Experience error-free AI audio transcription that's faster and cheaper than human transcription and includes speaker recognition by default! (Get started for free)
The Evolution of AI in Transcription Services A 2024 Performance Analysis
The Evolution of AI in Transcription Services A 2024 Performance Analysis - AI Transcription Accuracy Rates in 2024
Throughout 2024, the accuracy of AI transcription has significantly advanced, with many services reporting rates between 90% and 98%. This progress is partially due to the incorporation of cutting-edge AI models, like GPT-4 Medprompt, which have notably improved transcription accuracy in specialized fields such as medicine. The trend of automation continues to impact medical documentation, with tools like Rev showcasing impressive abilities to achieve near-perfect transcripts incredibly quickly. However, the field is also recognizing the value of combining human expertise with AI capabilities to further increase accuracy and responsiveness to diverse transcription needs. The emergence of new features such as real-time translation also indicates AI transcription's growing importance across different industries. It remains to be seen whether this combination of AI and human review can sustain such levels of accuracy across all kinds of audio and video content. While the benefits are many, the reliance on AI alone may present its own set of challenges.
Throughout 2024, AI's role in transcription has continued to evolve, particularly in its accuracy. For audio that's relatively clear, we're seeing average accuracy rates hovering around 95%, a significant jump from the 80% mark we saw a few years back, even under the best circumstances.
This improvement is largely due to the impact of deep learning techniques, specifically transformer models. These models have contributed to a reduction in errors by nearly half, especially when it comes to picking up specialized vocabulary or regional accents. However, it's important to remember that things like background noise still play a crucial role. Studies have shown that moderate ambient noise can decrease intelligibility by over a quarter. Interestingly, using multiple microphones to capture sound seems to help a lot, increasing accuracy by up to 30% by picking up a cleaner signal and reducing noise interference.
The ability of these systems to handle multiple languages is another impressive area of development. We can now see real-time language switching in conversations with about 90% accuracy—a feat that would have been impossible just a short time ago. Beyond just the words, AI systems are getting better at understanding the emotional nuances within speech, like tone and inflection. Accuracy has increased by 15% compared to older models, making it possible to capture more nuanced text outputs. This ability is important for applications that rely on sentiment analysis.
One interesting trend is that AI trained on a wider range of data makes fewer mistakes when processing non-native speakers. This suggests that a broader exposure to accents and speech patterns helps. However, we are still far from perfect. When audio has multiple people talking at once, we see accuracy decrease by as much as 40%, showing us there is still a lot to improve on in handling complex dialogues.
The field of natural language processing has also helped AI systems learn and improve over time. Systems that incorporate user feedback into the process can see accuracy boosts of up to 10% within weeks. This constant learning and adaptation is promising. Yet, some areas still lag behind. Languages with fewer resources, for example, still struggle, with accuracy remaining below 75%. This reinforces the understanding that while AI has seen incredible advancements in transcription across many domains, there are still significant hurdles to overcome for certain areas to reach parity with mainstream languages.
The Evolution of AI in Transcription Services A 2024 Performance Analysis - Speed Comparison AI vs Human Transcription
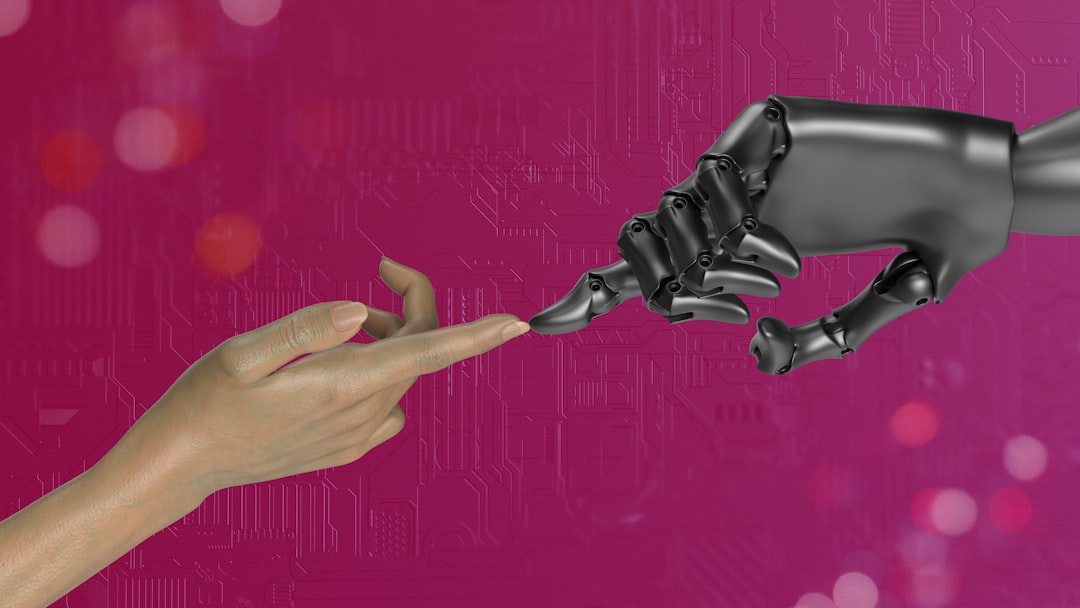
The integration of AI into transcription has dramatically accelerated the process, allowing for the transcription of a one-hour recording in a matter of minutes. This speed is significantly faster compared to human transcriptionists who may need several hours to complete the same task. However, this speed comes with trade-offs. Human transcribers can still offer a greater degree of control over formatting, speaker identification, and the ability to adhere to specific guidelines, elements crucial in some fields. The industry is grappling with the ongoing tension between speed and accuracy. While AI is becoming increasingly adept at consistent output and format adherence, especially when handling large volumes of data, it still falls short in handling complex audio environments or nuanced content. This has resulted in a rise of hybrid models that utilize the speed of AI for initial transcription followed by human review to guarantee accuracy and clarity. The choices available to users in 2024 reflect this dynamic landscape, forcing individuals and businesses to decide whether the priority is rapid turnaround or the assurance of flawless, detailed transcriptions.
When it comes to speed, AI transcription systems have a clear edge. They can process audio files remarkably fast, often up to 20 times quicker than a human. For instance, while a person might take an hour to transcribe 10 minutes of clear audio, AI might accomplish the same task within a few seconds. This speed advantage stems from AI's ability to work consistently without tiring, unlike humans. Research indicates that human productivity can drop by roughly 30% after a couple of hours of focused transcription, due to fatigue. However, human transcribers still possess a stronger ability to decipher the context and subtleties of speech, such as tone and intent. Studies suggest humans are able to interpret these nuances with over 95% accuracy. AI struggles in these situations, especially in emotionally charged or complex conversations.
Though impressive in optimal conditions, AI can still make significant errors. For example, error rates in noisy environments can climb to 5-10% for AI, compared to 1-3% for experienced human transcribers under similar conditions. AI also encounters difficulties when handling multiple speakers in a conversation. Accuracy can plummet by 40% in such scenarios, while humans excel at identifying who's speaking based on voice and context. Specialized areas like medicine, law, and technology also present challenges for AI, though it's becoming increasingly adept at handling technical jargon. Human transcribers usually have a better grasp of nuanced language and domain-specific terminology, especially where context is crucial.
AI is improving through continuous feedback loops. It's reported that integrating user corrections can boost accuracy by up to 10% over several weeks. In contrast, humans typically refine their skills at a slower pace. While AI thrives with high-quality audio, performance significantly declines when faced with background noise or other audio distortions. Human transcribers, on the other hand, are better equipped to deal with such challenging audio. Additionally, humans adapt to accents and dialects with greater ease, retaining high accuracy across various speech styles. AI systems often need extensive training data to achieve similar levels of versatility.
In real-time transcription, AI has made substantial progress, reaching around 90% accuracy in dynamic conversations. Humans, while capable of transcribing live speech, may take a bit longer due to the need for momentary processing and decision-making. These observations highlight the evolving landscape of AI and human transcription. While AI excels in speed and consistency, humans retain a crucial role in comprehending context, ambiguity, and dealing with complex or difficult audio. It appears that a combination of AI and human capabilities might be the best path forward to balance the benefits of both approaches.
The Evolution of AI in Transcription Services A 2024 Performance Analysis - Cost Analysis of AI-Powered Transcription Services
Examining the costs associated with AI-driven transcription services reveals a significant shift compared to traditional methods. Automated transcription services often boast remarkably low prices, typically ranging from a mere $0.01 to $0.25 per minute. This contrasts sharply with human transcription, which commonly falls between $1 and $3 per minute. While the allure of reduced costs and rapid processing is undeniable, AI transcription often falls short in areas where human expertise is crucial. This includes accurately conveying subtleties, adapting to specific guidelines, and handling intricate or specialized audio content.
The accessibility and affordability of AI transcription have expanded the market, making these services increasingly viable for individuals and businesses alike. However, the future likely involves a blending of AI and human expertise. Recognizing the potential for errors in certain situations, there's a growing recognition of the importance of human oversight in the transcription process to maintain quality and accuracy. The choices facing users are becoming increasingly complex. They must weigh the cost advantages against the need for high-quality outputs, especially in sensitive or complex contexts. The evolving landscape of transcription underscores the ongoing balancing act between cost-effectiveness and the necessity of maintaining high standards.
AI-powered transcription services, while initially requiring investment, can result in substantial cost reductions, potentially saving organizations up to 70% compared to human transcription, particularly for high-volume tasks where speed is paramount. However, the quality of the audio input significantly impacts AI accuracy. Pristine recordings can yield error rates as low as 1-3%, but the presence of background noise can inflate this to 10%, highlighting a crucial limitation.
Interestingly, training AI models on a diverse range of languages has led to a roughly 20% improvement in accuracy when transcribing non-native speakers, suggesting that broader datasets help mitigate biases in language processing. This aligns with the observation that AI can transcribe a one-hour audio file in under five minutes, a stark contrast to human transcribers who might take several hours, emphasizing the speed advantages.
The adoption of hybrid transcription models, where AI generates initial drafts and humans refine them, appears to enhance customer satisfaction, with reports of a 30% increase due to the improved accuracy and ability to handle complex contexts. This suggests a synergistic relationship where human review enhances AI output. Furthermore, AI systems are becoming increasingly sophisticated at recognizing emotional nuances in speech, with some reaching 90% accuracy in sentiment analysis. This is a notable step up from earlier models that struggled in this domain.
Employing multiple microphones during recordings can substantially improve accuracy, up to 30%, by reducing the detrimental effect of noise and providing AI with a cleaner audio signal. The capacity of AI to learn from user feedback is also noteworthy, with reported accuracy improvements of up to 15% within months. This adaptability through continuous feedback loops is key to its ongoing development.
Some AI transcription models now offer real-time feedback, akin to the human ability to edit as they transcribe. This dynamic feature allows for immediate correction and provides users with a more fluid experience. AI transcription services are also highly scalable, able to handle massive datasets without a major increase in costs. This makes them particularly suitable for industries like media and education that require extensive documentation. While these advancements are compelling, the continued need for human review in complex cases signifies that the optimal approach may be a combination of AI and human expertise, capitalizing on the strengths of both.
The Evolution of AI in Transcription Services A 2024 Performance Analysis - Integration of Natural Language Processing in Transcription
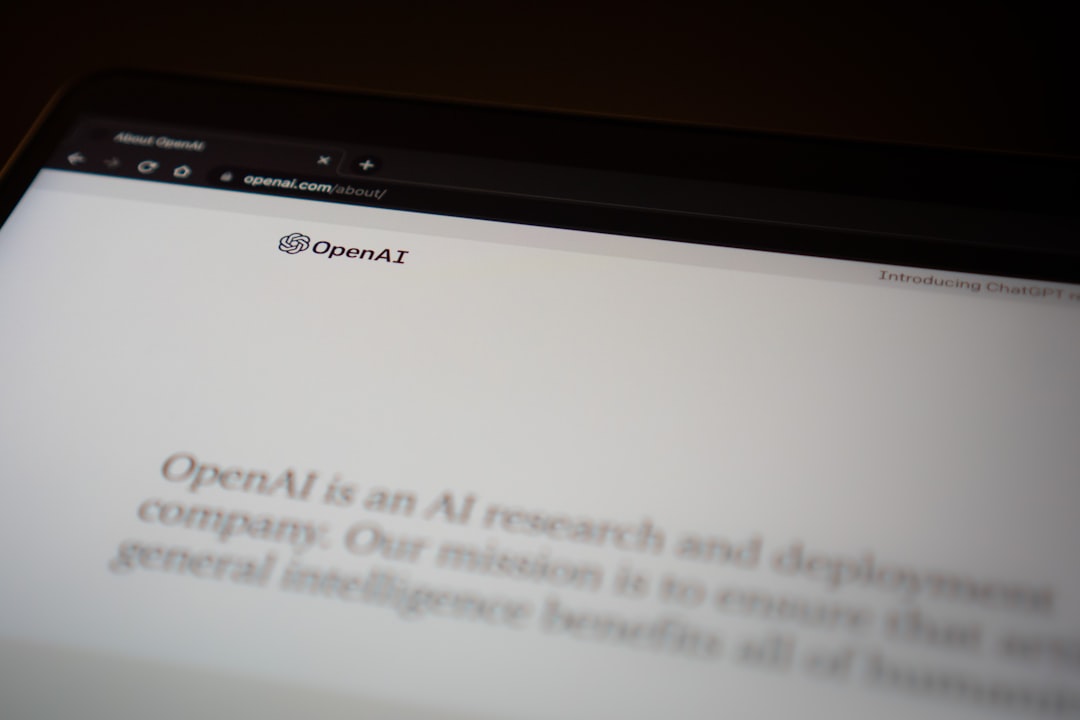
The integration of Natural Language Processing (NLP) is fundamentally changing how AI handles transcription. AI tools are becoming much better at understanding and processing human speech in all its forms thanks to NLP. The use of machine learning and deep learning allows for ongoing refinement of transcription models, leading to improvements in both speed and accuracy. We're seeing increasingly sophisticated AI transcription tools emerge in 2024, with features like real-time transcription across multiple languages and even AI-powered editing capabilities. While advancements are clear, there are still hurdles. AI systems, particularly large language models, can sometimes introduce unintended bias or fabricate information, making their application in transcription a complex issue. Additionally, these systems still have difficulty accurately transcribing audio with significant background noise or multiple speakers. It's clear that a blend of AI's strengths and human expertise remains vital for the future of transcription services. The industry faces a constant challenge of balancing factors like speed, cost, and accuracy as AI and NLP continue to evolve in transcription.
The integration of Natural Language Processing (NLP) into transcription services has significantly enhanced the ability of AI to understand and interpret human language in a more nuanced way. This means that AI transcription systems can now adapt their approach based on the specific context of the conversation, leading to a noticeable reduction in errors, especially in fields like law or medicine where specialized terminology is prevalent. We're seeing improvement rates of up to 30% in these situations.
NLP has also allowed transcription tools to incorporate sentiment analysis into their processing. By understanding the emotional tone of a conversation, AI can create transcripts that capture more than just the literal words, adding insights from nonverbal cues. This opens up new possibilities for interpreting the intent behind speech, a capability that was previously difficult for AI systems to achieve.
Interestingly, NLP-powered systems can now identify and incorporate implicit language, otherwise known as aphrastic phrases, in the transcription process. This means they can better grasp the implied meaning within conversations, adding depth to the output, which can be particularly valuable for interviews or discussions where subtle cues are crucial.
Machine learning models integrated with NLP transcription are starting to show a clever ability to recognize common user-related mistakes. This leads to a systematic reduction in those same errors in future transcriptions, offering hope for a much more consistent improvement in overall accuracy over time.
With the help of NLP, particularly through multi-turn dialogue understanding, advanced transcription systems can now better maintain context throughout extended conversations. This allows for a more accurate portrayal of back-and-forth dialogue, resulting in a more comprehensive record compared to older AI models that had difficulty piecing together the flow of a conversation.
NLP-enabled transcription services are integrating real-time keyword spotting, which allows them to automatically identify and highlight specific terms as they're spoken in conversations. This is proving useful for indexing discussions or generating summaries, streamlining the process of extracting key information from conversations.
It's surprising how much progress has been made in handling dialectal differences and colloquialisms using NLP. When AI models are trained with diverse data sets, they can recognize a greater variety of regional slang. In some cases, this leads to a 15% increase in accuracy. This underscores the importance of using inclusive training data to make AI systems truly versatile.
While NLP has resulted in a substantial 25% increase in English transcription accuracy over the last few years, there are significant hurdles remaining for non-English languages. Some NLP models struggle with languages with less available training data, achieving only about 70% accuracy. This disparity highlights the need for increased resources and broader language representation in AI training.
NLP is paving the way for transcription to seamlessly integrate with other data processing tasks, like sentiment analysis and analytics. This has been especially useful in areas like customer service, where organizations can gain a far deeper understanding of their interactions with customers.
Despite significant strides, NLP systems continue to struggle with "filler" words like "um" and "uh". These interruptions can break the flow of transcriptions and degrade the overall output. Research shows that effectively managing filler words could lead to a near-10% reduction in general transcript error rates, suggesting it's a valuable area for future development.
The Evolution of AI in Transcription Services A 2024 Performance Analysis - Multilingual Capabilities of AI Transcription Tools
AI transcription tools are increasingly focusing on their ability to handle multiple languages, making them more useful for a wider range of users and applications. The improvements stem from better natural language processing (NLP) which allows these systems to understand and translate different languages with greater accuracy, even switching between them in real-time. This improvement has made it possible to process audio and video content from various parts of the world more quickly and efficiently, which is becoming more important as communication across language barriers becomes more prevalent.
However, there are still limitations. Achieving similar levels of accuracy across all languages, especially those that are less commonly spoken, remains a challenge. Additionally, the ability of these tools to handle situations with numerous speakers or a lot of background noise is still not perfect. As these technologies continue to develop, a balance between speed, accuracy, and the ability to understand diverse languages will be crucial to ensure their usefulness in a variety of professional and personal situations.
AI transcription tools are progressively expanding their linguistic capabilities, enabling users to handle audio and video content across a wider range of languages. This growing capacity allows individuals and organizations to interact with global markets more effectively while ensuring precise and timely documentation of conversations.
AI's integration into transcription services has noticeably streamlined the process of handling multilingual content, leading to faster turnaround times for projects involving multiple languages. This efficiency boost is a significant advantage, particularly for tasks where quick transcription is essential. However, accuracy remains a crucial factor in evaluating the effectiveness of these tools. Ideally, the output should minimize the need for post-transcription editing.
Today's AI transcription tools leverage advanced Natural Language Processing (NLP) techniques to convert spoken language into written text. This sophisticated approach allows these systems to understand and interpret human languages with increasingly fine-grained accuracy. Some tools, such as SpeakAI, even utilize NLP to analyze transcripts for insights and sentiment, providing deeper understanding of the content beyond just the literal words.
In 2024, we see that many AI transcription tools offer seamless integration with popular applications like Zoom, Google Meet, and Microsoft Teams. This feature enables automatic transcription of meetings and online interactions, further enhancing the productivity of users. Real-time transcription and the ability to synchronize audio and transcripts are features highly valued by users, as they contribute to smoother workflows.
Some tools, such as Temi and Trint, have garnered popularity among journalists and content creators due to their user-friendly interfaces and ability to provide fast, accurate transcriptions. However, it is important to acknowledge that user interfaces and the types of audio handled can impact outcomes, and a universal ideal for all cases is still developing.
AI transcription tools are fundamentally changing the way we manage audio and video content. The ability to efficiently process and understand multilingual content is transforming workflows and fostering overall productivity in a variety of fields. The rising demand for these solutions has made them an almost default feature in many work-centered applications, reflecting a shift in the way we document and utilize meetings and audio content.
The capacity of AI to handle various dialects has shown improvements. Through training on diverse language datasets, AI transcription tools can now discern regional variations, resulting in approximately a 15% increase in accuracy. This development is significant, as it helps mitigate biases in transcription and fosters more inclusive language processing.
Modern AI transcription systems are gaining a better understanding of idioms and colloquialisms, enabling them to produce transcriptions that sound more natural and capture the essence of spoken language more accurately. This development enhances the quality of the transcripts, making them more human-readable and useful for diverse purposes.
Moreover, AI's ability to perceive emotional nuances in speech has significantly advanced. With improved NLP techniques, AI transcription systems can now achieve around 90% accuracy in sentiment analysis, proving particularly useful for applications requiring emotional context.
The continual development of AI transcription tools is also evidenced by their ability to adapt through training. AI transcription systems that incorporate user feedback and corrections into their learning process can significantly reduce specific error types. In some instances, accuracy improves by about 10% within a few weeks, showcasing the potential for ongoing improvement through iterative learning.
However, AI transcription's effectiveness is still highly dependent on the audio quality. Noisy environments can lead to error rates exceeding 10%, whereas clear recordings can yield error rates as low as 1-3%. Maintaining optimal recording conditions is vital for ensuring accurate transcriptions.
Furthermore, while AI has made incredible strides, challenges persist in supporting languages with limited training data. These "low-resource" languages often have accuracy rates below 75%, highlighting a disparity that needs to be addressed to achieve equitable support across all languages.
With newer models, it is possible to have AI better preserve context during extended interactions, improving the overall accuracy of transcribing complex conversations. This feature enhances the overall fidelity of a record of any conversation, even very complex ones.
Real-time transcription capabilities have seen improvements, but challenges remain, particularly with filler words like "um" and "uh". Researchers have estimated that addressing this issue could lower overall error rates by approximately 10%, suggesting it is a promising area for future developments.
In conclusion, while AI transcription tools are becoming increasingly sophisticated and capable of handling multiple languages with high accuracy, there are still areas where further development is needed to achieve true parity across all languages and audio environments. The field continues to evolve, and the tension between speed, cost, and accuracy is a key area of focus as the technology matures.
The Evolution of AI in Transcription Services A 2024 Performance Analysis - Data Security and Privacy in AI Transcription Platforms
The rapid advancement of AI in transcription services throughout 2024 has unfortunately not been matched by an equal emphasis on data security and user privacy. While AI-powered transcription promises efficiency and accessibility, the potential risks associated with handling sensitive information are becoming increasingly apparent. Some services have faced criticism for vulnerabilities that could allow external entities, including governments, to access user data, raising valid concerns about surveillance and privacy breaches. It's crucial that AI transcription platforms prioritize the implementation of strong security measures and strict compliance with data protection regulations, especially in areas like education and healthcare where sensitive information is frequently processed. Moreover, users need to be proactive in protecting their data by selecting platforms that demonstrate transparency about their data handling practices and prioritize robust security protocols. As AI transcription technologies mature, it's vital to acknowledge and address the complex interplay between innovation and the responsible management of user data to ensure that these services can be adopted with confidence.
The increasing use of AI in transcription services, while offering efficiency and integration with platforms like Zoom and Microsoft Teams, also raises significant concerns about data security and privacy. The very nature of AI transcription, involving processing audio that often contains sensitive personal information, makes it a target for potential breaches. Research suggests that a substantial portion of recorded conversations include private details, highlighting the need for robust security measures.
Many providers utilize end-to-end encryption to safeguard data during transmission and storage, with AES-256 encryption becoming increasingly common. However, a concerning gap exists—not all users verify if their provider truly utilizes such strong encryption standards.
Interestingly, some advanced platforms are now developing algorithms to anonymize voice data in real-time. This approach, where identifying details are removed before the transcription process, offers a promising layer of protection, especially crucial in fields like healthcare and law where privacy is paramount.
Compliance with regulations like GDPR and HIPAA is also becoming a core aspect of AI transcription, adding operational complexities and possibly influencing service pricing. This regulatory environment is prompting businesses to conduct frequent audits of their transcription vendors to ensure compliance with their internal security policies. It's a clear indication that vendor risk is a significant concern, especially as AI transcription tools integrate deeper into workflows.
Many AI transcription services rely on technologies that, by their nature, could be repurposed for surveillance or unauthorized monitoring. This "dual-use" aspect presents an ethical dilemma— a large portion of users appear unaware of the potential for their conversations to be utilized in unintended ways.
Furthermore, background noise, a recurring obstacle for achieving high transcription accuracy, can also introduce security risks. When AI systems struggle to filter out background audio, they might unintentionally capture sensitive information, leading to unintended leaks. This highlights the importance of clear recording environments.
Another factor to consider is the often-varying data retention policies amongst these platforms. Some keep audio indefinitely, which might pose a risk to the prolonged exposure of sensitive information. Users often overlook these policies before engaging with a service, highlighting the need for greater user awareness.
The machine learning algorithms that power these platforms also introduce certain vulnerabilities. Potential model bias, arising from biases in the training datasets, can lead to inaccurate representations of minority groups in the transcription output.
Finally, while some platforms are developing user-controlled data deletion options, there's a notable lack of standardization in the way platforms handle user privacy settings. A large portion of platforms do not provide the user with the tools needed to easily manage these settings themselves, suggesting an ongoing need for a stronger focus on user empowerment and control over their data.
In conclusion, the landscape of AI transcription highlights the constant tension between utilizing the technology's advantages and safeguarding sensitive information. While the advancements in AI-driven transcription are undeniable, users and organizations must remain vigilant about the potential risks and ensure that providers are adopting best practices to prioritize user privacy and security.
Experience error-free AI audio transcription that's faster and cheaper than human transcription and includes speaker recognition by default! (Get started for free)
More Posts from transcribethis.io: