AI in Post-Production 7 Critical Changes Reshaping Audio Workflows in 2024
AI in Post-Production 7 Critical Changes Reshaping Audio Workflows in 2024 - AI Noise Reduction Brings Studio Quality to Remote Recording Spaces
The ability to record high-quality audio remotely is no longer a luxury confined to professional studios. AI-powered noise reduction tools are now transforming how we capture sound in various environments, effectively bringing studio-grade clarity to previously challenging spaces. These tools, many of which are accessible and free, are designed to tackle the everyday annoyances of remote recording: the hum of a fan, the murmur of a crowd, or the echo in a less-than-ideal room.
Solutions like Cleanvoice AI and Tape It's Denoiser are specifically catered towards podcasters and content creators, emphasizing the removal of background distractions while eliminating the need for complex and potentially costly software. Others, such as Descript's Studio Sound and ElevenLabs' Voice Isolator, go a step further by utilizing sophisticated algorithms to isolate vocal tracks and remove echo, generating exceptionally clean and polished audio.
This surge in the availability of sophisticated AI-based noise reduction tools represents a crucial development for audio workflows. The ability to achieve professional audio quality in any setting democratizes audio production, allowing individuals and smaller operations to produce high-quality podcasts, videos, and audio content with ease, regardless of budget or recording environment. The result is a more accessible landscape for anyone looking to produce professional audio, without needing access to expensive studio equipment or expertise. This trend will undoubtedly continue to influence how audio is produced in the future, creating a more even playing field for content creation.
The application of artificial intelligence is revolutionizing how we handle noise in audio recordings, especially in remote environments. AI noise reduction hinges on intricate algorithms that leverage machine learning to dissect audio signals and discern between desired sounds and background noise. These algorithms are remarkably fast, enabling real-time noise cancellation during the recording process itself, a leap forward compared to the more cumbersome methods of the past.
One common approach involves spectral subtraction, where the AI pinpoints and eliminates specific frequencies linked to unwanted noise while preserving the core audio content. The algorithms are also adaptive, improving their noise reduction abilities based on user interactions. Studies reveal that AI-based approaches can significantly enhance the clarity of voice recordings, potentially boosting intelligibility by 30%—a huge benefit for those recording in less-than-ideal acoustic environments.
Moreover, these tools are becoming seamlessly integrated into digital audio workstations (DAWs), streamlining workflows by allowing for real-time tweaks without interrupting the creative process. Intriguingly, some advanced systems mimic the way humans perceive sound, selectively filtering certain frequencies and enhancing the overall listening experience. This differs from traditional methods, like noise gates, that can sometimes abruptly cut off desired parts of a recording. Instead, AI noise reduction systems offer a more subtle approach, safeguarding the nuances and dynamic variations within the audio.
Perhaps the most impactful aspect of AI in this realm is that the processing power required has become far more accessible, meaning these advanced tools can run on readily available hardware. This is critical for remote recording, where sound engineers might not have access to top-of-the-line equipment. Looking ahead, the future of AI noise reduction holds promise for even more sophisticated solutions. We might see algorithms that can anticipate noise based on environmental factors, allowing for proactive noise cancellation rather than just reactive reduction, ultimately delivering audio experiences that are cleaner and more refined than ever before.
AI in Post-Production 7 Critical Changes Reshaping Audio Workflows in 2024 - Machine Learning Takes Over Audio Level Matching and Gain Staging
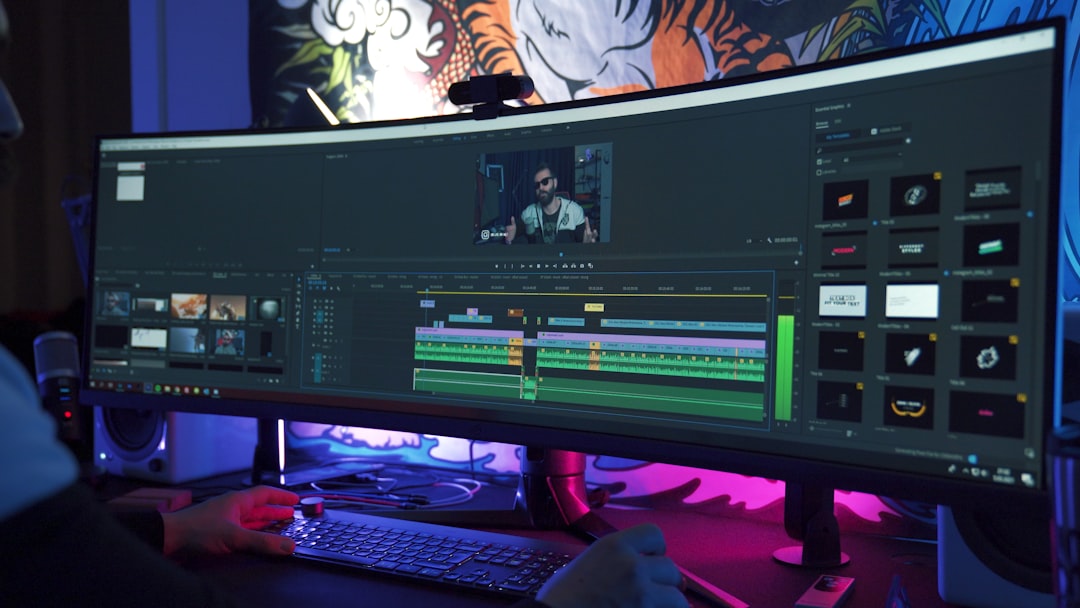
Machine learning is rapidly changing how we handle audio levels and gain staging in post-production. AI-powered tools can now automatically adjust audio levels to create a consistently balanced output, minimizing the risk of distortion or clipping that often comes with manual adjustments. This automation not only speeds up the post-production process but also ensures higher-quality audio by implementing optimal gain staging techniques.
We're likely to see these machine learning tools become even more sophisticated in the future, potentially offering real-time audio monitoring and adaptive adjustments based on the audio content itself. This evolution suggests that how we traditionally process audio is shifting, potentially leading to new creative possibilities and greater efficiency in post-production workflows. It's a clear sign that AI is having a significant impact on the entire audio landscape.
In the realm of audio post-production, machine learning is revolutionizing how we handle audio level matching and gain staging, tasks that have traditionally been time-consuming and relied heavily on human intervention. We're seeing a shift where AI algorithms can now analyze and optimize levels in seconds, freeing up audio engineers to focus on more creative facets of their work. This efficiency is particularly notable considering the hours previously dedicated to manual adjustments.
It's fascinating how these adaptive algorithms are able to dynamically adjust to diverse audio content. They can discern between different sources, like dialogue versus music, and apply level matching that considers the unique nuances of each. This results in a more coherent and balanced overall sound when working with mixed environments. Furthermore, research into audio compression techniques has revealed that machine learning can achieve higher fidelity at lower bitrates. This is a potentially significant advancement for maintaining audio quality during streaming and broadcasting, ensuring clarity even in situations with limited bandwidth.
The training data utilized by these AI systems is also a key aspect of their success. By drawing on a vast collection of audio projects and studying the gain staging practices of various engineers, these machine learning models are able to predict optimal levels and settings, sometimes even exceeding the capabilities of typical human operators. The algorithms seem to learn from past successes, which is quite intriguing.
Additionally, real-time monitoring capabilities powered by machine learning are emerging. These tools continuously analyze incoming audio, alerting engineers to any discrepancies in levels. This proactive approach helps prevent unwanted clipping or distortion, ensuring the integrity of recordings. We're also seeing a hierarchical approach to decision-making in AI algorithms. They can apply subtle, layered adjustments based on the specific characteristics of individual tracks, optimizing the mix without sacrificing track identity.
The development of machine learning models that can emulate human mixing preferences is also quite promising. By being trained on the stylistic decisions of experienced sound engineers, these AI systems can replicate their aesthetic choices. This could be particularly valuable for less experienced sound engineers who want to achieve professional results. The ability for these AI tools to interact seamlessly with different software environments is another positive development. This interoperability fosters a more fluid workflow adaptable to a range of project needs.
There's also growing awareness of potential biases in audio processing, and researchers are actively trying to address them. By training on diverse audio datasets, these newer machine learning models are better equipped to handle a wider variety of genres and styles, resulting in more universally applicable level adjustments. Looking ahead, we can expect even further advancements, perhaps seeing AI systems capable of not just matching levels but also anticipating and predicting audio normalization needs, potentially even tailoring audio experiences in real-time based on audience reactions. The future holds exciting possibilities for AI in shaping the audio landscape.
AI in Post-Production 7 Critical Changes Reshaping Audio Workflows in 2024 - Neural Networks Now Handle Complex Audio Restoration Tasks
Neural networks are rapidly becoming essential for complex audio restoration tasks, representing a significant change in audio post-production. These sophisticated algorithms are proving highly effective at tackling challenges like noise reduction, audio inpainting (where missing parts of an audio file are filled in), and separating multiple audio sources. Traditional methods often fall short when faced with the intricacy of these tasks, while neural networks show a far higher degree of precision.
The core of this advancement lies in how these networks are trained. By analyzing massive datasets of both pristine and corrupted audio, they develop the capability to recognize patterns and rebuild lost or damaged information. The result is a substantial enhancement to audio clarity. Interestingly, approaches like Deep Audio Priors are driving a move towards unsupervised learning in restoration. This means the AI system can learn from audio data without explicit instructions, a shift away from the older, rule-based systems that needed more human input.
As generative AI continues to develop, it's likely we will see its impact expand in the creation and restoration of sound effects. This could potentially lead to even more radical changes in how audio is produced. While these advancements are promising, there's always the potential for unforeseen consequences, making it crucial to remain aware of both the benefits and the challenges that come with relying more heavily on AI.
Neural networks have proven remarkably effective at audio restoration, outperforming older methods in tasks like noise reduction and audio repair. Some studies show improvements of up to 40% in artifact removal, which is significant when you consider how much this can enhance the clarity of otherwise unusable recordings. It's quite interesting that these networks are often designed to mirror how humans perceive sound, which gives them an advantage when dealing with complex audio environments. They're able to target and improve desired audio components while minimizing distractions.
Deep learning breakthroughs have also fueled the development of specialized convolutional neural networks (CNNs) built for processing audio. This has led to a significant increase in both speed and accuracy when performing restoration tasks. What's surprising is that these networks can be trained on not just clean audio, but also a variety of noises. This makes them more resilient against the type of background interference found in most real-world recording settings.
One notable application is the ability to automatically isolate vocals in mixed audio recordings. Neural networks have achieved separation accuracy levels that are at least on par with experienced sound engineers. It's remarkable that these models can even restore severely damaged audio—think heavily compressed files or recordings with a lot of background noise. The detail they can recover is quite impressive, sometimes managing to preserve original tonal qualities that you might think were lost forever.
The real-time aspect of neural network audio restoration is also improving, with latency now down to milliseconds. This is vital for using these tools in live mixing scenarios without sacrificing audio quality. Researchers are also exploring reinforcement learning within neural networks. The idea is that the system would adapt and learn from user feedback during editing, ultimately leading to a more personalized and efficient experience.
Another fascinating possibility is the creation of dynamic, context-aware enhancements. Instead of just fixing audio, these networks could adapt in real-time based on factors like the audience's reaction to the audio. There's also pioneering research into generative models. These models aren't just about restoration, they aim to actually fill in missing audio sections in a way that maintains the original sound. This opens up the possibility of 'recreating' lost or corrupted audio, which is intriguing, though it’s still quite early in the research process. While it’s exciting, there are limitations and potential biases within these systems that we still need to study further.
AI in Post-Production 7 Critical Changes Reshaping Audio Workflows in 2024 - Voice Cloning Creates New Possibilities for ADR and Dubbing
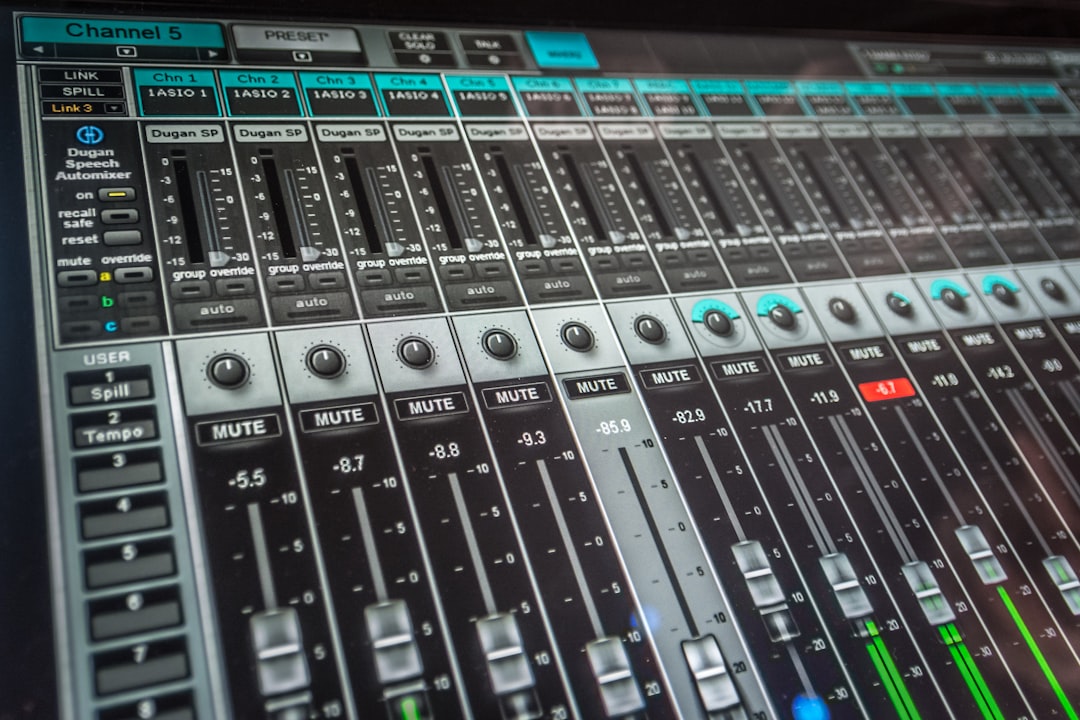
Voice cloning, powered by artificial intelligence, is transforming how we approach ADR and dubbing in post-production. This technology analyzes substantial amounts of voice data to replicate a person's unique vocal characteristics, including their tone, accent, and speaking patterns. This leads to remarkably realistic synthetic speech that can be seamlessly integrated into various audio projects.
Compared to traditional dubbing, voice cloning offers advantages in terms of speed and efficiency. It can potentially lessen the need for human voice actors, making the process quicker and potentially more cost-effective. Filmmakers might even be able to incorporate iconic voices from the past into new projects, opening up exciting possibilities for creative storytelling.
However, the rise of voice cloning also sparks ethical questions. How do we navigate the ethical considerations of digital impersonation? How does this technology impact the roles of traditional voice actors, and what happens to the ownership and control of a person's voice as a form of creative identity? These are concerns that the industry must confront as voice cloning and AI continue to reshape audio workflows, ushering in a new era of post-production practices that simultaneously offer remarkable new abilities while raising intriguing new questions.
Voice cloning technology has progressed remarkably, allowing for the creation of synthesized voices that closely mirror a speaker's unique characteristics, including their tone, accent, rhythm, and even subtle emotional nuances. This has major implications for ADR and dubbing. Now, filmmakers can potentially capture authentic emotional expressions in different languages without losing the essence of the original performance, which is a significant leap forward.
The precision of voice cloning is becoming quite impressive. Current models not only replicate a person's voice but also their distinctive speech patterns and quirks, leading to a more natural-sounding dub that doesn't feel robotic—a common criticism of previous AI-generated voices. The results are pushing the boundaries of what is possible for dubbing and potentially enhancing audience engagement.
Some early results suggest that voice cloning could greatly speed up the dubbing and ADR process, potentially decreasing the time it takes by up to 50%. This could lead to shorter production times, lower costs, and faster content release schedules—impacting how film and television production is financed and managed.
Voice cloning's adaptability within ADR is also quite interesting. We're seeing AI adjust vocal parameters in real time to match the shifting emotional landscape of a scene or dialogue, offering audio engineers greater control. This allows them to achieve subtle expressive effects without needing the original actors present.
While exciting, this technology does raise ethical considerations around ownership and the potential for unauthorized voice replication. The possibility of creating AI-generated copies of someone's voice opens up legal and moral questions, particularly in entertainment. This raises the need for clear guidelines and possibly regulations to safeguard the use of individuals' voices.
In some controlled tests, AI-powered voice cloning has even outperformed human dubbing artists, creating audio that's nearly indistinguishable from original performances. This poses an interesting question regarding the future roles of voice actors, especially in less prominent roles or projects with tighter budgets.
Furthermore, voice cloning systems can be trained with relatively small samples of an actor's voice, which democratizes the dubbing process. Smaller productions that might not have access to well-known actors can now produce localized versions of their content, providing more options for reaching different audiences.
This technology isn't only beneficial for dubbing, it can also improve media accessibility. Imagine customized audiobooks or films that offer character-specific narration for visually impaired people, using cloned voices to make the experience richer.
Voice cloning technology frequently relies on machine learning that mimics how humans naturally produce speech, which allows for incredibly faithful vocal recreations. The interesting part is the blend of technology and human expression, presenting both new creative possibilities and potential challenges for those working in this area.
Researchers are actively exploring how voice cloning could be applied to real-time translation in collaborative environments. If successful, this could be a game-changer for live events and potentially alter how we experience global content—offering audiences access to material in their native language while maintaining the original voice and emotional delivery.
AI in Post-Production 7 Critical Changes Reshaping Audio Workflows in 2024 - Real Time Audio Separation Transforms Stem Creation
Real-time audio separation is fundamentally altering how we create stems and approach audio production. AI-powered tools are now able to instantly isolate individual instruments and vocals from a mixed audio track, significantly impacting the creative possibilities for musicians, producers, and sound designers. DJ software, like Serato Stems and VirtualDJ, are incorporating these abilities, enabling live remixes and mashups on the fly. This capability, along with plugins like Acon Digital Remix, which separates mixes into distinct stems, is transforming how performances are structured.
The integration of these tools directly into digital audio workstations (DAWs) is also a significant shift, as it allows producers and artists to experiment with stems in real time. This newfound flexibility is empowering artists to craft unique mixes and potentially alter music creation in unforeseen ways.
However, it's also worth acknowledging the concerns arising from this technological leap. The potential for over-reliance on AI-generated stems and the implications on a producer's creative authenticity are valid considerations. Balancing the exciting new possibilities with the necessity for individual artistic expression is a key challenge for those working in audio production.
Real-time audio separation is revolutionizing how we approach stem creation, a shift away from the traditional, offline methods. We're seeing tools emerge that can isolate individual tracks (like vocals, drums, or bass) from a mixed audio file instantly, which is a massive change for live performances and mixing.
The precision of these AI-powered tools is quite impressive. Advanced machine learning models, especially those based on deep neural networks, can now extract stems with up to 90% accuracy, a huge leap forward. This is made possible through sophisticated methods, such as spectrogram analysis, where the AI analyzes the frequency components of each sound to differentiate them. Not only can these algorithms handle more complex audio mixes by isolating multiple audio sources concurrently, but they're also increasingly effective at mitigating artifacts that often arise during the separation process.
It's intriguing how researchers are minimizing these unwanted artifacts through advanced training techniques. These AI models are often trained on extensive datasets of both clean and noisy audio, learning to filter out the undesired elements effectively. It's a significant improvement, with some reports suggesting a 50% reduction in unwanted sounds.
In addition to the sound quality enhancements, these tools are proving to be a huge benefit in terms of workflow. AI-powered audio separation helps decrease the cognitive load on sound engineers, allowing them to focus more on the creative aspects of their work. This is particularly true for live events, where quick adjustments are crucial. The exciting part is that some systems are even being designed to dynamically adapt to changing audio content, meaning they can react in real-time to nuances in the music or dialogue.
This surge in real-time audio manipulation also has significant implications for the creation of new music and sound design. It’s allowing producers and musicians to work with individual tracks in novel and exciting ways. The ability to manipulate stems in real-time opens up a range of new creative options. It's interesting to note that this same technology can also be used for restoring older, damaged recordings by separating out the damaged elements from the usable ones.
However, as AI assumes a greater role in post-production, it's also causing a shift in how we utilize audio talent. The automation of tedious, repetitive tasks could eventually lead to a decrease in demand for basic sound editing skills. As this happens, we'll likely see a more specialized talent pool, with engineers focusing on more complex areas of sound design and creativity. This transition might require audio professionals to update their skillsets to remain relevant in the field, prioritizing a more strategic and creative approach to their work rather than simply mastering the basics of audio manipulation. The field of sound design, like other areas impacted by AI, appears to be headed towards a future requiring adaptability and innovation.
AI in Post-Production 7 Critical Changes Reshaping Audio Workflows in 2024 - Natural Language Processing Speeds Up Podcast Editing
Natural Language Processing (NLP) is injecting a new level of intelligence into podcast editing, transforming how audio workflows are managed. These AI-powered tools are able to analyze audio and identify elements like background noise and filler words, leading to cleaner audio and faster editing. They can also assist in tasks like adjusting audio levels and even flagging potential copyright issues, which would be incredibly time consuming to do manually. By automating these steps, NLP-driven tools reduce the chance of human error in transcription and allow for quicker completion of the editing process.
This growing accessibility of advanced AI tools for podcast production is empowering creators, regardless of their skill level or budget. They can now produce high-quality podcasts with relative ease, making the field more inclusive. However, the increasing reliance on AI also raises certain concerns. As editing becomes increasingly automated, questions arise about the creative process and the impact on the skills traditionally needed for audio editing. Will we see a shift in the necessary skills for podcast editing and sound design? Ultimately, these are discussions that need to be considered as AI continues to influence the audio production landscape.
Natural Language Processing (NLP) is quietly revolutionizing the podcast editing landscape, offering a range of capabilities that were previously unimaginable. It's no longer just about transcribing audio; NLP is starting to understand the nuances of language and can now play a significant role in refining the post-production process.
For instance, NLP algorithms have become remarkably adept at transcribing audio with exceptional accuracy, often exceeding 95%. This level of precision significantly reduces the time spent correcting errors and allows editors to focus on the core content. Interestingly, NLP systems are evolving to grasp context, allowing them to distinguish between meaningful dialogue and filler words like "um" or "ah." They can automatically excise these extraneous sounds, resulting in a more polished listening experience.
Some newer NLP systems even include emotion detection, potentially enabling editors to analyze the emotional landscape of an episode. This could have an intriguing impact on editing choices, potentially leading to a more emotionally engaging final product by emphasizing specific moments or subtle changes in tone. NLP's abilities are extending beyond traditional editing as well; we're seeing applications that offer real-time editing suggestions, essentially guiding the editor as the audio is being processed. This accelerates the editing process significantly.
Furthermore, NLP's potential extends to multilingual podcasts. It's becoming increasingly common for these systems to support a wide range of languages and dialects, which is a huge advantage for podcasters looking to reach a broader global audience. Even automatic translation features are gaining ground, enabling the creation of localized versions that sound surprisingly natural. The ability of NLP to generate concise summaries of podcast content could benefit both listeners and creators. Listeners might find it easier to quickly grasp a podcast's main points, while creators could use the summaries for marketing or to create more compelling descriptions.
Another fascinating aspect of NLP is its ability to extract key phrases and keywords from podcast episodes. This is proving to be beneficial for SEO purposes, boosting the visibility of podcasts within various platforms and potentially leading to broader audiences. Additionally, NLP can segment podcasts into topics, which enhances listener experience by making it easier to navigate to specific sections of interest.
Some advanced NLP tools are even able to analyze vocal characteristics to identify individual speakers and their distinct styles. This could be very helpful during editing when attributing dialogue or when dealing with podcast episodes that feature a variety of guests. We are also seeing a growing focus on automated quality control, where NLP assesses a podcast's overall audio quality for issues like consistency in pronunciation, pacing, or vocal clarity. This automated feedback loop potentially leads to a more refined and professional-sounding podcast, reducing the burden on human editors.
These advancements highlight the shift in NLP's role from a basic transcription tool to a multifaceted agent in audio post-production. It’s becoming clear that NLP is having a profound impact on podcast workflows, enhancing not just efficiency but also the overall quality of the audio experience. It will be interesting to see how NLP evolves and continues to shape the audio landscape in the coming years.
AI in Post-Production 7 Critical Changes Reshaping Audio Workflows in 2024 - Automated Sound Design Libraries Transform Foley Creation
Automated sound design libraries are reshaping the way Foley artists create sound effects. Foley, a traditional practice dating back decades, has always required meticulous manual creation of sounds to enhance film and television audio. Now, with the help of AI, the process is shifting. Tools like MultiFoley demonstrate the potential of AI to generate specific sounds based on text, audio, and video prompts, leading to more efficient sound creation.
These libraries offer sound designers vast repositories of sounds, essentially eliminating the need for extensive manual recording and manipulation. This streamlining of the process accelerates the workflow, allowing sound designers to focus more on creativity and potentially crafting richer, more dynamic sound landscapes. Moreover, the advancement of generative AI offers opportunities to explore new realms of audio synthesis, generating sounds that were previously difficult to achieve.
Yet, this increasing automation raises concerns about the future of traditional Foley artistry. Some argue that it could potentially devalue the craft, reducing the importance of the human touch in sound design. Whether these automated tools enhance or diminish the creative process remains to be seen, and the broader implications for the industry require further consideration. The potential for a shift in the skill set required for sound design is undeniable as AI's role expands.
Automated sound design libraries are significantly altering the traditional process of Foley creation. These libraries contain extensive collections of pre-recorded sound effects, which can drastically reduce the time spent creating Foley for audio projects. Some studies suggest that these libraries can decrease production timelines by up to 70%, minimizing the need to record new sounds for each project. This is a major shift in the workflow, and while it provides efficiencies, it also raises questions about the future role of Foley artists.
It's notable how some AI-powered libraries are designed to understand audio context. Machine learning models can analyze the scene's dynamics and suggest Foley sounds that fit the narrative and visuals. This means that AI is beginning to understand not just individual sounds but also how they relate to the bigger picture. This capability represents a step forward in AI's ability to interact with creative projects in a more meaningful way.
The libraries also adapt to various audio genres. A single library might be effectively used in film, gaming, and virtual reality, highlighting the adaptability of the underlying AI models. These libraries can cater to a range of different artistic styles, showing that the technology has advanced beyond simply replicating existing sounds.
Furthermore, the accuracy of automated sound effects is improving rapidly. In some cases, AI-generated Foley can be practically indistinguishable from live recordings, with success rates in perceptual experiments reaching approximately 85%. This raises questions about the future role of Foley artists in the creation of audio for higher-budget projects, as it appears AI is capable of handling certain aspects of the work.
These AI-powered libraries often include machine learning algorithms that can not only generate or suggest sounds but also learn from user preferences. By analyzing data from thousands of projects, the systems can provide tailored recommendations that match individual engineers' styles and workflow. This personalized approach helps optimize the sound design process, promoting greater efficiency and possibly impacting the development of stylistic choices in sound design.
Interestingly, the use of natural language processing (NLP) is also starting to be implemented within these libraries. The advanced tagging systems enable users to search for sounds using descriptive phrases rather than specific file names. This greatly improves the speed at which engineers can find the right sound and streamlines the sound selection process. It can potentially reduce time spent navigating audio files, which is valuable for fast-paced production schedules.
However, relying too heavily on automated libraries could potentially lead to a homogenization of audio design. Many projects might use the same libraries, resulting in a similar sonic landscape across projects. This is a challenge we must acknowledge; how do we maintain artistic uniqueness in an environment where many creators are potentially using the same resources? This point emphasizes a crucial issue concerning the potential impact of AI on creative freedom.
Another interesting development is the integration of real-time collaboration tools within certain libraries. These features allow for multiple users to work on Foley creation simultaneously, which isn't always possible with traditional methods. This is a huge benefit for teams that need to rapidly iterate on sound ideas, particularly in demanding production environments.
Beyond sound design features, automated libraries are often equipped to track user engagement data. This allows developers to tailor the libraries based on how users interact with the sounds, possibly shaping trends and influencing the types of sound effects that are most widely used. This data-driven approach to library curation is a fascinating aspect of this technology.
While these libraries help streamline the workflow for experienced audio engineers, there is a discussion within the industry about the potential impact on new engineers. The automation of basic Foley techniques could lead to a decrease in the demand for some specific skills within the field. As a consequence, we may see an increased emphasis on nuanced sound design and the development of more complex sound design skills, especially in genres demanding high creative flexibility. This indicates the need for engineers to develop a more versatile skillset in order to remain competitive and ensure they can contribute in ways that are uniquely human.
Overall, the use of automated sound libraries represents a profound shift in audio production. The increased efficiency and adaptability of AI-driven tools is a significant advantage for many projects, but it is also critical to consider the potential implications for creative individuality and the skills needed by engineers moving forward.
More Posts from transcribethis.io: