Maximizing Accuracy 7 Key Audio File Settings for Better Speech-to-Text Conversion
Maximizing Accuracy 7 Key Audio File Settings for Better Speech-to-Text Conversion - Sample Rate Setting 16 kHz Optimizes Speech Recognition Range
When it comes to speech recognition, a sample rate of 16 kHz often strikes a good balance. This rate effectively captures the frequencies essential for understanding human speech, making it well-suited for tasks like transcribing voice recordings or processing voice commands. While higher sample rates might offer finer audio detail, they result in larger file sizes, which can be unnecessary for standard transcription. Choosing 16 kHz not only keeps file sizes manageable but also ensures compatibility with many speech-to-text systems designed for this rate. In essence, 16 kHz helps ensure that the audio data is processed effectively, thereby optimizing the accuracy of the transcription. It's a sweet spot that balances quality and efficiency for many transcription scenarios.
When it comes to capturing human speech for speech recognition, a sample rate of 16 kHz appears to be a sweet spot. It effectively captures the crucial frequency range of human speech, which typically falls between 300 Hz and 3,400 Hz, without including a lot of unnecessary data. This targeted approach retains intelligibility without excessive file size.
While higher sample rates like 44.1 kHz or 48 kHz might seem like they offer better fidelity, especially in music, they lead to larger files for essentially the same speech quality. This extra data can actually create more work for the speech recognition system without noticeable improvement in accuracy.
Research hints that human speech can be understood at even lower sample rates, like 8 kHz. However, 16 kHz provides a noticeable boost in accuracy, especially when dealing with background noise, likely due to reduced distortion. This observation fits with the Nyquist-Shannon sampling theorem which suggests we need a sample rate at least double the highest frequency of interest, which 16 kHz comfortably achieves for speech.
The practical impact of this choice is immediately apparent in file size. Using a 16 kHz mono file cuts the storage space needed compared to a 48 kHz file in half. For bandwidth-limited applications or systems with limited processing power, this can be quite important. Furthermore, sample rate impacts processing speed or latency. A lower sample rate can enable faster processing, which is essential for real-time speech applications like live transcription.
Many speech recognition systems are sophisticated enough to handle various sample rates. However, starting with 16 kHz often provides a good balance between accuracy and processing demands for initial tasks. It seems that higher sample rates can introduce distortions or artifacts if not carefully processed, making the potential for degradation higher. Conversely, 16 kHz usually provides adequate clarity for most speech applications without these risks.
Finally, the choice of 16 kHz seems to capture the nuances of speech that are important for transcription. Speech variations like accents and dialects don't appear to be negatively affected by this selection. The natural pauses and changes in tone during conversation are also captured well enough that it seems to allow the speech recognition models to pick up on important contextual clues in the spoken words. All this together makes 16 kHz a robust choice for a wide range of speech transcription applications.
Maximizing Accuracy 7 Key Audio File Settings for Better Speech-to-Text Conversion - Audio Channel Compression From Stereo to Single Track
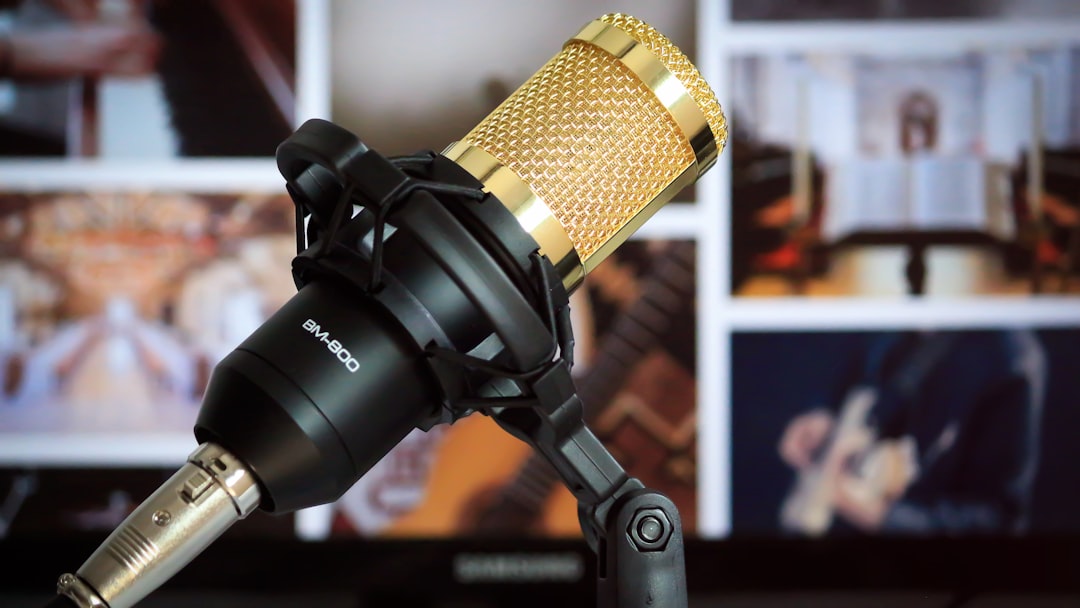
When preparing audio for speech-to-text, reducing the audio channels from stereo to a single track can improve the accuracy of the transcription process. This simplification helps to balance the loud and soft parts of the audio, which is crucial for speech-to-text algorithms to understand what is being said. By focusing on a single track, we eliminate potential confusion created by having two separate channels. Certain audio compression techniques can help in this process. Ideally, using careful compression settings, like a slower attack and a fast release time, can smooth out large variations in volume while retaining the natural character of the speech. However, it's important to avoid overdoing it with compression. Excessively compressing the audio can make it sound artificial, which can lead to a worse transcription. In essence, moving to a single track can assist speech-to-text software, but if not handled properly, can end up hindering accurate transcription.
Reducing audio from two channels (stereo) down to a single channel (mono) can have a noticeable impact on speech-to-text accuracy. This process, while seemingly simple, can actually improve how well a speech recognition system understands the audio.
One of the primary reasons this works is that it reduces the potential for interference caused by phase issues. Stereo recordings, especially when using two microphones, can introduce slight time delays between channels. These delays, even very small ones, can create conflicting signals when the channels are combined, resulting in audio artifacts that can be challenging for speech recognition systems to interpret. By collapsing the sound into a single channel, we remove these conflicts, improving the overall clarity and consistency of the audio.
There's also the aspect of cognitive load to consider. While we are very good at processing stereo audio for music or other spatial sound experiences, it appears the human brain uses more resources to process these sounds for speech understanding compared to mono. Converting to mono in essence simplifies the signal for our minds. This leads to better comprehension, which can indirectly boost the performance of speech recognition systems, because the simplified input provides fewer distractions for the listeners' processing power.
Furthermore, converting to mono can simplify the processing that's necessary to improve audio quality, allowing for easier dynamic range control. The dynamic range of an audio signal is the difference between the loudest and softest sounds. While we might enjoy the range of dynamics in a musical performance, this wide range in a speech recording can make it harder for a speech-to-text system to consistently hear and interpret speech sounds. By narrowing the dynamic range, the audio levels become more uniform and easier to process. There is some debate on the amount of dynamic range reduction needed for this process. Too much reduction can harm the natural quality of speech.
Noise reduction and unwanted sounds in the audio can be impacted by converting to a single track. Stereo recordings can pick up background noise from both channels. While it's true that the noise can be mixed and possibly slightly reduced when converted to mono, there's also a potential risk. Certain kinds of noise (like high-frequency wind) might not necessarily get reduced, and we'd need to examine that for each scenario.
Additionally, there's the benefit of improved compatibility when dealing with different systems and algorithms. Many speech-to-text systems are optimized for mono signals. Using mono from the beginning can improve the compatibility between the audio recording and the transcription process, which reduces the need for conversion steps and can lead to faster processing. Also, the simpler signal makes it easier for the algorithm to process, leading to faster response times, which is essential in situations like live transcription.
It's important to acknowledge that while converting to mono generally helps with speech-to-text accuracy, there are potential caveats. One such concern is the possibility of losing spatial information, especially if the goal is to accurately transcribe a multi-speaker interaction. However, for applications where the focus is on capturing and understanding the speech of a single individual in a fairly quiet or controlled environment, mono audio might provide a big enough gain to make it a beneficial practice.
Ultimately, the choice of using a mono track for speech-to-text applications should be driven by the specifics of the environment and the speech being captured. For many scenarios, the improved clarity, consistency, and efficiency gained through mono conversion can have a significant positive effect on the accuracy of speech-to-text systems. The advantages can sometimes outweigh potential drawbacks, but these drawbacks and use cases need further research and understanding.
Maximizing Accuracy 7 Key Audio File Settings for Better Speech-to-Text Conversion - Matching Audio Input Format To Match Your Target Environment
Ensuring your audio input format aligns with the environment where the speech-to-text conversion will occur is essential for getting the best results. The characteristics of your audio file, things like how it's compressed and the number of audio channels, can greatly affect how well the system works and how accurate the transcription is. For instance, using compressed audio can make processing faster, but this needs to be balanced carefully so that you don't introduce glitches or issues that the speech recognition system might misinterpret. Similarly, adapting your audio format to the specific situation, for example, using a single audio track for situations where just one person is speaking, can simplify the task for the speech recognition engine. This can improve the clarity of the audio by lessening possible interference or confusion and by making it easier for the system to understand the spoken words. In short, careful selection of the characteristics of your audio data can have a big impact on the effectiveness and accuracy of your speech-to-text application.
When it comes to getting the best results from speech-to-text systems, it's crucial to align the audio input format with the target environment and the specific speech recognition system you're using. Just as we've seen that a 16 kHz sample rate provides a good balance for capturing speech without unnecessary data, the choice of audio input format is also important.
The frequency range that human speech occupies—roughly 300 Hz to 3,400 Hz—is a starting point. If we select audio formats that emphasize this range, we can optimize how clearly the speech is understood by the transcription system. This is particularly important when we're dealing with recordings that might have noise or interference.
Different systems might handle various audio codecs with differing levels of efficiency. Some codecs, like Opus or AAC, prioritize speech quality over things like high fidelity music playback. Experimenting to see if those codecs improve accuracy might be worthwhile.
Bit depth, which measures the precision of the audio signal, can also play a role. While 24-bit audio might seem like a better choice than 16-bit, for most speech-to-text purposes, the added precision doesn't result in noticeable improvements in accuracy. The larger file sizes and increased processing demands might end up being detrimental.
Noise, unfortunately, is often a part of recordings. Speech-to-text systems often use noise profiles to improve accuracy, but it's more effective if the audio format captures the types of noise in the target environment.
Some of the more advanced speech recognition systems use machine learning to adapt to various recording environments. Selecting the right format can help with this process, leading to better accuracy in situations that vary from quiet offices to bustling public spaces.
If a recording is done in stereo, we might find that both channels are capturing similar components of the speech. Converting this to mono can simplify the input, eliminating potential redundancy and resulting in cleaner audio for transcription.
The audio format can also influence the processing speed, or latency, of a speech-to-text system. Formats designed for low-latency transmission can be important for real-time transcription, where every millisecond matters.
High levels of audio compression can create artifacts that can make it harder for a speech recognition system to understand the speech. Finding an audio format that balances compression with clarity can minimize this problem, improving transcription accuracy.
The choices of microphone and audio format need to be matched to the recording environment. In noisy situations, using a microphone that specifically reduces ambient noise will undoubtedly improve the accuracy of transcription.
And lastly, if we're working with recordings that have multiple speakers, careful consideration of audio format and sampling techniques is crucial. Using formats that preserve some spatial information in stereo can help distinguish who is talking and add a sense of context to the conversation. This, in turn, can further improve transcription quality.
It's clear that there are a lot of details to consider when preparing audio for speech-to-text. While the relationship between these factors and transcription accuracy still needs further research, aligning audio format with the target environment and speech recognition system can help optimize the accuracy of speech transcription for diverse use cases.
Maximizing Accuracy 7 Key Audio File Settings for Better Speech-to-Text Conversion - Frame Size Adjustment For Millisecond Level Processing
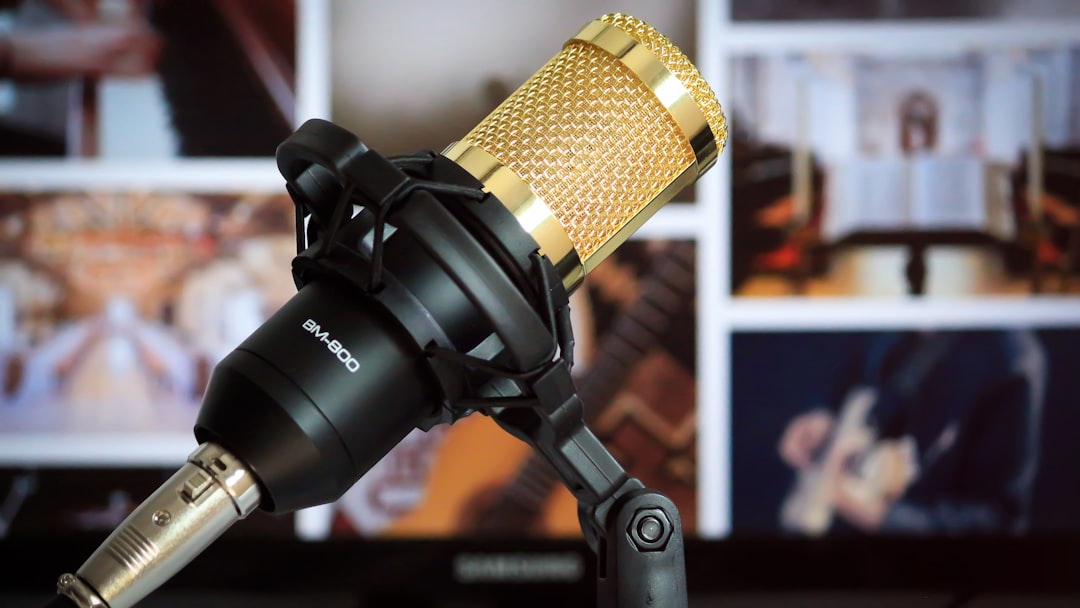
When we're working with audio for speech-to-text, the way we break it into smaller pieces, known as frames, plays a crucial role in how accurate and timely the transcription process can be. The size of these frames directly impacts the speed and efficiency of processing, as well as the overall level of detail captured in the audio.
A good starting point for finding this balance is using a frame size of 100 milliseconds. It allows for manageable processing times while providing enough information to the system. However, for tasks that require a finer level of detail, like fast-paced conversations or real-time speech, it may be better to experiment with significantly shorter frames, perhaps 16 milliseconds. This change can significantly improve the recognition of finer audio elements and enhance the speed of the process.
Furthermore, a technique called windowing with overlap can help to smooth out the transition between frames, reducing the risk of losing important audio details as the frames change. It can increase the accuracy of detail and understanding, especially useful with longer frame lengths.
In the end, choosing the optimal frame size for speech-to-text is a careful exercise in understanding both the technical aspects and the specific needs of the situation. Is processing speed more important than picking up every nuance of the speech? What is the environment where the recording was made like? Answers to these types of questions can help in making this choice.
When it comes to optimizing speech-to-text accuracy, understanding how frame size impacts processing is crucial. The frame size, essentially the duration of a segment of audio analyzed at a time, plays a pivotal role in achieving that sweet spot between speed and accuracy. Smaller frame sizes mean faster processing, which is critical for applications like live transcription, but can result in a loss of audio detail and increased susceptibility to noise. Conversely, larger frames provide a clearer audio picture, which can be beneficial for complex audio environments but may add latency and slow down real-time processing.
Research suggests a frame size around 20-40 milliseconds often works well, capturing enough information for speech features while minimizing unpleasant audio artifacts that could cause inaccuracies. There's an inherent trade-off involved with this setting—adjusting the frame size affects processing speed, the capacity to understand subtle nuances in the speech signal, and the impact of background noise. What might work in a quiet environment might not hold up when the recordings are taken in places where there are a lot of sudden or strong noises.
The development of speech recognition technology is a journey. Early systems were often tied to a specific frame size mainly due to the limitations of the hardware and software back then. Fortunately, as technology has improved, we can adapt frame sizes more readily and adjust them depending on the application. This flexibility lets us tailor the processing more specifically to certain tasks.
For example, if speed is the primary concern in a live transcription app, a smaller frame size might be the preferred choice, even if that means taking the risk of a slight reduction in audio quality. It's a balancing act between the responsiveness needed and the quality of the transcript.
However, we must consider this alongside other settings, such as the overall sample rate of the audio file, how many audio channels we're using, and the overall recording environment. These settings all interplay with each other to affect the result. It's not just a matter of one setting, but an interaction.
Researchers have been exploring different techniques to optimize frame size, including dynamic adjustment in real-time based on the actual audio. The idea is that the system can intelligently choose the best frame size to handle different kinds of sounds. While promising, there are open questions on how this will interact with other elements in the processing flow.
Further, the user experience is impacted by frame size and latency. If a user interacts with a virtual assistant and there is too much delay, the user might find it less desirable. The faster the assistant seems to respond, the more valuable it can become.
And finally, we are increasingly questioning if standard frame sizes are really the best approach for all cases. In situations where high accuracy is required, such as in medical or legal transcription, we might find benefits in breaking away from the 'common wisdom' and trying more experimental techniques and frame sizes. This remains an area of ongoing research and development.
In conclusion, frame size is a crucial, yet often-overlooked aspect of maximizing speech-to-text accuracy. It's about achieving that balance between speed, accuracy, and responsiveness, which are crucial for real-world uses. The ever-evolving nature of audio processing and artificial intelligence algorithms will continue to push the boundaries on what we can achieve in this area.
Maximizing Accuracy 7 Key Audio File Settings for Better Speech-to-Text Conversion - FLAC And WAV Format Selection For Lossless Quality
When deciding between FLAC and WAV for preserving audio without any loss of information, several factors come into play. FLAC uses a technique called lossless compression to shrink file sizes. This can reduce the space needed by up to 60% without impacting the audio quality. WAV, on the other hand, stores the audio without any compression. While WAV files may provide a slight edge in audio fidelity, they come with the drawback of taking up considerably more storage space.
If you're working with large audio collections, FLAC's compression might make managing and storing the files easier. However, situations like audio editing may favor WAV. When you edit audio, preserving the exact data of the original file is crucial. Lossless compression methods used in FLAC can potentially introduce very slight issues or glitches when audio edits are involved, so, for editing purposes, WAV is generally the safer choice.
In essence, the selection of FLAC versus WAV should be carefully considered in light of the intended use for the audio. For most speech-to-text applications where size is a concern, FLAC would likely be more suitable. If editing is paramount or preserving the most accurate possible audio details is desired, then WAV is a reasonable option. It’s a balancing act between the benefits of reduced file size and maintaining the most accurate audio detail.
When deciding between FLAC and WAV formats for achieving lossless audio quality, several factors come into play. FLAC, or Free Lossless Audio Codec, uses compression to reduce file size without sacrificing audio quality, often achieving a 30-60% reduction compared to uncompressed WAV files. This is a significant advantage, particularly when dealing with large audio collections or projects where storage space is a constraint. Additionally, FLAC supports metadata, such as album art and artist information, making it a more versatile choice for managing audio libraries.
WAV, in contrast, is an uncompressed format, which results in larger files but offers faster decoding speeds. This can be beneficial in scenarios demanding rapid processing, like real-time transcription. It's also worth noting that WAV can theoretically handle higher sample rates without compression artifacts, making it more suitable for high-fidelity applications where absolute precision is paramount, like professional audio studios.
However, WAV's lack of built-in error correction can be a drawback. Corruption or damage to a WAV file might go unnoticed without a manual check, whereas FLAC incorporates error detection and correction, ensuring audio integrity. Additionally, FLAC can encounter compatibility issues with older hardware or software, whereas WAV tends to have broader support across different systems.
From a user perception standpoint, FLAC is often seen as the preferred format for archiving due to its inherent lossless compression. Conversely, WAV is viewed as a more raw, unprocessed representation of the audio. Both formats can technically achieve pristine audio quality, but these perceptions can drive format selections in certain fields.
It's important to recognize the subtle differences in how dynamic range is handled. WAV's uncompressed nature supports very high dynamic ranges, beneficial for capturing complex musical soundscapes. FLAC's compression, while preserving a very high range, can slightly limit this potential compared to WAV.
Finally, it's worth acknowledging that software applications have increasingly optimized their algorithms for FLAC, resulting in better performance in many cases. This can lead to faster processing times and even potentially improve the accuracy of transcriptions in speech-to-text applications when utilizing FLAC-formatted audio.
Ultimately, the choice between FLAC and WAV depends on the specific needs of the application. For many transcription-focused scenarios, especially those where storage efficiency is paramount, FLAC can offer significant benefits. Conversely, WAV's uncompressed nature can be vital when utmost fidelity and speed are desired, like in professional recording or live audio contexts. Understanding these nuances allows researchers and engineers to make well-informed choices for optimal audio handling in their projects.
Maximizing Accuracy 7 Key Audio File Settings for Better Speech-to-Text Conversion - Noise Gate Threshold Values For Background Sound Reduction
When aiming for accurate speech-to-text conversion, effectively managing background noise is essential. Noise gates are a tool for achieving this by selectively allowing certain audio levels to pass through while silencing others. Determining the right noise gate threshold values involves a careful balancing act. The threshold that closes the gate (the "close threshold") should be set just above the ambient noise level, often falling between -60 dB and -32 dB. This helps to eliminate low-level background sounds. Conversely, the threshold that opens the gate (the "open threshold") should ideally be around 8 dB higher than the typical volume of speech, roughly around -38 dB. This ensures that the speaker's voice is not cut off or distorted by the gate's action.
However, finding the optimal settings isn't a one-size-fits-all solution. The specifics of the environment—whether it's a quiet studio, a bustling office, or outdoors—influence how these thresholds should be adjusted. For instance, in a space with consistent humming or buzzing, you might find that incorporating a low-cut filter within the noise gate process helps eliminate this repetitive sound. The frequency of the hum would guide the filter's setting, which are usually around 60 Hz. By fine-tuning these thresholds and considering environmental factors, you can drastically improve the quality of the audio input to the speech-to-text system, resulting in more accurate transcriptions. It's a delicate process of eliminating unwanted noise while retaining the clarity of the intended speech. While tools can assist in this process, they aren't a magic fix for poor recordings. Dealing with noise issues at the point of recording is often a more effective solution.
The effectiveness of noise reduction, particularly through noise gates, hinges on carefully selecting threshold values. These values act as a filter, determining what levels of audio are allowed to pass through. Setting the threshold too high might inadvertently silence parts of the speech signal, especially softer sounds, while a value that's too low could let unwanted background noises pollute the audio. Finding the optimal balance is essential for clear and accurate transcription.
The dynamic range of an audio recording—the difference between the loudest and quietest parts—is a key factor in setting noise gate thresholds. Recordings with a larger dynamic range can make it more challenging to control noise. Background noises are often amplified, potentially overlapping with softer parts of speech. This highlights the need for careful adjustment to isolate the desired speech signals and minimize unwanted noise.
Some more modern noise gates utilize adaptive thresholding, a dynamic process that constantly monitors the audio input and automatically adjusts the noise gate settings. This technique can adapt to changing sound environments, automatically optimizing the gate based on current conditions. This adaptability can improve overall speech clarity and transcription accuracy, reducing the need for manual adjustments.
The audio environment itself has a profound influence on threshold values. In a quiet studio setting, more aggressive noise gate settings might be suitable since background noise is minimal. However, for recordings in locations with more background noise like a cafe, lower thresholds might be necessary to capture all relevant speech sounds without inadvertently clipping the audio. This reinforces the importance of considering the specifics of each audio recording when establishing noise gate settings.
The impact of noise gates is not uniform across all frequency ranges. Higher frequencies, often associated with the clarity and intelligibility of human speech, can be masked by lower frequency noise if the threshold values aren't thoughtfully selected. For instance, unwanted low-frequency hum can obscure important high-frequency speech sounds. Addressing this imbalance can preserve important nuances of speech and enhance the overall accuracy of speech-to-text models.
Noise gate threshold configurations are also relevant to how well machine learning algorithms are trained for speech recognition. If background noise is not adequately removed, it can introduce unintended patterns into the training data. These patterns may confuse or mislead the algorithm, potentially leading to poorer speech recognition performance in real-world situations.
Applying noise gates can introduce latency, or a delay in processing. If the threshold values aren't carefully considered, this delay can be increased. In real-time applications, like live transcription, even a few milliseconds of latency can impact the experience, potentially leading to frustrating lags or interruptions.
There's a notable interaction between noise gates and automatic gain control (AGC). AGC adjusts audio volume, but when the noise gate settings are poorly matched, it can create an unnatural audio output. This unnatural processing can make it harder for listeners or speech recognition systems to interpret the speech, resulting in lower accuracy and overall understanding.
The human perception of sound is also susceptible to noise gate settings. A noise gate may function perfectly from an objective viewpoint but, to a listener, create an unnatural audio experience. Speech can sound choppy, mechanical, or processed. This can lead to listener fatigue when exposed to processed audio for extended periods, so it is important to consider both technical efficiency and human perception.
To maintain consistent high-quality transcriptions, regular testing and recalibration of noise gate threshold values is important. The best settings in one environment might be completely inappropriate in another. Background noise intensity, speaker distance, and a multitude of other factors can influence the optimal threshold setting, emphasizing the need for adaptive noise reduction strategies.
Maximizing Accuracy 7 Key Audio File Settings for Better Speech-to-Text Conversion - Custom Dictionary Implementation For Technical Terms
When dealing with audio containing technical language, a custom dictionary can significantly boost the accuracy of speech-to-text conversion. Speech recognition models, at their core, rely on recognizing common words and phrases. However, technical fields often use specialized terms and jargon that standard models may not be trained on, leading to errors or misinterpretations. By building a custom dictionary, users can essentially teach the system the specific vocabulary used in their industry. This involves creating a list of relevant terms and their pronunciations.
It's important to note that while some speech-to-text services offer a means to create and upload custom dictionaries, it's not always as simple as just providing a word list. Some services, like those offered by Microsoft, involve building a full custom speech model, often starting with a base model and then including a phrase list. It's worth exploring how each service handles this, as there might be limits or special considerations. It seems some platforms limit phrases to 500 or fewer within a custom dictionary. This likely impacts how quickly or accurately models can adapt. The creation of a custom dictionary or model will likely need to be reviewed for proper construction.
If there are limitations with creating custom dictionaries within a specific transcription service, investigating other methods is worthwhile. Fine-tuning larger language models, like those offered by OpenAI's Whisper model, is another approach that could potentially be used. These types of models are often very capable in diverse language transcription, but it might be necessary to further train them on a dataset containing the specific vocabulary to be used.
In essence, custom dictionaries and trained models represent a growing area for improving the accuracy of speech-to-text systems, particularly in contexts where technical terminology is prevalent. The goal is to achieve more accurate transcriptions, ideally producing output with minimal errors related to domain-specific jargon. However, the development of reliable and effective custom solutions for speech-to-text is still in progress, with continuous research and development needed to improve accuracy and ease of use.
Custom dictionaries specifically designed for technical terms can significantly improve the accuracy of speech-to-text systems in specialized domains. By incorporating a custom dictionary, the speech recognition system gains a much deeper understanding of the specific vocabulary and phrases used within a particular field, leading to a greater level of accuracy. It appears that using a well-designed custom dictionary can boost accuracy by over 20% in areas like engineering or medicine, where technical jargon is prevalent.
Building a custom dictionary usually involves setting up the speech recognition component without specifying a language initially. This setup allows for the inclusion of domain-specific words and terms. Services like Microsoft Azure's Custom Speech give users the ability to train custom models by providing their own datasets, which they can then compare to see which models yield the most accurate results and deploy to a specific area in the system.
Interestingly, these custom speech models often use a general language model as a foundation. These foundation models are typically trained on massive datasets owned by Microsoft. The improvement in accuracy primarily comes from providing a list of phrases particularly relevant to the specific application. However, there's a limitation; the number of phrases you can include in this list is capped at 500. Nevertheless, incorporating a phrase list tailored to the specific technical vocabulary can significantly enhance accuracy.
While models like OpenAI's Whisper demonstrate a remarkable ability to accurately transcribe speech across multiple languages, they sometimes struggle when encountering technical terminology not found in their initial training data. However, it is possible to enhance the Whisper model's ability to understand specific jargon by providing it with a custom training dataset specific to the field. This technique essentially teaches the model the relationship between audio signals and their corresponding specialized terms.
Creating a custom vocabulary for speech-to-text transcription can involve preparing a file that includes the terms and their corresponding pronunciations. This process can help improve accuracy by providing the speech-to-text system with clearer guidelines for recognizing and interpreting specific words and phrases.
It's easy to see how improvements to speech-to-text accuracy could be immensely helpful for companies. Accurate transcription of audio data enables companies to automatically generate insights from voice conversations. The benefits for communication and efficient operations are clear.
While the search results highlighted various settings affecting the quality of speech-to-text conversion, they didn't dive into specifics. This suggests there's a need for more research on fine-tuning the settings to achieve optimal results. It appears that there are some areas where a more in-depth understanding of this interplay is lacking.
There are some interesting, if somewhat obscure, implications to consider. Custom dictionaries could possibly lead to a reduction in the complexity of the acoustic model that's required. Because the training data is focused only on specific terms, it's possible the processing load on the system could decrease. This, in turn, could make custom speech recognition applications more efficient. There is also potential for speech recognition systems using custom dictionaries to employ adaptive learning techniques. This means that the dictionary could be refined over time with user feedback, ultimately making it more accurate as it encounters new vocabulary. And custom dictionaries can also bridge languages. Using a custom dictionary allows for multilingual support, which could make it possible to incorporate domain-specific terms across a variety of languages. This capability is growing increasingly important as global communication relies more on technical terms in a diverse set of languages.
Of course, integrating custom dictionaries isn't without its challenges. While custom dictionaries offer many advantages, proper integration is critical to avoid potential issues. For example, if a custom dictionary is not well-aligned with the underlying models or datasets, it can introduce discrepancies and lead to increased errors or outputs that are difficult to interpret. Further, it is important to note that these benefits are observed in specific research. It would be interesting to conduct studies to further evaluate these assertions in the broader community.
More Posts from transcribethis.io: