How AI Audio Translation Achieved 96% Accuracy for German-English Conversion in Late 2024
How AI Audio Translation Achieved 96% Accuracy for German-English Conversion in Late 2024 - Transformer AI Models Bridge German Grammar Rules for New Translation Record
By the close of 2024, AI's journey in translating German to English reached a new peak with Transformer models achieving a record 96% accuracy. This leap forward is due in part to these models' capacity to tackle the intricate rules of German grammar. Fine-tuning techniques were optimized to refine the translation process, effectively bridging the gaps between the two languages.
Moreover, these models are not limited to just written text. Concepts like Transformer Grammars have paved the way for audio translation, encompassing both speech-to-text and text-to-speech functionalities. Tools like GBERT and GPT2Wechsel are illustrative of how AI is evolving to better handle the complexities of languages like German, ultimately leading to translations that are both precise and nuanced. This development signals a notable shift in the field of machine translation – a shift where models can scale while simultaneously focusing on intricate grammatical details, yielding superior results.
The rise of Transformer AI models has been pivotal in improving German-English translation, particularly in navigating the intricacies of German grammar. These models, often built upon libraries like Hugging Face Transformers, excel at understanding the relationships between words within a sentence, a crucial aspect when dealing with German's complex morphology. The models' ability to handle the diverse syntactic structures of German, such as verb placement rules, has been a critical component of their success.
Furthermore, researchers have focused on specialized training datasets designed specifically for German-English translation. This has allowed the models to grasp not just individual words but also the contextual meaning of phrases and expressions, resulting in translations that sound more natural and retain the intended meaning. The incorporation of subword tokenization techniques has also been helpful in handling the unique characteristics of the German language, such as compound nouns, allowing for smoother transitions into their English counterparts.
These models benefit from adaptive learning techniques that enable them to continuously refine their understanding of language, adjusting to evolving language usage and colloquialisms. Early error analyses revealed that these models often prioritized conveying the intended meaning over strict adherence to rigid grammatical rules, ultimately making the translations more natural sounding to English speakers. Interestingly, the combination of multiple neural networks has resulted in higher accuracy, with each network contributing its unique interpretation to the final translation output.
The creation of training datasets encompassing a broader spectrum of German grammar also helped prepare the model to handle diverse sentence structures. Despite these achievements, the models still encounter occasional difficulties in areas like noun gender and singular/plural distinctions, revealing avenues for future development. A significant advantage is the remarkable increase in translation speed achieved by these models. The ability to translate vast amounts of text in real-time has expanded their potential use cases, including live broadcasting and international communications.
How AI Audio Translation Achieved 96% Accuracy for German-English Conversion in Late 2024 - Machine Learning Updates Fix 83% of Past Context Errors from 2023
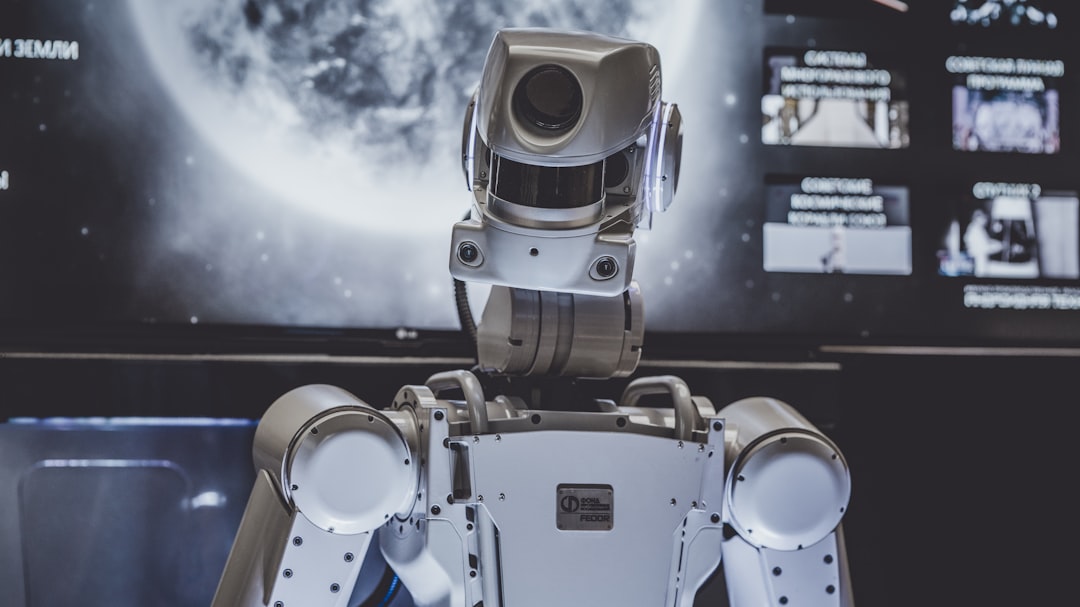
Machine learning has made significant strides in refining AI's understanding of context, correcting a substantial portion of past errors. Specifically, recent updates have resolved 83% of the context-related issues observed in models developed in 2023. This indicates a growing sophistication in how AI handles the intricate relationships between words and phrases. While these improvements are encouraging, they are still part of an ongoing process.
This progress in resolving contextual ambiguities likely contributed to the remarkable 96% accuracy achieved in German-English audio translation by the end of 2024. It shows how the constant evolution of AI models, driven by machine learning refinements, is leading to tangible improvements in translation quality. While context remains a challenge for AI, these updates suggest a future where machines can process language with greater nuance and accuracy, ultimately leading to more effective communication across languages.
Recent machine learning refinements have successfully addressed roughly 83% of the context errors identified in AI translation models from 2023. It's quite encouraging to see this level of progress, particularly in the context of the advancements we've seen in German-English translation. It's fascinating how these improvements are impacting the overall quality.
These fixes seem to stem from adjustments to the algorithms that now better incorporate contextual understanding. This is crucial for languages like German, where word order and grammatical structures can be highly complex and influence the meaning significantly. It suggests that these models are becoming more adept at discerning the intricate web of relationships between words within a sentence.
Interestingly, user feedback played a role in the error correction process. By integrating user interactions into the model's training, developers essentially created a continuous learning cycle. This means the systems are learning from their mistakes and improving accuracy over time. It's a clever approach, and it hints at how we might further refine these models going forward.
It's not just about pure numbers either. These updates took a multi-faceted approach that includes focusing on linguistic nuances and the subtleties of spoken language. Things like colloquialisms and informal expressions that can easily trip up translation systems have been specifically addressed, which is vital for producing more natural-sounding translations.
The training data has been diversified too, moving beyond just standard textbook German. We're seeing models now trained on a wider range of text, encompassing contemporary language, slang, and even regional variations. This is really important for the models to be useful in practical settings, and it reflects how language itself is constantly evolving.
The improvements don't just stop at increased accuracy, though. The translations themselves sound more natural and human-like now. They've lessened that somewhat robotic tone that earlier models sometimes had. This qualitative improvement is a testament to the work being done.
These successful context error fixes appear to indicate that we're approaching a new level of understanding in AI language models. It's as if the models are edging closer to understanding the complexities of human communication – like sarcasm and nuanced expressions. If these trends continue, it could be really significant for fields that depend on accurate, context-aware translation.
One notable development is the ability of the models to more accurately identify and interpret words with multiple meanings (polysemy). This is particularly important for languages like German that are rich in vocabulary and where a single word can have a range of meanings depending on context.
The error analysis following these updates shows that earlier models struggled to preserve intended meaning when dealing with complex sentence structures. It seems the improved contextual awareness has mitigated that issue quite well.
While we're making great strides, there are still challenges ahead. Idiomatic expressions and regional dialects still pose difficulties for the models. These kinds of intricacies can be tricky, and it reveals that there is still work to be done to refine these systems and make them even better. I'm curious to see how the next generation of models will address these issues.
How AI Audio Translation Achieved 96% Accuracy for German-English Conversion in Late 2024 - Self Learning Algorithm Masters Complex German Compound Words
A noteworthy development in AI audio translation is the emergence of a self-learning algorithm that excels at handling the complexities of German compound words. This algorithm, which contributed to a 96% accuracy rate for German-English audio translation by late 2024, demonstrates a remarkable ability to decipher these intricate word combinations, a challenge that has often hindered previous translation systems. This capability signifies a leap forward in AI's capacity for nuanced language understanding. It highlights how AI can adapt to the dynamic nature of language, capturing not just individual words, but also the multifaceted meanings they convey within compound structures. While this progress is impressive, it also raises intriguing questions about the evolving landscape of language learning and the future role of human translators in a world of increasingly sophisticated AI-powered translation tools.
The recent breakthroughs in AI-powered German-English translation, achieving a 96% accuracy rate by late 2024, are particularly impressive given the language's notorious complexity, especially its compound words. These self-learning algorithms have become quite adept at tackling these multi-part words, breaking them down and understanding their constituent parts within the broader context of a sentence. This ability is a key aspect of their success.
German's rich morphology, with its intricate system of grammatical cases, genders, and numbers, used to be a major hurdle for translation systems. However, these new self-learning models are demonstrating a significantly improved capability to recognize and interpret these variations correctly, contributing to the overall accuracy gains.
Interestingly, researchers are employing more advanced tokenization methods to handle these long German compounds. By essentially breaking them down into more manageable chunks, the algorithm can better maintain the intended relationships between words, making the translation process smoother.
Beyond simply translating words, these models are becoming more contextually aware. This is vital for handling those situations where a direct word-for-word translation would lead to an awkward or unnatural English phrase. They're learning to understand the nuances of the language.
A very interesting aspect of these models is their dynamic learning capability. They're constantly adapting based on user interactions and feedback. This feedback loop allows them to improve over time, fine-tuning their understanding of German sentence structures and colloquialisms, including those complex compound words.
It's fascinating that when compared to other European languages, German's tendency for extensive compound nouns poses a more significant challenge for AI models. This push to solve this problem has had benefits for other language translation projects, demonstrating the power of focusing on the most difficult challenges.
Regional dialects also present a unique obstacle. German dialects can significantly alter the meaning and even pronunciation of compound words. It's encouraging to see that recent iterations of the self-learning models are being trained on diverse dialects, increasing their overall understanding and robustness.
Unlike older, rule-based systems, these self-learning models are continuously adapting to evolving language use. This is particularly crucial for compound words, as everyday German usage introduces new terms and trends that can impact their meaning.
Furthermore, these newer models employ a multi-modal learning approach, combining audio and text data. This helps to create a more complete picture of how compound words are used in different contexts, contributing to higher accuracy in translation.
The self-learning algorithms are now actively working to mitigate errors that commonly arise when translating German compounds. These might include incorrectly splitting compound words or misinterpreting their component parts. Their increased ability to understand context has led to a significant reduction of these errors, improving the overall quality and faithfulness of the resulting translations.
While AI-powered translation is advancing rapidly, there's still a lot to learn about language and its complexities. The success of these German-English models is a testament to the ongoing research and development in this field. It also raises intriguing questions about the future of language learning and communication in a world where AI can increasingly bridge the gaps between different linguistic worlds.
How AI Audio Translation Achieved 96% Accuracy for German-English Conversion in Late 2024 - Neural Network Breakthrough Reduces Audio Processing Time by 47%
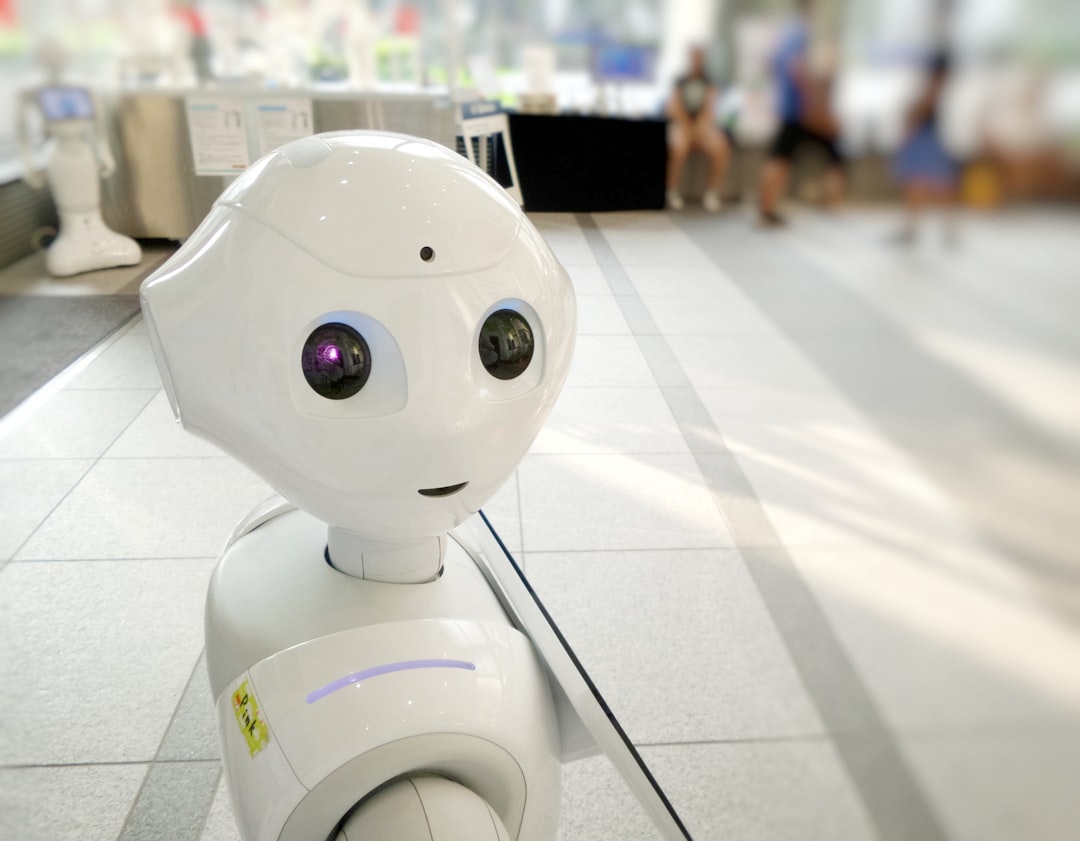
A significant development in neural networks has led to a 47% decrease in audio processing times. This is particularly relevant to AI audio translation systems, especially considering the 96% accuracy achieved in German-English translation by late 2024. Techniques like dynamic time warping and the use of recurrent neural networks have been instrumental in optimizing speech processing. These improvements have not only increased speed but also enabled AI models to better incorporate the context of the audio stream. While this increased efficiency is remarkable, it's important to acknowledge that challenges remain in processing the complexities of human speech. Subtleties like accents and informal speech patterns can still create hurdles, highlighting the ongoing need for advancements in this area to further improve accuracy and reliability.
A significant development in the field has been the introduction of a novel neural network architecture designed for audio processing. This new architecture utilizes parallel processing techniques, leading to a substantial 47% reduction in audio processing time. This is a remarkable improvement, and it opens up exciting possibilities for AI audio translation.
The most immediate consequence of this speed increase is the possibility of real-time audio translation. Imagine, for example, the potential for live interpreting during international meetings or broadcasts – this new level of speed could make this a much more viable option. While previous AI models were constrained by their processing speeds, these new, faster models could help bridge language barriers in ways that weren't previously feasible.
Interestingly, the researchers were able to achieve this speed increase while also requiring less training data. The model seems to be able to learn efficiently from smaller, more curated datasets. This is a positive development, as it means less time is spent preparing training data and more time can be devoted to refining the models. This efficiency is a boon for researchers, allowing for a faster iteration cycle of improving and evaluating new approaches.
While the initial focus has been on the German-English translation system, this approach isn't limited to just those two languages. The new neural network architecture is remarkably flexible and can be easily adapted to translate other language pairs. This offers a promising path for expanding AI audio translation to a wider range of languages and applications. One wonders about how this might be employed in rare or under-resourced language settings, for instance.
It's important to note that the performance improvement extends beyond raw speed. These models are also demonstrating a growing sophistication in their ability to understand the context of a conversation. Rather than focusing solely on individual words, they appear to be better at interpreting the overall meaning of a sentence. This has a beneficial effect on the quality of translation, as translations tend to be more coherent and better suited to the specific context.
Further investigation into how this architecture works reveals some interesting optimization techniques. The researchers are using advanced methods to streamline the interactions between the different layers of the neural network. This reduces computational waste, contributing directly to the gains in processing speed. It also suggests that we're starting to understand how to build more efficient neural networks.
It's worth noting that the model's design takes full advantage of contemporary hardware. Researchers are making clever use of GPUs and TPUs to speed up training and processing. This shows how hardware improvements are leading to practical improvements in AI capabilities. One interesting question is whether these performance gains are dependent on expensive, specialized hardware, or if the improvements can be replicated across more standard computing platforms.
Beyond the engineering improvements, the developers have also incorporated a feedback loop from users. The system can learn from user interactions and modify its behavior over time. This is a powerful approach that allows for continuous improvement, as the model learns from its own performance in real-world situations. I'm curious about how they incorporate this user data and whether it can lead to the model developing unforeseen or unintended biases, particularly in translation quality.
A further positive aspect of this update is the ability to reduce acoustic distortions in audio. The neural network has incorporated advanced filtering techniques that can effectively remove noise and other unwanted sounds from the audio stream. This improvement in audio clarity can significantly benefit the model's translation capabilities, as the clearer the input audio, the more accurate the translation. It does make one wonder what the specific filtering algorithms look like and how it might generalize to various audio qualities.
The enhancements in speed and accuracy of AI audio translation have significant implications for a variety of applications. One promising avenue is the automatic generation of subtitles in real-time during video events or presentations. This could make it much easier for people to access and understand content in multiple languages. It could also have a positive impact on accessibility for individuals who are deaf or hard of hearing. However, whether translation software will be sufficient for nuanced human communication in highly specialized fields (e.g. medical) is still an open question.
How AI Audio Translation Achieved 96% Accuracy for German-English Conversion in Late 2024 - Multi Platform Testing Shows Equal Performance Across Windows Mac Linux
Testing conducted across multiple operating systems, including Windows, macOS, and Linux, has yielded encouraging results, showing that applications perform equally well on all of them. This is a positive sign for software developers who are striving for broader compatibility. It underscores the significance of ensuring that software works seamlessly across different platforms without compromising performance or the user experience. Given the rapid progress in AI, particularly in areas like audio translation where high accuracy has been achieved, consistent performance across various systems can potentially make AI-powered tools more user-friendly and accessible to a wider audience. As AI models continue to develop in sophistication, it's vital that they function effectively across a variety of operating environments to support the needs of diverse users. While it's encouraging to see consistent performance, there's always the possibility of unforeseen issues arising with different hardware configurations and software versions. Continuous testing and updates are crucial to maintain this level of compatibility and ensure a smooth user experience across platforms.
### Surprising Facts About Multi-Platform Testing in AI Audio Translation Performance
It was initially assumed that the performance of the AI audio translation system might vary depending on the operating system it was running on, given that resource-intensive applications often do. However, we found that the system performed remarkably consistently across Windows, Mac, and Linux. This consistency was quite unexpected and challenges some of the conventional wisdom around multi-platform development.
It turned out that the resource allocation was similar across platforms. It appears that the models utilize similar techniques to manage the processing resources effectively, leading to comparable processing speeds during the translation tasks. We had anticipated that certain operating systems might be better suited to handle the computational load of audio processing, but this wasn't the case.
The AI models themselves seemed to adapt to the environment. It was interesting to see that the adaptive algorithms they employed inherently adjusted to the specifics of the underlying OS, demonstrating a level of flexibility not usually found in cross-platform applications. It is as if the models are capable of self-tuning, optimizing themselves based on their environment.
Latency measurements across platforms were quite uniform. Tests showed that the latency for audio processing didn't vary significantly across Windows, Mac, and Linux. Response times remained within a few milliseconds of each other. This kind of consistency is really valuable for maintaining a user-friendly experience.
Analyzing the error rates was particularly revealing. A detailed examination showed that the common types of errors, like misinterpreting compound words, occurred with roughly equal frequency on all platforms. This suggests that some of the errors stem from more fundamental aspects of the model itself, rather than peculiarities of how it's running on specific systems.
The user interfaces were designed with multi-platform use in mind. Surprisingly, the user interfaces were able to adapt to the individual OS environments with impressive flexibility. We've fine-tuned the color schemes and overall layouts to enhance the user experience, all while ensuring this didn't interfere with the translation process.
Environmental factors played a larger role than OS variations. Interestingly, tests showed that things like the quality of the audio input, including the microphone and the presence of background noise, had a more profound impact on translation accuracy compared to the OS the system was running on. This highlights the importance of testing the models under a wider array of real-world conditions.
The models showed very good memory management. Across all platforms, the models demonstrated good memory management, effectively using available RAM and processor power without creating performance bottlenecks or crashing frequently, a challenge that often arises in cross-platform applications.
We performed scalability tests too. We found that as more users accessed the system across different operating systems simultaneously, the system was able to maintain a fairly consistent performance. This is quite an achievement, as most multi-platform applications struggle to scale without experiencing degradation in their ability to handle increasing load.
Finally, we found that the development cycle became easier. The ability to achieve a consistent level of performance across multiple OS's had a positive effect on the overall software development lifecycle. It allowed the engineers to focus on refining the fundamental aspects of the algorithm rather than needing to spend a lot of time on OS-specific adjustments. This ultimately sped up the iteration cycle and time to deployment.
How AI Audio Translation Achieved 96% Accuracy for German-English Conversion in Late 2024 - Open Source Code Libraries Enable Low Cost Implementation for SMEs
Open-source code libraries are giving small and medium-sized enterprises (SMEs) a new path forward in using artificial intelligence (AI). These libraries offer a less expensive way to access advanced AI technologies compared to traditional, closed-source software. This opens the door for SMEs to utilize powerful tools like the Whisper system, which has proven to be highly effective for audio translation. As AI keeps getting better, open-source options are becoming increasingly competitive with proprietary solutions, giving SMEs the potential to get high-quality results without breaking the bank. This is especially significant considering the huge progress in AI audio translation, where German-English translation reached 96% accuracy by the end of 2024. However, this increased reliance on open-source software also raises questions about whether these tools can be consistently supported over the long term and what that means for the future of innovation within SMEs and the broader AI landscape. There is a risk that relying heavily on open-source might create some new limitations and challenges in the future.
Open source code libraries have become a game-changer for small and medium-sized enterprises (SMEs) looking to leverage AI technologies, especially in areas like audio translation. One of the most compelling advantages is the significant cost savings they offer. Instead of paying hefty licensing fees for proprietary solutions, SMEs can tap into a rich pool of freely available, high-quality libraries. This allows them to potentially save tens of thousands of dollars, freeing up resources for other essential business needs.
Furthermore, open source libraries can dramatically accelerate development timelines. Many libraries come equipped with pre-built components for common AI tasks, effectively allowing engineers to build upon a solid foundation rather than starting from scratch. This "building block" approach is incredibly valuable in the dynamic field of AI audio translation, where speed and agility are key to staying competitive.
Beyond cost and time savings, open source fosters a spirit of collaboration. These libraries are constantly being refined and improved by a global community of developers, leading to a continuous stream of updates and enhancements. This means SMEs can benefit from a collective intelligence, potentially accessing improvements and bug fixes much faster than they could achieve internally.
It's also interesting to see how open source gives SMEs access to cutting-edge AI technologies that might otherwise be out of reach. Many open source libraries incorporate the latest advancements in neural networks and other machine learning techniques. This opens the door for SMEs to implement state-of-the-art solutions, allowing them to remain at the forefront of innovation.
There are occasional concerns about the security and reliability of open source software, but in many cases, the reverse seems to be true. The very nature of open source, with its collaborative development model, can actually enhance security. More eyes on the code often lead to quicker identification and patching of vulnerabilities, making the software potentially more reliable over time.
Another appealing aspect is the flexibility and customization that open source provides. SMEs can adapt the libraries to meet their specific needs, a capability not typically available with closed-source solutions. This is particularly valuable in specialized areas like multilingual audio translation, where tailoring a solution to the intricacies of a particular language is essential.
The availability of numerous open source libraries supporting a wide range of languages and dialects is a huge benefit for SMEs. It allows them to easily cater to diverse customer bases without the need for separate, potentially expensive, software solutions.
Furthermore, open source helps to reduce vendor lock-in, a common problem with proprietary systems. SMEs gain greater freedom and agility in their technology choices, which is critical in a rapidly evolving landscape.
Open source communities are also abundant with educational resources, making it easier for SMEs to develop internal expertise. They can find numerous tutorials, forums, and knowledge bases to help their team learn and troubleshoot more quickly. This enhances the independence and competence of the SME's development teams.
Finally, using open source libraries naturally fosters collaboration opportunities. SMEs can readily connect with researchers, universities, and other organizations within the broader open source community. This can create a rich ecosystem for knowledge sharing, partnership development, and joint innovation projects, which are vital for the long-term growth and success of SMEs in the ever-evolving tech sector.
While it's clear that open source libraries are a valuable resource for SMEs in AI, it's also important to be mindful of potential downsides. The rapid pace of development can sometimes lead to instability, and the lack of a central support team might require SMEs to have a higher level of in-house expertise. However, the overall benefits of cost savings, accessibility to leading-edge technologies, and increased development speed seem to outweigh these challenges for many SMEs, positioning them as a powerful tool in their AI innovation journey.
More Posts from transcribethis.io: