Experience error-free AI audio transcription that's faster and cheaper than human transcription and includes speaker recognition by default! (Get started for free)
7 Key Challenges in Automated English to Urdu Translation A 2024 Analysis
7 Key Challenges in Automated English to Urdu Translation A 2024 Analysis - Handling Idiomatic Expressions and Cultural Nuances
The challenge of accurately translating English idiomatic expressions and cultural nuances into Urdu continues to be a hurdle for automated translation systems. Idioms, tightly woven into a language's cultural fabric, often lack direct equivalents in other languages. This presents a complex problem for translation algorithms, particularly as they rely on identifying patterns and relationships between words. While 2024's AI-powered translation tools are showing improved ability to deal with idiomatic language, the nuances of cultural context remain a stumbling block. To translate these phrases successfully, AI systems need to move beyond simple word substitutions and delve into understanding the deeper layers of meaning inherent within the expressions. This means incorporating a more comprehensive understanding of cultural references and ensuring translations don't just convey the literal meaning but also capture the intended impact and emotional resonance within the Urdu context. Ultimately, the ongoing development and evaluation of translation systems will need to focus on effectively managing this challenge, assessing how well they can navigate the diverse and complex tapestry of cultural implications that characterize idiomatic expressions. Failing to achieve this level of translation can lead to inaccurate and even misleading results.
The inherent difficulty in translating idiomatic expressions stems from their deep connection to cultural fabric. They encapsulate a community's history, customs, and social norms, making it tough for machines to capture their true essence. Automated translation systems often falter with idioms because direct equivalents may not exist in the target language. This necessitates ongoing refinement of algorithms to recognize and handle these unique expressions.
To effectively translate, AI must go beyond simple word-for-word substitutions. It needs to grasp the deeper cultural nuances embedded within language. This task is particularly challenging for idioms and other figurative language, which demand a thorough understanding of both the source and target cultures and their respective languages.
While 2024's machine translation systems exhibit improved capabilities in handling idiomatic expressions compared to previous iterations, the complexity remains substantial. A major challenge is maintaining coherence and context, especially when dealing with lengthy documents. Simply replacing idioms with literal translations often results in misinterpretations because the intended meaning gets lost.
Large language models (LLMs) offer promising avenues for improvement in this domain. Their ability to analyze historical and contextual language patterns allows them to potentially achieve better translations of idiomatic expressions. However, the evaluation of these AI systems must critically assess their handling of culturally sensitive and idiomatic language, identifying both their strengths and weaknesses. Traditional MT approaches struggle with the non-compositional nature of idioms, frequently falling short in delivering accurate translations without sacrificing context.
7 Key Challenges in Automated English to Urdu Translation A 2024 Analysis - Addressing Grammatical Structure Differences
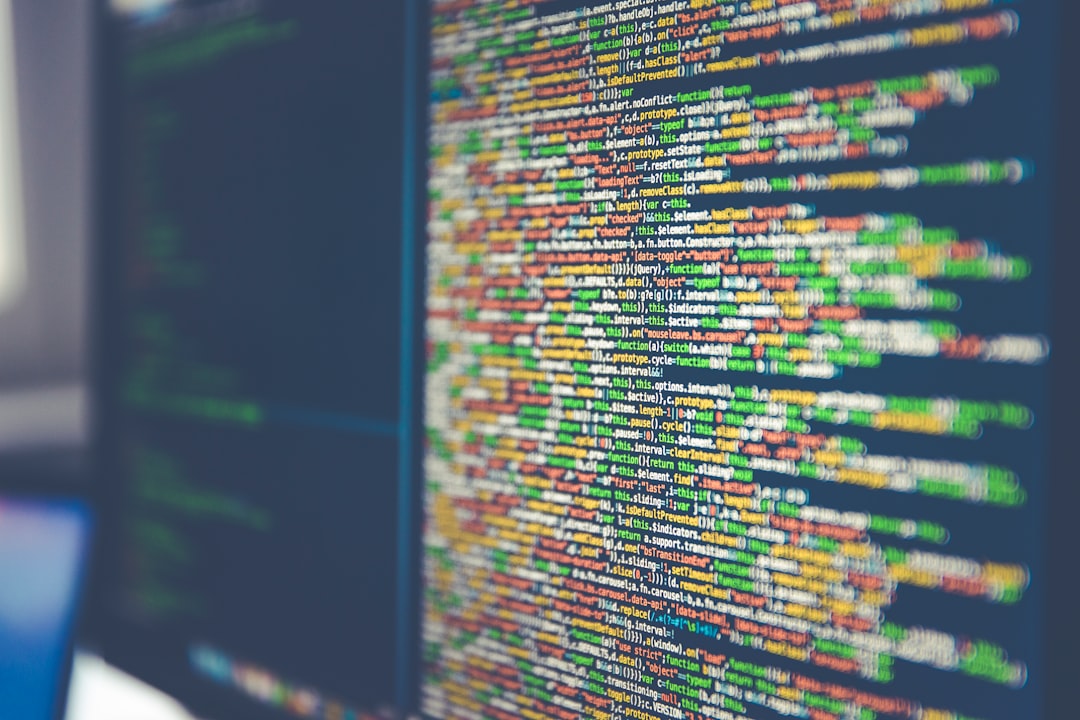
One of the core hurdles in automatically translating English to Urdu is the stark difference in their grammatical structures. English typically follows a Subject-Verb-Object (SVO) pattern, while Urdu often employs a Subject-Object-Verb (SOV) order. This fundamental difference can cause major problems if not handled with care, leading to translations that are inaccurate and potentially confusing. To produce effective translations, machine translation systems need to be sophisticated enough to parse the grammatical components of both languages, recognizing key elements like nouns and verbs in the input and adapting accordingly.
Though current AI systems demonstrate improved translation accuracy, they continue to face difficulties in capturing the wider cultural implications woven into the fabric of language. This makes achieving truly effective translations a challenge. To move towards more accurate and nuanced results, there is a clear need for a deeper, more detailed understanding of the intricate grammatical structures of both English and Urdu. Only then can the systems truly bridge the gap and deliver translations that are not just grammatically correct but also maintain the intended meaning and context.
One of the primary roadblocks in automated English to Urdu translation is the stark difference in how each language constructs sentences. English predominantly follows a Subject-Verb-Object (SVO) pattern, while Urdu often utilizes a Subject-Object-Verb (SOV) order. This variation can lead to errors if translation systems aren't designed to handle this structural shift.
Furthermore, Urdu's flexibility in pronoun usage, allowing for the omission of subject pronouns, presents a unique challenge for systems accustomed to the explicit pronoun requirement of English. This difference can result in misinterpretations if the system isn't trained to recognize and manage this nuance.
The complexity of Urdu verb conjugation adds another layer to the problem. Urdu verbs are modified based on tense, aspect, mood, gender, and number, a system considerably more elaborate than English verb conjugation. This presents difficulties for automated translation systems, which may struggle to accurately translate and maintain the intended meaning.
Beyond verb forms, the use of postpositions in Urdu instead of English prepositions is a fundamental shift in sentence construction. Automated systems that are built to anticipate prepositions before nouns may misinterpret sentences containing postpositions. Coupled with this is the lack of explicit case marking in Urdu. Unlike English, Urdu relies on suffixes to indicate grammatical cases, and if systems aren't adept at identifying these markers, translations might be grammatically inaccurate.
Another intricate aspect lies in the gendered nature of Urdu nouns, which necessitate matching adjective forms in gender and number. Many current AI models haven't been adequately trained for this complexity, resulting in translation errors. Similar challenges appear in forming compound sentences, as Urdu often employs mechanisms beyond simple conjunctions to link clauses. This deviation can lead to awkward or incoherent translations if the system isn't attuned to these alternative methods.
The varied levels of formality and respect embedded in Urdu through verb forms and vocabulary choices, dubbed "honorifics", lack direct equivalents in English. The translation tools need a robust understanding of context to ensure translations don't inadvertently convey an inappropriate level of formality. Similarly, Urdu negation structures often involve unique particles that vary depending on tense and verb form, further complicating accurate translation.
Ultimately, the challenges extend beyond pure grammatical rules. The deeper cultural context woven into the grammatical fabric of Urdu creates difficulties for AI systems trained primarily on English syntax. Successfully translating Urdu requires a deeper understanding of these cultural implications to avoid translations that are technically correct but culturally insensitive or inappropriate. This pursuit of accurate and culturally nuanced translation, in the end, highlights a crucial frontier in AI research.
7 Key Challenges in Automated English to Urdu Translation A 2024 Analysis - Managing Named Entity Recognition Across Languages
**Managing Named Entity Recognition Across Languages**
Successfully applying Named Entity Recognition (NER) across different languages, especially when dealing with English-Urdu translation, poses a significant obstacle. Urdu's intricate structure and rich morphology create difficulties in building effective NER systems. As a result, Urdu NER heavily depends on manual methods, while the potential of more automated, deep learning-based techniques remains largely untapped. The current state of research in Urdu NER is still developing compared to the research available for other languages, highlighting a gap in readily available and scalable solutions. This is a noteworthy issue because accurate identification of entities like individuals, companies, and places is vital for applications like information retrieval and question-answering systems. Future advancements in NER for Urdu will require a greater focus on advanced machine learning approaches. This will allow the systems to adapt more effectively to the unique linguistic features and cultural context that define the Urdu language, thus improving performance and overall accuracy.
1. Recognizing named entities like people, places, and organizations across languages is difficult due to varying cultural relevance. A name well-known in one culture might be obscure in another, adding complexity to translation systems that aim to understand context.
2. The inherent ambiguity of some names poses a challenge. A single name might refer to different entities in different languages or contexts, potentially leading to errors in automated translation.
3. The intricate nature of Urdu, with its diverse inflectional forms, adds another layer of difficulty for NER systems. Proper nouns in Urdu can change form depending on grammar, quantity, and gender, making entity identification more complex.
4. NER models primarily trained on English data struggle to handle Urdu well. This highlights the need for multilingual training datasets that provide a balanced representation of all the languages being targeted. This will help improve the ability to identify named entities.
5. Transliteration challenges arise when names written in one alphabet (e.g., Latin) need to be converted into another (like Arabic script in Urdu). This process often leads to multiple acceptable forms of the same name, introducing a level of ambiguity for the system to deal with.
6. The shortage of standardized Urdu datasets is a significant hurdle. While English has vast amounts of data for training NER models, Urdu translations and the categorization of entities remain under-researched, hindering progress.
7. Many NER systems leverage context to figure out the correct type of entity. However, the limitations in handling multiple languages mean these systems might not accurately infer meaning from the context when dealing with culturally unique names and terminology.
8. Language-specific slang and informal usages introduce unique names and phrases that can confuse NER systems not specifically trained on these variations. This results in incomplete or inaccurate entity recognition.
9. Gender distinctions in Urdu also pose problems. Many names have different forms depending on gender, which can affect how entities are classified and translated.
10. Existing NER systems must also handle documents containing mixed languages, which is particularly challenging. For example, names and phrases from English embedded in Urdu sentences could lead to misclassification if the system isn't designed to specifically manage such situations.
7 Key Challenges in Automated English to Urdu Translation A 2024 Analysis - Dealing with Urdu Script and Diacritical Marks
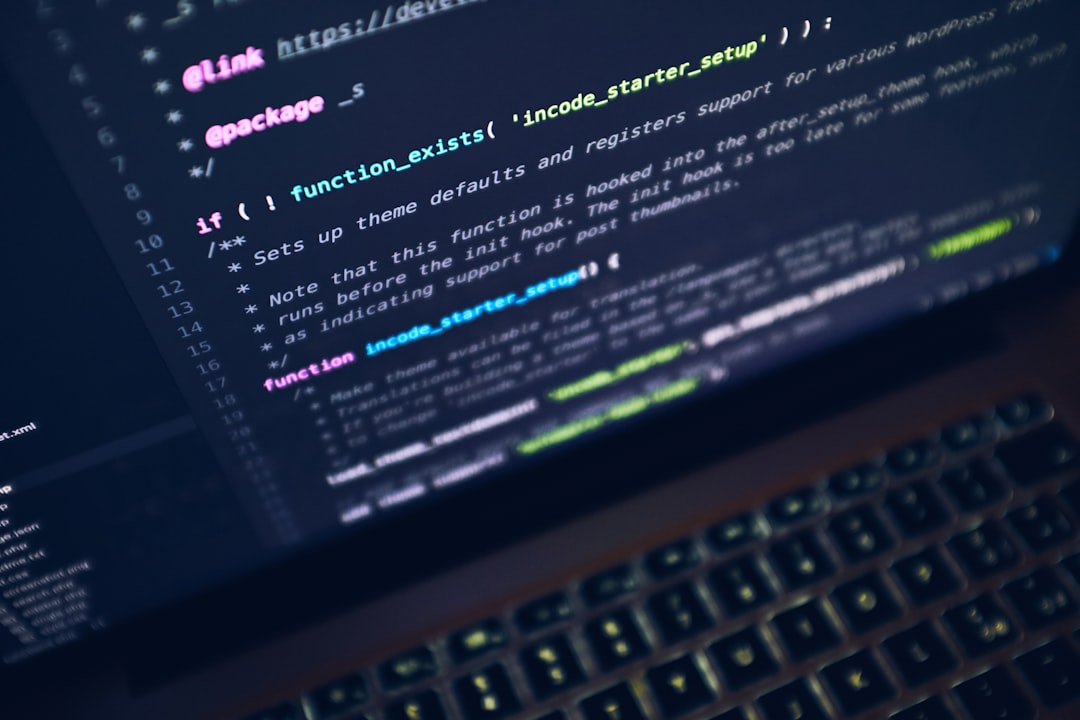
Automating English to Urdu translation faces a significant hurdle in handling the Urdu script and its diacritical marks. Urdu uses the Nastaliq script, a visually distinct writing system derived from Perso-Arabic, which differs considerably from the Latin script used in English. This difference poses a challenge for automated systems designed for languages like English, as they need to be adapted to process the unique structure of Nastaliq.
Adding to this complexity are diacritical marks, small additions to letters that denote vowel sounds and subtly alter pronunciation. These marks, like Zabar, Zair, and Paish, are essential for conveying precise meaning in Urdu, yet are frequently omitted in informal writing. This omission creates ambiguity, particularly for those unfamiliar with the language, making it challenging to discern the correct pronunciation and interpretation of words. The impact of this ambiguity is even more pronounced for those learning Urdu, who might struggle with proper word recognition and understanding.
While neural machine translation has made strides in other languages, Urdu remains a low-resource language in this context. This means there's a relative lack of readily available digital resources, especially high-quality training data. Without sufficient datasets, it's harder for translation systems to learn the intricate nuances of the Urdu language, including the proper application and interpretation of its unique script and diacritical marks. This further highlights the need for more specialized research and development to improve the performance and accuracy of AI-powered Urdu translation tools.
Urdu, written in a modified version of the Arabic alphabet with 38 letters, including some representing sounds not found in Arabic, poses a unique challenge for automated translation systems typically trained on other scripts. This adaptation requires specialized handling to ensure accurate processing.
Diacritical marks in Urdu, such as Zabar, Zer, and Pesh, are essential for conveying vowel sounds and subtle differences in meaning. Their absence can lead to ambiguity, as a single word can have multiple interpretations depending on how it's pronounced. This presents a significant hurdle for automated translation systems that are not specifically trained to recognize and process this aspect of the language.
The right-to-left nature of Urdu script adds complexity for machine learning models traditionally designed for left-to-right languages. Careful handling is needed to prevent errors in text rendering and proper alignment.
Furthermore, Urdu's use of the Nasta'liq script, a cursive style that alters letter shapes depending on their position within a word, adds another layer of complexity. This morphological variability can confuse systems without robust training specifically focused on this script style.
Unlike English, where vowel indications are usually consistent, Urdu allows for vowel omissions, leading to context-dependent interpretation. This reliance on context increases the computational burden for translation systems, which need to be capable of discerning the nuances that drive meaning.
The significance of diacritics in Urdu is illustrated by the potential for major misinterpretations when they are absent. For example, the word "بندہ" (bandah, meaning 'man') could be misread as "بند" (band, meaning 'closed') without proper diacritics, highlighting the critical role they play in accurate interpretation.
Although advanced Urdu keyboards allow for the easy input of diacritical marks, automated systems often fail to consider the variations resulting from human typing errors, which can lead to increased mistranslations.
Grapheme-to-phoneme conversion for Urdu proves especially tricky due to the fluidity in representing phonetically similar sounds without diacritical marks. This can result in systematic errors during pronunciation transcription within automated tools, as the systems may not accurately account for all the possibilities.
Historically, Urdu has undergone shifts in script due to sociopolitical events. This has led to varying orthographic styles, which machine translation models need to be capable of recognizing and accommodating for effective translation.
Finally, the challenges posed by diacritical marks in machine translation highlight the need for a more nuanced, semantic understanding of Urdu words. Mistranslations often stem not simply from incorrect vowel placement, but from the failure to grasp the idiomatic connections and deeper meaning of words, which are often essential in conveying the true essence of the language.
7 Key Challenges in Automated English to Urdu Translation A 2024 Analysis - Tackling Low-Resource Challenges for Urdu Language Data
The challenge of leveraging limited resources for Urdu language data is deeply connected to the wider field of automated English-Urdu translation. While Urdu boasts a vast number of speakers, the shortage of high-quality linguistic data significantly hinders the development of effective machine translation systems. Many recent breakthroughs in machine translation, often stemming from deep learning and neural network approaches, struggle to adapt to the intricate features specific to Urdu, including its complex grammatical structure and the demand for uniquely designed models. Furthermore, the dearth of Urdu-specific data has often forced researchers to lean on English-focused resources, which can magnify inaccuracies in translations and lead to a disconnect from the cultural nuances embedded in the language. This predicament underscores the crucial need for more extensive Urdu-centric datasets and the development of specialized methodologies. Overcoming these limitations is key to achieving meaningful improvements in automated translation for the Urdu language.
1. **Data Scarcity Hinders Progress:** Urdu, despite being spoken by a vast population, falls into the category of low-resource languages when it comes to machine translation. The lack of high-quality, parallel English-Urdu datasets significantly restricts the development of accurate and robust translation systems. This shortage makes it hard to train AI models on the specific features and nuances of Urdu.
2. **Complex Word Forms:** Urdu's morphology is intricate. Words can change extensively based on tense, case, and gender, creating a large number of word variations from a single root. This complexity presents a significant challenge for automated systems, as they need to learn how to generalize across these different forms.
3. **Script and Punctuation Challenges:** The Nastaliq script, used to write Urdu, is quite distinct from the Latin script of English. It's also a cursive script, meaning the letter shapes can change based on their position in a word. Furthermore, Urdu punctuation isn't always consistent with English. This creates issues when translating between the two, as algorithms need to be specifically adapted to handle these differences.
4. **Regional Variations:** Urdu, like many languages, has a range of dialects with different pronunciations and vocabulary. Translation systems need to be sensitive to these variations to avoid mistakes that could be linked to region-specific expressions. Handling these different variations accurately remains a significant challenge in developing universal translation tools.
5. **Context is King:** Many Urdu words can have a number of different meanings depending on the context of the sentence. This inherent ambiguity presents a substantial hurdle for translation models. Developing AI that understands context and avoids translating words based solely on their most common definition is key to achieving better accuracy.
6. **Missing Vowels Create Ambiguity:** Short vowels are commonly left out when writing Urdu, leading to words that can be pronounced in several ways without the use of diacritical marks. This poses a challenge for systems trying to translate because they have a hard time identifying the correct pronunciation and meaning.
7. **Cultural Context Matters:** Certain Urdu words are deeply intertwined with the culture and traditions of the Urdu-speaking community. Effective translation isn't just about substituting words with their English equivalents. AI systems need to learn to understand the cultural meaning behind words to avoid misunderstandings or translations that seem inappropriate to speakers of Urdu.
8. **Gender Impacts Grammar:** Urdu grammar has a gender system that affects the form of verbs and adjectives. Translation systems need to be aware of these gender distinctions and translate accordingly to ensure accuracy. Failing to account for this nuance can lead to incorrect and unnatural translations.
9. **A Gap in Educational Resources**: The absence of comprehensive resources designed to teach Urdu to AI models is a significant obstacle to advancement. More materials could help develop data-driven models necessary for the progress of machine learning in Urdu.
10. **Evaluation Methods Need an Upgrade**: Current ways of evaluating translation quality may not adequately reflect the challenges of translating Urdu. This suggests a need for evaluation frameworks that are specifically designed to handle the unique nuances and cultural aspects of Urdu, helping improve the development and assessment of Urdu translation tools.
7 Key Challenges in Automated English to Urdu Translation A 2024 Analysis - Resolving Ambiguity in Context-Dependent Translations
One of the key obstacles in automated English to Urdu translation is handling the ambiguity that arises from context-dependent language. Many words and phrases can have different meanings based on the surrounding text, making it difficult for automated systems to consistently determine the intended interpretation. Although neural machine translation methods, especially those employing transformer models, are getting better at considering context, they often fall short when it comes to the subtleties and cultural aspects that human translators readily understand. This complexity stems from the very nature of language, where ambiguity and cultural references are frequent, creating a difficult task for machines. It highlights the vital role human translators play in ensuring accuracy and ensuring that the translation captures the intended meaning. Moving forward, achieving a more effective translation system will necessitate the development of more sophisticated techniques that can expertly analyze context and cultural nuances, going beyond simple word-for-word replacements to truly understand and replicate the intent behind the original English text.
1. **Context's Role in Translation Errors:** AI translation systems often stumble when faced with words or phrases that have multiple meanings depending on the surrounding text. This happens because they rely on recognizing patterns in their training data, which might not capture the full range of a word's potential meanings.
2. **The Problem of Similar Meanings:** English words can have overlapping meanings that lead to errors in Urdu translation. Take "bank" as an example – it can mean a financial institution or the edge of a river. A translation model needs to be smart enough to figure out which meaning is relevant based on the context.
3. **Language's Historical Roots:** Urdu has a lot of words borrowed from Persian and Arabic. When translating these back to English, it can be tricky because they might not always mean the same thing in the original context. This creates challenges for AI systems trying to get the translation exactly right.
4. **Understanding the Big Picture:** Machine translation often struggles with maintaining a coherent meaning across a whole paragraph or text. While a sentence might be translated correctly on its own, the overall message can get lost when you look at it in the context of the other sentences.
5. **The Importance of Cultural Understanding:** Many Urdu idioms are closely connected to Urdu culture and don't have easy translations in English. Just substituting words doesn't always work; the translation needs to capture the cultural meaning and feeling of the idiom to be truly effective.
6. **Sorting Out Different Meanings:** A major issue for automated systems is the lack of a strong system for deciding which meaning of a word is the correct one. This leads to translations that miss the mark. AI tools need to be able to figure out which of the several possible meanings is the right one based on the context.
7. **Maintaining the Right Tone:** Translations often need to match a specific tone or level of formality, which can be very different in Urdu compared to English. For automated translations to be effective, they need to be sensitive to these differences in language style.
8. **Recognizing Contextual Clues:** Urdu uses specific markers and particles within sentences to show how different parts are related. If the AI systems don't pick up on these markers, it can cause misinterpretations and lead to confusing translations.
9. **Complex Sentence Structures:** English complex sentences can be broken down into simpler parts for translation. But in Urdu, these structures sometimes have additional meanings that are lost in translation if the AI doesn't understand how Urdu sentences are formed.
10. **User Awareness of Limitations:** People who use automated translation systems might not fully understand the limits of AI when it comes to context. This can lead to misunderstandings or over-reliance on inaccurate translations. We need better ways to educate users about the strengths and weaknesses of these systems.
7 Key Challenges in Automated English to Urdu Translation A 2024 Analysis - Improving Machine Learning Models for Urdu Language Processing
Improving machine learning models specifically for Urdu language processing is essential for advancing automated English-to-Urdu translation. While the transition from older statistical translation methods to modern neural machine translation (NMT) – especially those using deep learning and transformer architectures – shows potential, the fact that Urdu is considered a low-resource language presents distinct hurdles. These challenges are amplified by Urdu's complex grammar, rich vocabulary, and unique script, requiring specialized solutions. Researchers are actively exploring techniques like hybrid models that combine different machine learning approaches and contextual lemmatization using recurrent neural networks (RNNs). The goal is to better handle the intricate features of Urdu and ensure that translations are not only grammatically correct but also retain the subtle cultural aspects of the language. Ultimately, continued research and the development of more sophisticated machine learning methods will be critical to overcome the difficulties inherent in translating from English to Urdu, aiming for translations that accurately convey meaning and resonate with Urdu speakers.
1. **Navigating Urdu's Unique Syntax:** Urdu's grammatical structure often presents a challenge for machine learning models trained on languages with more rigid sentence formations like English. The ability to handle implicit sentence elements, common in Urdu, requires a more flexible approach to parsing and interpreting text.
2. **Handling the Richness of Urdu Morphology:** Urdu's complex morphology, where a single root word can yield a plethora of derivatives through prefixes and suffixes, poses a challenge for model training. Creating models that can generalize from these diverse word forms requires substantial and diverse datasets, which are unfortunately scarce for Urdu.
3. **Capturing Subtle Emotional Nuances:** Urdu expressions often convey a distinct emotional tone or cultural sensitivity related to notions of honor and shame that aren't always directly translatable to English. Simply translating words can lead to a loss of meaning or, even worse, to culturally insensitive renditions, highlighting the need for a deeper understanding of the cultural context.
4. **Resolving Pronoun Ambiguity:** The common practice of omitting subject pronouns in Urdu creates potential ambiguity for AI systems accustomed to English, where explicit subjects are the norm. This necessitates more sophisticated contextual analysis to ensure correct interpretations, especially when inferring the intended actor of a verb.
5. **Dealing with Non-Standardized Language Variations:** Urdu has a wide range of formal and colloquial styles, often without a universally accepted standard for usage. This variability can confuse machine translation systems trained primarily on more standardized datasets, leading to awkward or potentially incomprehensible translations for native speakers.
6. **Mastering the Intricacies of Urdu Verb Conjugation:** The complexity of Urdu verb conjugations, which signify various aspects, moods, and genders, is a hurdle for automated systems. Training models to reliably handle this vast array of forms requires significant effort and robust, well-annotated data that is currently lacking.
7. **Adapting to Script Variations:** Urdu script often shows subtle variations in orthography and letter forms across different regions and cultural contexts. Developing translation systems that can accurately process these variations, which can be tied to influences from other languages and historical factors, requires robust training data and sophisticated adaptation methods.
8. **Ensuring Cultural Sensitivity in Translations:** A successful Urdu translation not only captures the syntactic structure of the text but also mirrors the underlying cultural context and intended tone of the source language. Overly literal translations can often lead to lifeless, culturally detached outputs, highlighting the necessity of incorporating cultural knowledge into the translation process.
9. **Overcoming Urdu's Data Scarcity:** A key obstacle in advancing Urdu machine translation is the relatively small amount of machine learning data available compared to more widely studied languages like English. This data scarcity impedes the development of truly robust translation models tailored to the specific linguistic features of Urdu.
10. **Addressing User Expectations and Frustrations:** Users of machine translation tools for Urdu often encounter inconsistencies and limitations due to the language's complexities. The ability of the system to reliably discern between similar-sounding words and phrases that have different meanings in specific contexts or cultural contexts is a frequent point of frustration. This underlines the ongoing need for improvements in translation accuracy and clarity, as well as better user education regarding the current capabilities and limitations of these tools.
Experience error-free AI audio transcription that's faster and cheaper than human transcription and includes speaker recognition by default! (Get started for free)
More Posts from transcribethis.io: