The Evolution of Real-Time Translation Apps A 2024 Perspective
The Evolution of Real-Time Translation Apps A 2024 Perspective - Neural Machine Translation Sets New Industry Standards
Neural machine translation (NMT) has revolutionized the field of automated language translation, becoming the dominant approach in recent years. Its core innovation lies in using deep learning and artificial neural networks to view translation as a single, interconnected process. This approach has enabled NMT to generate more fluid and contextually appropriate translations compared to older methods. However, the gains from NMT are not universally experienced; its effectiveness can vary greatly between languages, highlighting potential inequities in access to improved translation technology. The integration of large language models suggests that the future of machine translation will continue to evolve, and NMT will likely remain the primary framework moving forward. The impact on real-time translation apps has been significant, fundamentally altering how we communicate across language barriers in personal and professional settings.
The field of machine translation has seen a dramatic shift with the rise of Neural Machine Translation (NMT). This approach, utilizing deep learning, treats translation as a unified learning problem, a significant departure from earlier rule-based systems. The groundwork for NMT was laid in the mid-2010s, and it's rapidly become the dominant method, showcasing impressive accuracy gains compared to older statistical techniques. We've seen accuracy improvements of up to 60% with NMT, which is remarkable.
However, the journey towards perfect translation is far from over. NMT, while powerful, can struggle with the nuances of language, particularly idioms and expressions. Building robust NMT systems requires enormous datasets, often involving millions of sentence pairs, highlighting the need for a lot of data across many language pairings. This highlights that, while NMT is a significant leap, language is complex, and the process of capturing all the intricacies of every language pair is still a research challenge.
The architecture of NMT typically involves complex neural networks, like LSTMs, which help the system retain information over longer parts of sentences. Some models also use attention mechanisms to focus on relevant parts of the input, leading to better handling of complicated phrases. The advancement of Large Language Models (LLMs) is now also influencing the future of NMT, suggesting even more exciting breakthroughs are on the horizon.
Furthermore, the application of NMT extends beyond text to encompass real-time speech translation, opening new possibilities in cross-lingual communication. User feedback is also crucial in refining these models, creating a cyclical improvement process. However, challenges persist in handling cultural context and specialized terminologies across different languages. The computational demands of NMT also remain high, often necessitating specialized hardware for optimal performance. In essence, NMT has set a new benchmark for machine translation, but a number of important research questions remain.
The Evolution of Real-Time Translation Apps A 2024 Perspective - Apple's Siri Expands Offline Translation Capabilities
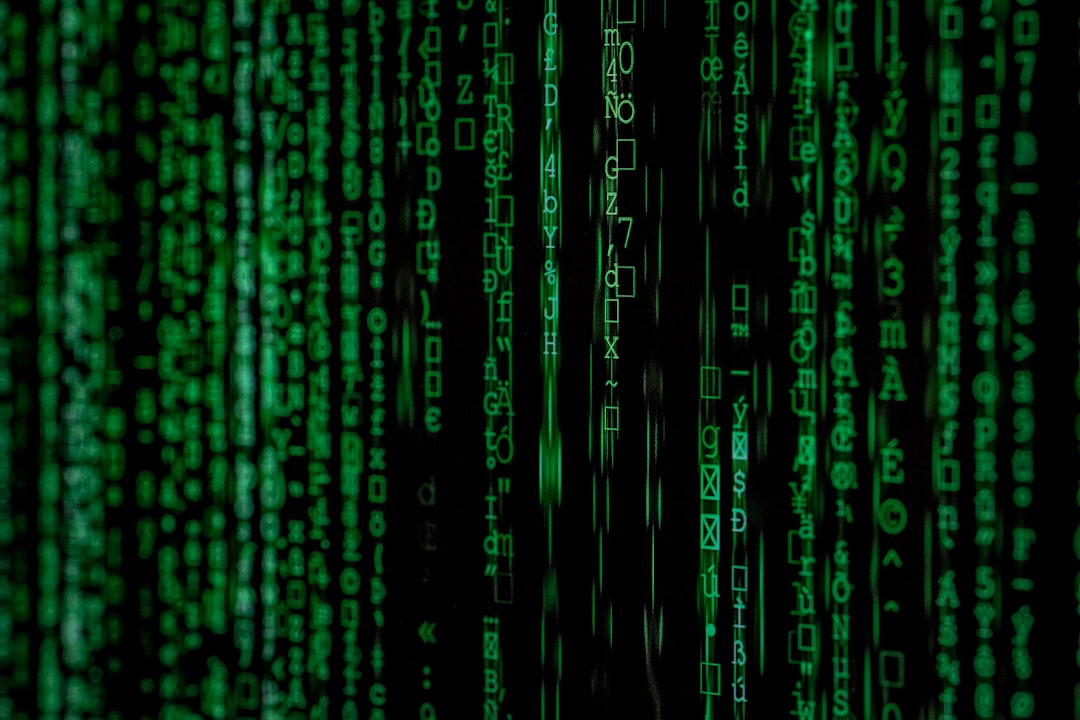
Apple's Siri has recently expanded its offline translation abilities, enabling users to translate between a range of languages without needing an internet connection. When first introduced, the feature offered translation support for 11 languages, covering major languages such as Spanish, Chinese, and Arabic. This offline capability is a boon for communication in situations where internet access is limited or unavailable.
The Translate app utilizes built-in machine learning to power real-time translation for both speech and text. Users can also download language packs for offline use, which is particularly helpful when traveling or in areas with poor connectivity.
While these advancements are noteworthy, accurate translation remains challenging, especially when dealing with subtle language nuances, idioms, and cultural context. These limitations highlight the ongoing complexities of producing truly effective and natural-sounding translations across different languages.
Despite the ongoing challenges, Siri's expanded offline translation capabilities reflect the broader push within real-time translation apps to bridge communication gaps and improve multilingual interaction in various contexts. The continued development of these apps suggests that we'll likely see further improvements and new approaches in this vital area of communication technology.
Apple's recent expansion of Siri's offline translation capabilities is an interesting development in the realm of real-time translation apps. Previously, these apps heavily relied on cloud processing for translations, but this change allows users to translate between languages even in areas with limited or no internet connectivity. It's a notable shift towards making translation more accessible in diverse environments.
This new capability leverages advanced algorithms capable of handling multiple languages offline, suggesting a growing proficiency in interpreting the subtleties of language without constant access to external data sources. This is especially useful in contexts where immediate translation is essential, like travel or emergencies.
The core technology powering Siri's offline translation feature appears to be neural machine translation (NMT). This allows the system to draw on learned patterns from existing data, resulting in translations that are more contextually relevant even without an active internet connection. This is a departure from earlier methods, which tended to produce more literal translations.
Interestingly, the offline translation models are trained on specific language datasets, including dialects. This targeted approach likely results in more accurate and localized translations when internet access is unavailable. However, this also means users have to download specific language packs, which can consume considerable device storage. Users with limited storage may need to make choices about which languages they want to access offline.
While impressive, offline translation capabilities still seem to encounter difficulties with intricate sentence structures and idiomatic expressions. This suggests that there are limitations to the current language models, which might not have been trained extensively on certain phrases or regional dialects.
On the positive side, the speed of offline translation through Siri has significantly improved thanks to optimizations in the underlying algorithms. This leads to almost instantaneous translations when the processing is handled locally.
It's important to note, however, that these offline functionalities won't receive updates or improvements in real-time. This could be a limiting factor in situations where language use is rapidly evolving.
From a privacy standpoint, the offline translation feature is an advantage. Sensitive conversations don't need to be transmitted over the internet, reducing the risks associated with data interception.
While exciting, the evolution of Siri's offline translation capabilities raises some questions about how language processing resources will be allocated across devices. As users expect more comprehensive and nuanced translations across a wider variety of languages, how this will affect both the device itself and the developers will be interesting to observe.
The Evolution of Real-Time Translation Apps A 2024 Perspective - Rise of Speech-to-Speech and Speech-to-Text Applications
The evolution of real-time translation apps continues to be driven by the growing importance of speech-to-speech (S2ST) and speech-to-text (STT) capabilities. The market for STT technologies is experiencing substantial growth, propelled by significant improvements in neural networks which have increased the speed and precision of converting spoken words into written text. While these technologies are improving rapidly, the inherent complexities of human language necessitate ongoing human involvement to ensure accuracy and to capture the subtle nuances that machines can struggle with.
S2ST applications are gaining traction as they combine elements of automated speech recognition, machine translation, and text-to-speech to make multi-language virtual meetings and cross-cultural interactions more seamless. The research landscape for S2ST is vibrant, with a focus on applications spanning from real-time video call translations to automated dubbing. This burgeoning area of technology is not only showing improvements in its core functionality but also raises intriguing questions about how well it can convey the depth and nuances of human languages in a way that fosters true understanding. As we move through 2024, these technologies promise to play an increasingly prominent role in shaping how we communicate across language barriers.
The field of real-time translation has seen a surge in the development of speech-to-speech (S2ST) and speech-to-text (STT) applications. These advancements are largely due to improvements in understanding the nuances of human speech, not just the words themselves, but also the emotional tone and inflection within them. Systems are becoming more attuned to the subtleties of human communication, allowing for translations that better capture the intended meaning.
Furthermore, there's a growing emphasis on recognizing the diversity of human language. Real-time speech recognition (ASR) systems are incorporating acoustic models that can discern various accents and dialects, which is crucial for providing effective cross-cultural communication. While promising, the accuracy of these systems varies depending on the environment. Controlled settings see impressive accuracy (upwards of 95%), but real-world situations are often more challenging due to factors like background noise and the inconsistencies inherent in human speech.
Researchers and engineers have made significant strides in the core technology behind speech recognition. End-to-end neural networks are gaining traction, simplifying the architecture of STT models while simultaneously reducing the latency of translation outputs. This is a crucial development for real-time applications where speed is essential. The demand for multilingual translation features is also on the rise, as users increasingly desire apps that can handle multiple languages seamlessly during conversations. We're starting to see a shift towards more intricate translation scenarios within real-time communication.
Another area of development is the integration of contextual understanding in STT. Through reinforcement learning, models are gaining the ability to learn individual speech patterns, which can enhance the accuracy and relevance of translations over time. The combination of automatic speech recognition (ASR) and neural machine translation (NMT) has proven powerful in creating real-time applications, but integrating a natural flow within translations, particularly in rapid-fire exchanges, remains a challenge. Synchronization of speech with the pauses and phrasing required for translation is something that needs continuous improvement.
It's also worth noting that S2ST and STT technologies are being explored beyond human-to-human communication. Researchers are investigating how these technologies can improve interactions between AI agents and humans, opening up the possibility for advancements in areas like customer service and virtual assistance.
Despite these leaps, one major hurdle remains: the inherent ambiguity of spoken language. Words can have multiple meanings based on context, making it a complex challenge for current models. Ongoing research aims to create more advanced parsing techniques that can accurately discern intent.
Thankfully, these powerful translation tools are becoming increasingly accessible. Many smartphones now incorporate deep learning algorithms, making high-quality translation services available to a wider audience, bringing the benefits of cross-cultural understanding to more people. The democratization of these technologies is potentially a significant development for global communication.
The Evolution of Real-Time Translation Apps A 2024 Perspective - AI-Driven Translation Dominates the Landscape
In 2024, AI-powered translation is reshaping the communication landscape, making it easier to bridge the gap between languages in our increasingly interconnected world. The evolution of AI, particularly in machine learning and generative AI models, has led to a significant leap in real-time translation, with applications delivering more accurate and natural-sounding translations than ever before. While these technologies are making conversations across languages more accessible, there are still hurdles to overcome in translating subtle linguistic elements and cultural contexts. Furthermore, combining AI with human expertise is proving crucial to streamlining and expanding the scope of translation, especially when dealing with diverse media formats. The translation industry is in a period of rapid change, and continued research will be critical to both addressing current limitations and realizing the full potential of these powerful tools for global communication.
The dominance of AI-driven translation, specifically neural machine translation (NMT), is undeniably reshaping how we communicate across languages. However, the landscape is far from uniform. While NMT excels with major languages, its performance with lesser-known tongues can be significantly weaker, creating an imbalance in accessibility. This disparity underscores the need for further development to ensure that real-time translation benefits all language communities equally.
The intricate workings of NMT often involve complex architectures like Transformers, which allow the models to capture long-range dependencies between words, leading to more natural-sounding translations. Yet, even with these sophisticated methods, translating cultural nuances remains a major obstacle. In sensitive scenarios like legal or medical interactions, overlooking cultural context can result in serious misunderstandings. Similarly, the diversity within languages themselves presents a challenge. Dialects and regional variations introduce a complexity that current models often struggle with, leading to inaccuracies and potentially frustrating experiences.
Even though speed is crucial for real-time translation, achieving consistently low latency remains difficult. Any noticeable lag can disrupt the natural flow of a conversation, especially in dynamic exchanges. Furthermore, the computational demands of advanced NMT models can be substantial, hindering their use on less powerful devices. This restricts accessibility, creating a potential divide based on users' technological capabilities.
Privacy concerns also arise with the increasing adoption of offline translation features. While beneficial for limiting data transmission, storing vast language packs locally raises questions about the security of user data. The potential for unauthorized access to personal devices storing sensitive information becomes a significant issue.
Moreover, the quality and bias of training data used to develop NMT models are crucial. Datasets reflecting biases can perpetuate stereotypes or exclude minority languages. This can lead to limited functionality and reinforce existing power structures within communication. The field is also exploring how to incorporate multi-modal inputs – blending speech, text, and visual cues – to enhance understanding and contextual accuracy, suggesting a future where translation goes beyond words to encompass the totality of human communication.
Crucially, user feedback plays a vital role in shaping these systems. By continuously adapting to users' preferences and providing corrections, translation models can steadily improve. This dependence on user engagement showcases both the limitations and the potential for future growth in the field, highlighting the constant interplay between human interaction and machine learning in achieving ever-more accurate and nuanced language translation.
The Evolution of Real-Time Translation Apps A 2024 Perspective - Real-Time Translation Streamlines Global Business Communication
Real-time translation is becoming vital for businesses operating across borders, enabling smoother communication and collaboration among people speaking different languages. AI's role in making these tools more accurate and readily available has been a game-changer, allowing companies to engage in international negotiations and partnerships with increased efficiency. Despite advancements, accurately conveying subtle language aspects like cultural nuances and idioms remains a hurdle, potentially affecting communication clarity. Businesses can use these tools strategically to gain a competitive edge, but understanding their limitations is crucial. Ultimately, real-time translation is playing a pivotal role in shaping the global marketplace and fostering more meaningful interactions across cultures.
Real-time translation applications have significantly compressed the lag in international business interactions, with some systems achieving near-instantaneous translation, enabling smooth conversations previously hindered by language differences. These apps often use sophisticated neural networks that can learn and refine their translations during use, allowing users to provide feedback that improves accuracy over time. This dynamic interaction between human expertise and machine learning is a unique aspect of this technology.
While these systems are becoming increasingly widespread, their success isn't uniform across all languages. There appears to be a correlation between the accuracy of translations and the frequency with which the language pair is used. For example, translating between English and Mandarin generally achieves higher accuracy rates compared to interactions involving less commonly spoken languages. This disparity highlights the impact of language resource availability on translation quality.
The capability of these applications to translate speech into text, particularly in quiet conditions, is quite remarkable, often exceeding 95% accuracy. However, the accuracy drops notably in real-world situations where ambient noise is prevalent. This difference underscores that, although the technology is advancing rapidly, challenges remain in translating human speech across various environments.
Furthermore, these apps can now support a large number of languages simultaneously, some exceeding 100. This ability enables them to serve teams and business interactions that involve a multitude of languages. However, efficiently managing the complexity of multiple languages within a real-time conversation presents a significant hurdle for developers.
Interestingly, some AI models are showing an ability to discern emotional cues in speech, thus allowing translation systems to convey the speaker's sentiments along with the translated words. This capability hints at a potential future where translation becomes a more holistic and nuanced experience.
The expanding features of these apps, like offline translation, bring increased accessibility but also introduce complexities related to security. Storing extensive language data on a user's device for offline use poses potential vulnerabilities if the device were to be compromised. This risk necessitates discussions regarding data privacy and security as these apps evolve with new functionality.
The limitations of even the most sophisticated AI translation systems become apparent when dealing with subtle cultural elements, such as idioms and region-specific expressions. These linguistic nuances can easily be misconstrued by AI without careful consideration, reinforcing the need for human supervision, particularly in sensitive contexts.
Researchers are exploring ways to integrate visual information, like gestures and facial expressions, into the translation process, aiming to establish a more complete understanding of conversations. If successful, this approach could represent a major breakthrough in how we communicate across language barriers, moving beyond simple word-for-word translation to a more holistic interpretation of human communication.
The field of real-time translation is continually evolving, with researchers pushing the boundaries of the technology to improve its accuracy, expand its language coverage, and address the complex challenges presented by diverse cultures and languages. These developments showcase the ongoing research efforts to create truly effective tools for global communication.
The Evolution of Real-Time Translation Apps A 2024 Perspective - Cross-Cultural Communication Demands Drive Innovation
The increasing importance of cross-cultural communication is fueling innovation in the field of real-time translation. Our interconnected world necessitates tools that can accurately and effectively convey meaning across languages, driving the need for more sophisticated translation technologies. This surge in demand for seamless communication impacts various aspects of our lives, including fostering global partnerships, making digital content accessible to wider audiences, and smoothing the path for travel experiences. The translation field is actively responding to these demands, continuously refining its methods through artificial intelligence and machine learning. These technological advancements are transforming how we navigate linguistic differences and interact across cultures. However, the intricate nature of language and the subtle nuances of cultural contexts pose ongoing obstacles that the field is striving to overcome through continued innovation.
Our increasingly interconnected world highlights the growing importance of cross-cultural communication. It's become clear that language barriers can be a significant hurdle to collaboration, especially in global businesses. Studies suggest that a substantial portion of employees perceive miscommunication as a drain on productivity and resources. It's not just about the words themselves, but also about the mental energy required to bridge cultural gaps and understand unspoken norms. This cognitive load can hinder decision-making and even stifle creative problem-solving in collaborative environments.
However, these communication challenges also serve as a powerful incentive for innovation. Companies with teams representing diverse cultural backgrounds are more likely to generate novel ideas and achieve greater revenue from their innovative endeavors. Recognizing and addressing cultural nuances through training programs has demonstrably improved communication, reducing the frequency of misunderstandings.
The shift towards remote work has further amplified the importance of clear communication, particularly in digital settings. In the absence of face-to-face interactions, subtle cues can be lost, increasing the chances of misinterpretations and conflicts. It's a challenge for both humans and the AI tools we're developing.
A notable inequality in the current state of translation technology is the uneven distribution of language resources. While widely used languages benefit from advanced translation systems, lesser-known languages lack the same level of support. This disparity creates a barrier to equitable collaboration. Moreover, the ability of current AI models to accurately convey emotional nuances within conversations remains a challenge. Research shows that they still struggle to properly capture the emotional tone of human speech in a significant number of cases.
However, fostering cultural intelligence within teams can help to mitigate these challenges. Individuals who are skilled at navigating cultural differences tend to create more effective and creative collaborative environments. Companies with a focus on cross-cultural initiatives gain a strategic edge by expanding their market reach and gathering valuable insights from diverse linguistic backgrounds.
The integration of advanced translation technologies plays a crucial role in this evolution. These tools help not only break down language barriers but also create a culture of continuous learning, encouraging individuals to enhance their linguistic and cultural understanding. This, in turn, fuels innovation and collaboration across borders. As the field progresses, it's vital to continue exploring solutions that promote more inclusive and effective global communication.
More Posts from transcribethis.io: