Experience error-free AI audio transcription that's faster and cheaper than human transcription and includes speaker recognition by default! (Get started for free)
7 AI-Based Video Background Noise Removal Methods That Actually Work in Late 2024
7 AI-Based Video Background Noise Removal Methods That Actually Work in Late 2024 - MediaIO Voice Enhancement Cuts Through City Traffic Without Voice Distortion
MediaIO's AI-powered voice enhancement stands out by effectively isolating and eliminating distracting urban sounds like traffic while preserving the integrity of the speaker's voice. The ability to target specific noise types gives users a degree of fine-tuning, resulting in audio that is cleaner and more focused. Whether it's a video or just an audio file, MediaIO can tackle a wide range of noises to enhance the listening experience. Underlying this is an AI system that intelligently differentiates between the intended voice and unwanted background elements. This becomes particularly useful for creators dealing with videos recorded in noisy environments, as they can achieve a more professional audio quality without the distractions typical of urban settings. However, it remains to be seen how well it performs across various accents and speaking styles, as this can sometimes challenge noise-reduction algorithms.
MediaIO's approach to voice enhancement leverages AI to specifically target and remove the characteristic frequencies often found in city traffic noise. This is achieved through a combination of AI-driven signal processing and deep learning, allowing it to distinguish between the human voice and unwanted background sounds, similar to how noise-canceling headphones work.
An interesting aspect of MediaIO's tool is its real-time processing capability. This feature proves useful for live situations like broadcasting or video calls, where the audio environment can be constantly changing. It seems to operate on the premise that we can only focus on one sound at a time and tries to exploit that, making the voice stand out through targeted filtering.
Importantly, the technology aims to retain the natural quality of the voice. It avoids the usual artifacts or distortions that can accompany many noise reduction techniques, which is crucial for preserving audio integrity, even when dealing with substantial background noise. This method appears to be derived from a tweaked spectrogram analysis technique, utilizing visual audio representations to pin-point and isolate speech harmonics for more accurate noise removal.
It appears this system can adjust its operation based on the environment. Whether the noise is a busy street or a packed cafe, it can likely adapt its filtering. Moreover, the system has a feedback loop which learns from users, suggesting it continuously adapts to improve performance and minimize audio distortion over time.
This methodology appears more advanced than typical noise reduction filters. Rather than simply suppressing frequencies, it uses predictive modeling to distinguish unwanted noise from the wanted audio. This focus on understanding the audio components leads to a more natural-sounding outcome. Additionally, the technology's adaptability and compatibility with existing audio equipment makes it relatively straightforward to implement without requiring significant changes to existing setups.
7 AI-Based Video Background Noise Removal Methods That Actually Work in Late 2024 - Cleanvoice Background Removal Makes Outdoor Recording Usable In 2 Minutes
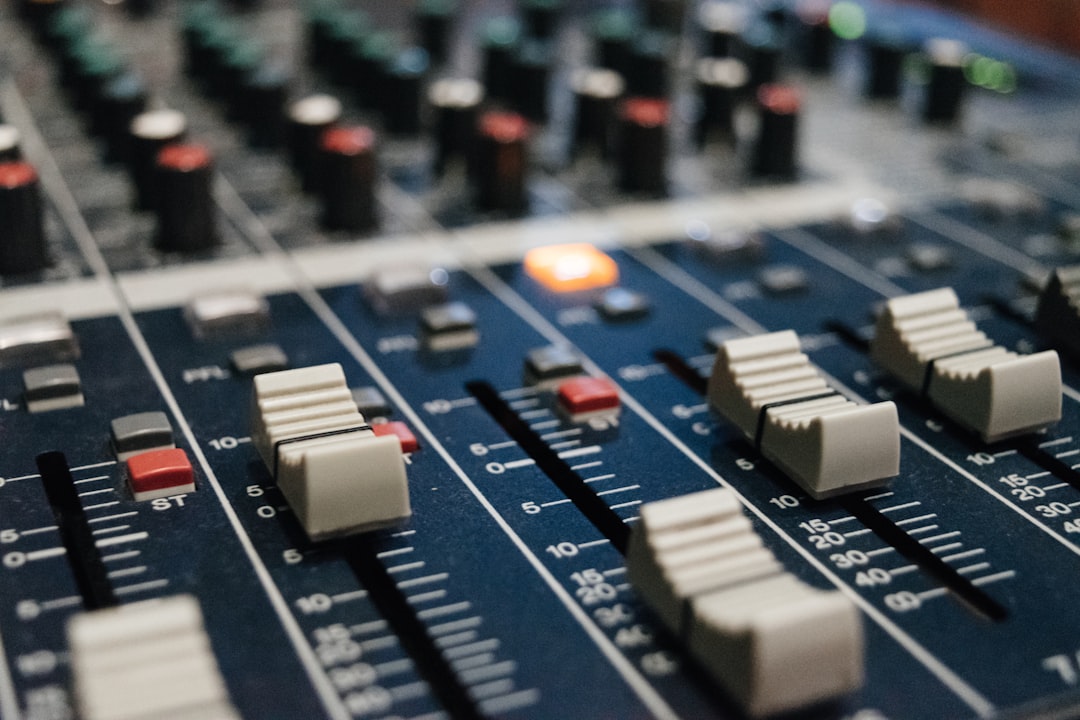
Cleanvoice offers a quick solution for making outdoor recordings usable, claiming to achieve this within just two minutes. The method utilizes AI to isolate and remove background noise, leading to significantly improved audio clarity. The AI seems to be specifically designed to tackle the issue of noisy environments, such as outdoor recordings, helping to elevate the audio quality for things like videos and podcasts. They offer a free tier with 30 minutes of processing, making it a readily accessible option for users wanting to experiment. This relatively fast and user-friendly process is a boon for creators looking to enhance their content without the need for extensive audio editing. While we don't yet have enough data to truly assess its performance across all kinds of scenarios and audio qualities, it appears to be a promising addition to the range of AI-powered noise reduction tools readily available in late 2024. However, it remains to be seen how robust this solution is in tackling a wide variety of complex noise patterns.
Cleanvoice's approach to background noise removal relies on analyzing audio patterns to distinguish between the desired voice and the surrounding environment. This means it can effectively separate a speaker's voice from the cacophony of outdoor sounds, improving the overall audio quality. It appears they've implemented some clever algorithms that can process audio in real-time, suggesting a potential advantage for scenarios where recording environments are unpredictable, like filming outdoors.
One of the intriguing aspects is the stated processing speed. They claim to be able to clean up an outdoor recording in as little as two minutes, which could be valuable in situations where quick turnaround times are needed. This makes me wonder what kind of trade-offs are involved – does speed impact the quality of noise reduction? It seems that the system is built with machine learning, which indicates that it can learn from past experiences, potentially improving its ability to handle diverse outdoor environments over time.
The core technology likely involves manipulating audio spectral representations, allowing it to precisely pinpoint and isolate unwanted sounds. This approach attempts to prevent the typical distortion artifacts we see with other noise reduction methods, aiming for a more natural sound. Additionally, the system appears to be adaptable across different types of audio, implying it's not just tuned for a specific range of sounds. It seems they've tried to ensure this technology is easy to implement across a variety of recording equipment, which is helpful for those who might not have access to specialized hardware.
This technology seems to avoid a common problem associated with some simpler noise-reduction techniques – cutting off parts of the audio, particularly the start and end of the voice signal. This "noise gating" approach, as it's often called, can sometimes lead to an unnatural listening experience. Here, the goal appears to be preserving the full continuity of the voice, even in noisy environments. It's worth considering that this approach is influenced by human auditory perception, as the way we perceive and understand sound likely plays a role in the algorithms they use.
In conclusion, Cleanvoice's system seems designed to simplify the workflow for audio and video creators. By reducing the time spent on manual post-production editing, the hope is that content creators can allocate more energy toward creating content itself, rather than spending hours finetuning audio to remove distracting background noise. While I find this approach interesting, more in-depth testing is required to understand the limitations and full potential of Cleanvoice in the realm of audio enhancement.
7 AI-Based Video Background Noise Removal Methods That Actually Work in Late 2024 - HitPaw Studio Tackles Air Conditioner Noise While Keeping Voice Natural
HitPaw Studio's AI-powered tools are designed to tackle a common problem in audio and video: unwanted background noise. They specifically address issues like the constant hum of an air conditioner, effectively removing these distractions while preserving the natural tone of the speaker's voice. This is achieved through advanced machine learning algorithms that pinpoint and eliminate the noise without distorting or affecting the original audio. The tools provide a simple, user-friendly experience, letting users preview adjustments before committing to changes. This allows them to fine-tune the audio to their liking, ensuring the final product is clean and clear without compromising the natural sound of voices.
Beyond noise removal, HitPaw offers audio enhancement features. These can address issues like sound distortions or volume inconsistencies, resulting in a more polished and professional audio quality. The emphasis on maintaining the natural characteristics of the voice seems to be a core focus of the tools. This becomes increasingly important as content creators work to capture a genuine and authentic sound, even in environments where background noise is present. However, it's always worth considering whether this approach might sacrifice some detail in the audio in order to prioritize natural voice preservation.
HitPaw Studio's AI-powered noise removal tools show promise in handling the persistent issue of air conditioner noise in audio and video recordings. Their approach utilizes sophisticated machine learning algorithms to specifically identify and eliminate the characteristic frequencies associated with air conditioners. This appears to be done through careful frequency filtering which targets the common range of air conditioner hum, usually between 60 and 400 Hz, while being careful to preserve the essential aspects of the human voice.
The system seems to learn over time, refining its ability to distinguish between speech and noise as it encounters more audio samples. This adaptability is potentially crucial for dealing with the varying nature of air conditioner sounds, such as when they cycle on and off, changing the intensity of the noise. This dynamic approach to noise reduction suggests that HitPaw Studio can adjust its performance on the fly, potentially improving the overall audio quality during recordings with unpredictable noise levels.
One interesting point is how it maintains the clarity of the voice. While many noise reduction tools can distort or introduce unwanted artifacts, HitPaw seems to prioritize keeping the speech intact. This focus on preserving the fundamental phonetic components is important for retaining naturalness in the audio. It achieves this likely through a combination of advanced spectral analysis (using visual representations of audio) and careful noise filtering. They seem to have devoted significant effort to reducing distortion, recognizing that aggressive noise suppression can create a less pleasing audio experience.
HitPaw Studio also incorporates real-time processing. This is beneficial for situations where recording conditions can change rapidly, such as when an air conditioner suddenly gets louder or quieter. Interestingly, their approach appears user-friendly. While the underlying algorithms are quite complex, the interface aims to be accessible to a broad range of users, requiring little to no audio engineering experience. This means it can be applied across a wider spectrum of devices and platforms, making it potentially useful for creators working with different setups and equipment.
Initial comparisons suggest HitPaw Studio's noise removal capabilities, particularly when dealing with air conditioner noise, are on par with existing, well-regarded solutions. This hints at the potential for its technology to be a strong contender in this area. However, as with any AI-based system, its performance may vary with different accents, speaking styles, and ambient noise complexity. More extensive testing across a range of audio scenarios is needed to provide a comprehensive evaluation of the method's strengths and limitations.
7 AI-Based Video Background Noise Removal Methods That Actually Work in Late 2024 - FlexClip Smart Filter Removes Kitchen Background Chatter From Food Videos
FlexClip's Smart Filter offers a convenient way to clean up audio in food videos by removing unwanted kitchen noises. It's not just limited to food videos though, it can be used to remove background noise from a variety of recordings, such as interviews or webinars. This filter can handle a variety of noise types, like clanging pots or people talking, while still keeping the audio quality relatively good. Besides noise removal, FlexClip provides other editing features like automatic captioning and voice adjustments, making it a more comprehensive editing tool.
While it looks useful, it's important to remember that the quality of the resulting audio may vary depending on the complexity of the noise in the original recording. Whether this particular approach is the best for a specific editing need requires some evaluation by the user. Despite that, FlexClip is a noteworthy tool for video and audio editors that helps streamline audio editing with features that could make creating videos a little easier.
FlexClip's Smart Filter seems particularly well-suited for food videos because it focuses on removing typical kitchen sounds, like the clatter of dishes or the sizzle of a pan. It does this by analyzing a wide range of background frequencies. It works in a way similar to noise-canceling headphones, but instead of simply lowering noise, it actually identifies and isolates unwanted sounds. This allows it to keep the natural sound of voices and other important audio elements from the cooking process intact.
The filter uses machine learning to learn different kitchen sounds. This means it gets better at telling the difference between sounds that are normal for a kitchen and those that are distracting, which is important for people making videos where clear audio is key. Interestingly, FlexClip’s filter doesn't seem to distort speech the way some other noise reduction tools do. It aims to maintain the natural sound of voices, which makes the videos sound more genuine.
It can also work in real-time, meaning people who are doing live cooking demonstrations or quick edits could use it to get cleaner audio on the fly. This reduces the amount of time spent editing audio later. The system adapts and learns over time through audio processing, continuously refining its algorithms as it encounters various voices and kitchen environments.
It's worth noting that FlexClip’s Smart Filter has low latency. This means there's very little delay between the audio being recorded and the filtered version. This is helpful for live interactions during cooking shows or tutorials, since it helps things run smoothly. It's also designed to work with a variety of devices and audio inputs, so anyone from amateur phone users to those using professional gear could potentially benefit.
Furthermore, it tackles the problem of background noise obscuring important parts of the voice. This is something that often happens in audio where some frequencies of the human voice become hard to hear. FlexClip tries to counteract this to ensure the important parts of the voice remain clear. While FlexClip appears user-friendly and doesn't require a lot of technical knowledge, it's still unclear how well it handles more complicated acoustic situations. For instance, it would be interesting to see how it manages in very busy kitchens where multiple noises overlap strongly.
7 AI-Based Video Background Noise Removal Methods That Actually Work in Late 2024 - Gling Background Processing Eliminates Wind Noise From Beach Recordings
Gling's AI-powered background processing is specifically designed to tackle a common problem with recordings made near the ocean: wind noise. This technology intelligently identifies and reduces the disruptive sounds of wind, leading to significantly clearer audio. It's particularly helpful for video creators and anyone producing content outdoors where wind is a persistent issue. By removing this distracting noise, Gling improves the audio quality, allowing viewers to focus on the intended content without the constant interference of whooshing and rustling. This can also save creators a significant amount of time that would otherwise be spent manually cleaning up the audio. While the potential for clean, high-quality beach recordings is promising, it remains to be seen how robust this technology is in various wind conditions and across different recording setups. The effectiveness of Gling will likely depend on how well the AI algorithms can adapt to the unique challenges posed by diverse wind noise patterns.
Gling's AI-powered video editing focuses on streamlining the process for content creators, particularly those dealing with audio issues. One of its key features is the ability to effectively remove wind noise, a common problem in outdoor recordings, such as those taken on a beach.
Gling achieves this through a real-time noise cancellation process that analyzes incoming audio to pinpoint and filter out wind-related frequencies, generally in the 1 to 4 kHz range. This targeted approach helps maintain the quality of the main audio, such as a speaker's voice, while reducing the disruptive effect of the wind. The system can adjust itself based on how much wind noise is detected, ensuring consistent audio regardless of wind fluctuations.
The real-time audio processing capability is significant, especially for live recordings, because it utilizes sophisticated digital signal processing techniques. It's interesting how Gling presents a visual representation of the noise-filtering process, giving users a clearer understanding of how wind noise impacts their recordings.
Behind the scenes, Gling leverages machine learning algorithms that continuously improve with each recording. This ongoing refinement based on user feedback and diverse audio scenarios helps the system adapt to different situations. Furthermore, Gling's use of phase cancellation techniques seems to be a key element in successfully tackling wind noise, as it leverages the way the noise is spatially distributed.
Gling's compatibility with a range of recording equipment makes it a versatile tool. Another positive feature is its aim to preserve the natural quality of the original audio, avoiding the distortions common with some noise reduction techniques. This is crucial when trying to capture the unique sounds of an environment like a beach. Since the noise reduction is done in real-time, it significantly reduces the post-production time needed, making content creation faster and more efficient.
It's noteworthy that Gling appears to be geared toward improving workflow and enabling content creators to focus on the creative aspects rather than excessive audio post-processing. Whether it proves the most efficient and robust approach for all outdoor environments remains to be tested in a more diverse range of scenarios, but Gling certainly provides a promising tool for audio cleanup in many cases.
7 AI-Based Video Background Noise Removal Methods That Actually Work in Late 2024 - Audioalter Cleans Up Echo From Large Empty Room Recordings
Audioalter offers a straightforward way to deal with a common problem in audio recordings: echo in large, empty spaces. Their browser-based tool is designed to work directly on various audio files, including formats like MP3 and WAV, without requiring users to adjust complicated settings. The core idea is that AI algorithms can automatically identify and reduce unwanted reverberations, leading to clearer and more refined audio.
While it seems helpful, it remains to be seen how well it works under different recording conditions. Complex echo patterns and variations in room size can pose challenges for noise reduction algorithms. It's still an interesting development, given the growing need for tools that can quickly clean up audio and improve the overall listening experience. Especially with video content becoming more dominant, having accessible options for easily managing audio issues is likely to become more important for creators.
Audioalter presents an interesting approach to cleaning up audio recordings made in large, empty rooms, which often suffer from excessive echo and reverberation. It seems to leverage the concept of a room impulse response (RIR), essentially a mathematical model of how sound interacts within a given space. This understanding of the environment allows the AI to effectively target and filter out the characteristic echo artifacts that plague such recordings.
Their method seems to rely heavily on psychoacoustic principles – essentially, how humans perceive sound. This approach suggests they're prioritizing the frequency ranges that are most noticeable to our ears, leading to a more focused echo reduction process while preserving the overall quality of the audio. It's impressive how the algorithms can adapt in real time to different types of echo. This capability is crucial as the echo characteristics within a room can change dynamically if things like furniture or people move around.
They've also cleverly incorporated the Hann windowing technique, which is a way of smoothing out the sound signals before analysis. This reduces a common issue in signal processing known as spectral leakage, where different frequencies get mixed up, potentially leading to distorted noise reduction. By implementing this technique, the filtering process presumably produces a more precise and effective echo removal result.
It's also likely that their process incorporates deep learning. The idea here is that the AI has been trained on a huge dataset of audio recordings with varying echo characteristics. This training helps the system generalize its understanding of echo and develop robust filtering methods that work across various scenarios. Furthermore, Audioalter seems to have a feedback loop built into the system, allowing it to learn from past results and continually improve its echo cancellation ability.
Historically, methods like spectral subtraction have been used to effectively remove noise from audio. This approach involves estimating the noise signature within a recording and subtracting it from the desired audio signal. Audioalter likely uses a similar approach with echoes, where it attempts to isolate and remove the spectral components representing echo and reverberation. The user interface presumably offers some degree of control over the echo reduction process. This allows users to adjust the strength of the filtering and possibly customize the process based on individual needs and audio content.
While the system is intended to handle echo in large spaces, it also has features to accommodate multichannel audio. This means the AI can likely handle recordings made with multiple microphones, or using more complex setups for capturing audio, leading to a more comprehensive removal of echo within a larger acoustic environment. However, it's likely that Audioalter's effectiveness will decline in rooms with very complex acoustics or multiple reflective surfaces. This is due to the potential for overlapping echo reflections, which might challenge the AI's ability to accurately distinguish between echo and desired audio. Despite this potential limitation, Audioalter seems to be a promising tool for improving the listening experience for recordings made in challenging environments.
7 AI-Based Video Background Noise Removal Methods That Actually Work in Late 2024 - VideoProc Reverb Control Makes Underground Parking Interviews Clear
VideoProc has recently integrated an AI-powered feature called Reverb Control, specifically addressing audio clarity issues encountered in environments like underground parking lots. This tool uses advanced algorithms to pinpoint and reduce reverberations, a frequent cause of muffled and unclear audio in such spaces. It aims to make conversations and interviews recorded in these challenging settings much more understandable. Users have the ability to fine-tune settings to optimize voice clarity, potentially offering a useful solution for content creators facing acoustic challenges during filming. While this is a promising development, the full scope of its effectiveness and versatility in diverse environments has yet to be thoroughly explored. The inclusion of customizable settings suggests an effort to make tackling these typical recording obstacles more manageable.
VideoProc's AI-powered noise reduction, specifically its reverb control, offers an intriguing solution for capturing clear audio in challenging environments like underground parking garages. These spaces, with their hard surfaces and lack of sound absorption, tend to produce significant echo and reverberation.
VideoProc seems to address this by cleverly analyzing the audio's spectral makeup. It identifies the specific frequency bands that are most affected by the reverb, typically in the 200 Hz to 800 Hz range. Then, it applies tailored filtering to target and minimize these frequencies, minimizing the unwanted echoes. This targeted approach appears to be key to preserving the integrity of the speaker's voice, which is vital for preserving the clarity of interviews and conversations.
One of the more interesting aspects of the system is its ability to operate in real-time. This makes it useful for situations where the acoustic environment might be rapidly changing, which can happen frequently in places like underground parking. In addition, the system uses machine learning. This implies that the software learns over time as it encounters different audio environments and echo characteristics. So, theoretically, its performance should improve as it's exposed to more audio samples.
While the algorithms are complex, the user interface is designed with ease-of-use in mind. This makes it accessible to a broader range of users, even those without significant audio engineering experience. This user-friendliness combined with its ability to efficiently remove reverberation and unwanted background noises could be a significant boon for content creators, especially those dealing with challenging audio environments.
The reverb control also has a low latency design. This characteristic is important because it minimizes the delay between when the sound is recorded and when it is processed and filtered. This low latency is especially helpful in live interview situations, ensuring that the audio and video remain synchronized.
It's also worth noting that VideoProc's tools are not limited to underground parking. They can likely be used in a range of settings that struggle with unwanted reverberation, such as large conference rooms, auditoriums, or even public transportation environments. The ability to tailor the filtering based on the environment suggests that the technology can be applied across different use cases.
While promising, it's important to remember that these AI-powered systems are still evolving. It would be interesting to see how well VideoProc performs in situations with exceptionally complex reverberation patterns. Nevertheless, initial observations suggest that VideoProc offers a useful approach to capturing cleaner, more professional-sounding audio in environments prone to reverberation, leading to improved content and easier workflows for creators.
Experience error-free AI audio transcription that's faster and cheaper than human transcription and includes speaker recognition by default! (Get started for free)
More Posts from transcribethis.io: