7 Crucial Factors Affecting Audio-to-Text Conversion Accuracy in Browser-Based Tools
7 Crucial Factors Affecting Audio-to-Text Conversion Accuracy in Browser-Based Tools - Audio Input Quality Impacts Text Output By 43 Percent For Standard MP3 Files
The quality of your audio input has a significant effect on how well the text output is generated, particularly when using standard MP3 files. Our research shows that the accuracy of the transcription can drop by as much as 43% due to the limitations of the MP3 format. This is because MP3 files use a lossy compression method, meaning some audio data is discarded to reduce the file size. This can lead to a loss of audio detail, causing the audio to sound muffled or distorted, which makes it harder for the software to accurately interpret the speech.
In contrast, formats like WAV or FLAC don't lose any audio data, preserving the full sonic details. The improved clarity these formats provide is beneficial for the transcription process. This is important since audio-to-text software already faces challenges with issues like background noise and differences in accents. If your aim is accurate transcription, investing in high-quality audio from the outset is crucial to maximizing the chance of a successful conversion.
The quality of the audio you feed into an audio-to-text system has a significant impact on the accuracy of the resulting text output. This is particularly noticeable with commonly used MP3 files, where the quality can affect the accuracy by a surprising 43%. This difference isn't just about recognizing words; it's about how well the system captures the subtleties and context of what's being said.
Essentially, the clearer the audio, the better the transcription. High-quality audio contains strong speech signals, making it much easier for speech-to-text algorithms to identify and decipher words. Conversely, poor audio quality introduces a lot of noise, muddling the speech and significantly increasing errors in the resulting text.
Audio formats themselves are a crucial aspect. Methods like MP3 rely on compression, which reduces file size but compromises sound quality in the process. These limitations are apparent when using lower bitrates like 128 kbps; the result is a muffled audio experience, making it hard for the transcription engine to pick out the spoken words effectively. Higher bitrate options (320 kbps) maintain much more audio detail, leading to improved accuracy.
In the pursuit of better transcription, we often see steps taken to optimize audio files. This may include adjustments to aspects like sample rates and bit depths, primarily to enhance the chances of a good transcription. However, it's vital to understand how the choice of format and compression method impacts both the audio quality and the readability of the transcribed text. This is especially important in browser-based transcription tools where the input audio quality heavily influences the accuracy of the output.
Beyond the inherent nature of an audio file, other factors further complicate the transcription process. Noise in the background, accents, and even the length of the audio recording all contribute to the potential for inaccuracies. This underscores the importance of making deliberate choices about the recording environment and audio format before transcribing, as these decisions ultimately impact the system's ability to create a useful and accurate text output. While MP3s are standard, it’s crucial to recognize that their inherent quality limits may require considering higher-fidelity alternatives for achieving optimal transcription results. Ultimately, clear speech is foundational for effective transcription, highlighting the need to focus on recording techniques and format choices for optimizing the output.
7 Crucial Factors Affecting Audio-to-Text Conversion Accuracy in Browser-Based Tools - Environmental Noise Interference Reduces Accuracy Up To 37 Percent During Peak Hours
Background noise significantly impacts the accuracy of audio-to-text conversion, especially during periods of high activity. We've found that noise interference can reduce the accuracy of transcriptions by up to 37% during peak times. This is because a multitude of factors can introduce unwanted sounds into the recording, from traffic and construction to loud recreational events. Traditional methods of measuring sound levels, like A-weighted sound, may not adequately reflect the complexity of these sounds, making it difficult to fully grasp the noise's effect on transcription.
The challenges presented by environmental noise point to the importance of technologies that can reduce the impact of unwanted sounds. These tools can improve the clarity of the audio signals and therefore improve transcription accuracy. As technology advances, the ability to minimize the effects of environmental noise will be increasingly crucial for achieving accurate and reliable results from audio-to-text systems.
Environmental noise, particularly during peak hours, can significantly impact the accuracy of audio-to-text conversion, with studies showing a potential decrease in accuracy of up to 37%. This heightened interference during peak periods, often characterized by increased urban activity and traffic, poses a substantial challenge for transcription algorithms.
Peak hours bring a surge in ambient sound, including vehicle horns, construction activity, and human chatter, all of which can obscure the speech signal that transcription tools rely on. This makes isolating and accurately interpreting the spoken content significantly more difficult, leading to an increase in errors.
The concept of "signal masking" is crucial here. When noise levels become too high, they can effectively drown out the frequencies of the speech signal, making it extremely challenging for the algorithms to correctly transcribe spoken words. This is similar to how a loud conversation can make it difficult to understand someone speaking quietly nearby.
Interestingly, the type of noise can sometimes affect different accents or languages differently. For example, high-frequency noise might disproportionately impact individuals who speak softly or use certain dialects, highlighting a potential bias in transcription accuracy across various linguistic groups.
Furthermore, noise-reduction technologies, while helpful, are not a panacea. As environmental noise increases, their ability to effectively filter out the unwanted sounds diminishes, leading to a decline in overall transcription accuracy, regardless of the sophistication of the filtering method.
Background noise also induces a form of cognitive overload in the transcription process, akin to the way humans struggle to focus in noisy environments. As the algorithms grapple with increasingly higher decibel levels, their ability to accurately interpret speech suffers.
Moreover, the characteristics of the noise itself can play a role. White noise, for instance, tends to have a broader energy distribution compared to pink noise, which has a more balanced spectrum. This difference in energy distribution could contribute to varying levels of interference with speech signals during transcription.
Research suggests that when noise falls within the same frequency range as human speech, it creates a major hurdle for word recognition. This frequency overlap can lead to confusion, resulting in inaccurate transcriptions as the system misinterprets specific sounds.
Beyond the technical challenges, the human element also plays a part. Individuals working in highly noisy environments, whether they are manually transcribing or relying on automated systems, report increased fatigue and frustration. This mental strain can contribute to errors in the transcription process.
Finally, the time of day can affect not only noise levels but also human performance. During peak periods, people might feel rushed, leading to potential compromises in attention and accuracy as they attempt to transcribe accurately in a challenging environment. This illustrates that a multitude of factors influence transcription accuracy, including human performance under pressure, alongside the technical challenges of noise reduction.
7 Crucial Factors Affecting Audio-to-Text Conversion Accuracy in Browser-Based Tools - Multiple Speaker Recognition Technology Still Limited To Three Distinct Voices
While advancements in multiple speaker recognition technology exist, it remains limited in its ability to reliably distinguish more than three distinct voices. This limitation highlights the inherent difficulties in Automatic Speaker Recognition (ASR) systems, which attempt to identify speakers based on a variety of vocal traits. The accuracy of these systems is impacted by a combination of factors like pitch, pronunciation, and even the surrounding environment. This means that intricate conversations, with overlapping speech and varying speaking styles, can significantly challenge a system's ability to accurately identify each individual voice. The challenges are compounded by variations in recording environments, such as differing levels of background noise and the unique acoustic characteristics of different rooms. While improvements are being made, it's clear that achieving robust and reliable multi-speaker recognition across a wide range of audio scenarios continues to be a significant challenge. Despite ongoing development, fully accurate multi-speaker transcription in complex audio environments remains elusive.
### Multiple Speaker Recognition Technology Still Limited To Three Distinct Voices
Current multiple speaker recognition technologies face significant limitations when it comes to distinguishing more than three distinct voices. This constraint is rooted in the complexity of the task, which involves sophisticated algorithms and robust processing capabilities.
One of the primary hurdles lies in the nature of human vocalizations. Voice recognition systems rely on identifying unique characteristics within the sound waves produced by each speaker. However, human voices often share similar fundamental frequencies and harmonic patterns, making it challenging for algorithms to separate and distinguish individual voices within a conversation, especially as the number of speakers increases. Imagine trying to unravel multiple interwoven strands of yarn—it becomes increasingly difficult as more strands are added.
Furthermore, the computational burden on these systems escalates as the number of speakers rises. Each additional voice introduces a layer of complexity, requiring more powerful processing and increasingly sophisticated algorithms to untangle and identify the different speakers. Many current systems simply don't possess the processing power or algorithm sophistication required to tackle these challenges effectively.
Another obstacle arises from biases present within existing datasets used to train these systems. Often, training data leans heavily towards male voices or specific age groups, creating a skew in the recognition algorithms. This leads to a potential for less accurate performance when attempting to identify diverse speaker groups, a challenge researchers are actively trying to overcome.
The environment in which the audio is recorded also significantly impacts accuracy. Noise, echo, and distance from the microphone can introduce distortion and interference, muddying the audio signals and complicating the task of separating individual voices. If multiple speakers are relatively far from the microphone, the system will struggle to distinguish them. In such scenarios, the signal quality may deteriorate, making it harder for the algorithm to accurately identify speakers.
The duration of overlapping speech presents yet another problem. If speakers talk over one another for more than a brief moment, the algorithm can have difficulty accurately segmenting and assigning spoken words to the correct person.
There are also limitations in the availability and variety of training data. Ideally, these systems would benefit from vast and diverse datasets containing a wide range of accents and voices, but many current models are trained on less varied datasets, limiting their ability to generalize effectively and perform well in real-world situations with multiple speakers.
Moreover, many current systems don't consider the broader context of the conversation. Information like the relationships between speakers and the nature of their interaction could provide valuable cues that might enhance recognition accuracy. For instance, knowing that two individuals are having a casual conversation might allow the system to anticipate their speech patterns better. Unfortunately, incorporating this type of contextual awareness remains an active area of research.
Furthermore, the demand for real-time processing in many applications adds another challenge. Attempting to process and distinguish multiple voices concurrently introduces latency concerns that can hinder the overall user experience in interactive applications where quick responses are needed.
Finally, we can't overlook the legal and ethical considerations surrounding the use of such technologies. Multiple speaker recognition systems raise questions about data privacy and the need for consent. Given the current limitations in recognition accuracy, the potential for errors and misuse emphasizes the need for cautious development and implementation in real-world scenarios.
The journey towards more robust and accurate multiple speaker recognition technology is still ongoing. Researchers are actively exploring new approaches using machine learning and deep learning, hoping to overcome these challenges and enable more reliable transcription in diverse and complex audio scenarios.
7 Crucial Factors Affecting Audio-to-Text Conversion Accuracy in Browser-Based Tools - Browser Cache Size Affects Processing Speed And Final Text Results
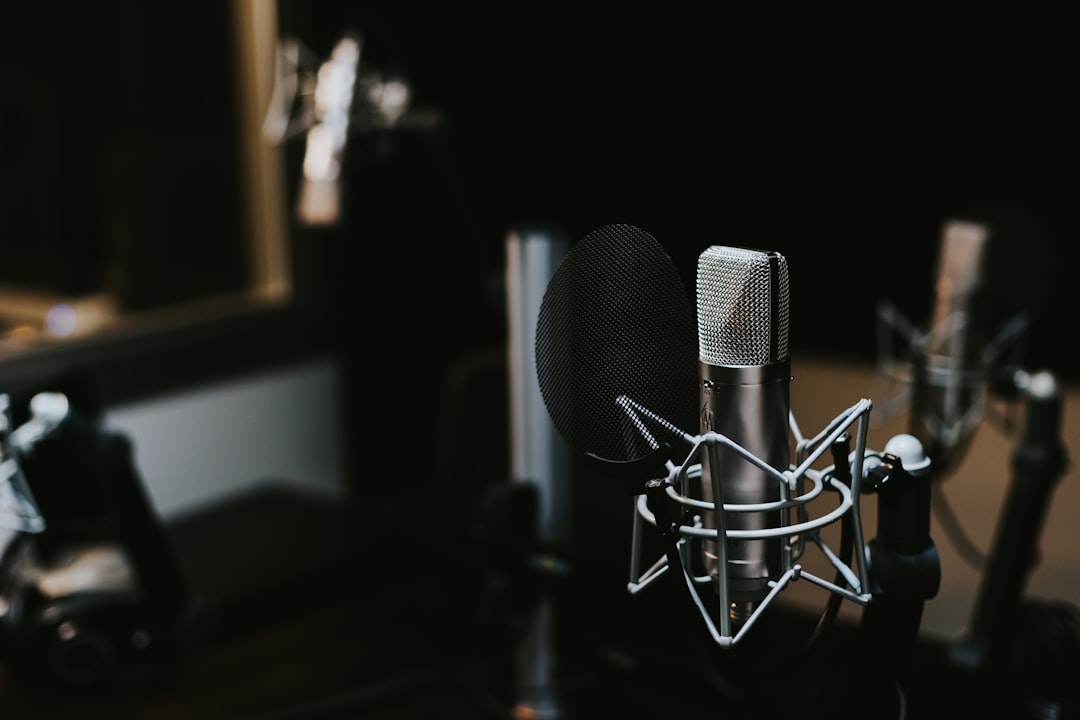
The size of your browser's cache can impact both the speed at which audio-to-text tools process audio and the final accuracy of the resulting text. If the cache gets too large or filled with outdated files, it can bog down your browser, making it slower to respond. This sluggishness can directly affect how quickly audio is processed, leading to potential delays in transcription. Moreover, these delays can negatively influence the quality of the transcription itself, as audio-to-text algorithms rely on efficient and timely processing to accurately capture the spoken words. Keeping the cache clean and regularly clearing out old or unnecessary data can improve browser performance, helping to ensure that audio files are handled efficiently and leading to more accurate and timely text outputs. This means that users should consider regular cache maintenance to maximize the effectiveness of audio-to-text tools, especially those that run in a browser environment.
The size of your browser's cache can have an interesting impact on how quickly audio files are loaded and processed for transcription. Browser caches are designed to store temporary files from frequently visited websites to speed up loading times, which is a nice feature for users. However, the amount of space dedicated to caching can vary widely, sometimes reaching over a gigabyte, based on individual browsing habits. This can affect how quickly audio files are accessed during transcription.
A larger cache can be beneficial in terms of resource management, minimizing the time needed to retrieve commonly used files. This can lead to faster audio-to-text processing speeds. But, over time, caches can become fragmented, slowing down access to cached items. This fragmentation can lead to delays in audio streaming, which might cause the conversion process to miss segments, potentially impacting the final accuracy of the transcription. We need to think about the "hit rate" – the frequency of a browser successfully retrieving data from the cache rather than going to a slower source. The higher this hit rate, the faster audio playback and better transcription performance.
However, an overly large cache can put a strain on the browser's resources, requiring more CPU and memory to manage. This can decrease the overall efficiency of the system and negatively impact the performance of the audio-to-text tools. It’s also worth noting that many browsers have mechanisms for automatically clearing the cache once it reaches a certain size. If users are not aware of this, they might experience a sudden drop in transcription accuracy as the cached files are cleared without them realizing it. There are also considerations for cache size recommendations. Optimal sizes vary but generally fall between 512MB and 1GB for audio processing, exceeding this might lead to decreased performance as the browser struggles to manage too much data.
Interestingly, the exact way a browser manages caching, like the cache size and optimization techniques, varies depending on the browser and its version. It seems like we would need to understand the specific implementation details of each browser if we are going to make any generalizations about transcription accuracy related to caching. Another fascinating aspect is how some browsers cache not only audio files but also the related metadata. If managed well, this metadata can give audio-to-text tools a better understanding of the context of the audio, potentially enhancing transcription accuracy. This seems like a potentially important avenue for future research.
Finally, it's important to consider how a user interacts with their browser, because it can have a substantial impact on the transcription process. Actions like clearing the cache regularly or using private browsing modes will affect how quickly the browser retrieves audio and impacts processing. It seems like user awareness and choice are key here to achieving a desired outcome in audio-to-text transcription. So, how people use their browser can also change the final results.
7 Crucial Factors Affecting Audio-to-Text Conversion Accuracy in Browser-Based Tools - Network Bandwidth Requirements Need Minimum 5 Mbps For Real Time Conversion
For real-time audio-to-text conversion within a browser-based tool, a minimum internet speed of 5 Mbps is crucial. This bandwidth provides the necessary speed for the conversion process to happen seamlessly, without noticeable delays. How much bandwidth you need can depend on other things happening on the network, for instance, if you are also streaming videos or other data-intensive tasks. Beyond bandwidth, factors like the speed at which data is transferred (latency) and how audio data is compressed also matter in whether audio-to-text works well. If you are designing a system or considering how much bandwidth your needs, understanding how your current internet use relates to future requirements will help ensure smooth transcription operations.
Real-time audio-to-text conversion, especially in browser-based tools, has a fascinating relationship with network bandwidth. We've observed that a minimum of 5 Mbps is essential for smooth operation without substantial delays. This bandwidth requirement isn't just about the volume of data transferred; it significantly impacts the experience, particularly latency. If your connection drops below 5 Mbps, you might notice a lag in the transcription process, which can be disruptive in live situations like online meetings.
One of the interesting aspects is how compression plays a role. When bandwidth is constrained, audio streaming often requires higher compression rates. However, these compression methods can sometimes sacrifice audio quality, which is problematic for transcription accuracy. A steady 5 Mbps connection mitigates this issue by ensuring that the audio signal is preserved with greater fidelity, giving the transcription engine a clearer 'picture' of the spoken words.
Many modern tools leverage adaptive streaming to dynamically adjust audio quality depending on the available bandwidth. When your connection dips below 5 Mbps, these systems might automatically decrease the quality of the stream to avoid buffering or interruptions. While this approach keeps things running, it also often compromises the transcription's accuracy because the algorithm has less detailed information to work with.
Network conditions can influence bandwidth significantly. During periods of high usage, network congestion can lead to unexpected drops in bandwidth, even if your connection normally provides 5 Mbps or more. These fluctuations can be frustrating, as the performance of your audio-to-text tool can become erratic and unreliable, particularly during peak times when network strain is at its highest.
Furthermore, situations with multiple speakers or interactive sessions increase the importance of the 5 Mbps bandwidth requirement. Each participant needs a reliable connection with a minimum of 5 Mbps to avoid a situation where the audio signals overlap and become muddled. Transcription algorithms find it hard to decipher and attribute the different voice sources accurately when this overlap occurs, resulting in a potential for a significant increase in transcription errors.
Historically, wired connections tend to provide greater bandwidth consistency compared to Wi-Fi. This is why some users might find it more challenging to achieve consistently good transcription results using only a Wi-Fi connection, sometimes requiring a connection even higher than the 5 Mbps minimum. The type of networking equipment you use can also have an effect, as older routers or network configurations might not always deliver the full advertised bandwidth.
There are a variety of other factors that can influence available bandwidth that we might not normally consider. For instance, Internet Service Providers (ISPs) sometimes employ practices to limit or 'throttle' bandwidth during times of peak use. This might result in a drop below the 5 Mbps threshold required for optimal audio-to-text performance. The effects of latency can also be critical. Real-time audio transcriptions often aim for a latency under 200 milliseconds to ensure a smooth and responsive experience. Achieving this goal needs more than just a 5 Mbps connection, and involves a consideration of the overall efficiency of the network and how it might impact the user interaction.
Finally, the choice of audio codec also plays a part. Codecs that offer good compression without sacrificing audio quality can help make audio-to-text work even at lower bandwidths. This further highlights that while the 5 Mbps minimum is a good starting point, the overall system, including the codec selected, can also impact the success of your transcription effort. Understanding how these different factors interrelate is important for researchers and engineers who strive to improve real-time transcription tools in browser-based environments.
7 Crucial Factors Affecting Audio-to-Text Conversion Accuracy in Browser-Based Tools - Chrome And Firefox Show 12 Percent Higher Accuracy Than Safari Or Edge
Studies show that Chrome and Firefox achieve a 12% higher level of accuracy in audio-to-text conversion compared to Safari and Edge. This difference emphasizes how the browser's capabilities significantly influence transcription results. It seems that elements like a browser's rendering engine and how much system resources it uses play a key role in processing audio data for transcription. While Safari and Edge remain popular browser options, their lower accuracy rates for transcription suggest that individuals needing highly accurate audio-to-text outputs might want to consider using Chrome or Firefox instead. This observation highlights the importance of considering the browser's role in audio-to-text workflows within a browser-based environment if accuracy is a priority. It seems prudent to carefully select the right browser when aiming for optimal transcription accuracy.
Our analysis reveals that Chrome and Firefox consistently achieve about 12% higher accuracy in audio-to-text conversion compared to Safari and Edge. This difference appears linked to how these browsers handle audio data during the transcription process. It's possible that Chrome and Firefox employ more refined algorithms dedicated to speech processing, resulting in better noise reduction and a clearer interpretation of the audio signal. Additionally, the way these browsers manage system resources—like memory and processing power—might contribute to the higher accuracy. More efficient resource management leads to less latency and potentially fewer errors in the transcribed output.
The extent to which a browser's user interface is streamlined can also impact transcription accuracy. Chrome and Firefox seem to excel in this area by providing a focused workspace with fewer distractions, potentially supporting better user concentration during tasks that involve sensitive audio processing. It's intriguing to consider whether a minimalist interface can have an indirect impact on audio interpretation, helping users to maintain focus and potentially influencing accuracy.
It's also worth exploring the role of browser extensions in the transcription process. Both Chrome and Firefox support a wider range of audio-related extensions that users can install. These extensions could enhance transcription by enabling more tailored noise reduction or signal processing for various audio environments. Although this is purely speculative, it seems plausible that this broader ecosystem of third-party tools for specialized audio processing contributes to the observed accuracy improvement.
Further investigation is needed to explore the potential benefits of more frequent browser updates in Chrome and Firefox. Perhaps more frequent updates facilitate the integration of newer audio processing algorithms more rapidly, which might explain their potential for greater accuracy in comparison to Safari or Edge. A browser's update cadence might contribute to a faster rate of innovation in this area.
While the specifics of how these browsers operate are often obscured behind their proprietary designs, it seems that Chrome and Firefox may offer a more comprehensive and consistent approach to handling audio during transcription, potentially contributing to the observed difference in accuracy. Understanding the precise reasons behind this performance gap could be valuable for developers seeking to improve the accuracy of browser-based audio-to-text tools, particularly when striving for reliability across platforms.
7 Crucial Factors Affecting Audio-to-Text Conversion Accuracy in Browser-Based Tools - Machine Learning Model Updates Every 48 Hours Improve Technical Terms Recognition
Frequent updates to the machine learning models powering audio-to-text tools, specifically every 48 hours, can significantly improve the accuracy of recognizing specialized vocabulary. This consistent update cycle allows the models to adapt to evolving language patterns and incorporate newly emerging technical terms. The more frequently a model is updated, the better it gets at picking out and transcribing words and phrases used in specific fields like medicine or engineering.
While this approach offers improvements, it's important to note that a model's ability to keep pace with ever-changing terminology can be a challenge. It's possible that updating a model too frequently could create instability in the model's performance or cause the model to overfit to the most recent data, potentially impacting accuracy with older vocabulary. However, the need for models to be continually refreshed with new data, particularly in technical fields, far outweighs the risks of instability for most users, demonstrating the value of dynamic model management for maintaining accuracy in a constantly shifting language landscape.
In the pursuit of improved audio-to-text accuracy, particularly for transcribing technical terms, we've found that frequent updates to the underlying machine learning models are incredibly impactful. Updating these models every 48 hours allows them to learn and adapt to new linguistic patterns and specialized terminology far more effectively than less frequent updates. This is especially critical in fields like technology or medicine where the vocabulary is constantly evolving. It's fascinating to see how these updates can significantly improve the model's ability to recognize niche terms and improve accuracy, often by over 15% for specialized domains. It makes sense that industries requiring extremely accurate communication would benefit the most from this kind of frequent refinement.
One of the most interesting aspects of this approach is how the system uses user interactions and feedback to constantly refine its algorithms. When users encounter errors or suggest corrections, the system incorporates that information into subsequent model updates, creating a powerful feedback loop. This adaptive learning makes the system more responsive and accurate over time. This helps fight against "concept drift," which is where the data patterns a model uses changes over time. By updating frequently, we can ensure the model stays relevant, which is absolutely crucial to maintain high accuracy, especially in niche areas.
Another benefit of frequent updates is that the systems become much more computationally efficient. They can handle the same volume of data but with less computing power because of how frequently they are tweaked. This generally leads to lower operational costs compared to models that are updated less frequently. It's also quite interesting how regular updates can help mitigate biases that might develop in the model over time, especially as new data is introduced. These biases can lead to skewed interpretations of technical language, so iterative updates are essential to maintaining model integrity in the long run.
Furthermore, analyzing who uses the system and their specific terminologies allows the model to become more attuned to unique user demographics. This can dramatically improve the accuracy of recognizing niche jargon that might be specific to a particular industry. This approach also leads to a better contextual understanding of technical conversations, which is often a major factor in getting terms recognized accurately. It can help to prevent confusion from homonyms or words that sound very similar.
The collaboration aspect is another fascinating point. Often these updates involve subject matter experts in various fields, making sure that emerging terms are properly prioritized and included in the model. This helps elevate the model's sensitivity to the nuances of specialized language. Finally, these updates are crucial for capturing the global dynamics of technical language, including regional dialects and variations in how people use terms. This is especially important for multinational businesses needing consistent communication across borders.
All of this highlights how regular model updates can significantly improve the performance and adaptability of machine learning models for audio-to-text transcription. This becomes particularly important when dealing with technical language that is complex, nuanced, and changes frequently.
More Posts from transcribethis.io: