Experience error-free AI audio transcription that's faster and cheaper than human transcription and includes speaker recognition by default! (Get started for free)
7 Critical Audio Quality Factors That Impact AI Transcription Accuracy in 2024
7 Critical Audio Quality Factors That Impact AI Transcription Accuracy in 2024 - Background Noise Reduction Through Digital Signal Processing
Minimizing background noise is fundamental for achieving high-quality audio and maximizing the accuracy of AI transcription. Digital Signal Processing (DSP) is a core tool used to accomplish this, applying both initial signal adjustments and later processing methods to manage unwanted sounds. Older noise reduction techniques face challenges, particularly in scenarios where the noise changes frequently and mixes with the desired audio. These limitations often result in distortions when trying to clean up the sound. In contrast, newer methods based on deep learning are showing encouraging results in improving speech quality, although they come with limitations, including the need for more real-time processing capabilities and the difficulty of fully understanding how these AI models reach their conclusions. Looking ahead, breakthroughs in DSP seem promising as it becomes possible to refine both recording equipment and refine the algorithms used to isolate and reduce noise. These dual advancements are key to ensuring that AI-powered transcription systems can reliably capture clean audio inputs, ultimately leading to more reliable results in the complex audio environments we experience today.
Cleaning up audio from unwanted sounds is a crucial step in improving the quality of recordings and, in turn, the accuracy of AI transcriptions. Digital Signal Processing (DSP) plays a key role in achieving this goal by employing clever algorithms that either prepare the audio before recording or clean it up afterwards. One common approach is adaptive filtering, which constantly adjusts itself to match the noise characteristics, thus enhancing the desired audio. The impact can be substantial, with DSP algorithms potentially reducing noise by 30 decibels. This makes them especially useful in places where background noise is a constant challenge, like busy public spaces.
Some advanced techniques go further by using machine learning to categorize different sounds. This allows them to selectively filter out undesirable noises while preserving the essential aspects of the target audio. A popular method in DSP is spectral subtraction, where the system creates a "map" of the noise and removes it from the audio signal, effectively improving speech clarity. DSP aims to mimic the "cocktail party effect", the way our brains filter out distractions in conversations. By concentrating on the desired audio and attenuating the interfering sounds, DSP can create a similar effect in digital audio.
Several DSP algorithms can achieve nearly instant noise reduction, with delays under 20 milliseconds. This feature is vital in applications that need immediate responses, such as live streaming or interactive voice communication. However, if the background noise stays consistent, as with equipment like fans, DSP systems can develop a 'noise profile', allowing for more targeted and efficient removal.
While the goal is to create a cleaner recording, it's also critical to ensure the remaining audio doesn't sound unnatural or distorted. Overly aggressive noise reduction can lead to audio artifacts that detract from the listening experience. Striking the right balance is crucial - suppressing unwanted sounds while ensuring the remaining audio retains its dynamic range and avoids excessive processing.
Furthermore, DSP techniques employing multiple microphones can leverage spatial filtering. By analyzing the location of different sounds, these systems enhance desired audio while silencing noise from other directions. This sophisticated approach allows for more precise control over the sound environment, enhancing overall audio quality. This constant quest for improved noise reduction underscores the ever-evolving nature of the field and its role in paving the way for more accurate AI transcription in the future.
7 Critical Audio Quality Factors That Impact AI Transcription Accuracy in 2024 - Microphone Quality and Source Distance Impact on Voice Recognition
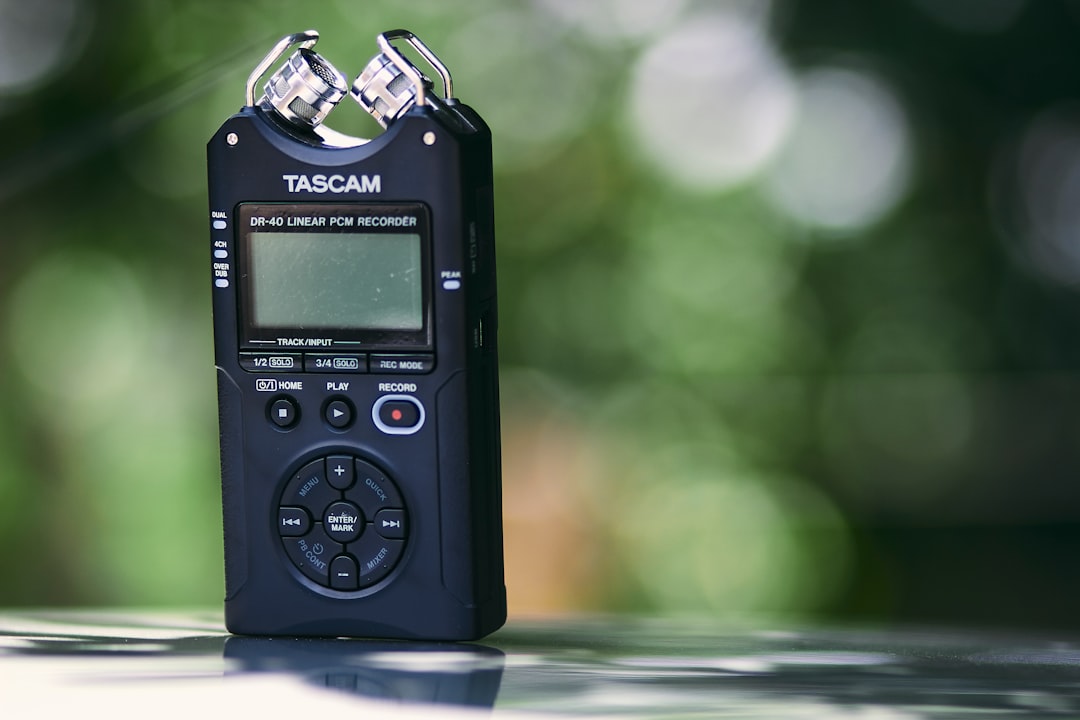
The quality of the microphone and the distance between the speaker and the microphone are crucial factors impacting how well voice recognition systems perform. A microphone's ability to capture clear audio is paramount for accurate speech recognition and transcription. Higher-quality microphones typically have a better signal-to-noise ratio, capturing audio more cleanly. Conversely, if the speaker is too far from the microphone, the captured audio will likely be quieter and potentially less clear, making it more difficult for the AI to process accurately, increasing the likelihood of errors. Further complicating matters, the presence of background noise adds another layer of challenge, as these extraneous sounds can interfere with the desired audio, making it harder for the AI to decipher spoken words. This challenge highlights the importance of optimizing both the recording equipment and the recording environment to minimize the impact of noise and ensure optimal audio quality for the AI transcription process. As voice recognition technologies continue to develop, paying close attention to these audio input factors will be essential for achieving the goal of even more accurate transcriptions.
The quality of a microphone and the distance between it and the speaker can significantly impact the accuracy of voice recognition systems. Different microphone types, like condenser vs. dynamic, have varying sensitivities and capture different levels of detail, which can become more pronounced as the distance between the source and the microphone increases.
Distance itself plays a crucial role in determining the strength of the audio signal relative to any background noise. As the distance between the speaker and microphone doubles, the sound intensity decreases by roughly 6 decibels, potentially affecting the signal-to-noise ratio (SNR) and making it more challenging for the AI to accurately process the speech.
Another interesting effect, the proximity effect, causes microphones to emphasize bass frequencies when the speaker is close. This can cause issues if the AI model isn't designed to handle such audio characteristics, potentially leading to misinterpretations of deeper voices compared to recordings taken from further away.
The directional characteristics of microphones are also affected by distance. Omnidirectional mics pick up sound from all directions, which can lead to an increase in unwanted background noise at larger distances, reducing the clarity of the intended audio. On the other hand, directional mics can focus on the speaker, improving the SNR, but require careful positioning.
As the distance between the microphone and speaker increases, the sound waves bounce off surfaces in the environment, potentially creating distortions due to phase cancellation. These distortions can interfere with the AI model's ability to correctly process the audio signal, leading to inaccuracies in the transcription.
Furthermore, while high-quality microphones can capture a wide range of frequencies, many AI transcription systems are primarily optimized for a narrower band of audio, typically between 4 kHz and 8 kHz. This means that some subtle details present in distant recordings, which might contain higher frequencies, could be lost during the transcription process, impacting overall accuracy.
Microphones with large diaphragms generally excel at capturing low-frequency sounds but can struggle with capturing distant high-frequency sounds, impacting the perceived clarity of the voice. This can lead to misinterpretations by the AI, potentially impacting the accuracy of the transcription.
Interestingly, the frequency response of a microphone changes depending on the distance to the audio source. Higher frequencies tend to decrease more rapidly with distance compared to lower frequencies, potentially distorting the perceived voice and, therefore, impacting the transcription accuracy.
Even environmental elements like temperature and humidity can influence sound transmission through the air, affecting how recordings are captured over distance. These alterations in sound can then impact the ability of the AI to accurately transcribe the speech, leading to errors in the transcription process.
Lastly, even the most sophisticated AI transcription systems may encounter challenges when faced with audio signals captured from a longer distance. Muffled or weak audio signals are more difficult for AI models to process, highlighting the importance of proper microphone placement and recording techniques to ensure optimal performance. The ability to mitigate the effects of distance through advanced algorithms is still an area of research and development, but it's clear that optimal mic placement plays a significant role in obtaining the best possible transcription results.
7 Critical Audio Quality Factors That Impact AI Transcription Accuracy in 2024 - Audio Sampling Rate Effects from 8kHz to 48kHz
The audio sampling rate, which essentially determines how often audio is captured, has a considerable influence on the quality of the recording and, as a result, the accuracy of AI transcription. When the sampling rate is lower, such as 8 kHz, which is often used for phone calls due to bandwidth limitations, the audio quality tends to suffer. This can lead to noticeable noise and distortion, impacting the clarity of the sound. In comparison, higher sampling rates, for example, 48 kHz or even higher, offer a more precise representation of the audio's frequency range. This translates to a more accurate capture of the nuances of speech, leading to a better transcription. While higher rates theoretically provide better sound quality, the benefits over the CD standard of 44.1 kHz might be less pronounced for everyday listening experiences due to the limitations of human hearing. The choice of sampling rate, therefore, represents a balance between preserving the details of the audio signal and ensuring it is optimized for the AI to accurately understand and interpret speech for the purpose of accurate transcription. Using too low a sampling rate can hinder the AI's ability to process subtle details of the voice or distinguish between similar-sounding words, which ultimately impacts the accuracy of transcriptions.
The selection of an audio sampling rate plays a crucial role in determining the quality of recorded speech and, consequently, the accuracy of AI transcriptions. While a basic 8 kHz sample rate is sufficient for simple voice communication, it can introduce artifacts like aliasing, where high-frequency sounds are misinterpreted as lower ones, making speech harder to understand for both humans and AI. At 16 kHz, the captured audio covers the essential speech frequencies, but it might still miss subtle tonal variations that help us distinguish between similar-sounding words.
Research has indicated that intelligibility noticeably increases with a higher sampling rate. For instance, a 32 kHz sample rate captures more detail within vowel sounds, facilitating better recognition of distinct speech patterns. This increased precision translates into fewer transcription errors. Going even further to a 48 kHz sample rate allows capturing frequencies beyond the range of human hearing, creating a more natural sound which, in turn, can make it easier for AI algorithms to accurately interpret the speech. Studies have shown that neural networks trained on higher sampling rates like 48 kHz often demonstrate improved performance in deciphering speech in noisy environments.
Interestingly, 44.1 kHz has become a standard due to its prevalence in music recordings and its close alignment with our auditory range. While primarily aimed at music, it's a viable choice for speech capture as well, striking a balance between detail and manageable data sizes. However, recording at 8 kHz can lead to misinterpretations of spoken words, increasing the reliance on human intervention to fix errors during the transcription process. Estimates suggest that using 8 kHz could potentially require up to 25% more correction time, which can be costly and time-consuming.
Higher sampling rates, while delivering more detail and enhanced accuracy, also translate to larger file sizes, requiring more storage space and demanding greater processing capabilities. It's an interesting engineering tradeoff that needs careful consideration when setting up transcription workflows. The challenges associated with frequency response and aliasing at lower sample rates also underline the importance of carefully considering the entire recording setup. Subtle variations in audio equipment or recording environments can worsen effects like distortion and introduce inaccuracies into the transcribed output. Ultimately, understanding the nuances of sampling rates and their interactions with other factors in the audio pipeline is crucial to achieving consistently accurate and reliable AI transcriptions.
7 Critical Audio Quality Factors That Impact AI Transcription Accuracy in 2024 - Environmental Echo and Room Acoustics Management
Environmental echo and room acoustics are critical factors in obtaining high-quality audio for AI transcription. How sound behaves in a space—its movement, reflections, and absorption—significantly affects the clarity of captured audio. Managing room acoustics well is crucial to minimizing undesirable elements like echoes, which can distort recordings and hinder accurate transcription. The size, shape, and the materials used in a room's construction all combine to define the unique acoustic environment. Understanding and controlling these aspects is essential for achieving good audio and optimal performance from AI transcription systems. While digital signal processing can help mitigate some issues, addressing room acoustics directly can often lead to a more natural and clearer audio recording. Ignoring these factors can lead to difficulties for AI models interpreting the recordings, ultimately causing inaccuracies in transcriptions.
Environmental echo and room acoustics are often overlooked factors that significantly influence the accuracy of AI transcription in 2024. The physical characteristics of a room, like its size and shape, profoundly impact how sound travels and reflects. Larger spaces often have longer reverberation times, causing a blurring of sound that makes it hard for AI to isolate speech amidst the echoes and reflections.
The materials used in a room's construction also play a crucial role. Hard surfaces, like glass or concrete, reflect sound, while softer materials, like carpets or acoustic panels, absorb sound waves. This interplay between reflection and absorption heavily influences audio quality, affecting how clear and accurate a recording is, which is critical for AI transcription systems.
Understanding the difference between echo and reverberation is helpful. Echo is a distinct, repeated sound caused by reflections from distant surfaces, while reverberation is a more blended, complex series of reflections that can blur or muddy the original sound, making it harder for AI to decipher words correctly.
Furthermore, each frequency of sound behaves differently in a room. Low frequencies tend to pool in corners and along walls, while higher frequencies spread out more quickly. Where you place microphones in relation to these frequencies can make a big difference in the quality of the audio that's captured. If the audio captured is unbalanced in terms of frequencies, the AI may have trouble interpreting the speech correctly, resulting in transcription errors.
One promising strategy for improving room acoustics is to manage sound diffusion. Using diffusers to scatter sound waves can help reduce strong, unwanted echoes and reflections, which can benefit the quality of audio for AI transcription systems. The layout and type of furniture can also impact sound. Strategic placement of furniture can help redirect sound, leading to a more even distribution of audio and minimizing undesirable echoes.
The type of microphone used in a room also matters greatly. Directional microphones are designed to pick up sound primarily from a specific direction. In complex acoustic environments, this ability to focus on the desired sound can significantly reduce the effect of reverberation and noise coming from other angles.
Controlling echo effectively often involves a combination of sound absorption using acoustic treatments and digital signal processing (DSP) algorithms for noise reduction. This combined approach can make a significant improvement in the clarity of audio for AI transcription systems.
Interestingly, our own human perception of echo is closely linked to speech clarity. When echoes are overly dominant in a space, we struggle to understand speech, leading to difficulties for AI systems as well. Understanding this link is key to developing better solutions for managing echoes in audio captured for transcription.
Finally, the delay between the original sound and its echo is a crucial temporal factor. Delays greater than 50 milliseconds can confuse AI processing. This underscores the importance of effective room acoustics design and treatment in minimizing delays and achieving optimal transcription accuracy. Overall, managing environmental echo and room acoustics is essential to ensure that the audio captured for AI transcription is of the highest quality and that the transcriptions produced are as accurate as possible.
7 Critical Audio Quality Factors That Impact AI Transcription Accuracy in 2024 - Audio Compression Artifacts and Their Effect on Word Detection
Audio compression, a technique used to reduce file sizes, can introduce unwanted artifacts that negatively impact the accuracy of AI transcription systems in 2024. These artifacts are essentially distortions caused by methods like MP3 and AAC compression, which prioritize efficiency by eliminating data deemed unimportant to the human ear. While humans might not notice these subtle changes in high-quality audio, AI algorithms can struggle with the altered audio signals, especially in speech. These artifacts can range from minor distortions to more noticeable changes, depending on the compression method and the original audio characteristics. Interestingly, speech appears to be more susceptible to the negative impacts of these artifacts compared to musical recordings. This highlights the need for further research into how compression affects AI performance and the development of new techniques that minimize detrimental impacts. Developing AI models that are more resilient to such artifacts is a critical path towards improved transcription accuracy. Essentially, we need to address these audio distortions to create a smoother pathway for AI to accurately process and understand spoken words.
Audio compression, a technique used to reduce file sizes, relies on principles like perceptual irrelevance and data redundancy. Techniques like MP3 and AAC achieve high compression by exploiting the fact that humans don't perceive all audio frequencies equally. However, this process often introduces artifacts that differ from traditional distortion. While these artifacts might be imperceptible to humans listening to high-quality audio, automated systems like AI transcriptions can be heavily impacted.
Some compression techniques, like those based on psychoacoustic models, try to minimize the downsides of compression while reducing file size. They do this by focusing on what is considered relevant to human perception. The issue is that music and speech react differently to compression, meaning each needs a custom approach. Modern signal processing allows for better compression, but it also brings the potential for more noticeable artifacts when the data is uncompressed.
When evaluating the audio quality of compressed files, we can rely on subjective listening tests, but these are less useful for objective evaluation and comparison. The interplay between objective metrics and human perception is crucial for understanding how the audio is perceived. Understanding how these artifacts arise and how they affect the audio data is fundamental for designing methods that can lessen their effect during compression.
Emerging audio technologies should focus on creating models to understand how compression generates artifacts, measure their presence, and control their impact. Doing this is important to maximize overall audio quality for both people and AI systems. Ultimately, how well AI transcribes audio is dependent on the input audio quality, and that quality can be degraded by the specific types and levels of compression artifacts introduced during processing.
It's fascinating that the same technique meant to improve the transfer and storage of audio can inadvertently make it harder for AI models to understand speech. It demonstrates the intricate relationship between the methods we use to manipulate audio data and the impact on AI's ability to interpret it. One area of focus for researchers is how the combination of multiple compressions, possibly introduced through editing and mixing, leads to progressively more complex effects. We need to understand how these interact to develop new solutions that can both maximize storage efficiency and preserve the original audio information for the AI.
7 Critical Audio Quality Factors That Impact AI Transcription Accuracy in 2024 - File Format Selection from WAV to MP3
When it comes to choosing audio file formats for AI transcription, the difference between WAV and MP3 is important. WAV files, because they are uncompressed, keep all the original audio information. This makes them a good choice for professional work where the quality of sound is very important. MP3s, however, use a method called lossy compression to make the files smaller. This can lead to a reduction in the quality of the audio, potentially removing subtle details that are needed for very precise transcriptions. This conflict between making files easy to handle and keeping the full detail of the audio becomes particularly noticeable in situations where every aspect of speech must be captured for accurate AI analysis. As a result, carefully thinking about which file format you choose can greatly affect how well your transcription process works in different situations.
When considering the transition from WAV to MP3 for AI transcription, a few crucial points emerge. WAV files, being uncompressed, capture the full spectrum of sound, including subtle variations and rapid changes in volume, which can be lost in the compression process. MP3 compression, while reducing file size significantly, can flatten the audio's dynamic range, potentially sacrificing fine details in both high and low frequencies.
Our hearing doesn't perceive all frequencies equally, a fact MP3 encoding leverages to remove or reduce inaudible components. This can introduce noticeable artifacts, especially in the higher frequencies of speech, creating challenges for AI algorithms that rely on precise audio interpretation. The standard 44.1 kHz sampling rate often used for music may not be optimal for AI speech recognition. Higher sampling rates, like 48 kHz, could better capture the intricacies of speech if recorded directly in WAV format, resulting in potentially improved AI transcription outcomes.
MP3 encoding often utilizes a Variable Bit Rate (VBR), meaning it allocates more data to complex parts of the audio while using less for simpler ones. This can sometimes result in better perceived audio quality compared to a constant bitrate but still introduces potential losses that may impact transcription accuracy. AI models are particularly challenged by the errors introduced during the compression process, which can be amplified when using lower-bitrate MP3 files. Essential phonetic information might be stripped away, leading to misinterpretations by the AI.
The recording environment also adds complexity to the conversion from WAV to MP3. Room acoustics and background noise can alter how compressed files are interpreted, influencing AI performance and potentially decreasing accuracy compared to an uncompressed original. Studies indicate that delicate cues like emotion, tone, and pitch, often present in uncompressed WAV files, can become distorted or lost in compression, hindering AI's ability to grasp the context of conversations.
Moreover, various MP3 encoders use differing algorithms and achieve varying degrees of compression, influencing audio fidelity and the introduction of compression-related artifacts. The specific encoder chosen can affect how well the AI model performs on a particular audio file. While MP3s offer storage efficiency, WAV files are better suited for long-term archiving due to their ability to avoid the accumulation of artifacts that can occur when files are repeatedly compressed and decompressed.
Lastly, the conversion from WAV to MP3 introduces errors that cause distortion. This can be especially noticeable in rapid speech or sounds like "s" and "sh". These distortions confuse AI transcription models, making accurate interpretation more difficult. It's a complex interplay between the chosen file format, the specific compression techniques used, and the intricacies of AI algorithms, highlighting the need to carefully consider these factors when aiming for the best possible AI transcription results.
7 Critical Audio Quality Factors That Impact AI Transcription Accuracy in 2024 - Input Device Settings and Gain Level Optimization
The quality of your audio recording significantly impacts the accuracy of AI transcription, and a crucial element of achieving high-quality audio is optimizing input device settings, especially gain levels. Gain essentially controls the amplification of the audio signal from your microphone or other input source before it's processed digitally. Setting gain correctly is crucial – it can enhance the quality of the audio by improving the clarity and reducing unwanted noise. However, if the gain is set too high, it can lead to clipping, a form of distortion where the audio signal exceeds the maximum level the device can handle. Clipping creates unpleasant artifacts that make it challenging for AI transcription systems to correctly interpret the audio.
Finding the sweet spot involves setting the gain level so that peak audio signals are close to the maximum but never exceed it. This practice, known as gain staging, helps ensure the optimal signal level across the entire audio processing chain, leading to cleaner, clearer audio. Utilizing level meters on your audio interface or recording software can help you visualize and manage these levels in real-time, allowing you to avoid clipping and optimize the recording. It's important to remember that the best gain setting might vary depending on the specific environment and type of audio you are recording. Paying close attention to these details and using the right tools for monitoring audio levels is critical for getting the best possible results from AI transcription.
Input device settings, particularly gain level optimization, play a surprisingly critical role in achieving high-quality audio for AI transcription. Gain, essentially the amplification of the audio signal, can significantly impact the microphone's frequency response, especially how low frequencies are handled. If set too high, it can introduce unwanted bass distortion, while setting it too low can prevent the capture of subtle speech nuances. Finding that 'sweet spot' is key.
Research indicates that optimal gain levels typically fall within a specific range where the balance between signal and background noise is maximized. Exceeding this range commonly leads to clipping, a phenomenon where the loudest parts of the audio are 'cut off', producing unpleasant and unintelligible distortion. AI algorithms, even advanced ones, struggle to decipher clipped audio, leading to transcription inaccuracies.
There's an interesting distinction between digital and analog gain. While it's possible to apply gain digitally after recording, analog gain—applied before recording—often provides superior audio fidelity. This is because analog gain preserves the original dynamic range and sound clarity, critical for AI transcriptions that require precision.
Furthermore, increasing gain magnifies everything captured by the microphone, including unwanted background noise. This can severely impact the quality of the audio input, making it harder for the AI to accurately distinguish between speech and unwanted sounds. This emphasizes the importance of a quiet recording environment when using higher gain settings.
Where you place the microphone also affects the gain settings. With close miking, you can often use lower gain levels without introducing too much unwanted noise. However, with distant miking, higher gain might be required, leading to amplification of unwanted sounds, potentially degrading transcription quality.
Using a unity gain setting, where the output level matches the input level, can sometimes provide the cleanest audio. This helps to avoid any unnecessary signal degradation, which is important for AI transcription accuracy, as it relies on clean, undistorted waveforms.
The process of adjusting gain through different stages of the audio production process—known as gain staging—requires careful consideration. The cumulative effect of amplification at each stage can degrade the signal over time, leading to unexpected distortions that can easily confuse AI models.
Dynamic microphones are inherently less sensitive than condenser microphones and need higher gain levels to achieve optimal recording. However, this often leaves less headroom for sudden, loud sounds (like a shout), potentially inducing distortion and transcription errors.
Even a microphone's directional pattern can affect the ideal gain levels. A shotgun microphone, for instance, might be able to operate at higher gain when aimed at the speaker, but improper management of the gain can overload the microphone, generating artifacts that negatively impact the reliability of AI transcription.
While automatic gain control (AGC) systems attempt to automatically manage audio levels in changing environments, their constant adjustments can reduce the clarity of the audio signal. This inconsistent audio level can confuse AI transcription systems, leading to unsteady performance and errors in word recognition.
Gain optimization, therefore, isn't just about making the sound louder; it's about fine-tuning the amplification of the audio signal to achieve the ideal signal-to-noise ratio and prevent unwanted distortion. A deeper understanding of how gain settings impact the audio signal is essential to improve the accuracy of AI transcriptions in a variety of real-world audio environments.
Experience error-free AI audio transcription that's faster and cheaper than human transcription and includes speaker recognition by default! (Get started for free)
More Posts from transcribethis.io: