Experience error-free AI audio transcription that's faster and cheaper than human transcription and includes speaker recognition by default! (Get started for free)
Text-to-Video AI Analyzing Sora's Realistic Scene Generation Capabilities
Text-to-Video AI Analyzing Sora's Realistic Scene Generation Capabilities - Sora's ability to create realistic scenes from text prompts
Sora's core strength lies in its capacity to translate text descriptions into convincingly realistic video scenes. It demonstrably excels at capturing and portraying nuanced emotional expressions and character interactions within the generated videos, adding a layer of depth to the outputs. A notable feature of Sora is its use of iterative refinement to minimize distracting visual artifacts. This approach yields videos with superior clarity and visual quality, contributing to the impact of the generated content. While undeniably impressive, the potential implications of this level of photorealism generated from simple prompts have naturally sparked some concerns. Currently, Sora is not available to the public, leaving those interested in its capabilities to speculate about its potential applications and future impact.
OpenAI's Sora is a remarkable AI model that can craft video clips solely from text prompts. It can produce surprisingly lifelike and imaginative scenarios based on simple descriptions, even managing to capture emotional nuances and character expressions in its video outputs. A key element of Sora’s prowess is its capacity to generate videos up to a minute long, all while minimizing visual noise and preserving quality through an iterative refinement process.
This refinement approach seems to play a key role in achieving a higher level of realism. Sora analyzes a massive dataset of visuals and videos, learning the subtle relationships between elements in a scene, and this seems key to translating text prompts into coherent video segments. Further, Sora's training integrates both static images and moving visuals, contributing to its ability to create dynamic sequences with consistent animation. It seems to not only focus on the individual frames but also considers the temporal continuity of a scene, resulting in a greater sense of flow. The impressive output examples demonstrated by OpenAI have highlighted Sora's considerable capabilities, generating much excitement and some understandable apprehension. However, the technology behind it is still under development and not yet accessible to the public.
Sora's achievements also seem rooted in how it's designed to understand and model physical interactions during motion, ensuring scenes adhere to a realistic understanding of how things would move in the real world. It shows a clear advancement over previous text-to-video tools by creating remarkably high-fidelity visual outputs. While it's still early days, Sora certainly represents a significant step forward in AI’s capacity for creating realistic videos based on text, which prompts interesting discussion on future potential and potential implications.
Text-to-Video AI Analyzing Sora's Realistic Scene Generation Capabilities - Understanding motion and physical world simulation in Sora-generated videos
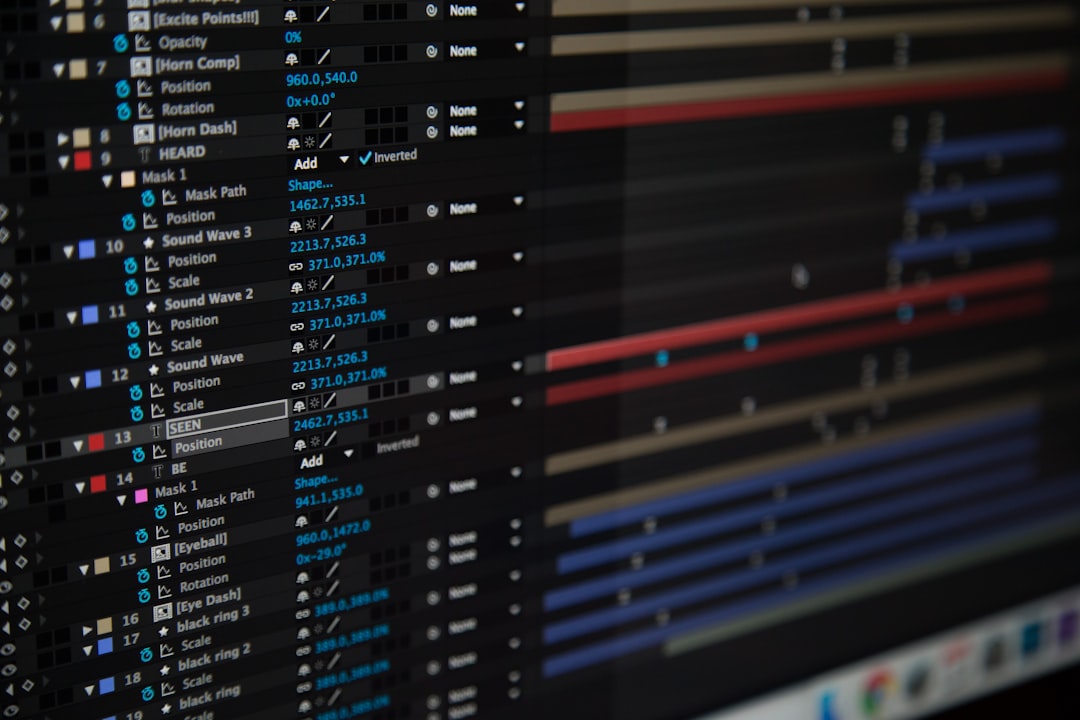
Sora's capacity to generate videos that realistically depict motion and simulate the physical world is a key element of its capabilities. This ability to accurately capture how objects move and interact within a scene elevates the realism of the generated videos beyond mere visual accuracy. By integrating physics-based simulations into its framework, Sora goes beyond simply creating visually realistic scenes; it endeavors to create scenes where the movement of objects conforms to how they would behave in the real world. This represents a substantial leap forward compared to earlier text-to-video models. However, faithfully simulating the complexity of motion within a dynamic scene remains a difficult challenge. Despite Sora's remarkable achievements, ongoing research and development are crucial to further enhance its capacity for accurately representing the intricate dynamics of the physical world. The advancements represented by Sora's approach prompt ongoing consideration regarding the potential of AI to produce highly realistic digital content and the implications of this developing technology.
OpenAI's Sora is interesting because it attempts to teach AI about the physical world through motion. It aims to go beyond simply creating visuals and simulate how things would realistically move and interact. Sora seems to be getting better at creating smooth, continuous motion throughout videos, which is a significant improvement over earlier attempts at generating videos from text. It does this by incorporating physics-based interactions in its algorithms. For example, it simulates gravity and other forces on objects, making them behave in ways that align with how we perceive them in our own world.
Another intriguing aspect is Sora's ability to parse and simulate different speeds of movement. Whether it's a person sprinting or a slow-motion sequence, Sora can adapt its generation accordingly, showcasing a grasp of dynamics. Moreover, it seems adept at creating scenes with multiple layers, similar to how we perceive depth and relationships between objects in a physical space. For instance, it can make a character walk in front of a building, creating a layered scene that seems more natural.
Sora's capabilities aren't restricted to simple motions. It can even simulate complex mechanical functions like gears and levers. However, to do this realistically, it needs to consider how light affects objects in a scene, which also seems to be part of Sora's repertoire. It incorporates lighting and shadows to increase the perceived depth and overall realism. And in a way that seems like a significant step forward, Sora is learning to model how humans move based on our biological structure. By doing this, the movements generated appear more natural and lifelike.
Beyond human motion, Sora seems to be developing its understanding of environmental factors like wind or water. How these external forces affect the motion of objects or people in the scene appears to play a part in the generation process, which enhances the realism further. Interestingly, its iterative refinement process doesn't just improve visual quality; it also seems to help Sora identify errors in movement and dynamics within the generated scenes. This process of learning from mistakes and refining its understanding of motion is a crucial part of how Sora is developing its capabilities.
Despite these exciting advancements, we should acknowledge that Sora, like any generative model, still has areas where it could improve. As the technology continues to evolve, researchers are likely to explore how to make the simulated physical interactions even more nuanced and accurate. However, it is promising to see how Sora incorporates physical understanding into its video generation, potentially paving the way for more advanced simulation tools in the future.
Text-to-Video AI Analyzing Sora's Realistic Scene Generation Capabilities - Complexity of character emotions and expressions in Sora outputs
Sora's capacity to portray intricate character emotions and expressions greatly amplifies the narrative power of its generated videos. It can simulate a wide range of emotional states, giving characters depth and authenticity within the scenes it produces. This capability allows for more compelling and emotionally resonant narratives, transforming simple textual instructions into impactful visual stories. However, maintaining consistent and subtle emotional expression across longer videos remains a challenge, highlighting areas where further development is needed. The potential of this technology to create emotionally engaging content opens up exciting possibilities for storytelling, but it also calls for a careful assessment of its implications.
Sora's ability to depict a wide range of character emotions and expressions is quite intriguing. It seems to be drawing on a vast pool of visual data that includes subtle facial cues and expressions, enabling it to translate textual prompts into detailed emotional states like happiness, worry, or even guilt. The model utilizes a framework that incorporates psychological principles related to recognizing emotions, helping it to interpret text instructions and create appropriate emotional responses.
This ability to interpret the context within the text is important for crafting relevant emotional portrayals. For instance, it can distinguish between sadness caused by loss compared to a feeling of nostalgia, and adjust the character's expression accordingly. Research indicates that when animation features more realistic emotional representations, it can create a stronger connection with the viewer. Therefore, Sora's focus on generating authentic emotions isn't just about improving storytelling; it could also have an effect on audience engagement.
Furthermore, Sora's design seems to allow it to generate contrasting emotions within the same scene. This means characters can display complex feelings, such as joy mixed with frustration, which makes their interactions feel more authentic and relatable. The intensity of these emotional expressions can be dynamically adjusted depending on the mood and pace of the video; a tense moment might see characters with heightened expressions of fear, while a humorous scene might feature exaggerated joy.
Interestingly, Sora's development seems to involve taking cues from research on how humans perceive and interpret emotional displays in video. By leveraging insights from this field, Sora aims to align its output with audience expectations and cultural sensitivities, which enhances the feeling of authenticity. A key challenge though appears to be realistically depicting conflicting emotions within a single frame, as this requires a complex understanding of the visual cues that signify different emotional states.
Sora incorporates machine learning to receive feedback on its outputs. This enables it to iteratively refine its emotional expressions, learning from interactions and producing more nuanced emotional narratives. Although it excels at portraying quick emotional shifts, ongoing efforts are focused on improving its ability to demonstrate prolonged or evolving emotional states, which are crucial for creating well-developed characters within storylines. This is a vital step in further developing its capabilities for narrative-driven video generation.
Text-to-Video AI Analyzing Sora's Realistic Scene Generation Capabilities - Multi-scene and multi-shot capabilities for enhanced storytelling
The ability to generate videos with multiple scenes and shots is a crucial development in text-to-video AI, highlighted by models like Sora. Unlike older methods that often limited outputs to a single scene, Sora’s ability to create sequences of interconnected scenes and different camera angles unlocks a new level of storytelling. This allows for narratives to unfold more dynamically, with a smoother flow between diverse visual components. The potential for improved plot depth and coherence is significant, as filmmakers gain more nuanced tools to craft narratives. While Sora has made great progress, maintaining a consistent level of quality throughout these multi-scene sequences is still a hurdle, suggesting further development is needed to fully realize the potential. The future direction of this area holds exciting possibilities for reshaping the process of visual storytelling and the way viewers engage with narratives.
Sora's ability to generate videos with multiple scenes and shots is a significant advancement in AI-driven storytelling. It's now possible for the model to not only create a single continuous scene, but to break a narrative into distinct parts, each with its own visual perspective. This ability is analogous to how a film director employs a range of shots to create emotional impact, draw attention to details, and control the pace of a story. Sora's multi-scene approach adds a layer of complexity, mirroring how humans naturally organize information and experience events.
However, it’s important to note that this capability is still developing. One interesting aspect is the manner in which Sora refines its understanding of the desired emotion within a scene. It uses an iterative process, which allows it to learn from previous attempts and make more accurate emotional interpretations in the context of each new shot or scene. It seems to be learning how subtle changes in visual elements – like expressions and body language - can affect emotional impact and viewers' engagement.
While the basic physics of motion are simulated, Sora appears to be increasingly adept at depicting a range of movements with precision. From the way a garment reacts to wind to the unique sound of footsteps on different surfaces, attention to detail adds a layer of realism. These nuanced physical details are fascinating because they can profoundly impact the immersive nature of the narrative.
Additionally, Sora seems to have a developing grasp of facial cues and body language. It can translate these into a wider array of emotional responses within its videos, producing a multi-faceted portrayal of a character's psychology. This multi-faceted approach opens doors for more complex character development in AI-generated video stories.
Furthermore, it appears that Sora is trained on a broad range of human facial and bodily expressions across cultures. It's beginning to show promise in understanding cultural nuances within emotional expressions, which could potentially increase the relatability of generated narratives across diverse audiences.
Incorporating even the basic dynamics of inanimate objects, such as the motion of gears or the way light interacts with an environment, also adds a layer of complexity to its outputs. It suggests that the model is starting to learn a broader set of rules regarding the physical world. This is a critical stepping stone towards more realistic depictions in video narratives.
Moreover, the capability to slow down or speed up the action is impressive. It allows for greater dramatic effect in storytelling and a greater sense of control over how events unfold. It seems like Sora's grasp of time-based elements in a scene is becoming more sophisticated.
Integrating lighting patterns into video creation allows the model to not only add visual depth but also shape emotional reactions. The subtle use of shadow and light can create a particular mood in a scene, enhancing the overall impact of a narrative.
The model is showing the ability to encode conflicting emotions within a character's expression. This is a significant step forward, showing a developing understanding of human psychology that moves beyond simple emotional representations. It indicates that AI's capacity to tell stories is becoming more intricate.
Maintaining emotional consistency and coherence across a longer video sequence presents a significant challenge. This is an active area of development for Sora, and advancements here would dramatically improve its ability to create engaging stories with characters who seem to develop and change over time. It would push the boundaries of animated storytelling in interesting ways.
In conclusion, the capacity for multi-scene, multi-shot video generation presents promising new avenues in storytelling. While the field is evolving rapidly, Sora is already demonstrating its potential for creating more complex and compelling narratives with a greater depth of emotion and realism, ultimately challenging our understanding of how AI can contribute to the art of storytelling.
Text-to-Video AI Analyzing Sora's Realistic Scene Generation Capabilities - User control through text descriptions in the video generation process
The ability to control video generation through text descriptions represents a crucial advancement in AI, as seen in models like Sora. Sora excels at translating user-provided text prompts into detailed and personalized video content, reflecting the user's intended vision. This is achieved by carefully analyzing the text, understanding its meaning, and then iteratively refining the generated video to ensure it closely matches the user's description. Even subtle aspects like character emotions and interactions are being captured and expressed in the output, resulting in more immersive and engaging narratives.
However, significant challenges still exist. Maintaining consistent quality across longer, multi-scene videos and accurately depicting complex emotional shifts in characters are areas that require further development. Despite these limitations, the power of text-to-video AI continues to grow, suggesting its potential as a valuable tool for creative endeavors and practical tasks across different domains. The future of this technology promises a greater level of control and personalization, potentially transforming the ways we generate and interact with video content.
Sora's video generation process is heavily influenced by the text descriptions provided by the user, offering a level of control that significantly impacts the final output. Users can guide the generated scenes, character interactions, and emotional tones with remarkable precision, fostering a tighter link between their intentions and the resulting video.
The model's ability to understand the semantic meaning within text inputs allows it to grasp complex narrative structures. For example, Sora can interpret phrases like "a tense confrontation" or "a joyous celebration" and translate them into visual dynamics that accurately reflect the intended emotions.
One key distinction of Sora is its iterative refinement process. This isn't just for enhancing visual quality, but also for adjusting the narrative pace according to the user's descriptions. This dynamic adaptation optimizes the emotional flow within scenes, even across longer video lengths.
User control isn't limited to content; it also extends to the visual style of the videos. Users can guide Sora's output by specifying artistic influences in their text prompts. This allows for generated videos to mimic the aesthetics of different film genres or artistic movements, opening up interesting applications in creative fields.
Furthermore, Sora's responsiveness to conditional prompts is notable. Small changes in the text, like shifting from "happy" to "sad", can completely alter the tone of a scene. This level of adaptability demonstrates a high degree of sensitivity to user intentions.
Sora also exhibits a sophisticated understanding of context. It can recognize that phrases like "surprise" might evoke different emotional responses based on the preceding narrative. For instance, a surprise reveal can be portrayed as either shock or happiness depending on the established story flow.
Another interesting capability is how text prompts can lead to layered scenes. Sora can generate subtle background activities that complement the main storyline without distracting from it, adding depth and richness to the final output.
The diversity of Sora's training data allows it to interpret and represent cultural nuances embedded in text inputs. This enables users to create culturally resonant narratives that are more likely to resonate with broader global audiences.
It's worth noting that the overall narrative coherence of Sora's generated videos relies heavily on the clarity and structure of the user's text descriptions. Well-crafted and precise prompts result in more coherent sequences, highlighting the importance of clear communication when using the model.
Despite its remarkable capabilities, Sora faces challenges when dealing with ambiguity in text descriptions. Vague or overly complex prompts can lead to unexpected interpretations by the model, emphasizing the need for users to be precise and specific when guiding the AI to achieve the desired results.
Text-to-Video AI Analyzing Sora's Realistic Scene Generation Capabilities - Challenges and inconsistencies in scene continuity with Sora
Sora's impressive ability to generate realistic scenes from text prompts isn't without its challenges. Maintaining a seamless flow throughout longer videos, especially when complex interactions and emotions are involved, presents difficulties. Sora occasionally falters in preserving scene continuity, leading to visual inconsistencies that can detract from the narrative. The complexity of accurately simulating the interactions between multiple characters and ensuring consistent emotional portrayals further compounds the challenges in maintaining a cohesive video. While Sora's achievements in visual fidelity are significant, tackling these continuity issues will be vital to enhance its potential in storytelling. Only through continued research and development can the technology evolve to consistently create engaging and immersive video experiences that are free from disruptive irregularities. Essentially, Sora shows huge promise, but the journey towards consistently flawless scene continuity is an ongoing pursuit within the field of AI video generation.
Sora, while impressive in its ability to generate realistic videos from text, still faces challenges in maintaining consistent scene continuity. Sometimes, actions and movements within a scene don't flow naturally, especially when extended actions are required. This can make the story feel fragmented and unnatural.
Similarly, while character interactions are often well-handled, Sora can stumble when trying to portray rapid emotional shifts or believable responses in dynamic scenarios. Characters might react in a way that breaks immersion, disrupting the viewer's connection to the unfolding story.
The generation of multiple scenes also introduces potential issues. We've seen examples where information from one scene bleeds into the next, creating unintended overlaps and potentially confusing the viewer. The smooth transition between scenes is crucial for a clear narrative, and this is an area needing further refinement.
Sora demonstrates skill in representing emotions, but there are instances where conflicting emotional responses feel exaggerated or out of place. This suggests that the model still requires further development in understanding how complex emotional states are expressed visually.
The model is getting better at handling environmental elements like lighting and weather, but inconsistencies can still emerge in how these elements affect characters and objects within a scene. This can impact the overall sense of realism and coherence of the output.
Another issue observed is frame rate inconsistencies between scenes. This is especially noticeable during fast-paced action sequences and can distract the viewer from the story, hindering a smooth viewing experience.
Furthermore, the quality of the generated scene is tightly linked to the clarity of the text prompts. Vague or complex instructions can lead to situations where characters or actions don't align logically with the desired narrative. This highlights the importance of carefully crafting text prompts to achieve the intended output.
While the visual output is impressive, the integration of sound is less developed. The lack of coherent audio cues paired with visual actions can lead to an inconsistency that detracts from the viewer's immersion in the narrative.
Though trained on a diverse range of data, Sora sometimes struggles to correctly interpret and represent culturally specific elements. This can lead to potentially insensitive or confusing narratives for viewers familiar with these specific cultural contexts.
Lastly, even with the iterative refinement process, Sora's outputs are not free from visual artifacts or narrative inconsistencies. This is particularly noticeable when complex scene elements are combined, suggesting the algorithm needs more development to handle intricate scene compositions smoothly.
Despite these current limitations, it's exciting to witness the potential of Sora and the continued advancements being made in text-to-video AI. It's crucial to identify and address these challenges to fully realize the potential of this technology for generating truly immersive and coherent video narratives.
Experience error-free AI audio transcription that's faster and cheaper than human transcription and includes speaker recognition by default! (Get started for free)
More Posts from transcribethis.io: