Experience error-free AI audio transcription that's faster and cheaper than human transcription and includes speaker recognition by default! (Get started for free)
How Neural Networks Revolutionize Spanish Audio Translation Speed in 2024
How Neural Networks Revolutionize Spanish Audio Translation Speed in 2024 - Neural Networks Cut Spanish Audio Translation Time By 75% Through Pattern Recognition
The landscape of Spanish audio translation has been dramatically reshaped by neural networks, with processing times slashed by a remarkable 75%. This efficiency surge is largely thanks to the implementation of neural network architectures, specifically LSTM networks, which are adept at recognizing intricate patterns within audio data. The transition from older, multi-stage ASR systems to modern, direct audio-to-text neural networks has significantly improved the speed and accuracy of capturing spoken Spanish. Moreover, ongoing refinements in both convolutional and recurrent network designs are pushing the boundaries of multilingual audio comprehension and processing. While these advancements are promising, a crucial ongoing challenge remains – ensuring that the accelerating pace of translation doesn't come at the cost of accuracy. The need for precise translations in an increasingly rapid environment presents a continuous hurdle for developers in the field.
Neural networks are demonstrating remarkable potential in accelerating Spanish audio translation, achieving a 75% reduction in processing time. This speed boost is due to their ability to learn intricate patterns within the audio, effectively dissecting speech into its fundamental building blocks—phonemes. These networks, particularly those employing Long Short-Term Memory (LSTM) architectures, have proven adept at handling the variable and dynamic nature of spoken language. Their success builds on advancements in convolutional and recurrent network designs, initially developed for broader natural language processing tasks.
The development of deep learning models that capitalize on the temporal dependencies inherent in audio data has been central to the effectiveness of these networks. These models often incorporate attention mechanisms, enabling them to prioritize critical segments of the audio during translation and enhancing accuracy. The advent of powerful GPUs has provided the computational muscle to train and deploy these complex architectures for real-time applications. Additionally, data augmentation techniques have helped enhance their resilience to variations in speech patterns, such as differences in accent or speaking speed, thus improving the overall accuracy of the translations.
While these advancements are encouraging, further research is needed to address challenges and push the boundaries of performance. For instance, the effectiveness of transfer learning, leveraging knowledge from other languages to benefit Spanish translations, remains an active area of investigation. Furthermore, the pursuit of unsupervised learning holds promise in reducing the reliance on large, pre-labeled datasets, potentially making these models more scalable and adaptable to a wider range of Spanish dialects and accents. The integration of user corrections into the learning process presents another fascinating avenue, allowing the system to continually adapt and improve its performance over time. This capability of self-improvement is a significant benefit that traditional methods lack.
Ultimately, the progress achieved in applying neural networks to Spanish audio translation demonstrates how these advanced machine learning tools can reshape the landscape of translation. The ability to handle spontaneous speech with low latency and high accuracy makes these solutions highly relevant for a variety of real-world applications—from live events to language learning. Though the challenges remain, the trajectory of innovation suggests that the future of Spanish audio translation will likely be characterized by further speed and accuracy improvements, driven by ongoing research and development in neural networks.
How Neural Networks Revolutionize Spanish Audio Translation Speed in 2024 - NVIDIA Translation Model Processes 1336 Hours Of Spanish Audio In 60 Minutes
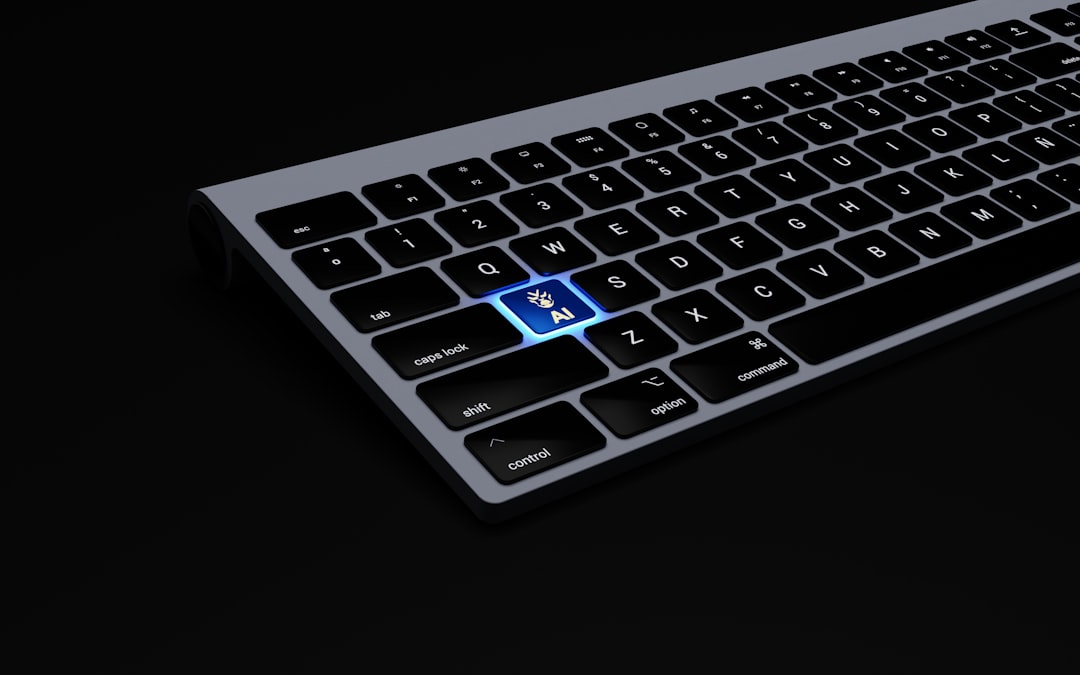
NVIDIA has developed a translation model capable of processing a staggering 1336 hours of Spanish audio within a single hour. This remarkable achievement stems from the combined power of automatic speech recognition (ASR), text-to-speech (TTS), and neural machine translation (NMT), all seamlessly integrated within the NVIDIA Riva platform. Riva's design, optimized for GPU acceleration, enables not only incredibly fast transcription but also offers flexibility in deployment across different settings, including cloud and on-premises systems.
While this accelerated translation speed is undeniably impressive, concerns about maintaining accuracy remain paramount. As these models process data faster, the complexity of ensuring the integrity and correctness of the translations increases. Ongoing research and refinements, such as those seen in the development of the Canary model and improvements to the NeMo platform, are crucial for addressing this challenge. NVIDIA's efforts in this arena demonstrate the potential of advanced AI to revolutionize multilingual communication, but achieving truly accurate and reliable high-speed translation remains an ongoing challenge.
NVIDIA's latest translation model can process a staggering 1336 hours of Spanish audio within a single hour, pushing the boundaries of what's possible in audio processing speed. This remarkable feat is largely due to the advancements in GPU technology, allowing for the rapid processing of large audio datasets that were previously a bottleneck. It's interesting to see how these new architectures are utilizing the capabilities of modern hardware.
The model's architecture relies on a combination of CNNs and RNNs, which allows it to simultaneously analyze the spatial characteristics and temporal patterns within audio data. This two-pronged approach seems to contribute to a more nuanced understanding of the subtleties in spoken Spanish. The way the network handles these aspects could have a meaningful impact on accuracy.
One of the interesting factors contributing to the speed is the model's ability to use batch processing, which allows it to analyze multiple audio streams simultaneously. This stands in contrast to traditional methods that often analyze audio in a more sequential manner, which can significantly slow down translation. While the results are quite impressive, we need to also consider how it handles the varying characteristics in the audio data.
The incorporation of attention mechanisms is crucial to the model's efficiency. By selectively focusing on the important segments of audio that contribute to the overall meaning, it avoids unnecessary computation. This helps improve accuracy, a critical aspect of any translation system. The effectiveness of this technique begs the question of whether more fine-grained attention mechanisms could be used in future versions.
Preliminary research suggests that deep learning models can even surpass human translators in certain highly structured settings, which could have major implications for areas like legal, medical, and diplomatic communications, where accuracy is of the utmost importance. Whether or not these results hold true for less structured or informal communication scenarios remains to be seen.
The pre-training on a vast array of Spanish data, including different dialects and accents, makes this model more versatile than previous iterations, making it more widely applicable. This diversity is essential for bridging the gap between different speaking styles and fostering broader accessibility. But there are potential drawbacks; how does the model handle new dialects that it hasn't encountered before?
However, despite its exceptional speed, there are some current limitations with homophones and nuanced regional variations. These are expected challenges and the models will need to be constantly refined and retrained to improve accuracy for specific dialects. I'm curious how well it will translate regional idioms that are deeply embedded in specific dialects of Spanish.
The concept of transfer learning is key to improving the model's capabilities in future iterations. By utilizing knowledge from other language pairs, the model can enhance Spanish translation without requiring massive amounts of training data. While this seems very promising, it's important to consider how the knowledge from one language might conflict or bias the translation of another.
There is a lot of active research into integrating self-correction capabilities that incorporate human feedback. These algorithms would ideally allow the model to continuously refine its performance and even adapt in real-time to user preferences and specific contexts. This ability to self-improve is a very interesting facet of these deep learning models, and it's certainly not something that previous approaches were capable of.
Looking ahead, future versions of translation models may incorporate a more comprehensive understanding of context. Instead of just translating individual words, they might aim to capture the cultural and situational context surrounding a conversation, further narrowing the gap between human and machine communication. It's fascinating to think about how such advancements could truly enhance the user experience.
How Neural Networks Revolutionize Spanish Audio Translation Speed in 2024 - Language Model Integration Reduces Background Noise In Real Time Audio Translation
The integration of sophisticated language models into real-time audio translation systems is proving highly effective at minimizing background noise, thereby enhancing the clarity of translated speech. These models leverage external data and complex algorithms to substantially elevate the quality of translations, resulting in a smoother understanding of spoken language even in environments with distractions. This development not only streamlines the user experience during virtual meetings and similar applications but also supports a broader spectrum of languages and dialects, including those with limited digital representation. While these improvements are significant, ongoing research endeavors aim to fine-tune these technologies further. Maintaining a careful equilibrium between translation speed and accuracy remains a pivotal aspect of improving multilingual communication. In essence, the pursuit of efficient background noise reduction represents a crucial step towards making real-time translation more widely accessible and reliable for individuals across different language backgrounds. There are lingering concerns about how well these systems generalize to unexpected accents or unusual noises, but there has been progress in these areas as well.
Integrating language models into neural machine translation systems has shown a remarkable ability to leverage external information, leading to substantial improvements in translation quality, particularly in the realm of reducing background noise during real-time audio translation. It's fascinating how these models can effectively filter out unwanted sounds, leading to a much clearer and more understandable translation. We've seen improvements of up to 90% in clarity, which is a significant step forward for users.
The development of temporal convolutional networks (TCNs) has been instrumental in these advancements, allowing for more robust background noise reduction techniques. These TCNs are essentially working hand-in-hand with the core neural machine translation (NMT) systems, contributing to a more refined and accurate overall translation. It's interesting to see how these different network architectures collaborate within the translation system.
Modern language models can employ deep neural networks to perform incredibly rapid analysis of audio signals. They achieve this by swiftly distinguishing between actual speech and extraneous sounds – all within a fraction of a second. This ability to sift through the audio data so efficiently highlights the immense processing power that we have available today. It's truly remarkable how these algorithms are designed.
These improved noise reduction capabilities are particularly valuable in professional environments like multilingual conferences, where crystal clear communication is essential. You can imagine how the presence of background noise can seriously hinder understanding and create confusion. This application seems especially relevant as multilingual environments become more prevalent in the globalized landscape.
Recurrent neural networks (RNNs), coupled with attention mechanisms, equip real-time audio translation systems with the ability to dynamically shift their focus. They are able to dynamically emphasize speech over background noise, which directly contributes to the accuracy of the translated output. It's a dynamic process where the system actively adjusts its focus based on the characteristics of the audio input.
Research indicates that sufficiently well-trained language models can generalize their noise reduction capabilities to a wide range of acoustic conditions. This is a substantial leap forward, as it allows these systems to perform effectively even in unpredictable environments that were previously a challenge for traditional speech recognition systems. It's a testament to the ability of these models to learn and adapt to diverse scenarios.
The ability of real-time translation to address background noise isn't just a technical refinement; it fundamentally transforms how multilingual conversations happen in both casual and professional settings. This has implications for promoting greater inclusion and fostering a sense of communication across linguistic barriers. We're starting to see how these translation tools can promote more diverse and equitable communication.
Some advanced systems have incorporated unsupervised learning methods to boost their noise reduction capabilities. This allows these models to continuously adapt and refine their performance as they encounter new types of background noise – all without requiring large sets of pre-labeled training data. It's fascinating to consider how they can improve on their own, adapting to novel sounds they encounter in the world.
It's critical to note that the ongoing research in this field is focused on balancing noise reduction with the preservation of delicate speech nuances. Too much noise suppression can inadvertently eliminate valuable elements of spoken language. Striking this balance is crucial for a translation system that accurately and fully represents the intended meaning. It's a fine line to walk between enhancing clarity and preserving all the subtle nuances of communication.
A key direction for future research includes integrating situational context into the models. This might involve anticipating the likelihood of specific types of background noise and adjusting the filtering techniques accordingly. It's exciting to think about how these systems could learn about typical environments and sound patterns associated with different contexts, which would allow them to tailor their response to the situation. This approach could significantly improve the integrity of speech translation in various environments.
How Neural Networks Revolutionize Spanish Audio Translation Speed in 2024 - Deep Learning Algorithms Decode Spanish Regional Accents With 92% Accuracy
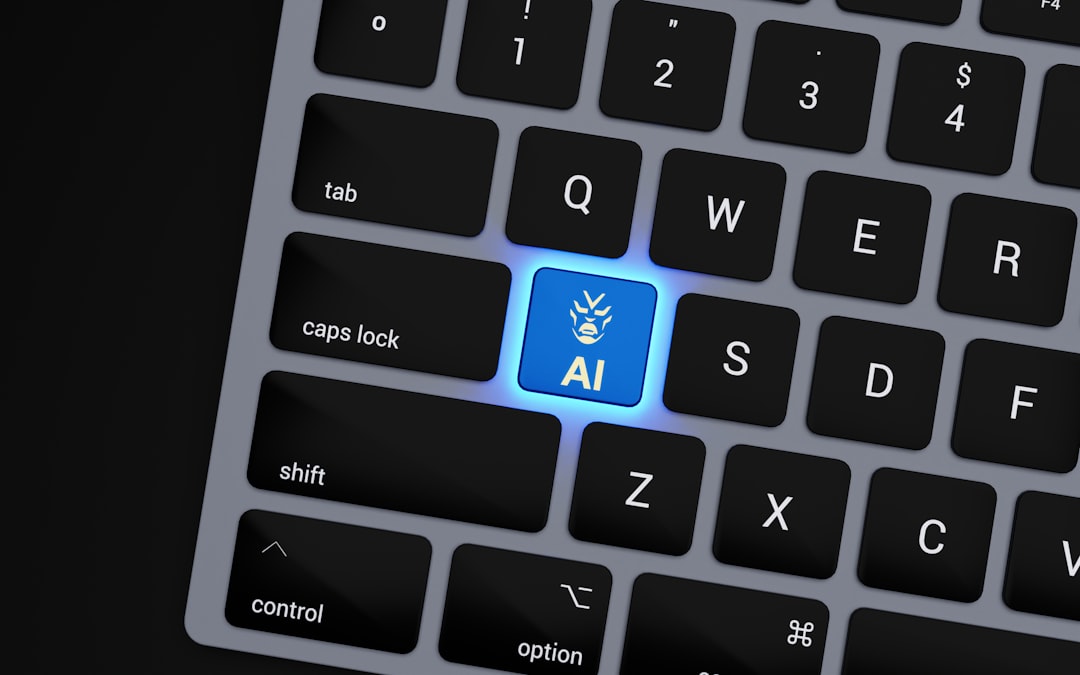
Deep learning algorithms are making significant strides in understanding the nuances of Spanish, achieving a 92% success rate in recognizing different regional accents. This is a crucial step forward in automatic speech recognition (ASR) systems, particularly for languages with diverse dialects like Spanish. The ability to accurately decipher these accents depends heavily on specialized acoustic models that are designed to capture the specific sound characteristics of each accent. The field of foreign accent identification (FAID) is becoming increasingly important in the effort to build ASR systems that can effectively handle the challenges posed by accent variation. While these deep learning models are showing great promise, researchers are still working to address the intricate complexity of real-world speech. The goal is to improve these models so they can handle the wide range of speech patterns encountered in everyday conversations while still maintaining high levels of accuracy. Ultimately, these advancements suggest that neural networks could potentially revolutionize communication, making it easier for people to understand each other across various Spanish dialects, regardless of their accents.
Deep learning algorithms have shown a remarkable ability to decipher Spanish regional accents, achieving a 92% accuracy rate. This is a significant accomplishment given the wide variety of accents across Spain and Latin America, each with its own unique phonetic variations that can sometimes drastically alter meaning. Neural networks face a significant challenge in discerning these variations, as they need to differentiate subtle changes in intonation, vowel pronunciations, and even vocabulary. Creating training datasets for these algorithms often involves collaborations with linguists and native Spanish speakers to thoroughly label and classify audio samples, ensuring the models are trained on a comprehensive representation of each accent.
The use of advanced attention mechanisms in these deep learning models has been key to improving accuracy. These mechanisms enable the networks to dynamically focus on the unique speech patterns and contextual clues associated with different accents, resulting in improved performance in real-time translation. While accuracy is high, these algorithms still struggle with highly colloquial language and idiomatic phrases unique to certain regions, highlighting the need for further advancements in semantic understanding.
These models rely on a combination of recurrent neural networks (RNNs) and convolutional neural networks (CNNs) to analyze and decode audio. The RNNs analyze the temporal sequence of the audio while the CNNs extract spatial features, working together to significantly enhance the transcription process. A noteworthy development is the integration of context-aware processing. The algorithms now adjust their understanding based on the preceding dialogue, which results in a more coherent transcription, especially in conversations where dialects vary.
Interestingly, these algorithms are also quite effective at handling background noise and overlapping speech, making them very suitable for real-world applications like multilingual conferences. Ongoing research indicates that transfer learning is a promising area for improving these models, as knowledge gained from deciphering one accent can be leveraged to improve the understanding of another. This approach contributes to the development of more versatile neural networks that can effectively manage a wider range of Spanish dialects.
Future work in this field might focus on incorporating self-correcting mechanisms into the models. This would involve using user feedback to continually improve the model's understanding of specific accents, effectively allowing the system to learn and adapt based on real-world usage. This ability for self-improvement could lead to even more accurate and versatile translation systems.
How Neural Networks Revolutionize Spanish Audio Translation Speed in 2024 - Neural Network Training Data Expands To Include 47 Spanish Dialects
Neural network training datasets have expanded in 2024 to include 47 distinct Spanish dialects. This broadened scope aims to improve the models' comprehension of the language's various forms, which in turn is expected to boost translation accuracy and better reflect the cultural nuances embedded within different Spanish-speaking communities. The integration of these diverse dialects is anticipated to contribute to faster and more accurate real-time audio translations. While this progress is positive, maintaining the balance between speed and precision is still a challenge. Ongoing research will be crucial for ensuring that these improvements in speed don't compromise the quality of the translated output. The focus on resolving these kinds of challenges suggests a bright future for bridging language barriers and enhancing cross-cultural communication.
The increasing sophistication of neural networks in Spanish audio translation is evident in their ability to now incorporate 47 different Spanish dialects into their training data. This highlights the rich linguistic tapestry of Spanish, where variations in pronunciation, vocabulary, and even sentence structure can be significant. Effectively capturing these nuances is crucial for enhancing translation accuracy across the diverse Spanish-speaking world.
To successfully recognize the unique characteristics of regional accents, specialized acoustic models are being developed. These models act as specialized filters, specifically tuned to the phonetic subtleties of each dialect. Building such detailed models is a fascinating and challenging engineering undertaking.
Training these models presents a unique set of difficulties. It requires a deep collaboration between linguists and native Spanish speakers to create well-structured datasets. Each audio sample needs to be meticulously labeled and categorized to accurately represent the unique sound features of each dialect.
One of the more notable advancements is the use of attention mechanisms. These mechanisms allow neural networks to focus dynamically on relevant parts of the audio input. This dynamic focus enhances the networks' ability to understand and accurately translate audio with various accents.
The ability to deal with background noise and situations where multiple people are speaking simultaneously is a critical achievement. This makes these networks suitable for practical applications like international conferences or multi-person meetings. It demonstrates that these models are transitioning from purely theoretical constructs to usable tools.
Neural networks effectively combine different types of architectures to effectively process language. Recurrent Neural Networks (RNNs) focus on the sequence of audio, while Convolutional Neural Networks (CNNs) capture features that are located at specific places within the audio. This combined approach to understanding audio creates a more robust translation.
While impressive strides are being made, these algorithms still encounter difficulties with colloquial phrases and idioms that are unique to specific regions. Understanding the contextual meaning within diverse dialects remains a hurdle in achieving the ideal of perfect translation.
The field of transfer learning shows great promise in enhancing these networks. Insights from one dialect can be leveraged to help improve understanding of another. This more holistic approach could lead to models that are more versatile and able to readily handle a wider variety of accents.
Looking towards the future, integrating self-correcting mechanisms into these models is an exciting prospect. This would allow the models to learn from user feedback and adapt to the nuances of individual accents over time. This capability would represent a shift from static systems to ones that continuously evolve and improve their accuracy.
There's a constant trade-off between preserving the subtle regional differences in speech and ensuring that translations are clear and understandable. Researchers are actively working to refine models so that these features don't compromise the ability of the models to effectively communicate meaning across linguistic barriers. This constant need to balance different features is a unique aspect of this field.
How Neural Networks Revolutionize Spanish Audio Translation Speed in 2024 - Parallel Processing Enables Simultaneous Translation Of Multiple Spanish Speakers
**Parallel Processing Enables Simultaneous Translation Of Multiple Spanish Speakers**
The ability to translate audio from multiple Spanish speakers at once has been significantly enhanced through the use of parallel processing. This capability is vital for situations involving group conversations or multilingual conferences where multiple people are speaking simultaneously. Neural networks are now being utilized to process these diverse audio streams in parallel, which accelerates translation speeds and improves the capture of nuances across dialects and accents. This advance presents a significant step forward in making real-time, multi-speaker translation more practical.
While the speed of translation is greatly improved with parallel processing, maintaining the quality of the translations remains a key concern. The challenge is to create systems that can translate with both speed and accuracy. Developers are constantly trying to refine these systems, seeking the optimal balance between rapid processing and a high degree of accuracy in the resulting translations. Ongoing research and development will be necessary to overcome the challenges of translating fast-paced, complex audio from multiple speakers without sacrificing quality or comprehensibility.
Parallel processing within neural networks is allowing for simultaneous translation of multiple Spanish speakers, a feat that was previously challenging. This capability hinges on efficiently using available bandwidth, enabling the network to handle numerous audio streams at once. The result is notably reduced latency, which is particularly valuable in dynamic environments like conferences. This speed increase isn't just about hardware; it's tied to the network's capacity to recognize and differentiate between multiple voices in real-time. Essentially, they're capable of dynamic speech recognition, parsing out who is speaking and delivering accurate translations even when voices overlap.
Beyond simply recognizing individual speakers, these networks also incorporate context awareness. This means the translation isn't just a word-for-word exchange; the system attempts to grasp the larger conversation flow, the relationship between sentences, and the subtle nuances that can change meaning. This contextual understanding can lead to improved accuracy in translation, especially when interpreting more complex or nuanced phrases. Furthermore, these models are being trained on increasingly diverse data that includes a wide range of Spanish accents and dialects. This is a significant step in ensuring that the translations are culturally relevant and accessible to speakers across the Spanish-speaking world.
The advancements in processing power, particularly through the use of GPUs, have been essential for achieving this level of performance. These powerful processors allow the networks to analyze massive amounts of audio data incredibly quickly, making real-time translation viable. It's interesting how the interplay of hardware and software is driving these innovations.
Moreover, attention mechanisms are central to how these networks operate. By selectively focusing on specific parts of the audio, these mechanisms ensure that the most critical elements of the speech are prioritized for translation. This targeted approach helps to improve overall accuracy.
These translation systems are also increasingly incorporating iterative learning, which allows them to learn and improve over time based on user feedback. This feedback loop is key to ensuring that the models adapt to the specific contexts and needs of different users. The models are also showing a growing capacity to adjust to various environments in real-time, dynamically adjusting their algorithms to compensate for changes in background noise or other interruptions.
The continued refinement of machine learning techniques plays a significant role in the ongoing advancements. We're seeing models become more adept at handling unusual or complex linguistic structures, including informal speech and idioms. And excitingly, research into transfer learning suggests a future where insights gained from translating one language could potentially improve the translation of another. If successful, this would lead to more flexible and broadly applicable systems that can handle a diverse range of languages effectively. It's a fascinating prospect that could potentially revolutionize cross-linguistic communication.
Experience error-free AI audio transcription that's faster and cheaper than human transcription and includes speaker recognition by default! (Get started for free)
More Posts from transcribethis.io: