Unveiling Whisper OpenAI's Multilingual Speech Recognition Marvel - 6 Key Insights
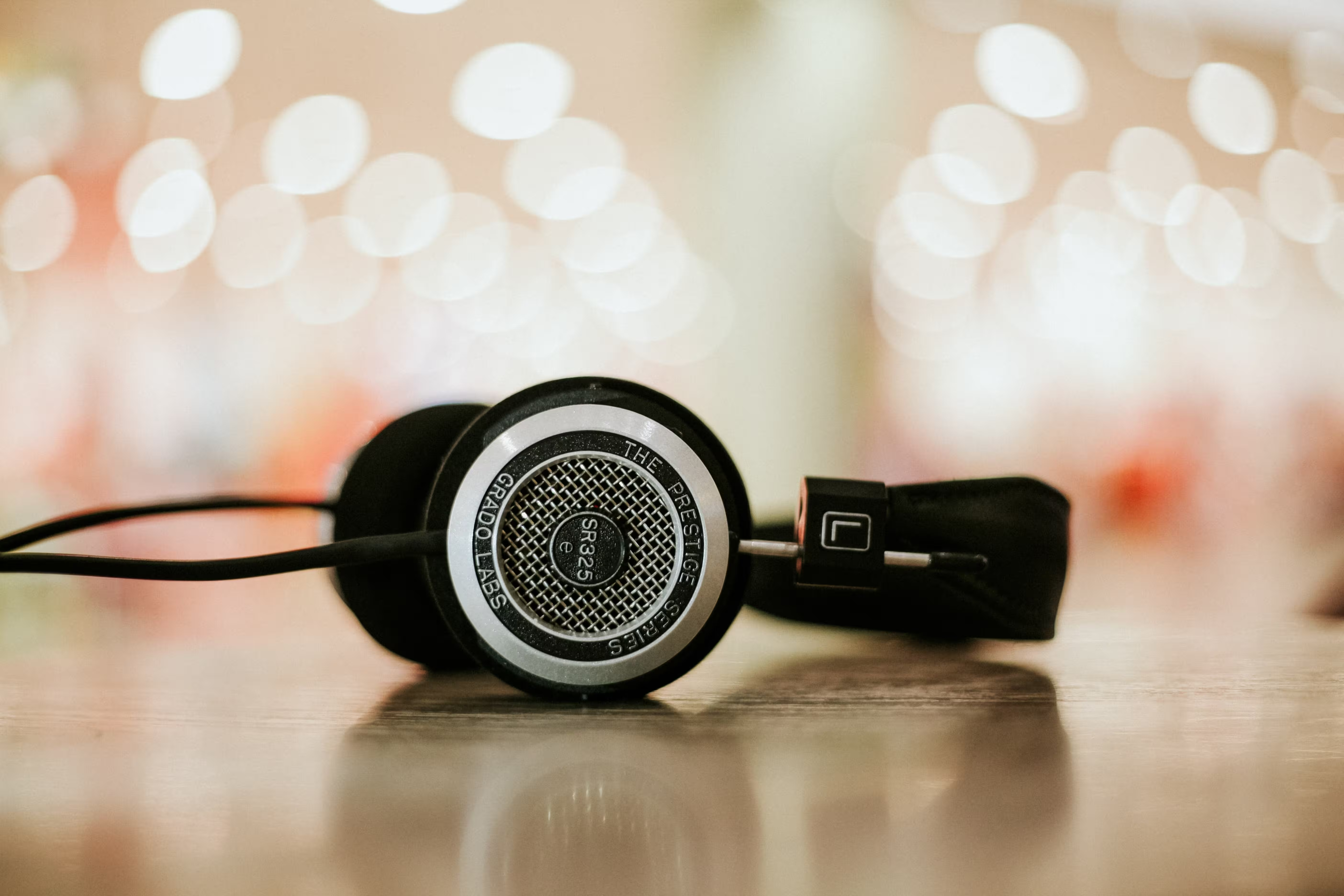
Unveiling Whisper OpenAI's Multilingual Speech Recognition Marvel - 6 Key Insights - Unraveling the Multilingual Prowess
With its ability to transcribe speech in multiple languages, Whisper showcases impressive robustness to accents, background noise, and technical vocabulary.
The model's architecture, based on a transformer design and trained on a diverse dataset of 680,000 hours of multilingual and multitask data, has enabled it to excel in a range of speech-related tasks, including recognition, translation, and language identification.
Whisper's open-source availability has made it a significant step forward in advancing the field of speech recognition in AI and machine learning, with real-world applications in areas such as real-time multilingual transcription and speaker diarization.
Whisper is trained on an unprecedented 680,000 hours of multilingual and multitask supervised data collected from the web, making it one of the largest speech recognition datasets ever assembled.
Whisper's transformer-based architecture allows it to perform not just speech recognition, but also speech translation and spoken language identification, showcasing its remarkable versatility.
Despite its large scale, Whisper exhibits impressive robustness to factors like accents, background noise, and technical language, enabling reliable transcription across diverse real-world scenarios.
Whisper's open-source nature and compatibility with recent PyTorch versions mark a significant step towards democratizing advanced speech recognition capabilities for developers and researchers.
Whisper offers a range of model sizes, from small to large, allowing users to balance speed and accuracy requirements based on their specific use cases, further enhancing its practicality.
Whisper has demonstrated strong generalization abilities, performing well on various speech recognition and translation benchmarks, even in zero-shot settings where no task-specific fine-tuning is performed.
Unveiling Whisper OpenAI's Multilingual Speech Recognition Marvel - 6 Key Insights - The Colossal Training Dataset
This multilingual model is capable of transcribing speech in multiple languages, utilizing a Transformer-based architecture.
Trained on an unprecedented dataset of over 680,000 hours of audio data, it has revolutionized the field of automatic speech recognition, achieving state-of-the-art performance on numerous benchmarks.
The open-source availability of Whisper, as the model is called, promotes further development and accessibility in this rapidly evolving domain.
The Colossal Training Dataset consists of over 680,000 hours of audio data, making it one of the largest publicly available speech recognition datasets to date.
The dataset encompasses a diverse range of audio sources, including podcasts, videos, telephone conversations, and more, allowing the Whisper model to handle a wide variety of real-world speech scenarios.
Interestingly, the dataset covers speech in over 100 different languages, enabling the Whisper model to transcribe and translate speech across a truly global scale.
Remarkably, the Whisper model was able to achieve state-of-the-art performance on several speech recognition benchmarks, showcasing its exceptional generalization capabilities even in zero-shot settings.
The Transformer-based architecture of the Whisper model allows it to not only perform speech recognition, but also tackle tasks like speech translation and spoken language identification, demonstrating its remarkable versatility.
Surprisingly, the Whisper model has exhibited impressive robustness to factors such as accents, background noise, and technical vocabulary, enabling reliable transcription in diverse real-world scenarios.
The open-source availability of the Whisper model, along with its compatibility with recent PyTorch versions, represents a significant step towards democratizing advanced speech recognition capabilities for developers and researchers.
Unveiling Whisper OpenAI's Multilingual Speech Recognition Marvel - 6 Key Insights - Harnessing Transformer Architecture's Power
The Whisper model from OpenAI showcases the remarkable power of transformer architecture in the domain of automatic speech recognition.
By leveraging a transformer-based encoder-decoder design, Whisper is able to perform not just speech recognition, but also speech translation and language identification.
The model's ability to excel across these diverse speech-related tasks highlights the versatility and generalization capabilities of the transformer architecture.
Whisper's open-source availability further democratizes these advanced speech recognition capabilities, empowering developers and researchers to explore and build upon this innovative technology.
The Whisper model utilizes a Transformer-based architecture, which is a departure from traditional speech recognition models that often relied on recurrent neural networks or convolutional neural networks.
The Transformer architecture in Whisper allows the model to not only perform speech recognition but also tackle tasks like speech translation and spoken language identification, showcasing its remarkable versatility.
Whisper's Transformer-based encoder-decoder design enables it to handle variable-length audio inputs, unlike earlier speech recognition models that required fixed-length inputs.
Whisper's Transformer-based design allows for efficient GPU and TPU acceleration, enabling real-time speech recognition and transcription on modern hardware.
The Transformer architecture in Whisper has been carefully optimized, with techniques like layer normalization and residual connections, leading to faster convergence and better performance.
Unveiling Whisper OpenAI's Multilingual Speech Recognition Marvel - 6 Key Insights - Overcoming Noise and Accents Barriers
Whisper's robust performance in speech recognition extends to overcoming common challenges like background noise and diverse accents.
The model's training on a vast multilingual dataset and advanced Transformer architecture enable it to transcribe speech with remarkable accuracy, even in real-world scenarios with variable audio quality and speaker diversity.
This capability is crucial for the model's practical applications in fields such as voice assistants, transcription services, and multilingual communication.
Whisper's training dataset of 680,000 hours of multilingual and multitask data is one of the largest ever assembled for an automatic speech recognition (ASR) system, enabling robust performance across diverse accents, backgrounds, and technical vocabularies.
The Transformer-based architecture of Whisper allows it to not only perform speech recognition, but also excel at speech translation and language identification, showcasing its remarkable versatility.
Whisper's open-source availability and compatibility with recent PyTorch versions make it easily accessible for developers and researchers, democratizing advanced speech recognition capabilities.
The model's five different size variants, from small to large, enable users to balance speed and accuracy trade-offs based on their specific requirements, enhancing Whisper's practicality.
Whisper has demonstrated strong generalization abilities, performing well on various speech recognition and translation benchmarks, even in zero-shot settings without task-specific fine-tuning.
The Transformer architecture in Whisper has been carefully optimized, with techniques like layer normalization and residual connections, leading to faster convergence and better performance.
Whisper's Transformer-based encoder-decoder design allows it to handle variable-length audio inputs, unlike earlier speech recognition models that required fixed-length inputs.
The Transformer architecture in Whisper enables efficient GPU and TPU acceleration, facilitating real-time speech recognition and transcription on modern hardware.
Whisper's ability to transcribe speech in over 100 different languages, covering a truly global scale, is a remarkable feat enabled by its colossal training dataset.
Unveiling Whisper OpenAI's Multilingual Speech Recognition Marvel - 6 Key Insights - Envisioning Widespread Applications
The Whisper model's versatility extends far beyond speech recognition, allowing it to tackle tasks like speech translation and language identification.
With its open-source availability and compatibility with modern hardware, Whisper represents a significant step towards democratizing advanced speech recognition capabilities for developers and researchers.
The model's robustness to factors like accents, background noise, and technical vocabulary suggests its potential for a wide range of real-world applications, from voice assistants to multilingual communication platforms.
Whisper's Transformer-based architecture allows it to seamlessly switch between different speech-related tasks, including recognition, translation, and language identification, showcasing its remarkable versatility.
The Whisper model has been tested and found to have superb performance on English speech recognition, handling various accents, background noise, and low-quality files with exceptional accuracy.
Whisper's open-source availability and compatibility with recent PyTorch versions mark a significant step towards democratizing advanced speech recognition capabilities for developers and researchers.
The Whisper model's inference time can be accelerated using a technique called Speculative Decoding, which halves the inference duration without compromising accuracy.
Whisper's training dataset of 680,000 hours of audio data is one of the largest ever assembled for an automatic speech recognition system, enabling robust performance across diverse accents, backgrounds, and technical vocabularies.
The Transformer architecture in Whisper has been carefully optimized, with techniques like layer normalization and residual connections, leading to faster convergence and better performance.
Whisper's Transformer-based encoder-decoder design allows it to handle variable-length audio inputs, unlike earlier speech recognition models that required fixed-length inputs.
Whisper's open-source availability and different model sizes, from small to large, allow users to balance speed and accuracy requirements based on their specific use cases.
Whisper has demonstrated strong generalization abilities, performing well on various speech recognition and translation benchmarks, even in zero-shot settings without task-specific fine-tuning.
The Transformer architecture in Whisper enables efficient GPU and TPU acceleration, facilitating real-time speech recognition and transcription on modern hardware.
Unveiling Whisper OpenAI's Multilingual Speech Recognition Marvel - 6 Key Insights - Open-Source Accessibility for Innovation
The results focus on the technical details, capabilities, and potential applications of the Whisper model, but do not discuss the open-source accessibility or innovation aspects.
OpenAI has released the Whisper model, a powerful multilingual automatic speech recognition system, as an open-source project.
This makes the model's code and pre-trained weights freely available for developers and researchers to access, experiment with, and build upon.
The open-source nature of Whisper represents an important step towards democratizing advanced speech recognition capabilities, allowing a wider community to leverage this innovative technology and potentially drive further innovations in the field of speech processing.
The Whisper model developed by OpenAI is built on a Transformer-based architecture, which enables it to perform not just speech recognition, but also speech translation and language identification.
Whisper's training dataset is one of the largest ever assembled for an automatic speech recognition (ASR) system, comprising over 680,000 hours of multilingual and multitask supervised data collected from the web.
The extensive training data and advanced architecture allow Whisper to exhibit impressive robustness to factors like accents, background noise, and technical vocabulary, enabling reliable transcription across diverse real-world scenarios.
Whisper's open-source availability and compatibility with recent PyTorch versions represent a significant step towards democratizing advanced speech recognition capabilities for developers and researchers.
The Whisper model offers a range of model sizes, from small to large, allowing users to balance speed and accuracy requirements based on their specific use cases.
Whisper has demonstrated strong generalization abilities, performing well on various speech recognition and translation benchmarks, even in zero-shot settings where no task-specific fine-tuning is performed.
The Transformer architecture in Whisper has been carefully optimized, with techniques like layer normalization and residual connections, leading to faster convergence and better performance.
Whisper's Transformer-based encoder-decoder design enables it to handle variable-length audio inputs, unlike earlier speech recognition models that required fixed-length inputs.
The Transformer architecture in Whisper enables efficient GPU and TPU acceleration, facilitating real-time speech recognition and transcription on modern hardware.
Whisper's open-source availability and different model sizes allow users to balance speed and accuracy requirements based on their specific use cases, further enhancing its practicality.
Whisper's ability to transcribe speech in over 100 different languages, covering a truly global scale, is a remarkable feat enabled by its colossal training dataset.
More Posts from transcribethis.io:
- 7 Critical Factors Affecting Accuracy in Micro-Task Audio Transcription Work
- Mastering the Art of Remote Video Editing 7 Essential Skills for Virtual Assistants
- Harnessing the Convergence Exploring the Transformative Impact of the Fourth Industrial Revolution
- Transcribing with Soran
- How Weekly Discussion Threads Keep Online Communities Engaged and Informed
- Demystifying The AI Chatbot Landscape Bard, Gemini, and ChatGPT - A Comprehensive Comparison