Google DeepMind's Pivot From Research Lab to AI Product Powerhouse
Google DeepMind's Pivot From Research Lab to AI Product Powerhouse - Google's strategic shift from AI research to product development
Google's approach to artificial intelligence has taken a decisive turn, moving away from its historical emphasis on basic research and embracing a more product-focused strategy. This shift involves the integration of Google Brain and DeepMind, two of the company's leading AI research entities, into a unified force dedicated to developing commercially successful AI applications. The transfer of the team behind Gemini, Google's AI assistant, to DeepMind signifies a centralizing of product development. The objective, spearheaded by DeepMind's CEO Demis Hassabis, is to fortify Google's position in the competitive AI landscape, especially considering the increasing pressure from competitors like OpenAI and Microsoft. This new direction, however, raises questions about the future role of DeepMind in fundamental AI research, a domain where it has historically been a prominent player. Will this pivot lessen its contributions to the broader field of artificial intelligence, or can it maintain its research focus alongside its newfound commercial drive? The coming years will undoubtedly reveal the true impact of this strategic realignment on both Google's AI ambitions and the future of the field itself.
Google's strategic redirection from primarily focusing on fundamental AI research to a product-centric approach signifies a shift towards making AI accessible and useful to a wider audience. This change is evident in the increasing integration of AI into Google's core services, aiming to improve existing experiences with search, recommendations, and other features. This involves a closer collaboration between research and product teams, an interesting development considering the historical separation between these functions.
However, the accelerated path to integrating AI into products presents new challenges, especially concerning the ethical implications and potential misuse of these powerful technologies. It forces Google to navigate the urgent need to deliver innovative products alongside the responsibility of developing AI in a safe and equitable manner. Interestingly, this pursuit of practical applications has led to a heightened focus on user-centric design, with AI increasingly tailored to individual needs and preferences.
The company is embracing a more holistic approach, fostering interdisciplinary teams encompassing expertise from various fields like psychology and user experience design. This broadened perspective is encouraging in the context of responsible AI development. Another noteworthy aspect of this transition is the shorter development cycle for AI innovations. What once took years to mature into a product now finds its way to users in significantly less time.
Yet, this prioritization of product delivery raises concerns about sustaining a strong culture of foundational research and open exploration. Metrics related to user engagement and product success are now guiding decision-making, in contrast to the traditional emphasis on technical breakthroughs. This shift isn't unique to Google, reflecting a broader industry trend as technology firms are increasingly driven by the commercial potential of AI. This creates a competitive landscape where rapid application-driven advancements become crucial for market leadership. It will be intriguing to see how this new era of AI commercialization influences the development of the field, especially in areas previously dominated by fundamental research.
Google DeepMind's Pivot From Research Lab to AI Product Powerhouse - Competitive pressures drive DeepMind's new focus on commercial applications
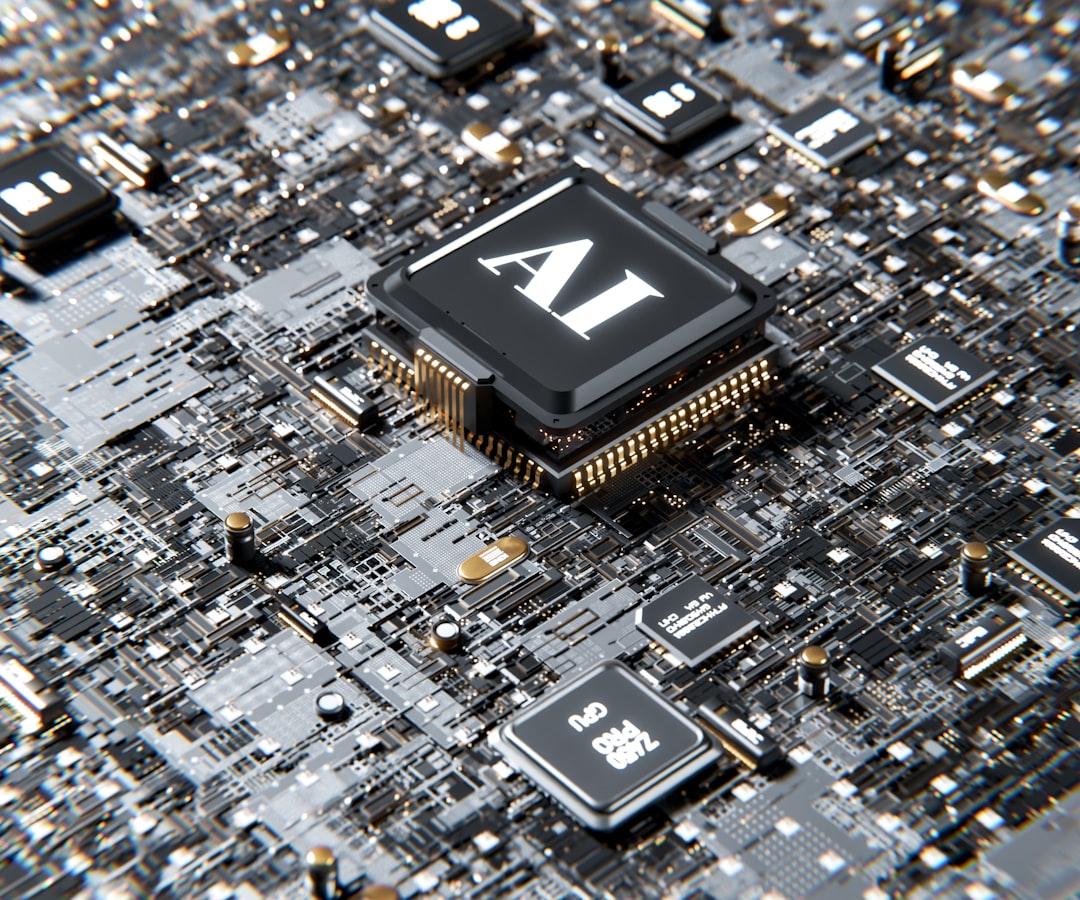
The intensifying competition within the AI field is driving a notable shift in DeepMind's priorities. Historically known for its focus on basic AI research, DeepMind is increasingly turning its attention towards creating commercially successful AI products. This change, partly driven by the need to keep pace with competitors like OpenAI and Microsoft, involves the merger of DeepMind with Google Brain, a move aiming to streamline the development of commercially viable AI applications. The urgency to deliver products quickly, fueled by market demands and investor expectations, could potentially impact DeepMind's longstanding dedication to fundamental AI research. There's a growing concern that this transition could shift the balance away from the exploration of fundamental AI concepts, potentially hindering DeepMind's capacity to contribute meaningfully to the broader field. The coming years will likely reveal how this emphasis on commercialization impacts DeepMind's overall contributions to the field of artificial intelligence. It is a critical moment that could alter DeepMind's long-held role within the wider AI landscape.
The competitive landscape of AI has undeniably influenced DeepMind's shift in focus, moving from its foundational research roots towards a more commercially driven approach. This change, driven partly by the need to keep pace with competitors like OpenAI, especially after the success of products like ChatGPT, has resulted in a consolidation of research divisions within Google, merging DeepMind and Google Brain. This merger, while potentially streamlining the development of practical AI solutions for Google's product ecosystem, could also impact DeepMind's ability to continue its trailblazing research efforts.
Historically, DeepMind's research has been the driving force behind groundbreaking advancements, such as AlphaFold's impact on protein folding, a field with immense potential for drug discovery. The pressure to deliver commercially viable AI products quickly may divert resources away from such frontier explorations. Furthermore, the integration of Google Brain into DeepMind, while intended to enhance AI product development, raises questions about how the strengths of each team will be integrated. This concern stems from the potential for unique research approaches to be overlooked in the rush to deliver commercially successful products.
DeepMind's history is characterized by long-term research, epitomized by its reinforcement learning projects in games like Dota 2 and Go. These endeavors were driven by a more deliberate approach to achieving breakthroughs, a philosophy that might be sidelined by the need for quicker market adaptation. This pressure for speed may lead to a reduction in the rigorous research process and the careful development of robust AI models.
Furthermore, this pivot to a product-focused approach necessitates relying on large-scale user data for model training and development. While this accelerates the refinement of user experiences, it also introduces risks associated with data privacy and ethical data usage. Balancing the benefits of personalized experiences with data privacy concerns is a constant challenge that Google DeepMind will now have to face.
The push towards user-centric design has encouraged interdisciplinary collaborations, combining insights from fields like cognitive science with engineering. While this broadening of perspectives has potential benefits, it could potentially dilute the technical rigor that is fundamental to DeepMind's research identity. It will be interesting to see how they manage this delicate balance in the coming years.
Finally, the accelerated product development cycle championed by DeepMind runs the risk of overshadowing fundamental research. The rapid development of prototypes could eclipse more theoretical explorations that have historically given rise to innovative advancements. Balancing market demands with its foundational research mission is a significant challenge, potentially impacting DeepMind's reputation as a leader in fundamental AI research.
It remains to be seen whether DeepMind can maintain its position at the forefront of AI research while simultaneously fulfilling its new commercial obligations. The broader tech industry's shift towards prioritizing AI's commercial potential over fundamental advancements is a trend that warrants monitoring. It is crucial to assess how this focus on profitability affects the long-term health of the field and the quality of innovation produced. DeepMind's future will undoubtedly be shaped by its ability to navigate this complex terrain and ensure that its research legacy remains intact while it adapts to its evolving commercial responsibilities.
Google DeepMind's Pivot From Research Lab to AI Product Powerhouse - Consolidation of AI divisions under DeepMind banner in May 2024
In May 2024, Google restructured its AI operations by bringing various divisions under the DeepMind umbrella. This consolidation aimed to improve Google's ability to create and release AI products. The merger included teams from Google Research, encompassing model development and responsible AI efforts, all merged into the new Google DeepMind entity. This strategic decision coincided with an escalating competition within the AI space, particularly from companies like OpenAI and Anthropic, and reflected Google's goal of bringing its research into more tangible, commercial AI products. While the integration promises to streamline AI development and delivery, there are worries that DeepMind's historically strong emphasis on foundational AI research might be lessened in the push for fast product releases. This organizational shift is a key moment for Google DeepMind, challenging it to remain at the forefront of innovation while balancing its goals with the demands of a competitive AI market.
In May 2024, Google announced a significant restructuring by bringing all of its AI divisions under the DeepMind banner. This move signaled a notable shift in strategy, aiming to streamline efforts and enhance the development of AI products. The reorganization involved merging teams from Google Research and DeepMind, including units focusing on AI model development and responsible AI, into a unified entity called Google DeepMind. This merger aimed to create more cohesive teams and accelerate the delivery of AI products.
This decision is clearly part of a larger plan to solidify Google's position in the increasingly competitive AI landscape, particularly in the face of challenges from companies like OpenAI and Anthropic. The Gemini team, Google's AI model initiative, was incorporated into DeepMind, furthering the focus on developing advanced AI capabilities for use in products. Simultaneously, including responsible AI teams under DeepMind was meant to better align the development of AI models with safety and ethical considerations. The primary goal of this change was to shorten the time it takes for AI innovations to reach users.
The consolidation was intended to foster more effective communication and collaboration between the groups involved in AI research and those working on implementing these ideas in actual Google products. Google's CEO, Sundar Pichai, communicated the strategic shift to employees, emphasizing that the goal was to leverage AI advancements to help Google meet its business objectives more quickly. As a result of the reorganization, DeepMind is now seen as much more than just a research lab—it is envisioned as a key force in creating and deploying Google's AI products. This reorganization, in essence, underscores Google's determination to enhance its AI capabilities by combining the strengths of its various research teams to deliver tangible AI applications.
Google DeepMind's Pivot From Research Lab to AI Product Powerhouse - Hassabis and Raghavan spearhead DeepMind's transformation
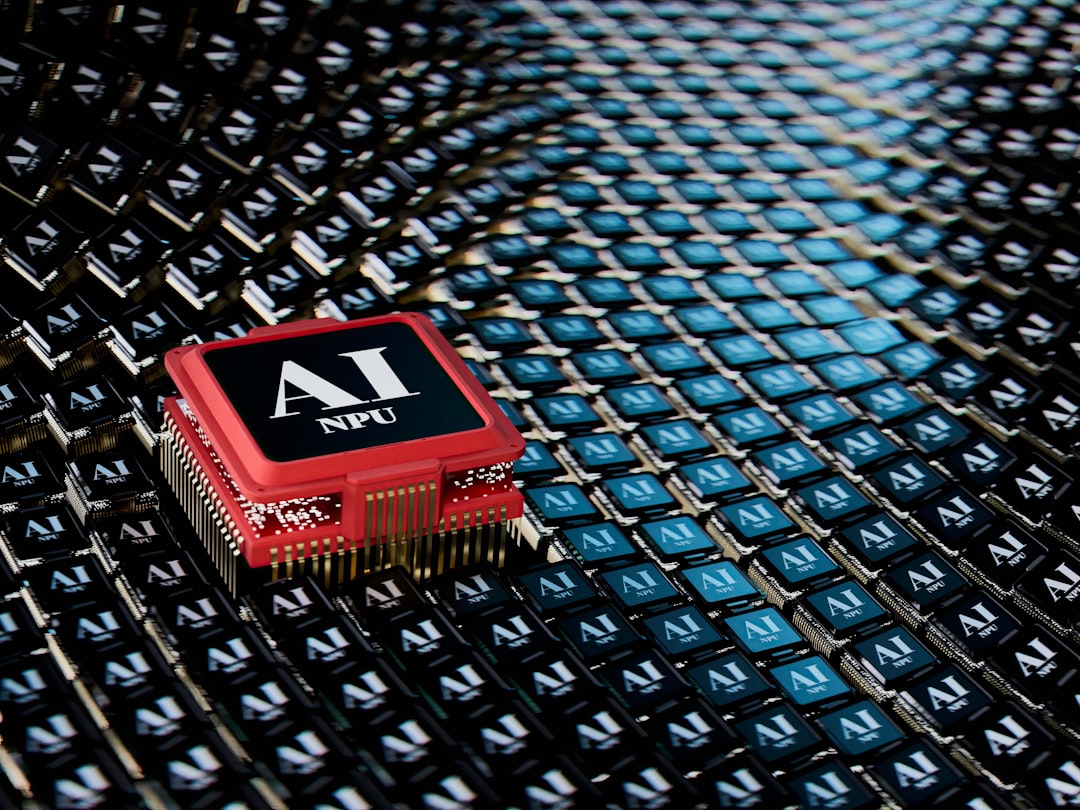
Demis Hassabis and Prabhakar Raghavan are leading DeepMind's transition from a research-focused entity to a powerhouse for AI product development. This transformation involves merging DeepMind with Google Brain, signifying a strategic shift towards developing commercially viable AI solutions. The goal is to accelerate the delivery of AI products, which is partly driven by the growing competition in the AI space. However, this shift raises concerns that DeepMind's longstanding commitment to fundamental AI research might be sidelined. Hassabis's role becomes pivotal in navigating this dual focus, ensuring DeepMind continues to make significant contributions both to the creation of practical AI products and the expansion of core AI knowledge. It remains to be seen whether the company can seamlessly manage these potentially conflicting aims and maintain its position as a leader in both commercial and theoretical AI advancement.
Demis Hassabis, DeepMind's co-founder, has been at the helm since 2010, guiding the company through a significant shift. From its origins as a research-centric organization, it's now transitioning into an AI product powerhouse within the Google ecosystem. His background in both cognitive science and AI makes him well-suited to navigate this transformation.
Hassabis and Manohar Raghavan are leading DeepMind's efforts to leverage their expertise in deep learning models into practical applications that can enhance user experiences across Google's product line. This signifies a departure from their initial focus on theoretical AI explorations.
The 2024 integration of Google Brain into DeepMind mirrors a broader trend in the tech sector. Companies are increasingly consolidating research and product teams to expedite AI development and get solutions to market faster. While this could streamline the innovation process, it's a move that could impact the broader AI landscape.
AlphaFold, one of DeepMind's most remarkable achievements, illustrated the power of applying deep learning in the life sciences, resolving the protein folding problem that had stumped scientists for years. This success underlines the impressive research foundation DeepMind now aims to balance with its product-oriented ambitions.
The corporate restructuring is a direct response to the accelerating pace of AI development, largely fueled by competitors like OpenAI and Microsoft who have successfully commercialized their own models. This emphasis on speed raises questions about DeepMind's ability to maintain its dedication to fundamental AI research as it faces increased pressure to deliver products quickly.
Shortening the development cycle is a core aim of the new Google DeepMind, but this potentially challenges the rigorous approach necessary for groundbreaking discoveries. Historically, truly innovative AI models have stemmed from extensive research periods; a faster pace could inadvertently limit these theoretical advances.
By consolidating various teams, DeepMind is attempting to forge a stronger link between AI research and practical application, ensuring responsible AI practices are built into their product development. This dual focus is essential in a time when public concerns about AI's ethical implications are growing.
Hassabis and Raghavan envision fostering collaborations that extend beyond computer science, incorporating disciplines like behavioral science and cognitive psychology to shape AI products with users in mind. This multidisciplinary approach could increase the products' relevance but also carries the risk of diluting specialized technical knowledge.
DeepMind's pivot towards commercialization also means that the metrics for success are changing. Instead of purely scientific accomplishments, the focus now includes user engagement and product performance. While this shift might lead to immediate gains, it potentially jeopardizes the long-term impact and research legacy that DeepMind has built over the years.
As part of this change, DeepMind has strategically prioritized AI applications, resulting in the creation of innovative products like the Gemini AI assistant, meant to significantly improve Google's services. This move reflects a calculated decision to combine advanced AI capabilities with user-friendly interfaces in an effort to secure Google's competitive position.
Google DeepMind's Pivot From Research Lab to AI Product Powerhouse - AlphaFold success sets stage for DeepMind's product-oriented future
DeepMind's AlphaFold project, particularly the recent release of AlphaFold 3, represents a pivotal moment in its journey from a primarily research-driven lab to an organization focused on creating impactful AI products. The remarkable ability of AlphaFold 3 to accurately predict the structures of a wider variety of molecules, including protein-DNA and protein-RNA interactions, demonstrates its maturing potential beyond the purely scientific. This advance significantly strengthens its value for scientific endeavors in areas like drug development and molecular biology. This showcases DeepMind's broader strategy: to leverage its groundbreaking research into practical applications. Yet, there's a tension inherent in this transition. The push towards rapidly delivering AI-powered solutions might come at the cost of the deep exploration and fundamental research that historically fueled DeepMind's achievements. Maintaining a balance between creating commercially viable tools and continuing to pursue ambitious, curiosity-driven research will be a crucial challenge as DeepMind moves forward.
AlphaFold's journey has been remarkable, moving from a research curiosity to a tool capable of predicting protein structures with impressive accuracy. John Jumper, at Google DeepMind, highlights the impressive evolution of the models, with AlphaFold 3 handling an even broader range of molecular inputs than its predecessors. The original AlphaFold, launched in 2020, was a game-changer, enabling swift and accurate predictions of protein structures, a capability that has fundamentally shifted how researchers study protein functions and interactions.
This accuracy extends to the majority of molecules cataloged within the Protein Data Bank (PDB), often achieving atomic-level precision. This level of detail helps us better understand a wider array of biomolecules, including ligands, which play critical roles in cellular processes. Google DeepMind's 2022 decision to release AlphaFold predictions for nearly all known proteins was a major step towards democratizing access to this powerful technology. The launch of the AlphaFold Server, a freely accessible tool, empowers researchers to generate molecular complex predictions based on their own sequence inputs.
Interestingly, AlphaFold 3 stands out as the most accurate tool globally for modeling protein interactions with other cellular molecules, with a dedicated focus on research applications. It's fascinating that its abilities extend beyond proteins, now accurately predicting structures involving DNA and RNA as well, suggesting broader applicability within biological modeling. This evolution points to a strategic shift for Google DeepMind, moving away from a purely research-driven lab and toward a more product-oriented focus to leverage AI for scientific progress.
This focus on widespread adoption through freely available tools like the AlphaFold Server promotes broad scientific exploration. The improvements seen in AlphaFold are a testament to AI's potential to drive scientific discovery, particularly within the field of biology. It accelerates the pace of research, potentially leading to faster breakthroughs in a field that has historically relied on painstaking experimental techniques. However, it remains to be seen how this push for widespread use impacts the broader research landscape, particularly regarding access and ethical considerations. While the promise of AI-driven progress is huge, it’s important to acknowledge the responsibility that comes with such capabilities and ensure that they are developed and applied ethically and equitably.
More Posts from transcribethis.io: