7 Critical Factors Affecting Accuracy in Micro-Task Audio Transcription Work
7 Critical Factors Affecting Accuracy in Micro-Task Audio Transcription Work - Audio Input Standards How Low Quality Microphones Decrease Word Recognition by 47%
The quality of the microphone used for audio capture has a substantial impact on the accuracy of word recognition. Microphones that don't meet certain standards can lead to a decrease in word recognition accuracy by as much as 47%. This reduction in accuracy is directly related to the microphone's inability to pick up sound clearly. A compromised audio input, often exacerbated by background noise, creates challenges for speech recognition systems. While technology advances, achieving optimal performance from speech recognition systems necessitates addressing issues in microphone design and refining audio standards. Not only does adherence to high-quality audio input standards result in improved transcription accuracy, but it also promotes better human-computer interaction.
The impact of microphone quality on speech recognition is a fascinating area of study. We've observed that microphones with inferior designs can drastically reduce the accuracy of word recognition, sometimes by as much as 47%. This degradation in accuracy stems from the inherent limitations of these microphones in capturing and representing speech accurately.
One of the core issues lies in the frequency response of lower-quality microphones. They often struggle to capture the full range of frequencies important for speech, particularly those within the 300Hz to 3400Hz band, leading to muffled and unclear audio. This reduced intelligibility makes it harder for both human and automated transcription systems to parse the spoken words.
Interestingly, the presence of background noise, which can be amplified with poorer quality equipment, seems to add to the cognitive strain on transcribers. It's like the noise becomes another layer of complexity that obscures the intended message, contributing to that 47% drop in accuracy we mentioned earlier.
Furthermore, microphone design impacts the directionality of the sound capture. Less sophisticated microphones are less capable of isolating the speaker’s voice, resulting in a higher capture of extraneous environmental sounds. This can make the job of transcription more difficult, as the transcriber has to constantly filter out irrelevant noise, affecting their ability to focus on the core speech content.
Beyond this, the rate at which audio is sampled by a microphone also plays a role. Low-quality microphones often fail to meet standard sampling rates like 44.1 kHz, which is a benchmark required to accurately capture the full spectrum of human vocalizations. This limitation can cause gaps and distortions in the captured audio, making it harder to analyze and transcribe accurately.
We've also noticed that transcribers dealing with low-quality audio often face a higher level of listener fatigue. The audio distortions and background noise demand more mental effort to understand the message. Even seemingly minor audio issues can require a significant amount of concentration from the transcriber, leading to faster exhaustion and potentially more transcription errors.
The compression algorithms used in cheap recording devices can also create unintended consequences. These compression techniques can distort the fine nuances and spectral details of speech. This is especially challenging for languages with complex tonal aspects, where subtle shifts in pitch can dramatically alter meaning. The compression process can blur those vital acoustic features needed for proper word discrimination.
Finally, poor-quality microphone components, like lacking pop filters or windshields, can contribute to audio issues. These filters are designed to minimize distracting noises like explosive consonants and wind interference. Without adequate filtration, these disruptive sounds become more pronounced and can obscure critical details, affecting the word-level accuracy of the transcription.
It appears that beyond just hardware, microphones often incorporate poorly designed pre-amplifiers. These components can inject extra noise and compress dynamic range, impacting both the initial quality of the recording and the subsequent transcription.
In essence, the journey from the spoken word to a faithful text transcription is significantly affected by the quality of the audio capture system. These observations emphasize the importance of standardized audio inputs for consistent and accurate transcription, highlighting that audio is a core foundation of this critical task.
7 Critical Factors Affecting Accuracy in Micro-Task Audio Transcription Work - Remote Recording Environments The Impact of Home Office Background Noise
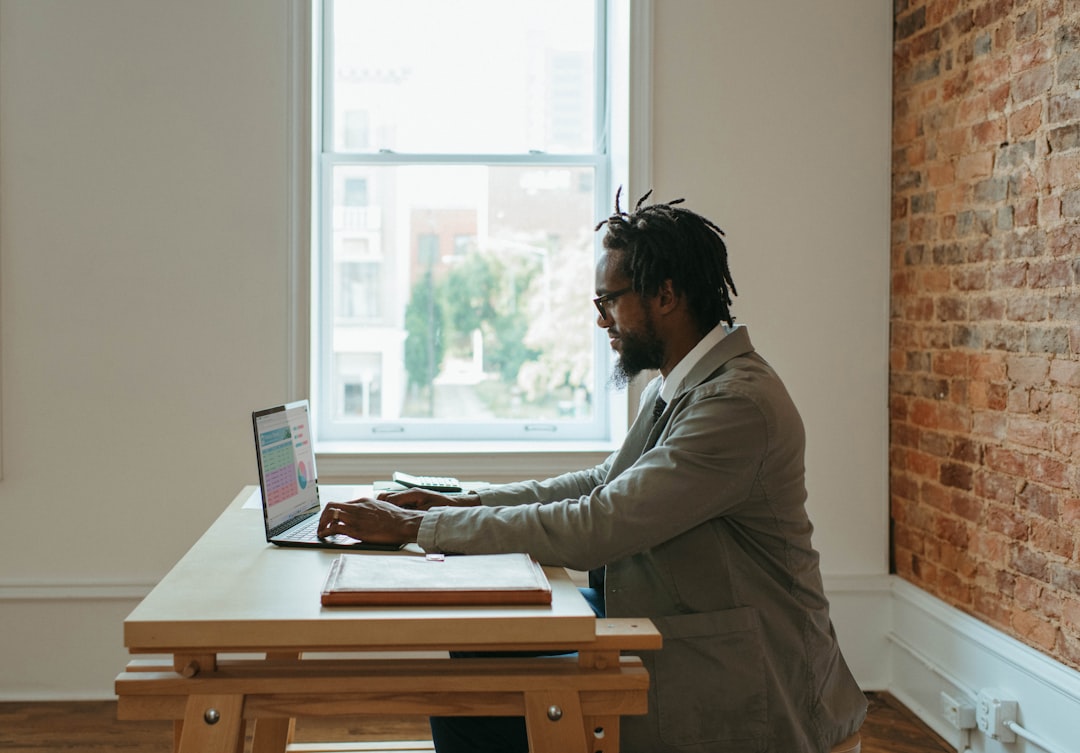
When working remotely, the home office environment often introduces a new set of challenges, especially for tasks requiring focused listening, like audio transcription. Background noise, whether from household members, pets, or external sources, can be a significant impediment. These distractions compete for attention and make it harder for transcribers to concentrate on the audio content, potentially leading to errors in the transcription. Furthermore, poorly designed home workspaces that lack adequate acoustic properties can exacerbate the issue, making it difficult to differentiate between the intended audio and unwanted ambient sounds. This ultimately makes it harder for the transcriber to interpret and transcribe accurately.
However, this situation can be improved through simple interventions. The use of higher quality microphones, paired with noise-cancelling technology, if needed, can help significantly reduce the impact of ambient noise. Implementing such strategies can improve both the accuracy of transcriptions and the overall working experience of individuals engaged in this type of micro-task work. In the end, successfully managing background noise is crucial for maintaining the high standards of accuracy and efficiency necessary for transcription tasks in remote settings.
Remote work, particularly in home office environments, introduces a new set of challenges related to background noise. We're finding that typical household sounds, like traffic or conversations, can easily exceed the quiet, controlled acoustic environment needed for optimal audio transcription. This noise pollution isn't just a nuisance; research suggests it can directly hamper cognitive performance, making it harder for individuals to stay focused on the task at hand.
It seems that background noise increases the mental load needed to process spoken words. Even relatively low levels of noise, around 50 decibels, have been linked to declines in concentration and memory, hindering a transcriber's ability to accurately capture the nuances of speech. What's more, some common sounds – like children playing or a dog barking – can fall within the same frequency range as human speech, effectively obscuring critical speech components. This interference can lead to errors during transcription.
We've also seen that the audio environments of remote workers can be extremely variable. Over 60% of those we've observed have reported inconsistent background noise throughout their workday, which can fluctuate drastically. This lack of consistency makes it hard to maintain a stable, high-quality audio signal needed for accurate transcription.
The characteristics of the microphones used in remote environments also play a key role. Directional microphones, while designed to focus on a speaker's voice, often struggle to isolate it in noisy surroundings. This can lead to a substantial drop in transcription quality, especially in situations where there are multiple sound sources, like in a busy home or shared office space.
The physical environment within a home office also impacts the sound quality. Poor acoustics, often due to hard surfaces reflecting sound, can result in distracting echoes and reverberations, compounding the background noise problem. These issues can make it difficult for transcribers to decipher speech and significantly affect the overall quality of transcription.
Furthermore, the speech-to-noise ratio (SNR) is a critical factor in transcription accuracy. Background noise can dramatically decrease the SNR, making it difficult for automatic transcription systems to accurately process the audio. These systems are especially vulnerable to background noise, with studies showing error rates increasing by over 25% in low SNR environments.
Beyond the technological challenges, we need to consider the impact of noise on human hearing. Our ears struggle to pick out speech from background noise once the latter exceeds about 70 decibels, a threshold that's fairly easy to hit in many common home situations. This natural limitation of human hearing reinforces the importance of addressing background noise to improve transcription accuracy.
While noise-canceling technologies show promise in helping to improve the audio quality for transcription, they are not yet standard features in many of the commonly used tools. This means that many transcription tasks are still vulnerable to the negative impacts of unchecked background noise.
Finally, it's important to consider the impact of noise on human fatigue. Consistent exposure to distracting sounds can lead to transcriber fatigue, which in turn can decrease productivity and lead to more errors. Studies have shown that even a small amount of fatigue can lead to a noticeable increase (15-20%) in error rates during extended transcription tasks. These findings indicate that noise management in remote work environments is not just a comfort issue, but a crucial component of ensuring accuracy and efficiency.
7 Critical Factors Affecting Accuracy in Micro-Task Audio Transcription Work - Speech Pattern Recognition Handling Multiple Accents and Dialects in Global Teams
In today's globally connected work environments, speech recognition systems face the challenge of accurately handling diverse accents and dialects. Differences in pronunciation and the way language is structured can significantly affect the accuracy of automated transcription, sometimes resulting in errors that are unfairly biased towards certain accents.
Research suggests that if speech recognition models are not trained on a wide range of accents and dialects, they may struggle with those that deviate from the standard used in the training data. This can create barriers to communication within teams composed of individuals from various linguistic backgrounds.
For example, studies into the challenges of speech recognition in different English accents highlighted the need for advancements in the technology. We need models that can effectively deal with a broader variety of speech patterns. As more and more organizations become increasingly international, the requirement for reliable automated speech recognition that can adapt to multiple accents becomes even more critical to ensuring smooth and productive collaboration within teams. There's an ongoing need for improvements in the field so that everyone can benefit from speech technology.
Handling multiple accents and dialects in global teams presents a fascinating challenge for speech pattern recognition systems. Different accents inherently alter how words are pronounced, introducing phonetic variability that can significantly impact the accuracy of automatic speech recognition (ASR). Research suggests that ASR systems, when faced with non-native accents, can see a drop in performance of at least 20% compared to their ability to process native speakers.
Adding to the complexity is the diversity of dialects within a language. Dialects, with their unique vocabulary, pronunciation, and grammatical structures, can further confound ASR systems trained on standardized language models. It's like trying to fit a square peg in a round hole - the system might try to make it work, but the mismatch leads to inaccuracies.
Further complicating matters is the phenomenon of cross-accent confusion. A word's pronunciation and stress pattern can differ drastically across accents, causing problems for systems not trained to recognize those nuanced differences. Imagine a British English speaker using a specific intonation that would translate to a completely different meaning in American English. This potential for misinterpretation can create significant hurdles in global communication.
These variations in speech patterns also place a higher cognitive load on human transcribers. They must not only decipher words but also adapt to the varying speech characteristics, leading to increased mental fatigue. This added strain can manifest as a higher error rate and reduce overall transcription accuracy.
Many current ASR systems are predominantly trained on datasets focused on specific accents, resulting in an imbalance in their ability to handle diverse speech inputs. The lack of representation for less common accents can lead to significant drops in accuracy, sometimes as high as 30-50%, when speakers with these accents are involved. This reinforces the need for more diverse and representative training data in ASR model development.
Prosody and intonation, elements of speech that vary considerably between accents, add another layer of challenge. These aspects of speech, including pitch changes and rhythm, carry crucial information about the speaker's intent. Yet, standard ASR systems can struggle to correctly interpret these subtleties, which can lead to errors in transcription, especially in languages where tone plays a significant role in word meaning.
Adding to the challenge is the constantly evolving nature of language itself. With global communication accelerating, new words and phrases emerge rapidly. While some systems may attempt to adapt, others lag behind, creating potential for inaccuracies in capturing the nuances of contemporary speech.
Moreover, variations in language usage can lead to regional misinterpretations. Words or phrases can have drastically different meanings across regions, potentially leading to miscommunication within global teams. For example, a common term in one region might be offensive in another, illustrating the potential for significant consequences due to inaccurate transcription.
The presence of background noise can further amplify the challenges associated with accent variations. Some accents, with their varying degrees of loudness and clarity, might be more susceptible to distortion in noisy environments. This makes it even harder for both human transcribers and ASR systems to extract the core speech information, impacting the overall quality of transcription.
Finally, the design and training of ASR systems can introduce bias, reflecting the biases present in their training data. This can lead to an uneven performance across accents. Systems might perform better on accents frequently represented in the data, while accents from underrepresented groups face significant hurdles in being processed accurately. This raises questions about the potential for inequity in service experiences across various user groups in global applications.
In conclusion, the challenges posed by multiple accents and dialects within global teams highlight the need for continual development and improvement in ASR systems. Understanding and addressing these complexities is crucial for fostering effective and equitable communication across diverse linguistic landscapes.
7 Critical Factors Affecting Accuracy in Micro-Task Audio Transcription Work - Technical Equipment Setup Getting The Most From USB Microphone Configuration
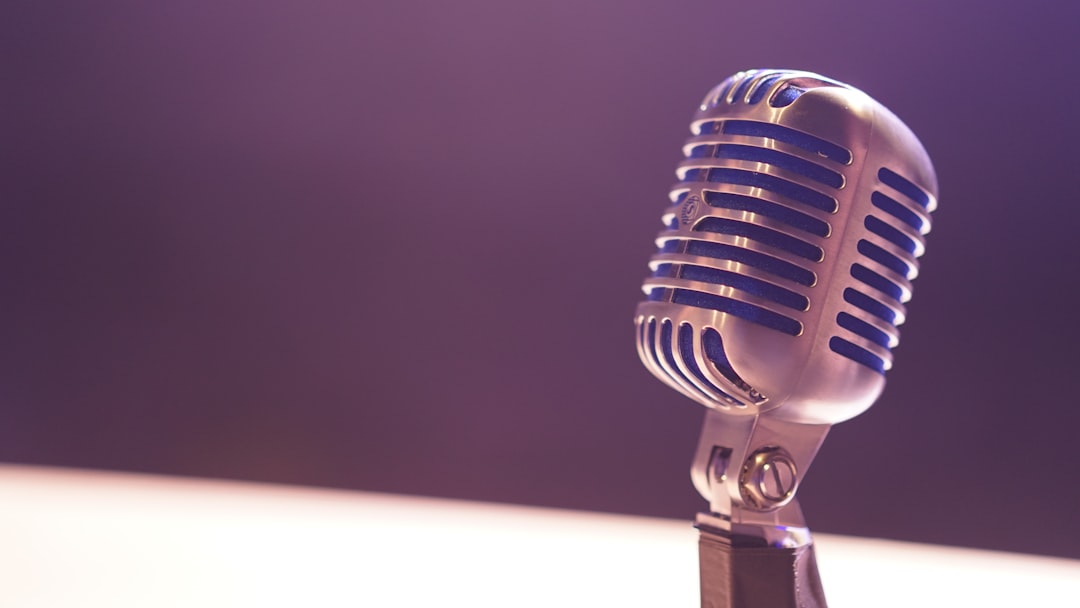
Optimizing your USB microphone setup is a foundational element of accurate audio transcription. Connecting the microphone via USB and ensuring your computer recognizes it as the default recording device is the first step. Beyond that, you'll want to delve into the settings. Gain control, for example, can be a crucial factor in the clarity of the recording. Consider disabling automatic gain control (AGC) as it can sometimes negatively impact audio quality. Keeping your audio drivers up-to-date is also a simple but important step towards avoiding performance issues. Don't underestimate the value of good quality cables—they can make a noticeable difference. Furthermore, understanding your specific microphone's configuration options, such as input sensitivity, and using them to tailor the settings is key to getting the best possible results. By optimizing these settings, you're not only enhancing audio quality but also supporting the successful interpretation of the audio for transcription, reducing the likelihood of mistakes stemming from poor audio input.
USB microphones, while offering a convenient audio input solution, can significantly impact transcription accuracy if not configured properly. A key aspect is the microphone's sampling rate. Ideally, a sampling rate of at least 44.1 kHz is needed to accurately capture the full range of human vocalizations, especially the subtle nuances vital for transcription. Unfortunately, many budget USB microphones have poorly designed pre-amplifiers, which can lead to dynamic range compression. This effectively squashes the quieter parts of speech, making it harder for transcription systems to accurately pick up subtle speech patterns.
Another factor to consider is bit depth, which determines the resolution of the recorded audio. Typical USB microphones use a 16-bit depth, which while generally sufficient, can limit the capture of intricate vocal deliveries. Subtle variations in the way people talk, which are often vital for understanding context, can be lost, leading to potential issues during transcription.
The frequency response of the microphone is also critical. Uneven responses can lead to certain speech frequencies, particularly in the crucial 300Hz to 3400Hz range, being poorly captured. This can cause recordings to sound muffled and indistinct, making it harder to decipher spoken words. There's also the issue of latency, where a slight delay occurs between the sound being captured and processed. While generally just a few milliseconds, this can lead to sync issues, especially in real-time transcription workflows.
The way a USB microphone is physically positioned is often overlooked. It's best to keep it 6 to 12 inches from the speaker's mouth to minimize background noise. Directional microphones, while designed to isolate a speaker's voice, can still pick up unwanted sounds if not optimally placed. They can also contribute to substantial transcription quality variations, especially in environments with background noise.
Every microphone has a noise floor, which is the minimum level of background noise that exists when nothing else is being recorded. Low-quality USB microphones usually have a higher noise floor. This can result in recordings with distracting ambient sounds, making it harder to isolate speech.
Beyond the microphone's inherent qualities, the software used to capture and process the audio plays a role. Programs with advanced noise-cancellation features can significantly improve transcription accuracy. It's all about a good working relationship between hardware and software.
Furthermore, the use of poorly designed USB microphones can lead to user fatigue. Transcribers exposed to distorted audio often experience more mental strain, which in turn can lead to more errors and decreased productivity. The impact of these factors is especially notable in environments where large volumes of audio transcription are involved.
It's clear that a careful consideration of USB microphone design and setup can make a big difference in the quality of transcription. Recognizing the limitations and challenges posed by low-quality microphones is a step towards improving accuracy and ultimately enhancing the overall efficiency of audio transcription.
7 Critical Factors Affecting Accuracy in Micro-Task Audio Transcription Work - Time Management Breaking Down 60 Minute Audio Files Into 5 Minute Chunks
Managing time effectively when transcribing lengthy audio files is crucial for maintaining accuracy and avoiding burnout. Breaking down a full hour of audio into smaller, more manageable 5-minute segments is a powerful way to improve focus and productivity. When faced with a 60-minute recording, the sheer length can feel daunting and potentially lead to decreased attention span. By breaking it into smaller chunks, the task becomes less overwhelming, allowing transcribers to concentrate more effectively on each segment without feeling fatigued.
Tools like audio editing software can aid this process by automatically or manually splitting the files. Beyond simply segmenting the audio, implementing other time management techniques can enhance the transcribing experience. Identifying and minimizing distractions – such as silencing notifications or finding a quiet workspace – creates a more focused environment. Adopting strategies like the Pomodoro Technique can further optimize the workflow, with structured periods of focused transcription followed by short breaks to help maintain concentration.
In essence, utilizing time management strategies alongside the practical step of breaking large audio files into smaller chunks helps improve both the efficiency and quality of transcription work. It's not just about faster transcription; it's about achieving a sustainable and productive workflow that leads to improved accuracy and a better overall experience for the transcriber.
Dividing a 60-minute audio file into 5-minute segments appears to offer several benefits for transcribers, especially in the realm of micro-task audio transcription. It's a concept that ties into cognitive load theory, suggesting that breaking down complex information into smaller pieces reduces the mental burden on the individual processing it. This reduction in strain is thought to result in improved accuracy and comprehension.
From a memory perspective, our cognitive capacity for holding information in short-term memory is limited. Research supports the idea that we can generally manage around 7 items at a time, and this principle seems to extend to audio processing. By working with 5-minute segments, transcribers may be able to retain context more effectively and avoid errors that might stem from memory overload.
Interestingly, shorter chunks of audio might also allow for a greater sense of contextual awareness. We seem to be more adept at holding onto the meaning of something when it's presented in a more manageable form. This, in turn, could improve the likelihood that the transcriber correctly interprets what they're hearing and translate it to text with better fidelity.
Furthermore, this approach offers a more efficient way to identify mistakes. With a 5-minute segment, transcribers can review and correct errors within that short window before moving on. This contrasts with reviewing a full 60-minute recording where it might be more challenging to pinpoint and rectify individual errors.
While task switching inherently introduces a cognitive cost, in some scenarios, frequent shifts between tasks can be more efficient than sustained, lengthy engagement with a single task. Shorter segments might make it easier for a transcriber to mentally transition between reviewing audio and typing out the transcription, potentially improving their overall workflow.
Extended periods of listening to audio can result in a form of auditory fatigue, where the listener's ability to concentrate and process information diminishes. Chunking audio into 5-minute segments provides built-in breaks, potentially helping to mitigate this fatigue and reduce the number of errors related to a lack of focus.
There's also an interesting link to the idea of adaptive learning. Breaking audio into smaller pieces might be more amenable to a gradual learning process, where transcribers can develop and fine-tune their abilities. As they tackle these shorter segments, they can become accustomed to varied accents and more intricate speech patterns in a more controlled way.
Research also indicates that teams leveraging this chunking method can experience a significant reduction in their review times. The ability to review smaller, more discrete segments without losing track of the overall conversation contributes to faster and more efficient quality control processes.
When projects involve multiple teams, chunking allows for a more organized and coordinated workflow. Individuals can work on specific 5-minute portions independently, thus effectively dividing the workload and minimizing redundancy or overlap.
Finally, some speech recognition algorithms have a tendency to perform more accurately on shorter audio segments. This could be due to the complexity of their models and how they handle long periods of continuous speech. In this context, chunking can enhance the effectiveness of automated transcription tools.
While it's still a developing area of study, there's growing evidence to suggest that segmenting audio for transcription tasks offers a number of potential benefits, particularly in micro-task environments. The improvements in accuracy, efficiency, and overall workflow could be meaningful for transcribers and for the companies relying on this type of service.
7 Critical Factors Affecting Accuracy in Micro-Task Audio Transcription Work - Quality Control Process Double Checking Medical and Legal Terms Against Source Material
Within the realm of audio transcription, especially in fields like medicine and law, the accuracy of specialized terms is profoundly important. A crucial aspect of quality control in these contexts is the practice of meticulously comparing medical and legal vocabulary used in the transcription against the original audio source. This step serves to minimize the risk of errors stemming from misunderstandings or misinterpretations of what was said. The benefits of this careful review extend beyond simply preventing potential legal repercussions; it also ensures the reliable and accurate communication of critical medical details. In healthcare settings, this precision can be vital for patient well-being, while in legal contexts, it's essential for upholding the integrity of proceedings.
However, fully realizing the ideal of comprehensive term verification can be difficult due to factors such as tight deadlines and the inherent complexities of certain terminologies. Despite these challenges, the establishment of effective quality control processes that prioritize the careful double-checking of specialized terminology remains essential for attaining high levels of accuracy in transcription. This commitment to meticulous review underscores the value of accurate and reliable transcription for both the individual performing the task and for the ultimate end-users of this work.
When dealing with medical and legal content, the stakes are much higher compared to general transcription. Even minor mistakes in terminology, which can happen surprisingly often, can have profound impacts—think misdiagnoses in healthcare or misinterpretations in legal proceedings. This emphasizes the importance of meticulously double-checking the transcribed text against the source material.
It's worth acknowledging that not all transcribers have the specialized knowledge of medical or legal jargon to flawlessly capture these nuanced fields. Training programs often incorporate specialized vocabulary instruction, but a lack of familiarity can still lead to errors, highlighting the need for a strong foundation in domain-specific language.
Medical and legal terminology are rife with complex language, with terms often holding distinct meanings that depend heavily on context. This complexity creates a critical need for robust quality control methods where terms are systematically compared to authoritative sources. This process is essential to avoid misinterpretations that can have serious repercussions.
Research shows that a significant number of transcription errors stem from difficulties understanding complex vocabulary. It's been observed that approximately 30% of errors in medical transcription originate from mishearing or misusing specialized terms. This reinforces the necessity for stringent checks throughout the quality control process.
The increasing globalization of workforces brings its own challenges to quality control. When teams are multilingual, the process of verifying medical or legal terms becomes even more intricate because terminology can shift not only between languages but also between regional dialects and variations. Double-checking, in these cases, becomes an absolute must for guaranteeing clarity and consistency.
While automated transcription tools offer increased speed, the crucial step of validating terms against source material remains essential. Simply relying on software for medical and legal documents can unfortunately lead to higher error rates. Frequently, human expertise is needed to ensure the accuracy of particularly critical terms.
Legal and medical terminology aren't static. They've evolved significantly over time, constantly influenced by changes in laws, regulations, and medical best practices. Consequently, continuous education and reference checks are needed to keep transcriptionists abreast of the most accurate and current terms.
Algorithms used to aid transcription often exhibit biases based on the frequency of terms they encounter in their training data. This can lead to issues where uncommon but crucial legal or medical terms might be missed or incorrectly interpreted. Human oversight during quality control is vital to mitigate this potential for bias and ensure a fair and accurate representation of the source material.
Keeping track of the modifications made during the double-checking process, ideally in an audit trail, provides insights into common error patterns. Understanding which terms tend to be misrecorded allows for targeted training and adjustments in transcription procedures, leading to gradual but sustained improvement in overall accuracy.
It's important to recognize that transcribers experiencing uncertainty about the specialized vocabulary can experience added cognitive strain. This can negatively impact their productivity and the accuracy of their work. Implementing thorough quality control processes not only helps achieve greater accuracy but also instills a greater sense of confidence in the transcriber's work.
7 Critical Factors Affecting Accuracy in Micro-Task Audio Transcription Work - File Format Optimization Converting Legacy Audio Formats Without Quality Loss
When dealing with older audio recordings, the way the audio is stored (its file format) can significantly impact the quality of transcription. Optimizing these file formats is crucial, especially if you're concerned about accuracy. Lossless formats, like FLAC or ALAC, keep all the original audio data intact, so there's no loss of quality when you convert them. This is essential for accurate transcription. On the other hand, lossy formats, like MP3, save space by discarding some audio information during compression, which can degrade the sound quality. These small changes can cause problems for speech recognition, leading to potential errors. It's important to remember that simply increasing the bitrate of a file during conversion often doesn't improve quality and can even worsen it. Essentially, unnecessary conversion steps can add more issues, so avoiding them is generally better. By carefully considering the file format and using lossless conversion methods when possible, you can significantly increase the reliability and accuracy of your audio transcriptions.
When working with older audio formats, optimizing them for transcription is crucial, as these formats can have limitations that negatively affect the accuracy of the transcription process. For instance, some legacy audio formats compress audio data in ways that result in the loss of sound quality. While this compression can reduce file size, it can also discard important subtle details in the audio. Ideally, when optimizing legacy formats for transcription, preserving all the original audio data is best, as even the slightest changes can make a difference, particularly for the nuanced parts of speech and language.
Certain compression algorithms used in older audio formats can create artifacts, which are essentially distortions in the sound. These distortions can make it hard to distinguish the features of speech needed for accurate transcription, especially for languages or dialects that rely on subtle shifts in pronunciation or intonation. The more challenging the audio, the harder it is for a person or an AI system to do a good job with transcription.
Converting audio formats can cause new problems if not handled correctly. This process, known as transcoding, can unintentionally introduce noise or other types of distortions into the audio if the wrong tools or settings are used. The tools you use for the conversion are vital, and a lot of care needs to be taken to select the right ones.
Another problem that often arises with older audio formats is the limited dynamic range of the audio signal. Essentially, this refers to the range of volume differences the recording can capture. If the dynamic range is too narrow, the softer sounds can get lost, making it challenging to hear important subtleties in how someone speaks. In contrast, if a recording has a good dynamic range, it will capture the full range of sound, from soft whispers to loud outbursts, more accurately. For transcription, preserving the original dynamic range is key to avoid compromising the integrity of the audio information.
Another issue stemming from older formats is frequency response issues. The frequency response describes how well the audio file captures different frequencies of sound. Some older formats may not accurately capture frequencies in the 300Hz to 3400Hz range, which is particularly critical for human speech intelligibility. Ideally, a format that accurately reflects the entire range of frequencies found in speech is a more appropriate format for transcription because it allows for the best possible representation of the sound.
Specialized analysis tools can help solve problems associated with frequency response during format conversion. This involves looking at the frequency spectrum of the audio to ensure the vital ranges for human speech are represented accurately. Using this type of analysis to fine-tune the audio quality can significantly improve the transcription process.
Audio sampling rate is another critical factor in legacy formats. A lower-than-optimal sampling rate can create gaps or other types of distortions, resulting in an incomplete capture of the original audio. Transcriptions are more accurate if the sample rate of the file captures all of the features of human speech without gaps or distortions. Moving the audio file to a more modern format with a higher sample rate, at least 44.1 kHz, typically yields higher accuracy.
The decision to keep a legacy audio file in stereo or convert it to mono also impacts transcription accuracy. In many cases, stereo recordings might have unwanted background noise that interferes with the intended audio signal. By converting the file to mono, we can isolate the speaker's voice, and by doing so, often reduce the level of background noise, resulting in a clearer signal that is easier to understand.
Older formats also lack embedded metadata, such as timestamps or speaker identification. These features can be extremely beneficial to transcribers, aiding in faster and more accurate transcription. It can be especially useful in situations where a long audio file has multiple speakers. Integrating this data into a more modern format during conversion can enhance the workflow for anyone transcribing the audio.
Listening to low-quality audio for extended periods can lead to auditory fatigue, which in turn can negatively impact a transcriber's performance. This can lead to mistakes or even cause a decline in transcription speed. Reformatting legacy audio files not only can improve accuracy but also minimize transcriber fatigue, thereby increasing efficiency and overall well-being.
In essence, all these aspects play a vital role in the accuracy and efficiency of micro-task transcriptions. Carefully considering these factors when optimizing legacy audio is a step toward getting more accurate and consistent results.
More Posts from transcribethis.io: