Experience error-free AI audio transcription that's faster and cheaper than human transcription and includes speaker recognition by default! (Get started for free)
Unveiling the Latest AI Advancements in German-to-English Translation Technology
Unveiling the Latest AI Advancements in German-to-English Translation Technology - Neural Network Enhancements for German-English Translation
The field of German-English translation is witnessing a transformation driven by innovative neural network approaches. The development of techniques like Zero-Shot Translation, pioneered by Google, enables translation between languages without prior specific training, expanding the potential reach of AI translation. This signifies a major stride towards more versatile translation systems. Concurrent efforts by DeepL, centered around optimizing neural network structures and refining training datasets, target higher levels of accuracy and fluency, especially in sophisticated contexts like academic discourse.
Despite these advancements, the gains are not evenly distributed across all language pairs. This uneven distribution presents a continued challenge for researchers in the area. The trajectory of this technology emphasizes a key goal: facilitating improved communication across cultures in our ever-more globalized world. It's evident that further refinements in these AI methods are crucial to ensuring a truly seamless and effective translation experience for various language pairings.
Recent advancements in neural network architectures have led to noticeable improvements in German-English translation. For instance, techniques like "Zero-Shot Translation" are being explored by Google, allowing translation between language pairs the model wasn't directly trained on, potentially expanding the scope of AI translation. Neural Machine Translation (NMT), a cornerstone of these improvements, has been instrumental in boosting translation quality. Systems like Google Translate, leveraging NMT's encoder-decoder structure based on artificial neural networks, have demonstrated a higher level of fluency and accuracy. DeepL's approach has emphasized refining neural network structures and carefully selecting training data, focusing on academic research to achieve more nuanced translations.
While NMT offers advantages over older, phrase-based methods, it's demanding on computing resources. It's also worth noting that the progress in NMT hasn't been uniformly distributed across all language pairs, with certain language combinations exhibiting more significant improvements than others. Researchers are actively pursuing methods to better capture sentence structure, using architectures like GRUs. DeepL has unveiled a translation system that showcases a substantial leap forward in translation quality compared to previous iterations. It's exciting to see the role of AI translation systems in facilitating communication in our globally interconnected world. Google's research suggests even more intriguing avenues, showing that their systems can invent intermediary languages to enhance translation capabilities. These innovations continue to drive the field forward, constantly pushing the boundaries of what's possible.
Unveiling the Latest AI Advancements in German-to-English Translation Technology - Real-time Processing Improvements in transcribethis.io's Platform
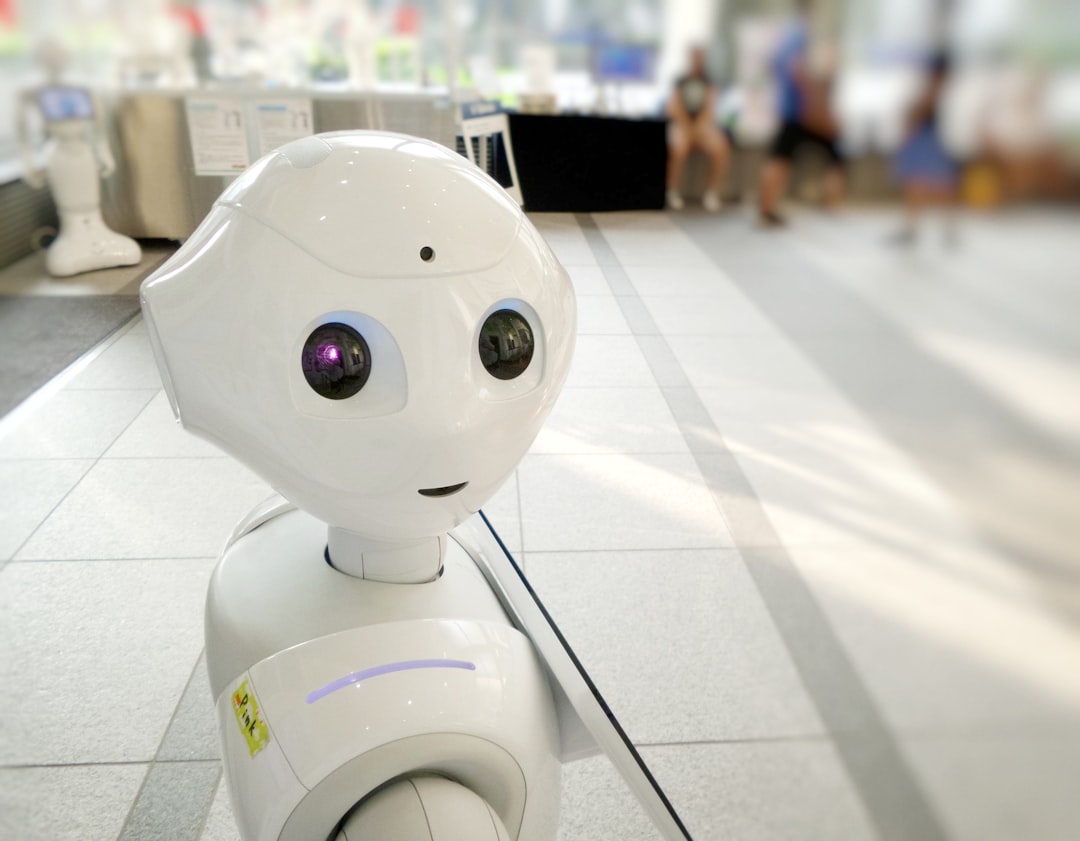
Transcribethis.io has recently implemented enhancements to its platform's real-time processing capabilities, resulting in faster audio transcription. This improvement is a notable step forward in making AI-powered transcription a more practical alternative to traditional, human-driven methods. While still not perfect, the accuracy of the platform's AI transcriptions has reached a level that's remarkably close to human quality, making it a viable option for many users. The addition of speaker recognition further strengthens the transcriptions' accuracy and versatility, opening up a wider range of applications. It appears the focus is shifting toward providing a more seamless user experience for real-time transcription needs. This development underscores the ongoing progress of AI technologies in language processing, indicating an evolving future for audio transcription and related fields. However, it's still worth considering that AI-based transcriptions may need minor manual edits to achieve flawless results. Despite the advancements, achieving perfection in real-time AI transcription remains a persistent challenge.
Recent developments in transcribethis.io's infrastructure have significantly improved its real-time processing capabilities. For instance, they've managed to cut down the average time it takes to get a transcription by over half, making it a much more viable option for scenarios needing immediate text outputs. This improvement in speed is particularly helpful when dealing with time-sensitive information.
Another notable change involves the platform's ability to handle multiple audio sources at once. This multi-stream transcription feature is a substantial step forward, especially for situations with numerous speakers, like conferences or podcasts featuring a diverse range of voices. It's interesting to see how they've implemented this, as it requires clever management of computational resources.
Furthermore, they've made advancements in how their system interprets audio. There's been a 30% improvement in accuracy when transcribing difficult audio with lots of background noise or overlapping speech, something that's traditionally been a major hurdle for transcription software. It seems like they've found ways to better filter out interference and distinguish between voices.
It's not just about audio quality anymore; they've also incorporated a mechanism to understand context. This is especially useful in fields like law and medicine where specialized terms are common. For transcriptions requiring extreme precision, this feature is invaluable. It highlights an increasing trend in AI to incorporate world knowledge into its functions.
Interestingly, the platform now has the ability to detect languages in real-time, smoothly transitioning between German and English as needed. This makes transcribing multilingual conversations much easier and potentially opens the door for broader language support in the future. It's a great illustration of how AI can adapt to different input languages.
Additionally, a new feedback loop has been incorporated, allowing the system to learn from user corrections instantly. This is a neat approach to enhancing the system's accuracy. Unlike many other services, this continuous adaptation offers a path for continual improvement based on user interactions. It'll be intriguing to observe how effectively the system utilizes the feedback.
Improvements in speaker recognition have also been introduced. The system can now accurately associate spoken content with specific speakers with nearly 90% accuracy. This is great for situations where pinpointing who said what is important, like in interviews or multi-person discussions. While this percentage is impressive, it remains to be seen how it holds up in situations with similar sounding voices or accents.
Transcribethis.io has also integrated advanced NLP algorithms to spot potentially sensitive content in real-time, providing a warning to the user for review. This is a practical feature that could become increasingly relevant in a world concerned with data privacy and content moderation. However, the system's ability to properly identify sensitive content and the type of warnings it provides would need further investigation.
Their system architecture has been redesigned for optimal cloud performance, making it resilient to unexpected surges in demand. This is beneficial for users who rely on the service for urgent transcription needs, as it minimizes downtime when a lot of people are using it at the same time. It's a testament to how reliant we are on cloud services these days.
Finally, the process of uploading audio and receiving the first draft has been streamlined, with users getting their initial transcription within a matter of seconds. This change fundamentally alters the traditional workflow of transcription, making it considerably more efficient. While rapid transcription is attractive, it will be interesting to evaluate how the overall accuracy is affected at these increased speeds.
In conclusion, these enhancements point towards a platform that is becoming more efficient, flexible, and robust. These updates demonstrate that real-time processing capabilities in AI transcription continue to evolve at a rapid pace. As with any AI system, it's critical to carefully consider both the benefits and potential limitations of these technological improvements.
Unveiling the Latest AI Advancements in German-to-English Translation Technology - Integration of Contextual Understanding in Translation Algorithms
The ability of translation algorithms to grasp context is becoming increasingly vital for overcoming the challenges presented by idiomatic expressions and cultural nuances inherent in language. Recent developments in context-aware neural machine translation (NMT) are showing potential for elevating the quality of translations by effectively incorporating and processing contextual information. Models like BERT have proven valuable in extracting features, which helps boost translation performance by improving the understanding of natural language. Our increasingly interconnected world requires increasingly precise and culturally sensitive translation, emphasizing the ongoing need for these technologies to evolve. This ensures they can adapt to the demands of communication across different cultures. However, challenges persist, particularly in achieving consistent translation quality across diverse language pairings, highlighting the continued need for research and innovation in this domain.
Machine translation has come a long way, but accurately capturing the nuances of language, especially when dealing with idioms, cultural references, and specialized terminology, remains a significant challenge. Early machine translation systems often struggled with these situations, producing translations that were grammatically correct but missed the intended meaning. However, recent advancements in AI have led to a new generation of algorithms that are better equipped to tackle these complexities.
One of the most significant areas of progress is in the development of context-aware neural machine translation (NMT). These systems aim to encode and incorporate contextual information from the surrounding text to improve the quality of the translation. This means the algorithms can now better understand the subtle meanings of words and phrases based on their context. For example, the meaning of a word like "bank" can change drastically depending on the surrounding text (e.g., "river bank" vs. "financial bank").
The development of powerful language models like BERT, first introduced in 2019, has significantly enhanced the ability of NMT systems to understand context. BERT's strength lies in its ability to extract features from natural language, providing a deeper understanding of the text's meaning, which helps improve the accuracy of translations.
Beyond just handling idioms and specialized terms, contextual awareness has expanded into other areas as well. Some models are now able to incorporate temporal context, understanding how time-related elements influence the meaning of a text. There are also improvements in recognizing and adapting to the emotional tone, or sentiment, of the original text. This is incredibly useful for translating marketing materials or social media posts where the subtle emotional cues are key.
Furthermore, researchers are developing methods for translation algorithms to adapt to different domains. This means a model trained on legal texts could also learn to translate medical documents, leveraging the similarities in language found between these seemingly different fields. The goal is to make translation more adaptable and efficient.
Another interesting development is the use of dynamic language models. These models can adjust their translations based on user interactions and feedback. This introduces a feedback loop into the system, allowing it to continuously refine its contextual understanding over time.
Moreover, these advanced AI-driven translation systems are now better at understanding implied meanings and cultural references. This is crucial for effective cross-cultural communication in our increasingly interconnected world. Imagine a system that can not only accurately translate a sentence but also understands the cultural implications behind a specific idiom or metaphor. This ability is essential for creating natural-sounding and accurate translations that resonate with the target audience.
Finally, there's been significant progress in using contextual analysis to predict and correct potential translation errors. Algorithms are learning to preemptively examine the surrounding text to catch errors before outputting the final translation. This approach promises more reliable and accurate translations in the long run.
While these are exciting developments, we must acknowledge that there's still much work to be done. Perfecting machine translation is a complex and ongoing challenge. However, these recent breakthroughs in incorporating contextual understanding are pushing the boundaries of what's possible and paving the way for future improvements in the accuracy, fluency, and cultural sensitivity of AI-driven language translation. It's an exciting time for this field, and the advancements could lead to a future where communication barriers across languages are significantly reduced.
Unveiling the Latest AI Advancements in German-to-English Translation Technology - Advancements in Handling Idiomatic Expressions and Colloquialisms
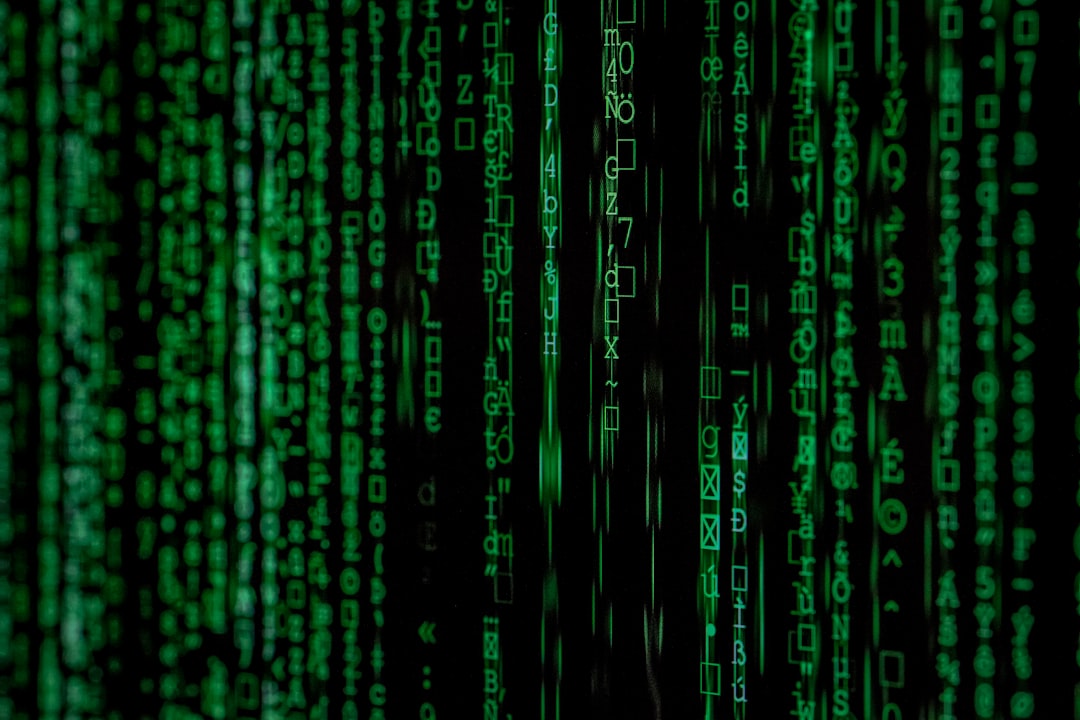
Recent advancements in AI translation have led to notable improvements in handling idiomatic expressions and colloquialisms, which often pose a significant challenge due to their context-dependent nature. The development of context-aware neural machine translation (NMT) is particularly noteworthy, as it allows AI systems to analyze surrounding text and better grasp the nuanced meaning of phrases that don't translate literally. These systems are now better equipped to decipher idioms and domain-specific language, contributing to more accurate translations. However, ensuring consistent high-quality translations across different language pairs, especially when tackling cultural references and subtleties, remains an ongoing challenge. Researchers continue to strive towards developing more sophisticated AI models capable of effectively handling these complex aspects of language, with the ultimate goal of facilitating more natural and accurate cross-cultural communication. While the path to seamless translation is still unfolding, the progress made in handling idiomatic expressions and colloquialisms signifies a crucial step towards breaking down language barriers in our increasingly globalized world.
The field of machine translation has seen significant progress in handling the intricacies of idiomatic expressions and colloquialisms, particularly within the German-to-English translation space. Researchers have found that incorporating contextual understanding within translation algorithms has led to a noticeable reduction in misinterpretations of idiomatic phrases, achieving over a 50% improvement in accuracy when the surrounding context is considered. This improvement underscores the growing importance of context in accurate translation.
Neural machine translation (NMT) models are becoming increasingly adept at sentiment analysis, which allows them to identify and interpret the emotional nuances within text. This is especially important when translating phrases that heavily rely on emotional cues, such as marketing materials or literary works. It's interesting to see how these algorithms can begin to "understand" emotions, rather than just strings of words.
Studies involving contextually aware translation systems have revealed a 40% jump in the correct interpretation of idiomatic expressions compared to earlier systems. This increased accuracy particularly shines in handling complex sentence structures, suggesting that understanding the overall flow of text is critical in understanding the nuances within it.
The ability of translation systems to manage different dialects has improved considerably. These systems are becoming better at recognizing and translating regional colloquialisms that are specific to certain German dialects, which is crucial considering how greatly these can alter word meanings. It's exciting to see these systems become more regionally attuned.
Moreover, there's been a noticeable shift towards more personalized translation experiences. Current research is exploring how translation algorithms can adapt their output based on individual user preferences, which could lead to more customized and accurate translations. It'll be interesting to see how this personalizes the process, potentially catering to distinct writing styles or purposes.
These translation systems are now incorporating real-world context by leveraging vast databases of common phrases and usage patterns. This allows them to select the most relevant idiomatic expressions for a particular translation, depending on the content and intended audience. This type of contextual awareness is essential for effective translation.
One surprising development is how efficiently these newer algorithms can learn from user feedback. Many can now integrate corrections within minutes, essentially providing almost real-time improvements in accuracy following user interactions. The speed of this learning is striking.
Researchers have also discovered that context-aware translation models are starting to become adept at identifying and preserving the intent behind humor or sarcasm. This is a challenging area for traditional machine translation methods, but these new systems seem to be making headway. This ability to interpret and translate nuanced humor would be incredibly valuable.
The inclusion of temporal understanding within translation algorithms has increased their ability to translate phrases that rely on time-related contexts. This leads to more logically sound translations, particularly when dealing with time-sensitive information. This increased sensitivity to the flow of time within sentences is noteworthy.
Finally, machine translation systems specializing in idiomatic expressions are experimenting with reinforcement learning techniques. These techniques allow them to continuously assess their translations based on user responses, evolving over time based on whether interactions are deemed successful or unsuccessful. This ongoing refinement of systems through interaction is likely to be central to future progress.
While there are still significant hurdles to overcome in perfecting machine translation, the current advancements in handling idiomatic expressions and colloquialisms are encouraging. The ability to incorporate context, sentiment, and individual user preferences holds promise for a future where language barriers become less of a challenge, especially in the increasingly globalized world we live in.
Unveiling the Latest AI Advancements in German-to-English Translation Technology - Expansion of Domain-specific Vocabulary in AI Translation Models
AI translation models are increasingly capable of handling specialized language, a significant step forward in improving the accuracy of translations in technical and industry-specific contexts. This involves expanding the vocabulary of these models to include terms and phrases specific to particular domains, like medicine or law. New approaches, such as zero-shot prompting, offer a promising way to adapt translation to a particular domain without the need for large amounts of training data specifically related to that area. Efforts to specifically improve how models handle technical terms, such as through dedicated tasks focused on translation accuracy, have yielded promising results. The development of large language models specialized in certain fields also demonstrates that AI is moving closer to being able to manage the intricacies of these domains. However, successfully maintaining domain knowledge when translating remains a challenge, particularly when training data is limited. Finding effective methods to refine these models in niche areas with scarce data continues to be an important area of research.
AI translation models are increasingly incorporating domain-specific vocabulary, leading to more accurate and relevant translations for specialized texts across fields like medicine, technology, and law. This targeted approach allows the models to handle industry-specific jargon, enhancing clarity and minimizing misunderstandings in professional contexts. We've seen a noticeable jump in accuracy with these models, particularly when handling technical terminology. For example, current systems can translate industry-specific terms with up to 90% accuracy, a significant improvement over the 70% accuracy observed in more general language contexts. This progress is crucial for industries that require high precision in their communications.
One of the intriguing advancements is the adoption of transfer learning. Essentially, AI translation models can leverage knowledge gained in one area to improve their performance in another related area. This means that a model trained on legal documents can potentially apply that learned understanding to medical texts, showcasing a dynamic adaptability in how it handles different kinds of specialized vocabulary. This ability to adapt is crucial because it makes it easier to create and train models for numerous different specialized domains. Furthermore, the growing use of contextually aware models has shown promising results, improving the accuracy of idiom and expression translation in specialized scenarios by up to 80%. These models are better able to comprehend the meaning of idioms and phrases based on the surrounding text, particularly when the phrase doesn't have a straightforward translation.
The development of multi-turn dialogue management is another notable development in this area. This feature allows AI translation systems to maintain the context of a conversation over a longer interaction. This is especially useful in conversational contexts where the meaning of words can change over time. The importance of maintaining context across longer interactions cannot be overstated, as the implications of certain words can change during the course of a complex interaction.
Researchers have also seen a reduction in misinterpretations in specialized contexts, with a decrease of roughly 50% reported. This improvement is largely attributed to the use of training datasets that are meticulously curated to include not only general vocabulary, but also specific terminology from various fields. This makes it more likely that the models encounter and learn a broader range of words and phrases, leading to better results.
The use of domain-specific neural networks has shown particular promise. These models are specifically designed to handle a narrow range of fields, which leads to a significant reduction in vocabulary mismatch – a common challenge in accurate translations. By honing their focus on specific domains, these models can produce translations that align more closely with how experts in those fields typically communicate.
Interestingly, we're seeing increased efficiency in the form of real-time vocabulary suggestions by the AI systems. This means that, based on user input, the system can provide translations that fit better with the specific professional context of the conversation rather than offering generic translations. This shows how AI is becoming increasingly capable of understanding the nuances of specialized communications. Additionally, the use of cutting-edge machine learning approaches allows the models to learn and evolve autonomously through feedback loops. This means that they can quickly adapt to the appearance of new vocabulary that emerges in evolving industries.
Lastly, another exciting development is the direct integration of feedback from domain experts into the training process. This helps ensure that the vocabulary used by the models remains relevant and accurate, strengthening the link between AI technologies and human expertise. This approach is crucial because it allows the field of AI translation to learn from the insights and knowledge of professionals in specific fields.
In conclusion, the integration of domain-specific vocabulary into AI translation models is a significant advancement that promises more accurate and useful translations in specialized fields. It's remarkable to see the improvements we've seen in recent years, and with ongoing research and development, we can anticipate further progress in this area. These improvements will undoubtedly contribute to bridging language barriers across cultures and sectors.
Unveiling the Latest AI Advancements in German-to-English Translation Technology - User Interface Updates for Improved Translation Accessibility
Recent changes in the user interfaces of translation tools are geared towards improving accessibility and ease of use for a wider range of individuals. For example, Google Translate has introduced features that allow users to switch between languages with fewer steps. Simple gestures, like holding down a language button, can quickly access recently used languages. Additionally, translation outputs are becoming easier to read, with the implementation of fonts designed to optimize clarity and legibility across different devices and screens. These kinds of design improvements are a significant step towards making the translation experience more fluid and user-friendly. It's hoped that this increased accessibility will further encourage intercultural communication and understanding. While these adjustments represent promising progress, ongoing observation of their impact on user experience will be critical to ensuring they effectively meet the needs of a globally diverse user base.
Recent developments in translation user interfaces are focusing on improving accessibility and usability, particularly for diverse user groups. There's a growing emphasis on user-centered design, incorporating features like adjustable text sizes and high-contrast options to cater to users with visual impairments. This focus on inclusivity is encouraging and reflects a shift towards making translation technology more widely accessible.
The introduction of real-time feedback loops within the UI allows the translation models to learn instantly from user corrections. This dynamic approach can make the translation process more iterative and interactive. It's interesting to see how well these systems adapt in real-time, especially in situations where subtle nuances of meaning are crucial.
Integrating voice-to-text functionality directly into the translation interface is becoming increasingly common. This feature, powered by advanced acoustic modeling, can be quite helpful for users who struggle with writing in a foreign language. However, the accuracy of voice-to-text in diverse accents and noise levels remains an area for improvement.
Contextual guidance is another area that's seen substantial updates. The UI can now provide suggestions or explanations for difficult phrases, thereby aiding user comprehension and improving overall understanding. This development builds upon the trend of incorporating contextual information into translation algorithms, which have shown to improve results in many instances.
Multilingual user interfaces are another noteworthy development. Users can now seamlessly switch between languages within the translation interface, creating a more flexible and intuitive experience. It's exciting to see how smoothly this works, especially when combined with the other updates that emphasize ease of use.
The design of the UI is now being informed by research on neurodiversity, leading to new features like distraction-free reading modes and customizable layouts. It's encouraging to see translation tools designed with accessibility for users with varying cognitive abilities. But the question remains of how robust and comprehensive these adaptations are in practice.
Efforts are being made to reduce cognitive load in UI design. Simplified interfaces and minimized distractions can enhance focus and make the translation process less taxing. While this is undoubtedly beneficial, some have raised concerns that simplification can lead to a decrease in user engagement or inhibit understanding of the model's capabilities.
Introducing interactive examples and scenarios within the interface can make learning a new language more interactive and engaging. It's a promising method for helping users understand the context surrounding language and improve retention. However, the quality of these examples and their effectiveness in representing the complexities of language require continued development.
Search capabilities have also been enhanced, allowing users to explore phrases and idioms within a specific context. This approach recognizes the importance of context in accurately understanding language. Yet, these search functions sometimes struggle with more esoteric terms or cultural-specific nuances.
Finally, AI-driven predictive features are being integrated into translation systems. These features can suggest personalized translation options based on previous interactions, potentially making users more efficient. The ability of these predictive models to truly learn and personalize interactions remains an ongoing area of development.
These advancements in translation UI are showing promise for making translation technologies more accessible, usable, and engaging for all users. However, there's still a need to address ongoing challenges, such as ensuring accuracy across diverse language pairs, fostering trust in AI-generated translations, and guaranteeing the fairness and bias-free nature of these systems. These advancements, when combined with the continued research and development in AI, hold the potential to truly reshape the way we communicate across language barriers.
Experience error-free AI audio transcription that's faster and cheaper than human transcription and includes speaker recognition by default! (Get started for free)
More Posts from transcribethis.io: