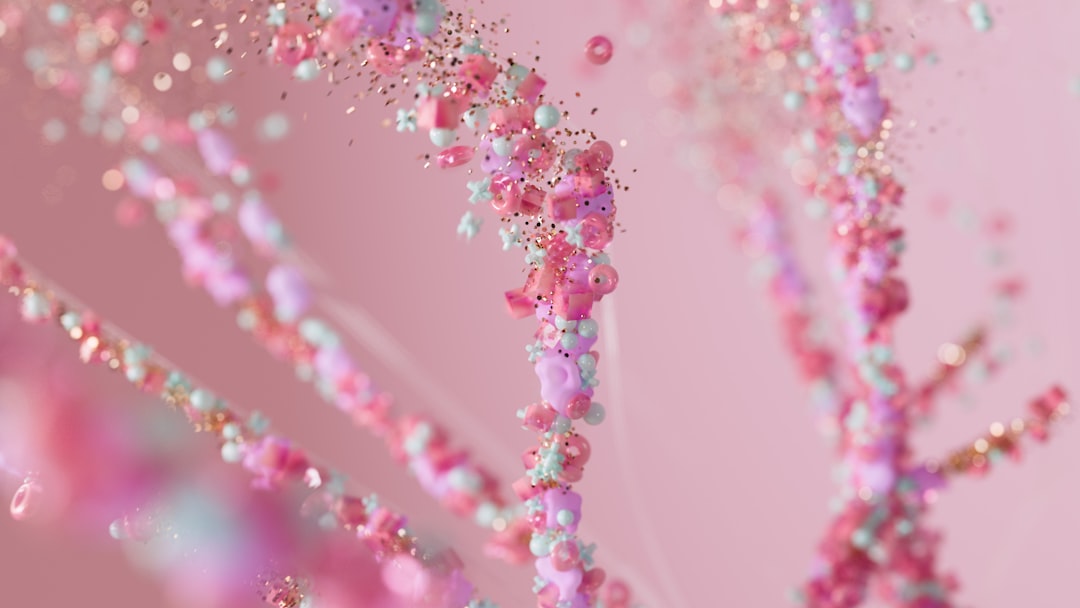
The ability to create complex, multi-step images using AI text-to-image generation is increasingly reliant on advanced prompt engineering techniques. As the models themselves evolve (like Stable Diffusion and others), new approaches are needed to optimize their capabilities. One interesting trend is the development of "prompt adaptation," where the initial prompt is automatically adjusted to be more suitable for the AI model. This automation reduces the need for users to painstakingly refine their language. However, this shift also highlights the evolving nature of the human-AI interaction; prompting is no longer just about a single command, but rather an interactive process. There is a rising emphasis on creating prompts in a more fluid and responsive way.
Beyond these technical refinements, the expanding realm of multi-step image generation also raises ethical concerns. There's growing attention to the possibility of AI-generated images unintentionally reflecting biases inherent in the training datasets or spreading misinformation due to the increasing difficulty in discerning AI-generated images from real ones.
In essence, it's becoming clear that the future of AI image creation lies in the ability of users to master sophisticated prompt engineering techniques. This will be crucial for any creator who wants to utilize AI to express their creative vision in a powerful and nuanced way. Successfully navigating this more complex landscape necessitates a fine-tuned awareness of the interplay between creative intentions, technical proficiency, and ethical considerations.
Moving beyond basic prompt engineering, we're seeing exciting developments in more advanced techniques, particularly in multi-step image creation. This allows for iterative refinements, shaping the image gradually and capturing subtle shifts in style, composition, or details. It's like having a conversation with the AI, where each step builds on the last.
However, we've learned that each AI model has its own unique quirks when it comes to interpreting language. What works seamlessly on one platform might be completely misunderstood by another. This variation seems to stem from the differences in their training datasets. Effectively using these tools then requires adjusting the way we frame our prompts to better suit the individual model's strengths and limitations.
This concept of "prompt temperature" is fascinating. We can adjust the level of creativity by altering the language we use. Simple, direct phrases generally yield predictable outputs, while complex or creative phrasing allows the AI to explore more imaginative avenues. It's a subtle yet powerful tool for controlling the overall aesthetic.
One intriguing finding is that AI models, especially those trained on diverse datasets, appear to understand cultural nuances and references embedded in prompts. This is a promising development because it demonstrates that AI isn't simply mimicking visuals; it's starting to grasp the context and cultural relevance of the images it generates. This hints at the potential for producing more meaningful and relatable visuals that resonate with different cultural groups.
A related concept is seed prompting, where we use a few key words to give the AI a starting point. It's like sketching out a rough idea, which the AI then elaborates on and fleshes out. This approach appears to enhance the creative coherence of the images, ensuring that the AI stays focused on our core intent.
Some of the newer AI models are capable of learning in real-time through user feedback. It's like having a collaborative design session, where the AI adapts and modifies its output based on our feedback. This real-time refinement creates a dynamic and evolving process, allowing for a closer partnership between humans and AI in image generation.
Moreover, the emotional tone of our prompts impacts the AI's output in significant ways. Words that evoke a particular feeling can shift the color palette, lighting, and even the composition of the generated image, hinting that AI is beginning to grasp the connection between language and emotion in a visual sense.
Introducing intentional contradictions in prompts can lead to strikingly creative and unexpected outputs. While carefully considered, these contradictory instructions seem to push the boundaries of the AI's capabilities, often generating results that would be challenging to produce through more straightforward prompts.
Surprisingly, the precision of our color terminology seems to have a considerable impact on the final output. Simply using “turquoise” instead of “blue” yields a more nuanced and refined color, indicating that AI requires precision when it comes to specific visual elements.
We also found that being assertive with our language often produces better results. Using direct instructions like "Create an image of…" is generally more effective than softer language like "I would like to see…" This reinforces that a more clear and concise approach helps guide the AI's interpretation in a way that achieves our vision.
The field of AI-driven image generation is in constant evolution. While the core technology continues to develop at an impressive pace, understanding these advanced prompt engineering techniques is critical for users looking to leverage the full potential of these powerful tools. As AI image generation becomes more ingrained in our creative workflows, understanding the subtleties of human-AI communication through language will undoubtedly become a valuable skill.
Experience error-free AI audio transcription that's faster and cheaper than human transcription and includes speaker recognition by default! (Get started for free)
More Posts from transcribethis.io: