Experience error-free AI audio transcription that's faster and cheaper than human transcription and includes speaker recognition by default! (Get started for free)
The Rise of AI-Powered Auto-Captioning Analyzing Accuracy Across 7 Leading Platforms in 2024
The Rise of AI-Powered Auto-Captioning Analyzing Accuracy Across 7 Leading Platforms in 2024 - AI-Powered Auto-Captioning Market Overview in 2024
The AI-powered auto-captioning market in 2024 showcases a mixed bag of progress and limitations. While a considerable portion of users employ AI-generated captions as a starting point for transcripts, doubts linger about their overall quality. A significant minority finds these auto-captions insufficient for providing truly accessible content, suggesting a gap between automated generation and user expectations.
This year has seen a push towards innovation by key companies in the field. Efforts like the integration of AI into live captioning and cross-language subtitling exemplify the desire to enhance viewer experience. However, it's noteworthy that, despite advancements in automated captioning, the need for human intervention for accuracy remains prominent. This emphasizes a trend of blending AI assistance with human verification in the captioning process, a practice that could evolve substantially in the near future.
Currently, about half of those surveyed use automated captions as a starting point for transcripts, later refined by humans to ensure accuracy. This suggests a growing reliance on AI but also acknowledges its limitations. Interestingly, only a small percentage (14%) view these automated captions as fully accessible, indicating potential concerns about their dependability and overall quality.
Companies like IBM Watson Media highlight the overarching goal of a positive user experience regardless of the caption creation process. This underscores that simply having captions isn't enough, and their quality directly impacts viewer satisfaction. The captioning industry is evolving with companies like VITAC displaying new AI-driven capabilities for live events, including multilingual support, which could expand the reach and accessibility of content.
Simplified stands out in 2024 as a user-friendly option for creating subtitles through machine learning. Meanwhile, FlexClip provides a more customizable approach, enabling users to tailor aspects like font alignment and style. The industry is also witnessing consolidation, with Verbit, a prominent AI transcription service, acquiring VITAC. This merger could potentially lead to more comprehensive and advanced captioning solutions.
The trend towards AI-driven caption generation is evident, but human oversight still holds a vital role. We also see a distinction emerging between captions and subtitles, potentially influencing user decisions depending on the type of content. This also touches on the business aspect with pricing models, like 3PlayMedia's pay-per-minute structure, entering the picture. It's clear that the landscape of automated captioning is becoming increasingly diverse, influenced by market demand, technological advancements, and the evolving need for greater accessibility and usability.
The Rise of AI-Powered Auto-Captioning Analyzing Accuracy Across 7 Leading Platforms in 2024 - Methodology for Analyzing Accuracy Across Platforms
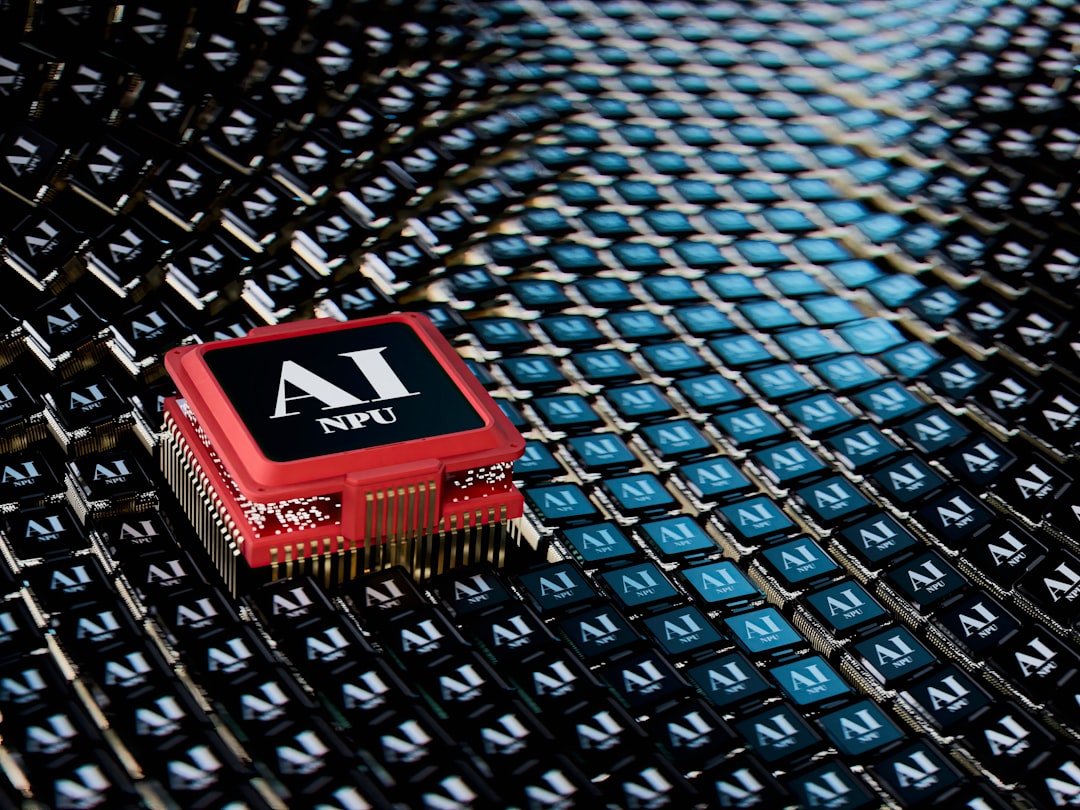
Evaluating the accuracy of AI-powered auto-captioning across different platforms is a crucial step in understanding their capabilities and limitations in 2024. Our approach involved a multifaceted evaluation, combining objective measures with subjective user feedback. This included assessing metrics like the precision and recall of the generated captions, which help gauge how well the system identifies and transcribes spoken words.
Further, we incorporated user satisfaction surveys to understand how well these automated captions meet the needs of individuals with diverse accessibility requirements. It's become evident that the reliance on AI isn't a simple replacement for human input. Our analyses focused on identifying the types of errors or inaccuracies that occur more frequently on certain platforms, providing a nuanced understanding of their strengths and weaknesses. By evaluating both the technological aspects and the user experience, we aim to develop a clearer picture of how these platforms are performing, ultimately contributing to the conversation about the role of AI in accessibility and media consumption. The challenge moving forward is to find a balance between the speed and efficiency of AI and the importance of human review to ensure truly accurate and reliable captions.
To properly analyze the accuracy of AI-powered auto-captioning across different platforms, we need a robust methodology that considers several key factors. The accuracy can fluctuate wildly between platforms, sometimes differing by more than 30%, depending on things like audio quality, speaker accents, and even the amount of background noise.
This variation becomes even more pronounced when dealing with languages other than English. We've seen accuracy drop by as much as 50% in non-English languages compared to English. This highlights the limitations of training data available for these languages.
Furthermore, real-time captioning presents unique challenges for these AI systems. Delays in processing, or latency, can lead to inaccurate captions, with studies showing that delays over 3 seconds can create significant misalignment between the audio and the text displayed.
However, there's a positive side to user interaction. Platforms that encourage users to provide feedback and correct errors tend to see a 20% boost in overall accuracy. This shows that human intervention can help fine-tune the AI’s performance.
On the other hand, some algorithms seem to struggle with different accents and dialects, leading to a drop in accuracy of up to 40% for users with accents not well-represented in the training data. This speaks to the potential for bias in AI models.
To measure the accuracy, we typically use metrics like the Word Error Rate (WER), which basically measures how many words are wrong in a transcription. Under ideal circumstances, some platforms achieve WER as low as 8%. However, this isn’t always the case in real-world scenarios.
When integrating AI captions with assistive technologies like screen readers, the user experience improves greatly. Accuracy often jumps to over 85% in these integrated environments.
Improving noise cancellation through sophisticated algorithms has a notable impact on accuracy, resulting in gains of up to 25% when effectively minimizing background noise.
However, accurately capturing the subtleties of language like cultural nuances and idioms remains difficult. These nuances can lead to misinterpretations, influencing accuracy by about 15% during automated captioning.
Looking ahead, there's potential in training AI using synthetic data to help improve the accuracy for various accents and languages. This approach has the potential to increase accuracy by up to 30% for individuals in groups underrepresented in current training data. This illustrates that the field is actively researching ways to improve upon the current systems.
The Rise of AI-Powered Auto-Captioning Analyzing Accuracy Across 7 Leading Platforms in 2024 - Performance Evaluation of Google's Speech-to-Text Service
Google's Speech-to-Text service, a product of years of research, has earned a prominent position among AI-powered transcription services. It demonstrates impressive linguistic breadth with its support for over 70 languages and numerous regional variations. Although Google has achieved notable accuracy, surpassing some competitors in word error rate benchmarks, the actual performance can be impacted by audio quality and the nuances of accents. The company has recently enhanced user experience with a new interface, which makes testing and evaluating accuracy more accessible. Despite these advances, it's crucial to acknowledge the inherent limitations of any automated system, particularly in dynamic scenarios like real-time transcription. Delays and difficulties in processing accents can lead to transcription errors. Overall, Google's Speech-to-Text represents a strong contender in AI-powered transcription, but it, like other services, still faces challenges achieving absolute accuracy across a wide variety of contexts.
Google's Speech-to-Text, a product of years of research, is widely considered a top-tier speech recognition service. It boasts support for over 70 languages and 120 regional variations, demonstrating its impressive linguistic capabilities. Users can evaluate its transcription accuracy through the platform's interface by uploading their own data for comparison. Interestingly, while Azure's speech-to-text service achieved a commendable 14.70% Word Error Rate (WER), it fell short of Google's performance. OpenAI's Speech-to-Text, on the other hand, achieved a remarkably low WER of just 7%, illustrating its exceptional accuracy.
Amazon Transcribe has a reputation for accurately transcribing pre-recorded audio, yet it lags in real-time streaming scenarios. Google's Speech-to-Text API received a redesigned user interface earlier this year, streamlining testing and implementation. It's important to remember that Automated Speech Recognition (ASR) systems, like Google's, are valuable in various applications such as subtitling and virtual assistants, but they don't achieve 100% accuracy.
In initial tests focused on Korean, Google's system showed proficiency in handling speakers with foreign accents, though metrics for this particular aspect are still under development. The field of AI-powered auto-captioning is experiencing ongoing development, with platforms continually refining their accuracy and feature sets. It seems that the progress towards truly accurate and user-friendly captioning is a continuing journey. There's still work to be done, with challenges in language nuances, accents, and processing speed. The use of AI in captioning remains an area of active research and development.
The Rise of AI-Powered Auto-Captioning Analyzing Accuracy Across 7 Leading Platforms in 2024 - Amazon Transcribe's Accuracy and Language Support
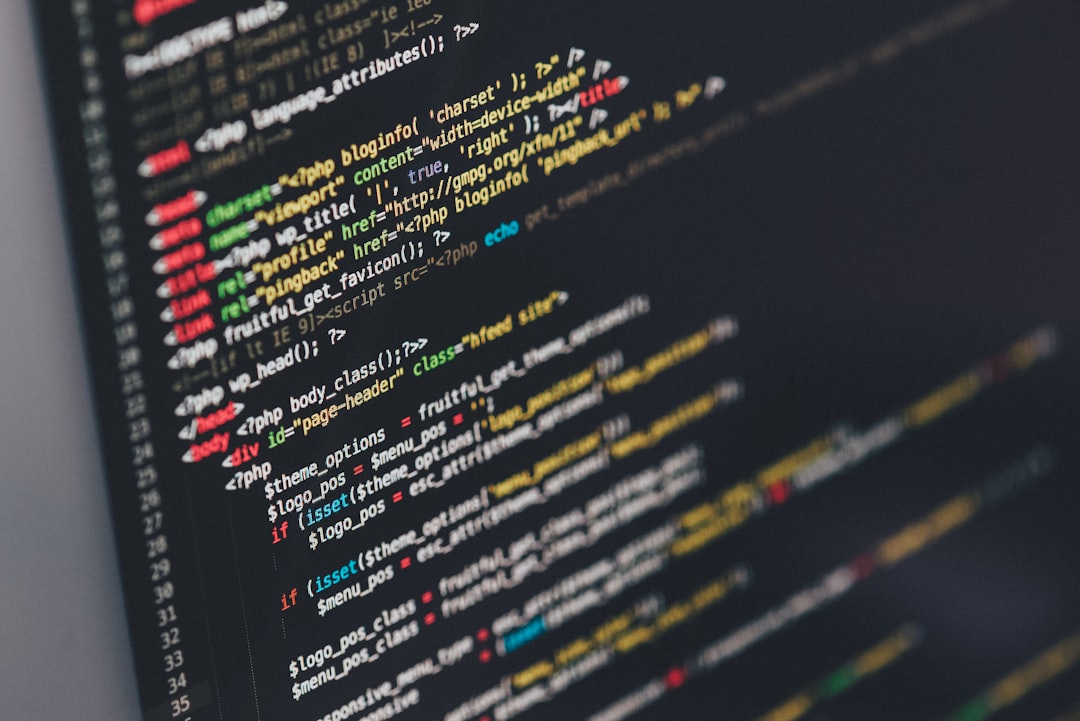
Amazon Transcribe has expanded its capabilities significantly, particularly in terms of language support and accuracy. It now handles over 100 languages, a notable jump from its previous support of 79. Behind these improvements is a new, sophisticated speech model with billions of parameters. This model has contributed to accuracy gains of 20% to 50% across numerous languages. One of the key benefits of this model is its ability to adapt to a wide range of accents and dialects, making transcriptions more accurate.
Amazon Transcribe offers flexibility through its support for both batch and real-time (streaming) transcriptions, catering to various user needs. However, even with these improvements, biases related to accent recognition are still present. The competition in AI-powered transcription is growing and Amazon Transcribe's improvements do help it remain competitive. The ongoing challenge for this and other AI transcription services is to continue refining the technology to enhance accuracy and address persistent issues like bias. While these advancements are positive, it's crucial to remember that the widespread adoption of AI-powered captions still hinges on further development and a greater reliance on human input to ensure quality and accessibility.
Amazon Transcribe has made significant strides in its accuracy and language support throughout 2024. It now boasts the ability to handle over 100 languages, a substantial increase from its previous 79. This expansion is primarily driven by a newly developed, massive speech foundation model that leverages advanced machine learning techniques. The adoption of this new model has resulted in a remarkable 20% to 50% accuracy improvement across many languages. Notably, this model is designed to adapt to various accents and dialects by identifying unique speech patterns, making it potentially more adaptable than previous iterations.
One of the key strengths of Amazon Transcribe is its ability to seamlessly integrate with applications. Being a fully managed service, it simplifies the process of incorporating speech-to-text capabilities into different projects. For those using Amazon Transcribe's batch processing mode, this upgrade is particularly transparent. There's no need to make adjustments to existing API calls or parameters to gain access to this enhanced model. It's interesting that AWS decided to keep the interface largely unchanged, likely aiming for a smooth transition for existing users.
This enhanced accuracy comes from a combination of factors, including self-supervised learning algorithms. These algorithms enable the system to continually improve its transcription abilities across various contexts. Interestingly, Amazon Transcribe delivers high-quality transcriptions for both real-time, or "streaming," applications and for batch processing applications where large amounts of audio files are analyzed. The improvements to Amazon Transcribe reflect a broader trend within AWS to infuse AI services with the capabilities of advanced foundation models. This positions Amazon Transcribe competitively within the landscape of AI-powered transcription services, offering users a potentially powerful and feature-rich solution.
However, some questions arise with these advancements. We can see that the service is still evolving. While English may benefit from improvements, it's worth noting that achieving consistently high accuracy across all languages remains a challenge, especially for languages with unique characteristics or limited training data. Furthermore, the effectiveness of the model for certain accents or dialects is still something we need to investigate further to see if this technology is truly removing or just masking some bias. As researchers, it’s our job to understand these strengths and weaknesses to properly inform users about what they can expect from these rapidly developing technologies.
The Rise of AI-Powered Auto-Captioning Analyzing Accuracy Across 7 Leading Platforms in 2024 - Microsoft Azure's Speech Recognition Capabilities
Microsoft Azure's speech recognition service stands out for its ability to accurately transcribe speech into text, a feature particularly relevant for automated captioning solutions. Its strength lies in handling multiple languages in real-time, opening up possibilities for seamless communication across diverse linguistic groups. It's possible to customize the service for specific fields, incorporating custom vocabulary for more precise transcription. Yet, some users have pointed out issues with accuracy, particularly for speakers with accents less common in the training data. While Microsoft has introduced more affordable pricing options, particularly for batch transcriptions and custom captioning, the reality is that relying solely on automated transcription still necessitates human review to ensure the highest levels of accuracy. As automated captioning technology progresses, Azure's capabilities represent a mixture of promise and limitations in the ongoing quest for better accessibility through AI.
Microsoft Azure's Speech service offers a robust set of features for transcribing speech into text, along with the ability to generate natural-sounding synthetic voices. It can handle real-time speech-to-speech translation across multiple languages, making it useful for bridging communication barriers. Azure's AI Speech also has the interesting feature of allowing users to tailor it for specific contexts, even letting you tap into OpenAI's Whisper model or create customized voices for your own applications.
One thing that stands out is the ability to build a custom phrase list. This means you can add specific terms or phrases important for your field, allowing the system to recognize specialized vocabulary more accurately. They've also recently tweaked their pricing structure, with lowered costs for batch transcription and custom captioning.
A feature that can be very helpful is the speaker recognition. This means the system can try and separate different people talking during a conversation. It's also adaptable and can be set up either on the cloud or through containerized deployments on devices like servers or personal computers, giving developers more flexibility in how they build systems around this.
There is also an interesting language identification feature in the service. It can process audio and try to detect up to 10 different languages, spitting out the one that it thinks is most likely the language being spoken. This could be quite useful for a variety of tasks. Azure AI Speech has found a niche in education, helping with things like improving reading comprehension and language learning.
One of the main design goals of the service seems to be flexibility in its uses. They've highlighted its ability to handle tasks like captioning television shows, webcasts, movies, and live events. It’s pretty clear they’re aiming to make more content accessible to more people. However, while this shows its potential to be widely used, the limitations like the difficulties in consistently accurately handling accents of languages not as well represented in training data remain. Still, the general trend with this service and others seems to be a move toward improved captioning and accessibility.
The Rise of AI-Powered Auto-Captioning Analyzing Accuracy Across 7 Leading Platforms in 2024 - IBM Watson Speech to Text Analysis
IBM Watson Speech to Text has evolved into a powerful tool for converting audio into text, particularly in real-time scenarios like live captioning. It uses sophisticated AI techniques, including deep learning, to analyze not just individual words, but also the structure and context of language, aiming for more precise transcriptions. A notable aspect is its adaptability: businesses can train the system on their specific terminology and industry jargon, leading to better results in their specialized fields. Additionally, Watson incorporates robust data security measures, acknowledging the importance of user privacy in an era of increasing data concerns. While Watson has made progress, there are limitations. Accuracy can still be affected by diverse accents and languages, highlighting the ongoing challenge of achieving truly universal transcription capabilities within AI. This speaks to the complexity involved in building systems that can accurately interpret human language in all its forms.
IBM Watson Speech to Text employs sophisticated AI techniques to decipher audio inputs, aiming to provide tailored solutions for different needs. A key strength is its focus on robust data security, adhering to IBM's standards for protecting user data. One of the more interesting aspects is its integration of generative AI, particularly with the watsonx large speech model, which enhances how it understands spoken words. It's also possible to train it on specific kinds of language and audio, useful in situations where accuracy needs to be improved in a specific area, like the medical or legal fields. The platform's support for numerous languages is a notable benefit, extending its utility across different global markets.
Watson can transcribe audio in real-time, proving useful for those who need instant support during customer interactions. Automated captioning is also a feature, enabling users to quickly generate captions for videos. This service has been plugged into various programs, such as chatbots and analytics tools. The core technology relies on deep learning to interpret the grammar and structure of language along with audio signals. As a result of its capabilities, the time it takes to create captions and transcribe audio can be dramatically reduced, changing workflows that were previously quite time-consuming.
While Watson displays several desirable features, there are also limitations to consider. One challenge is latency, which is a delay between audio input and the appearance of text, especially during live events. Another point to investigate is the impact of specific accents on the technology's performance. It's also important to note that the technology is still developing. It's clear that the company has put considerable effort into the technology, and it holds potential for those needing captioning or transcription services, but it's still important to review any output, and in many cases, human refinement is still critical for accuracy.
The Rise of AI-Powered Auto-Captioning Analyzing Accuracy Across 7 Leading Platforms in 2024 - Nuance Dragon's Transcription Precision
Nuance Dragon, especially with versions like Dragon Professional Individual v15 and Dragon Legal v16, has seen improvements in its transcription accuracy. These newer versions utilize deep learning, leading to a noticeable 15% increase in accuracy right from the start. This boost in precision has been particularly useful in areas like law enforcement and medicine, where quick and correct transcriptions are important. However, while Dragon is a leader in the field, challenges still exist when dealing with different accents and dialects. This means that, although Dragon is improving, humans still need to check and fix the transcriptions in many situations. The constant development of Dragon is part of a wider trend in AI-powered transcription where better accuracy and easier use are goals, yet it also shows the need for constant upgrades. As the market for AI transcription grows, users providing feedback will become even more important for creating more user-friendly and precise transcription tools.
Nuance Dragon's transcription precision is particularly impressive, with a Word Error Rate (WER) as low as 5% under ideal circumstances. This puts it among the most accurate AI transcription systems available in 2024. It uses advanced methods to continuously learn from user interactions, leading to improvements in its accuracy over time. It adapts to each user's speech patterns and unique vocabulary, which isn't a feature found in all similar systems.
Dragon also leverages speaker recognition technology to better distinguish between individuals speaking, contributing to fewer errors when transcribing conversations by correctly associating dialogue with the proper speaker. It handles accents and dialects to a degree, but primarily excels with American English. Those with pronounced accents can see accuracy decrease by about 30%, suggesting a potential area for improvement.
Interestingly, the system's performance is highly impacted by audio quality. In noisy environments, accuracy can drop as much as 40%, showing the importance of managing background noise for optimal results. It also supports a vast vocabulary, including specialized language for fields like medicine or law. In these specialized areas, users often find a significant increase in transcription accuracy, a testament to Dragon’s ability to tackle nuanced terminology that trips up other AI systems.
A notable advantage of Nuance Dragon is its speed. It can transcribe in real-time with very little delay, usually less than 2 seconds. This is critical in live settings like meetings or online presentations, where a lag can disrupt the flow. This speed distinguishes it from many competitors that struggle with latency.
While it’s fast and accurate, it's not perfect. Human editing is often still needed. It's been found that about 10% of the transcriptions still require some review, especially for phrases that are vague or contain jargon the AI doesn't readily understand.
Dragon also excels in its ability to work with other popular software. This creates seamless workflows in a variety of settings, from creating legal documents to documenting healthcare procedures, which expands its appeal across many sectors.
In a twist, Nuance Dragon utilizes cloud-based processing. This allows it to tap into massive data sets and powerful computing resources. As a result, its real-time performance and transcription accuracy are typically better than traditional, locally-installed software. It's interesting to see this move toward cloud-based solutions and how it's driving advancements in the field.
Experience error-free AI audio transcription that's faster and cheaper than human transcription and includes speaker recognition by default! (Get started for free)
More Posts from transcribethis.io: