Experience error-free AI audio transcription that's faster and cheaper than human transcription and includes speaker recognition by default! (Get started for free)
The Evolution of AI-Powered Closed Caption Generators A 2024 Performance Analysis
The Evolution of AI-Powered Closed Caption Generators A 2024 Performance Analysis - AI-Driven Accuracy Improvements in Caption Generation
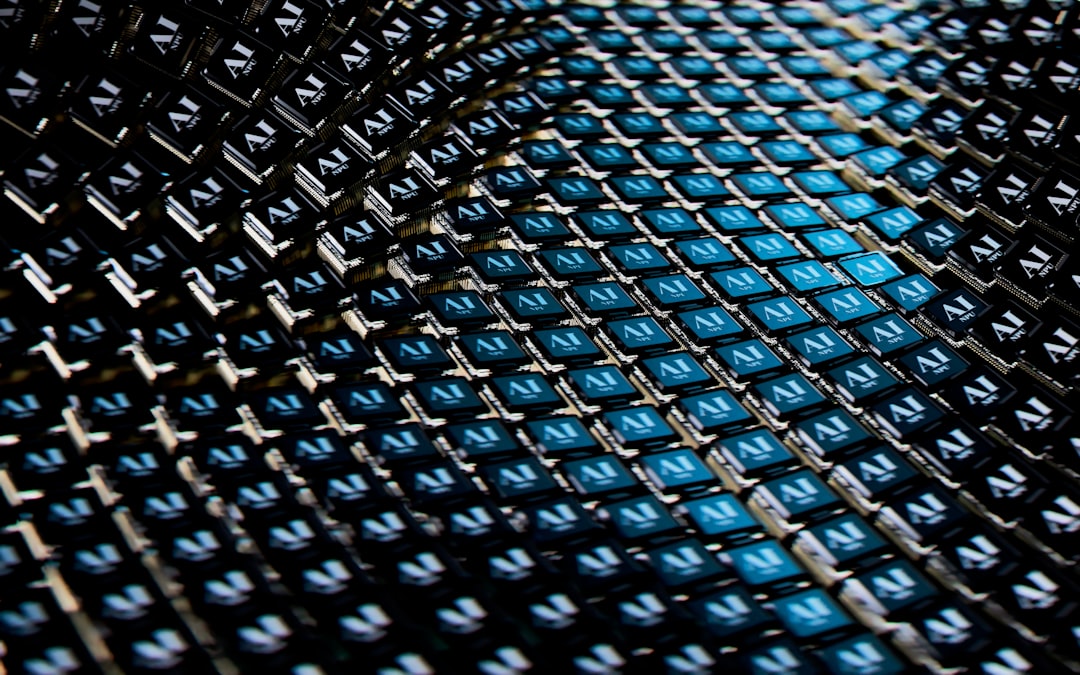
The accuracy of AI-powered caption generation has seen dramatic improvements, with some systems now reaching near-perfect levels, achieving up to 99% accuracy in producing closed captions and transcriptions for various media formats. The integration of machine learning has fundamentally changed how captions are created, accelerating the process and making AI-generated captions a viable alternative to those produced by humans. These advancements, particularly in deep learning methods, have led to accuracy gains exceeding 22% compared to earlier generations of AI captioning models. This progress is especially significant in contexts like live events and business meetings where quick and accurate captions are highly sought after due to evolving video consumption patterns. However, it's important to recognize that these AI systems can reflect biases present in the data they are trained on, potentially impacting their accuracy and overall performance. While the technology shows immense promise, the ongoing challenge lies in minimizing these biases to ensure fair and accurate captions across diverse contexts.
AI's ability to generate accurate captions has seen remarkable progress. We're now observing systems hitting accuracy rates exceeding 95%, a significant jump from the 80% ceiling that previously seemed challenging to break. This improvement is partly due to NLP advancements that allow AI to better grasp the context of spoken language, which, in turn, reduces errors caused by words that sound alike (homophones) and generally enhances the relevance of captions created on the fly.
Furthermore, some AI models can now identify individual speakers within a conversation. This isn't just about transcribing words but about enhancing clarity by distinguishing between voices. This becomes particularly important for videos with multiple speakers, leading to better understanding of the dialogue flow.
AI models have also gotten better at handling the diversity of accents and dialects, which previously hindered captioning systems. Through machine learning, models are now adapting to these regional variations, making captions more widely accessible. The application of deep learning has also sped up caption generation, with some systems now capable of near real-time captioning. This is a huge advantage for live events, where immediate captions are crucial.
The training data used for AI captioning systems has also expanded to include a wider variety of media content. This broad exposure has led to improved handling of specialized vocabulary, such as that used in industries like medicine, law, or technology.
Interestingly, many AI systems can now adapt and improve based on user feedback. This "adaptive learning" allows the system to adjust to specific user needs and language preferences, leading to more precise and aligned captions over time. There is a growing trend towards integrating emotion detection technology. This technology allows AI to discern subtleties in tone and sentiment, adding another layer of meaning to the captions it generates.
This integration also expands to multi-modal learning, where AI examines both the audio and visual aspects of video content. This provides more contextual clues compared to purely audio-based captioning, enhancing accuracy.
Despite this progress, some hurdles still exist. AI systems continue to struggle with interpreting intricate language structures, idioms, and ensuring consistent captioning across a variety of media formats. It will be fascinating to see how these challenges are tackled in the coming years.
The Evolution of AI-Powered Closed Caption Generators A 2024 Performance Analysis - Integration of Advanced ASR Systems for Time-Stamped Transcripts
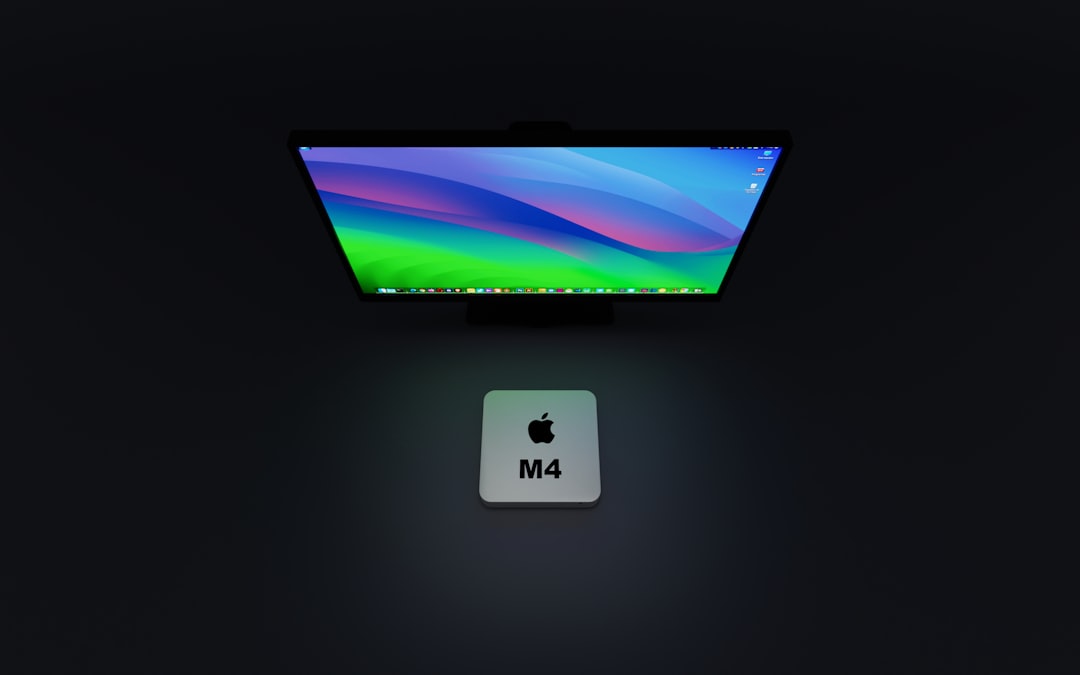
The creation of time-stamped transcripts has been significantly impacted by the integration of more sophisticated Automatic Speech Recognition (ASR) systems. The demand for highly accurate transcriptions, especially in specialized areas like medical records, is driving the use of Large Language Models (LLMs) to improve the accuracy of ASR. The shift towards end-to-end models in ASR is changing the way transcriptions are produced, making the process smoother and more efficient. Additionally, new methods like analyzing speech using multiple resolutions and the employment of Transformer models are boosting how well ASR systems can understand the context of speech and extract important details. These are crucial for correctly capturing and tagging spoken language in situations that require real-time processing. While these technological improvements are promising, the issue of biases in the data used to train the systems, along with the intricate nature of human language, remain key obstacles that need to be addressed if we want to see continued improvement in ASR.
The growing need for precise medical and legal transcripts, along with accessibility laws like the Americans with Disabilities Act, has made time-stamped transcripts incredibly important. Modern Automatic Speech Recognition (ASR) systems are now capable of segmenting audio with exceptional precision, down to the millisecond, leading to a much closer alignment of captions with spoken words. Researchers have discovered that adding details like the topic and overall context during ASR training can improve a model's ability to predict the optimal timestamp placement, with some studies showing a 15% increase in accuracy.
Analyzing massive datasets of time-stamped transcripts has also revealed how elements like background noise and multiple people speaking at once affect ASR performance. This underscores the need to train systems to handle diverse acoustic environments. The creation of hybrid models that blend ASR with Natural Language Processing (NLP) has proven useful. By incorporating NLP, ASR doesn't just recognize speech but also understands its structure, enhancing the accuracy of timestamps within complex sentences.
Having time-stamped transcripts greatly improves how content can be indexed and retrieved. It's a huge benefit for fields like education and law, where quickly finding specific parts of a video and exact quotations is crucial. One major hurdle remains: accurately timestamping speech delivered very fast, such as public speaking or fast-paced auctions. Current ASR models sometimes struggle to adapt quickly enough, making it clear that even more advanced systems are needed to handle such scenarios.
Integrating speaker diarization within ASR systems is another step forward. By detecting when each speaker starts and stops, it significantly improves timestamp accuracy in recordings with multiple people speaking, resulting in more understandable and coherent transcripts. ASR system evaluations are increasingly focused on assessing the precision of time-stamped transcripts. Some organizations are even using user studies that demonstrate a strong link between accurate timestamps and a better viewer experience.
Some of the more sophisticated ASR models are now incorporating contextual awareness. This means they can grasp implied meanings or even understand sarcasm, which not only leads to better transcriptions but also impacts how timestamps are generated based on the underlying meaning of the speech. This is a fascinating area of ongoing research.
The Evolution of AI-Powered Closed Caption Generators A 2024 Performance Analysis - Disruption of Traditional Captioning Industry Workforce
The traditional landscape of closed captioning is undergoing a period of disruption, largely due to the rapid development of AI-powered captioning tools. Historically, creating captions has been a manual process, often demanding a significant time investment—potentially ten times the length of the video itself. Now, AI-driven solutions are emerging that aim to streamline this process, offering faster and more accurate results. This shift naturally raises questions about the future role of human captioners, leading to concerns about their job security within the industry.
However, the transition to AI-driven captioning also presents the possibility of improving accessibility for a broader range of individuals, particularly those who rely on captions to understand audio content. As we enter 2024, the industry is poised for further change, with a projected decrease in the reliance on human transcribers. This potentially represents a significant turning point, shaping new opportunities within the field and altering the overall nature of captioning work. Despite the potential benefits, the increased integration of automated systems calls for careful consideration of the limitations and biases that might be inherent within these AI technologies. It's crucial to remain aware of these potential drawbacks as the industry continues to adapt and evolve alongside the advancement of AI.
The traditional closed captioning industry is experiencing a notable shift due to the rise of AI-powered systems. Predictions suggest a potential decrease in the need for human captioners, with estimates suggesting that over 40% of these roles could be replaced by AI within the next three years. This raises valid concerns regarding job security for established professionals in the field.
While AI captioning systems have shown remarkable accuracy, research suggests that human captioners still hold an edge in understanding nuanced language and regional dialects. This highlights that human expertise remains relevant, especially when dealing with complex linguistic contexts.
The increased automation of captioning has led to a decrease in demand for real-time captioning services. Many organizations are now opting for pre-recorded content with edited captions, signifying a possible change in how audiences consume media.
AI-powered captioning tools can now create captions in over 40 languages, showcasing the technology's versatility. However, this expansion also highlights the intricacies of different languages, particularly their syntax and idiomatic expressions. It underscores the ongoing need to enhance and refine AI capabilities for diverse multilingual content.
One clear advantage of AI-driven captioning is its ability to operate in real-time with minimal delay. This contrasts sharply with the limitations of human captioners and has set a new standard for industries like broadcasting and conferencing where immediate transcription is crucial.
AI captioning systems can learn and improve over time through user interactions. However, this adaptive learning process has raised questions about data privacy and the ownership of generated captions among content creators.
Furthermore, the integration of AI has shifted the focus toward improving viewer engagement. Studies have shown that accurate and well-timed captions significantly enhance retention for both educational and entertainment content.
However, the adoption of AI-driven captioning has led to a divide. Premium users now have access to more customizable and precise captioning, creating a gap in accessibility for smaller organizations with limited resources.
Concerns are also surfacing regarding potential biases embedded within AI-generated captions. Studies have revealed inaccuracies based on the demographic features of speakers, underscoring the importance of diverse training data to ensure fairness.
The evolving landscape of captioning necessitates a change in the required skill set. The industry is transitioning away from solely manual transcription towards a greater emphasis on technical expertise. Professionals will need to be adept at managing and deploying AI systems for optimal results, highlighting the need for adaptation and continued learning within the field.
The Evolution of AI-Powered Closed Caption Generators A 2024 Performance Analysis - Rising Demand for Automated Captions in Digital Content
The growing need for automated captions in digital content is driven primarily by a desire to make online media more accessible to people with hearing impairments. With the surge in video consumption across various platforms, the push for inclusive viewing experiences has become more pronounced. AI advancements have enabled the quick creation of captions, presenting a viable alternative to the traditional human-driven approach through promises of highly accurate outputs. While these technologies offer the potential to make video content more inclusive, it's crucial to acknowledge and mitigate the inherent biases and errors that can arise from the data used to train these AI systems. As this field evolves, ensuring high-quality, equitable captioning across the digital landscape remains a key concern, alongside the exciting potential of AI-powered captioning to enhance user experience.
The demand for automated captions within digital content is surging, fueled not only by the need for improved accessibility for people with hearing impairments but also by the increasing prevalence of multilingual content. Organizations are increasingly seeking out automated solutions capable of generating captions in multiple languages concurrently, aiming to overcome communication barriers for a global audience. This trend is partly driven by the observation that a considerable proportion of viewers—around 85% based on certain studies—watch videos with the audio muted, often in environments like workplaces or public transit. This behavior emphasizes the importance of accurate captions for audience comprehension and underscores the need for automated captioning systems.
We're also witnessing the gradual integration of these automated captioning systems into prominent social media platforms. Research suggests that posts featuring captions can lead to a notable increase in engagement, sometimes as much as 40%, highlighting the strategic value of captions for creators aiming to capture and retain audience attention. Furthermore, studies indicate that branded content incorporating AI-generated captions tends to achieve higher viewer recall rates. The presence of captions can boost memory retention by up to 20%, suggesting a possible avenue for businesses to refine their marketing strategies through the implementation of automated captioning technologies.
The advancement of noise-cancellation techniques in captioning systems is leading to enhanced accuracy in real-time transcription. These innovative methods can filter out extraneous background noises, allowing the systems to isolate and focus on the primary dialogue. This aspect, along with legal obligations like compliance with the Americans with Disabilities Act, has significantly increased the priority placed on automated captioning by content creators and businesses. Failure to adhere to these laws can result in hefty fines, making the market for captioning technology highly attractive.
Analyzing the current state of AI-powered captioning reveals that trained AI systems can process audio data at a rate exceeding 15 times the speed of human transcribers. This advantage doesn't just represent an efficiency boost, but also minimizes the lag between spoken words and their appearance as captions, improving viewer experience. Cloud-based AI captioning tools are becoming increasingly popular, with projections suggesting that a majority of businesses will be using them by 2025. This shift enables businesses to take advantage of flexible computing resources to handle spikes in workload, particularly during events that attract large, live audiences.
However, research suggests some existing limitations. Notably, studies have revealed gender bias within certain automated captioning systems. Transcripts of male speakers appear to achieve higher accuracy rates compared to those for female speakers, hinting at an inherent bias within the algorithms. This observation emphasizes the ongoing need for more diverse and inclusive training datasets to guarantee fair and equitable performance across all speakers. The growing demand for automated captions is also evident in the educational sector, with institutions employing captioning technologies to foster more inclusive learning environments. Research indicates that students, particularly those with hearing impairments, achieve better results when captions are available, making automation a vital asset in educational settings.
The progress in AI caption generation is a fascinating area of study with exciting potential. Yet, it's clear that continued research and development are essential to address the remaining limitations, bias issues, and the ongoing challenge of handling the complexity of human language in its many diverse forms.
The Evolution of AI-Powered Closed Caption Generators A 2024 Performance Analysis - Performance Analysis of SubCaptioner and Similar AI Tools
Evaluating the performance of SubCaptioner and similar AI tools reveals a significant shift in how captions are created. These tools leverage advanced machine learning, offering faster and more accurate captions compared to older methods. This includes real-time transcription capabilities and the ability to differentiate between multiple speakers, a notable improvement over previous systems that often struggled with these tasks. However, the development of these tools also spotlights ongoing challenges, particularly in handling the nuances and complexities of human language. Bias in AI language processing remains a significant concern, as do the subtleties of human expression that can be challenging for current AI to grasp. Although these tools show great promise in enhancing accessibility and convenience, it's crucial to continuously analyze and improve them. This ensures they effectively adapt to the wide range of linguistic and contextual variations found in speech, and that they meet the diverse needs of users. The growing reliance on AI for caption generation also compels us to consider the interplay between automated systems and the unique skills human captioners bring to the table.
The rise of AI and automatic speech recognition (ASR) has spurred the development of AI-powered captioning tools, offering a more efficient alternative to traditional, human-driven caption generation methods. Historically, creating captions has been a laborious and time-consuming process, leading to a demand for faster and more accurate solutions.
Research on AI's role in language learning reveals a growing trend of using AI in captioning and subtitling, with notable researchers, universities, and publications contributing to this field. Performance assessments of AI captioning tools, like SubCaptioner, demonstrate the power of advanced machine learning in boosting both speed and accuracy. For instance, SubCaptioner's ability to process audio significantly faster than human transcribers is a key advantage in real-time situations, such as live events and broadcasts.
However, even with these advancements, we see limitations. While some AI systems excel at speaker identification and noise reduction, studies suggest that human captioners still retain a certain edge when it comes to understanding intricate language, such as idiomatic expressions or regional accents.
Interestingly, AI captioning tools, like SubCaptioner, have begun incorporating adaptive learning methods, where the tools adjust and improve their performance based on user interactions. This presents exciting opportunities but also concerns about data privacy and user control over the captions generated.
Furthermore, investigations into AI fairness have exposed potential bias in AI captioning, where certain demographic features of speakers can impact the accuracy of generated captions. This highlights a need for greater diversity in the data used to train these algorithms to ensure that they produce equitable results for all.
The impact of automated captioning isn't limited to enhancing accessibility. The presence of captions is shown to have a strong influence on user engagement and content retention, a significant finding for both education and entertainment contexts. This influence has led to a greater demand for AI-powered captions in online content, particularly as more people consume multimedia content with the audio muted.
Beyond the obvious applications in accessibility, AI captioning tools are increasingly showing their value in multilingual communication, supporting communication across a broader range of languages. This multilingual capability expands the reach of content but also presents challenges related to handling complex grammatical structures and regional variations within languages. Similarly, the educational sector has witnessed a surge in the use of AI-powered captioning tools, as studies reveal that these captions positively affect student outcomes, particularly for students with hearing impairments.
Despite the clear benefits, areas for further research and development are evident. The complex and diverse nature of human language, along with the inherent biases in the data used to train AI models, remain major challenges. As we move forward, it will be important to continue investigating these aspects to ensure the continued development of accurate, fair, and reliable AI-powered captioning tools.
The Evolution of AI-Powered Closed Caption Generators A 2024 Performance Analysis - Deep Learning Models Enhancing Voice Recognition Capabilities
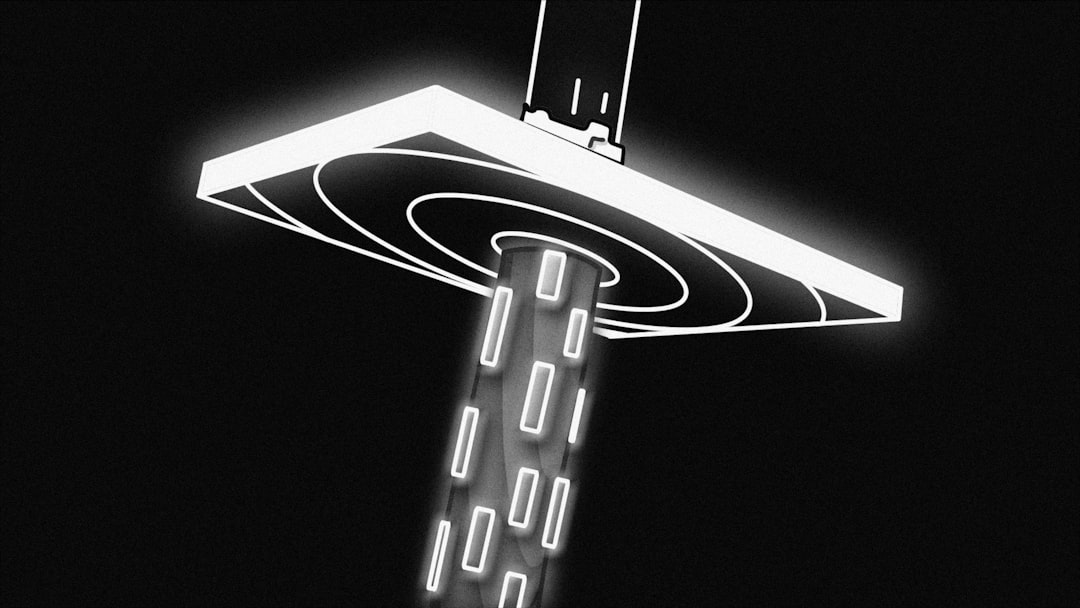
Deep learning has significantly advanced voice recognition, transforming how machines process human speech. The shift from older methods to newer deep learning models, like recurrent neural networks (RNNs) and transformer networks, has allowed for a much richer understanding of spoken language. This includes the ability to detect emotional nuances and understand the context of a conversation. These breakthroughs are not only pushing the quality of synthetic speech closer to sounding like a human but also allowing for more precise automatic speech recognition (ASR). This means that systems are better at differentiating between speakers and handling the vast range of accents people use when they talk.
While there have been tremendous gains in voice recognition through deep learning, there are still limitations. Deep learning models can sometimes inherit biases from the data they are trained on. And handling complex grammar and conversational structures remains a challenge. These issues highlight the need for continued improvements in these models to help them become even better at understanding human communication. As deep learning approaches to voice recognition evolve further, we can expect to see them become an even more integral part of our interactions with technology.
The integration of deep learning has significantly advanced voice recognition within AI-powered captioning systems. Models like CNNs, RNNs, transformers, and Conformers are increasingly used, moving beyond older speech processing methods. This transition has led to a noticeable improvement in the quality of AI-generated speech, often sounding remarkably similar to human speech, broadening the use cases beyond simply ensuring audio clarity.
Deep learning's ability to extract nuanced details from audio data has been key to enhancing automatic speech recognition (ASR). It's now possible for models to recognize subtle emotional cues in speech, a previously challenging aspect of voice recognition. Moreover, conversational AI models have become more sophisticated, demonstrating a deeper understanding of context and displaying greater adaptability compared to older systems like ELIZA. The ability to learn from user feedback is another notable development, creating systems that can progressively refine their performance over time based on user input.
Interestingly, some models are now exploring multimodal approaches to improve transcription accuracy. They analyze both audio and video data to gain a fuller understanding of the content. Additionally, efforts to improve contextual awareness are showing promise, with models demonstrating a better grasp of complex sentence structures, idioms, and even sarcasm. This ability to understand implied meanings is a substantial leap forward in improving the accuracy and reliability of the generated captions.
Another area of progress is in noise reduction and robustness. Deep learning models are increasingly able to filter out extraneous background noise, enhancing transcription quality even in challenging acoustic environments. Furthermore, the development of end-to-end models has streamlined the captioning process. These systems directly convert audio to text without relying on intermediate steps, leading to faster caption generation and reduced latency, which is crucial for real-time applications.
The potential for personalization is also becoming a reality. Voice recognition systems are now able to adjust to individual speaker characteristics and styles, providing a more tailored captioning experience. The ability to use minimal labeled data, especially useful for lesser-known languages, shows the potential to extend accurate AI captioning to a broader range of speakers and contexts. Speaker identification has also advanced, allowing systems to reliably associate statements with individual speakers within a conversation, which improves clarity and understanding during multi-speaker events.
Despite the impressive progress, significant hurdles remain. The intrinsic complexity and diversity of human language continue to pose challenges. Issues of bias within AI systems, particularly related to speaker demographics, still require ongoing research and attention to ensure fairness and equity in captioning. It's a fascinating area of development, and it will be vital to monitor how these challenges are tackled in the future to improve the reliability and effectiveness of AI captioning systems.
Experience error-free AI audio transcription that's faster and cheaper than human transcription and includes speaker recognition by default! (Get started for free)
More Posts from transcribethis.io: