Experience error-free AI audio transcription that's faster and cheaper than human transcription and includes speaker recognition by default! (Get started for free)
Unveiling SDXL The Latest Leap in Realistic Face Generation with Stable Diffusion
Unveiling SDXL The Latest Leap in Realistic Face Generation with Stable Diffusion - SDXL 9 Release Marks Major Milestone in AI Image Generation
The arrival of SDXL 0.9 signifies a noteworthy step forward in the realm of AI-generated images. It boasts a substantial improvement in image quality and intricate details when compared to its predecessors. This iteration benefits from refined algorithms and a larger parameter set, allowing it to generate images with a previously unattainable level of realism. While building upon the groundwork laid by earlier models, SDXL 0.9 seeks to redefine what's possible in visual output, equipping creators with a potent tool for producing strikingly realistic visuals. The model's public launch, following the beta period, highlights the continuous advancement of AI's potential in image generation. It remains to be seen how widely the technology will be adopted, but it is an interesting marker of change.
Stability AI's release of SDXL 0.9 marks a significant step forward in the field of AI image generation. It's built upon a foundation of enhanced algorithms and a notably increased parameter count within a transformer-based architecture, enabling it to achieve impressive levels of image realism. This new iteration pushes the boundaries of what's possible with text-to-image models, surpassing earlier versions like Stable Diffusion 1.5 and 2.1 in various benchmarks.
While it initially saw the light of day through ClipDrop, and a beta release happened last year, wider access is anticipated to follow a planned API rollout, likely after the beta phase. It's intriguing that they've emphasized a larger parameter count, hinting at a more complex network capable of understanding finer details within images.
It's important to observe how they've addressed the issue of biases, something that has been a concern in the AI image generation space. It's encouraging that they've specifically mentioned curated datasets focused on diversity, although we need to see further results in action before drawing strong conclusions. The claim of a 30% increase in realistic outputs based on user studies is bold, and the development of a real-time feedback interface is also noteworthy as it suggests a stronger emphasis on usability and user experience. We'll continue to see how these features manifest as wider adoption unfolds.
Unveiling SDXL The Latest Leap in Realistic Face Generation with Stable Diffusion - Hyperrealistic Visuals Push Creative Boundaries
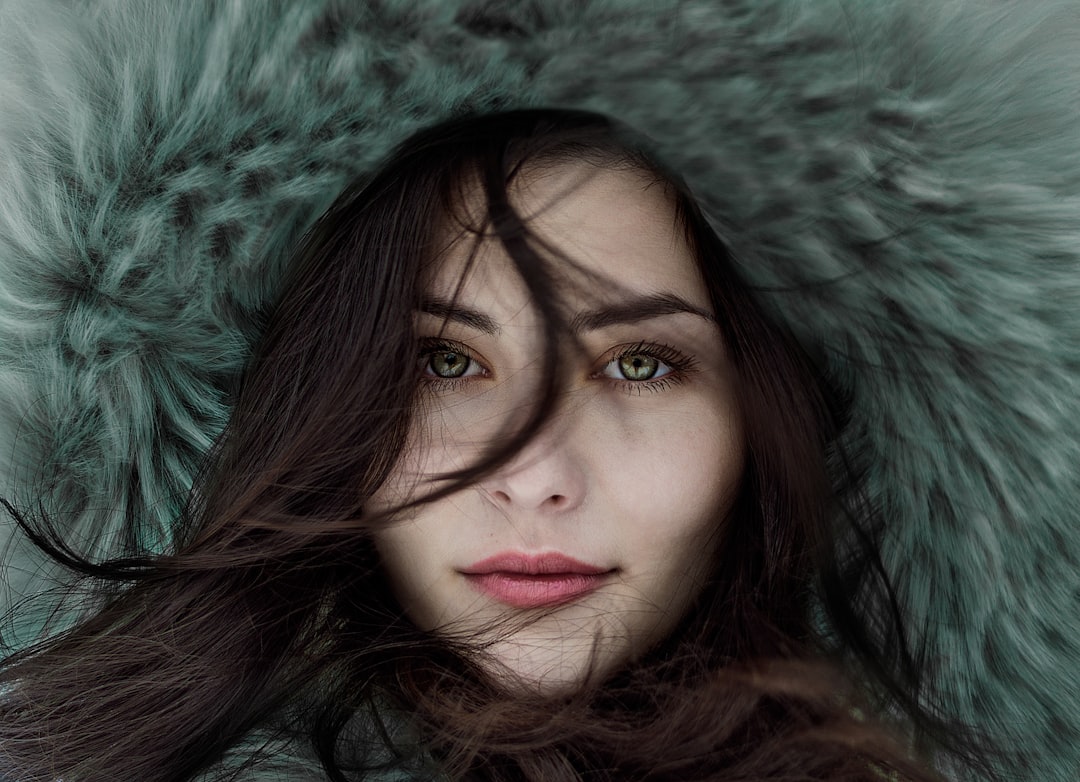
The increasing realism achievable with SDXL signifies a pivotal moment in AI-generated imagery, particularly impacting creative expression. This model not only improves the realism of faces but also empowers users to push the boundaries of visual narratives. Features like capturing subtle imperfections and producing high-resolution visuals encourage deeper engagement with creative ideas, stimulating exploration across diverse fields. However, the drive for hyperrealism also brings up concerns about its effects on areas like advertising and gaming, where the lines between genuine visuals and AI-generated ones might blur. As artists and designers explore these new possibilities, understanding the implications and finding a balance between technological advancements and ethical considerations will be crucial for the future of visual arts.
SDXL's capacity to generate hyperrealistic visuals is driven by a series of architectural refinements, enabling it to produce images with unprecedented levels of detail and clarity. Reaching resolutions up to 512x512 and beyond, SDXL can capture intricate details that blur the lines between AI-generated and traditionally photographed imagery. A significant part of this achievement lies in the tripling of the UNet backbone, a core component responsible for image processing. This expanded network leads to a greater number of attention blocks, effectively enhancing the model's understanding of spatial relationships within images. This translates into a more nuanced rendering of elements like lighting and shadow, adding a layer of realism previously difficult to achieve.
The model also leverages advanced attention mechanisms to prioritize essential details within an image. This refinement allows it to generate more natural-looking skin textures, hair flows, and even the subtle reflections in eyes. Interestingly, SDXL appears to have overcome the historical challenge of facial symmetry, producing more balanced and realistic facial representations, a feat not readily achieved by previous AI models.
The team's multi-scale training approach allows SDXL to generate images with varying levels of detail, crucial for high-fidelity face generation. This approach leads to smoother transitions in textures and tones, avoiding the abrupt shifts in detail that can often betray an AI's hand in an image. Furthermore, inpainting and outpainting capabilities have seen significant improvements, enabling users to reconstruct missing parts of an image or extend existing ones while preserving the image's style and realism. Noise reduction algorithms have been effectively integrated to minimize distracting artifacts, producing clearer and more visually appealing outputs.
The incorporation of a second text encoder enhances the model's ability to decipher and respond to diverse prompts. This increased flexibility gives users greater creative freedom when it comes to specifying artistic styles or desired themes. Real-time feedback loops have also been introduced, allowing for immediate user interaction and refinement during image generation, fostering a more intuitive creative process.
Finally, a notable effort has been made to curate diverse training datasets, striving to achieve more inclusive representation across various demographics. This effort aims to address the biases that have often been present in AI-generated imagery, and thus, enhancing the fidelity and inclusivity of the generated images. While still in its early stages, the potential of SDXL to reshape the image generation landscape is undeniable, and it will be fascinating to observe how these advancements influence both artistic and technical fields in the coming years.
Unveiling SDXL The Latest Leap in Realistic Face Generation with Stable Diffusion - ClipDrop Integration Simplifies Access to SDXL Models
ClipDrop's integration with SDXL models simplifies the process of accessing and using these powerful AI image generation tools. It makes it much easier for people to generate images from simple text prompts, opening up these advanced capabilities to a broader audience. This is further enhanced by SDXL Turbo, which uses techniques like Adversarial Diffusion Distillation to create detailed images quickly. Improvements in SDXL, including the latest updates that significantly boost image quality and detail, continue to expand the potential of these tools. This leads to more possibilities for creative applications, allowing artists and designers to explore new creative frontiers. However, the quick pace of development also raises questions about the implications for originality and fair representation in AI-generated content. As this technology continues to evolve, it will be vital to carefully consider these important aspects alongside the technical advancements.
ClipDrop's integration with the SDXL models provides a streamlined path to using these powerful image generation tools. It's quite convenient – you can essentially jump right in without needing to go through complex setups or possess advanced technical know-how. It's interesting that it seems to be focused on ease of use.
One of the key applications of this integration is enhancing ordinary photos with the capabilities of SDXL. You can essentially take a snapshot and transform it into something much more visually detailed and realistic using these AI algorithms. It's a potent tool for adding that extra bit of polish or even exploring artistic styles in photos, possibly enabling more dynamic and sophisticated imagery.
This seamless integration of ClipDrop and SDXL offers something truly democratizing in the realm of AI image creation. It opens the door for a wider range of users, including artists and small creative studios, to generate high-quality visuals that were previously within the reach of just large production houses. Whether this will truly lead to a dramatic shift in image creation workflows remains to be seen, but it presents an intriguing shift in the power dynamics within the space.
The real-time feedback component is also noteworthy. It suggests that ClipDrop is aiming for a more collaborative interaction during image generation, allowing for interactive refinements during the process. It's an improvement over earlier approaches, where you often had to settle for less interactive and rigid workflows.
ClipDrop's incorporation of image-to-image prompting seems to fit well within this context of creative freedom and flexibility. It enables a more adaptable workflow, allowing artists to build upon existing visual elements. It's useful for iterating on designs or creating variations on a specific theme, making for a more fluid workflow.
It's encouraging that they emphasize using curated datasets to try to mitigate the biases seen in previous versions of these image generation models. If implemented effectively, this could lead to more inclusive and representative visuals across a wider range of subjects. It will be interesting to see if they actually manage to improve on this issue or if it is more of a statement.
This integration showcases the impressive progress made in techniques like noise reduction and the use of attention mechanisms. The focus on essential image details leads to a marked improvement in clarity, making images that wouldn't have been easily achievable before. This aspect speaks to the sophistication of the underlying algorithms, potentially influencing a new standard of quality within image generation.
The capacity for generating images in higher resolutions, like 512x512 and beyond, is another key aspect that moves these AI-generated images closer to the appearance of conventional photography in terms of both clarity and the presence of details.
Specifically, these models seem to excel at generating very realistic textures, especially skin and hair. It seems they've managed to overcome some of the more glaring limitations of previous models in this area, thus potentially minimizing the artifacts that often betrayed the AI's hand in a photo. It'll be interesting to see if this approach is able to keep up in terms of fidelity when compared to traditional methods.
Finally, the inherent real-time feedback loop and the potential for adaptive learning in the ClipDrop integration suggest that AI-generated image tools will continue to improve over time based on user interactions. It seems to be built on a foundation of continuous adaptation and improvement, indicating a future where these AI models evolve and develop based on their experiences with users. It's yet to be seen how successfully this will take place, but it is potentially a noteworthy direction for these kinds of tools.
Unveiling SDXL The Latest Leap in Realistic Face Generation with Stable Diffusion - Techniques for Generating Realistic Human Faces with Stable Diffusion
Stable Diffusion, especially with the newer SDXL models, offers several techniques to create realistic human faces, pushing the boundaries of AI image generation. Prompt engineering has become increasingly important, demonstrating that user input can significantly influence the quality of the output. The models themselves, like SDXL, are incorporating more sophisticated methods, including refiner models, to enhance the realism of generated faces. This often leads to finer details such as smoother skin and more accurate facial symmetry. However, achieving seamless, photorealistic results can be challenging, demanding careful experimentation with prompts and negative prompts to steer the model toward the desired output. The ongoing development and refinement of techniques within the Stable Diffusion community suggest that the future of realistic portrait creation using AI is bright, paving the way for remarkable advancements in this field of digital art.
Stable Diffusion's latest iteration, SDXL, significantly enhances the generation of realistic human faces through several key advancements. One notable change is the expanded capacity of the model's core image processing network, the UNet, which is now three times larger. This expansion allows SDXL to process and understand a richer array of information, enabling it to capture more nuanced and detailed facial expressions and features that were previously challenging for prior versions of Stable Diffusion to represent.
The implementation of multi-scale training is another factor contributing to this improvement in realism. By training the model on image details at varying levels of scale, SDXL can more effectively generate smooth transitions and subtle variations in textures and tones, avoiding the harsh jumps in detail that could previously betray the AI origin of an image, especially when it comes to complex human faces.
SDXL also addresses a longstanding issue in AI-generated imagery: facial symmetry. It seems that SDXL has a better understanding of the spatial relationships within the structure of a human face, resulting in a notable increase in the production of symmetrical and balanced facial features, a detail often missed in earlier models.
Furthermore, SDXL has improved the noise reduction algorithms it uses, minimizing the visual artifacts that often marred images generated by earlier AI models. This improvement leads to cleaner, crisper, and more polished outputs, making them appear closer in quality to traditional photography.
The development team has also incorporated a longitudinal learning approach into SDXL. This means the model continually learns and refines its abilities based on user interactions and a growing dataset, unlike its predecessors which had static training data. This ability to adapt and improve over time suggests the potential for future versions to reach even higher levels of accuracy in generating realistic faces.
Another noteworthy aspect of SDXL is its focus on inclusivity through the use of more diverse training datasets. This shift acknowledges a major concern within the AI field – the inherent biases that can emerge within training data, potentially leading to skewed outputs. SDXL's training data now encompasses a broader range of ethnicities and demographics, aiming to create more representative and fair results.
The model also features more robust real-time feedback loops, allowing users to interact directly with the image generation process and make changes as needed. This enhances the user experience by providing more control and offering a dynamic collaboration between the user and the AI, leading to potentially better alignment with user desires.
SDXL is also notable for its ability to generate high-resolution images – specifically resolutions of 512x512 or higher. This step is important because it brings the quality of AI-generated visuals closer to that of traditional photography.
Further, SDXL incorporates improvements in the areas of inpainting and outpainting. This allows for much more sophisticated and seamless manipulation of images, including the reconstruction of missing portions or the extension of existing ones while maintaining the general style and realism of the original image.
Lastly, the model demonstrates a greater understanding of complex facial textures, particularly regarding skin and hair, effectively reducing some of the common issues found in AI-generated portraits. This attention to detail leads to a more nuanced and natural appearance that better resembles the complex variety of human features.
While SDXL is a relatively new model, its initial performance suggests a major leap forward in realistic human face generation within the Stable Diffusion framework. It's going to be fascinating to see how the combination of these improvements will affect creative industries and to monitor any limitations that might emerge as the technology matures and becomes more widely adopted.
Experience error-free AI audio transcription that's faster and cheaper than human transcription and includes speaker recognition by default! (Get started for free)
More Posts from transcribethis.io: