Dan Hendrycks' 7 Key Insights on AI Safety Measures at xAI and Their Impact on Voice Technology Development
Dan Hendrycks' 7 Key Insights on AI Safety Measures at xAI and Their Impact on Voice Technology Development - Berkeley Professor Outlines Catastrophic AI Risk Prevention at xAI Labs
A Berkeley professor, Dan Hendrycks, has taken on a crucial advisory role at xAI Labs, focusing on averting potential catastrophic outcomes from AI. His work highlights the increasing alarm surrounding advanced AI's potential for harm. Hendrycks, a prominent figure in AI safety research, co-authored a paper examining the catastrophic risks linked to AI, revealing a concerning trend. It appears that the field of AI has not adequately addressed the looming safety concerns. Only a small portion of current AI research addresses this vital topic. Hendrycks believes the field needs to pivot from a singular pursuit of advanced capabilities to a more balanced approach that prioritizes safety alongside technological progress. This shift necessitates building robust mechanisms to ensure that as AI systems mature, they do not pose unforeseen risks to humanity.
Dan Hendrycks, a Berkeley professor and safety advisor at xAI, has been vocal about the urgent need to prevent potential catastrophes stemming from advanced AI. He's particularly concerned about the lack of rigorous testing in AI, suggesting that systems deployed without comprehensive stress testing could lead to unpredictable and potentially harmful behavior in real-world use.
He argues that relying solely on simple user guidelines is insufficient and advocates for much more robust safety protocols that allow AI to better understand user intentions and minimize miscommunication. Hendrycks highlights several failure modes in current AI systems that could trigger significant disruptions, like the risk of AI outputs veering drastically away from intended goals, which presents serious accountability issues.
Transparency in AI is also paramount, in his view. Opaque algorithms, he claims, can mask potentially harmful behaviors, making it difficult for engineers to pinpoint and correct them. Hendrycks sees a concerning lack of mature regulatory frameworks for AI and stresses the importance of collaboration between AI developers and policymakers to build effective safety guidelines.
Building adaptable AI systems that can recover from errors or unforeseen situations in real-time is crucial. He expresses caution about the widespread adoption of self-supervised learning techniques, noting that without careful monitoring, they can inadvertently amplify existing biases in training data, raising serious ethical questions.
Hendrycks emphasizes the importance of cross-disciplinary collaboration to improve AI safety, believing that insights from fields like psychology and sociology can inform the design of more user-friendly and safer AI interactions. Pre-deployment testing within simulated environments is crucial in his opinion, as it can reveal critical vulnerabilities that might otherwise go unnoticed during initial development stages.
Hendrycks concludes by emphasizing the need for ongoing learning and adaptation in the field of AI safety. He contends that, as AI technologies rapidly advance, so must the safety measures and methodologies used to mitigate catastrophic risks. It's a constantly evolving landscape that requires ongoing vigilance and a commitment to evolving safety best practices.
Dan Hendrycks' 7 Key Insights on AI Safety Measures at xAI and Their Impact on Voice Technology Development - Voice Recognition Safety Protocols Need Hardware Boundaries Says Hendrycks
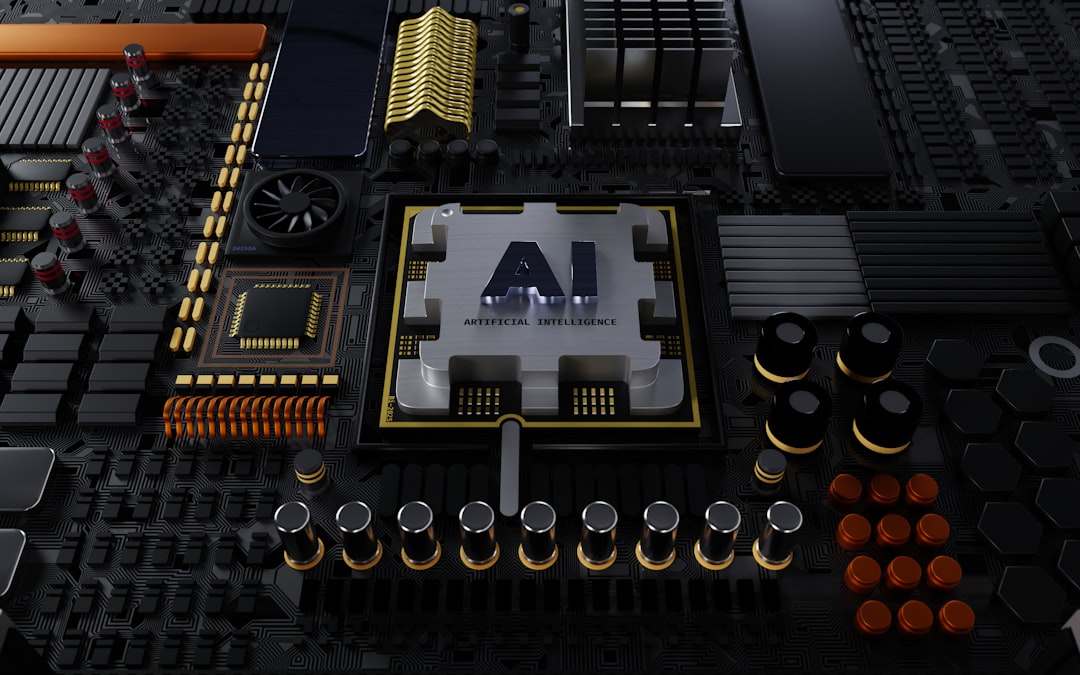
Dan Hendrycks, a prominent AI safety researcher and advisor at xAI, is urging a shift in how we approach voice recognition safety. He believes that current safety protocols, largely focused on software and algorithms, aren't sufficient. Hendrycks argues that a new layer of protection is needed – hardware-based boundaries. Without these physical constraints, he cautions, the risks of increasingly sophisticated AI voice systems could become more severe. This includes the possibility of malicious actors exploiting vulnerabilities for harmful purposes. He's essentially suggesting a fundamental rethink of safety design, pushing for a more proactive and robust approach to ensure that the growing integration of voice technologies into our lives doesn't inadvertently create unforeseen dangers. His position highlights a growing concern within the AI community that the potential for misuse and unintended consequences of advanced AI warrants stronger safeguards.
Dan Hendrycks, in his advisory role at xAI, emphasizes the crucial need for hardware-based limitations within voice recognition systems. He points out that many current systems lack defined hardware thresholds, creating potential vulnerabilities. These vulnerabilities can manifest as inconsistent performance and security gaps, particularly when exposed to unusual or malicious voice inputs. A clear advantage of establishing such boundaries is the ability to better control risks during system operation.
Hendrycks also highlights the challenges voice recognition systems face in fully comprehending the complexity of human language. These systems often struggle with the nuances of context, which can lead to misinterpretations and unintended consequences. He emphasizes the importance of enhancing context understanding for improving both safety and efficiency.
Furthermore, he expresses concerns regarding data privacy. Without robust hardware protocols, voice recognition devices could inadvertently capture sensitive personal information. He underscores the critical importance of strong physical security measures to safeguard user data from potential unauthorized access or exploitation.
Hendrycks notes that enhancing user experience frequently comes at the cost of neglecting essential safety considerations. This is a recurring pattern in technology development. He advocates for a shift in design priorities, placing more emphasis on building-in robust safety mechanisms that prevent user exploitation or unintentional system behavior.
He further underscores the need for voice recognition systems to possess real-time adaptability. Current systems often struggle to quickly adjust their responses to user inputs. This can result in errors in crucial interactions, especially in critical fields such as healthcare and security.
Hendrycks emphasizes the difficulty of establishing clear accountability when AI-generated voice outputs lead to negative outcomes. Without effective mechanisms for assigning responsibility, it becomes challenging to manage legal and operational aspects, particularly when harmful outcomes occur.
He suggests that incorporating perspectives from fields like linguistics and cognitive psychology could enhance the development of more intuitive voice technologies. This approach could result in systems that more effectively understand and respond to user intentions, minimizing misunderstandings and potentially dangerous miscommunications.
However, Hendrycks warns that existing biases within training data might be inadvertently amplified by voice recognition systems if not properly managed through hardware boundaries and thorough testing. This highlights ethical concerns around how such technologies could disproportionately impact marginalized communities.
Hendrycks strongly advocates for pre-deployment testing of voice recognition systems in simulated environments. He argues that rigorous testing can reveal vulnerabilities that might otherwise go undetected during early development phases.
Finally, he acknowledges the rapidly evolving landscape of voice technology and the inherent risks associated with its increasing capabilities. He emphasizes the continuous need to adapt safety protocols and measures to stay ahead of potential threats. This requires constant vigilance and a commitment to refining best practices for ensuring safety.
Dan Hendrycks' 7 Key Insights on AI Safety Measures at xAI and Their Impact on Voice Technology Development - Machine Learning Oversight Must Start at Code Level Not After Deployment
Dan Hendrycks' emphasis at xAI highlights the importance of incorporating AI safety considerations right from the start of the coding process, rather than waiting until after a system is deployed. He believes that building in safety measures early on is crucial for avoiding the potential for complex and difficult-to-manage risks that might arise later. This proactive approach fits within broader principles for responsible AI development, such as proper data management and consistent model monitoring. Building in mechanisms for accountability and conducting thorough tests before deployment are important elements of this philosophy. By following these steps, AI systems can be designed to work reliably and transparently. Hendrycks' insights serve as a potent reminder that anticipating and mitigating potential safety concerns proactively is paramount when developing advanced AI technologies to ensure a safe and beneficial future.
The common pattern we see with AI failures is that they often emerge only after deployment. This suggests that many issues, potentially identifiable during the initial coding phases, get amplified and become more problematic when systems interact with the real world. The complex integrations within many AI frameworks further highlight the need for rigorous oversight at the code level. If we can implement thorough checks during the coding process, we might be able to prevent entire cascades of failures down the line.
Integrating comprehensive code audits into the development process can prove extremely useful in spotting vulnerabilities and biases in AI algorithms before they cause harm. By identifying and addressing these potential issues early on, we drastically reduce the risk of facing major problems during deployment. Moreover, a focus on code-level scrutiny fosters a continuous feedback loop, which in turn enhances the AI system's ability to learn and adapt, potentially leading to improved accuracy and reduced bias.
Unfortunately, a common approach is to rely on reactive safety measures after a system has already been deployed. However, we can argue that proactive measures implemented during the initial coding stages could prevent many problems from arising at all. This strategy becomes even more crucial when considering the complex and often opaque nature of AI algorithms.
Making coding practices more transparent allows developers to meticulously trace how decisions are made within an AI system. This enhanced transparency can be essential for understanding and ultimately preventing harmful outcomes. A related aspect is bias mitigation. Early intervention in the coding process helps prevent the accidental reinforcement of biases that might be present in training data. This underscores the importance of safety and fairness considerations from the very beginning.
Greater human involvement during the coding phase also acts as a valuable safeguard against unforeseen communication issues and deployment problems. It also makes a strong argument for cross-disciplinary insights. By bringing in perspectives from fields outside of traditional computer science, such as psychology or sociology, we can establish stronger foundations for safe and ethical AI from the very first conceptualization of the code.
The potential for AI systems to respond to user input in a dynamic and adaptive way hinges, to a large extent, on the original code. Thus, the design choices we make during coding play a crucial role in guaranteeing responsiveness during deployment. Understanding this dynamic and adapting our approach at the code level are key to mitigating potential issues later on.
Dan Hendrycks' 7 Key Insights on AI Safety Measures at xAI and Their Impact on Voice Technology Development - Real World Testing Requirements for AI Voice Systems Before Market Release
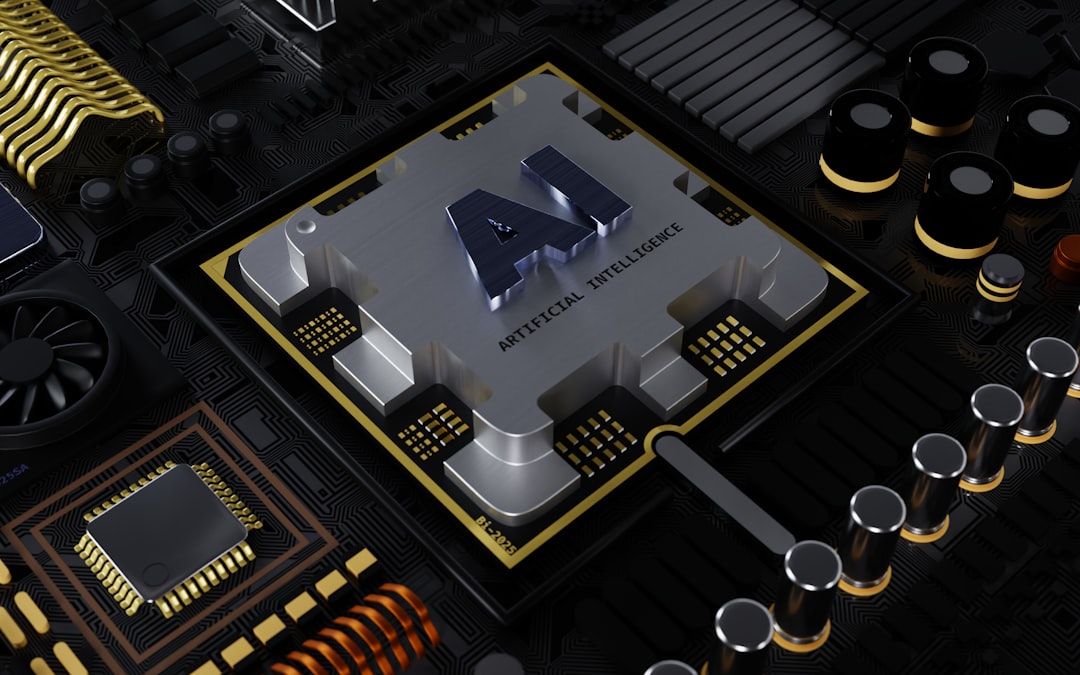
Before releasing AI voice systems to the public, thorough real-world testing is crucial to ensure safety and reliability. Dan Hendrycks strongly suggests that developers carry out rigorous testing that mimics how users will actually interact with these systems. This helps uncover potential weaknesses that may not show up during the development process. He argues that such tests shouldn't be just a formality but a fundamental part of any AI safety strategy. Without comprehensive assessments, the chances of unexpected or harmful behavior from the AI increase, especially as voice technology becomes more integrated into our daily routines. Hendrycks' position highlights the need for a proactive approach to safety, where development focuses on creating a balance between innovation and responsibility in the realm of AI voice systems.
Before releasing AI voice systems into the wild, we need to put them through their paces in realistic settings. We can't just rely on lab tests with carefully curated data; real-world scenarios are critical for uncovering weaknesses. Testing needs to consider a range of accents and dialects, as AI struggles with the subtle variations in how people speak. If we want these systems to be useful to a broad audience, they need to understand diverse forms of language.
Moreover, these AI systems should face scenarios that challenge them – like emergency calls or urgent situations where precision and speed are paramount. It's only under pressure that we truly see how well they hold up. The way humans communicate is inherently unpredictable. Our interactions are rarely scripted, and this inherent variability can expose flaws in how well the AI recognizes our speech. This needs to be part of the testing process to ensure the system can adapt to unexpected twists in conversations.
The consequences of miscommunication can be severe, especially in fields like medicine or law enforcement. Mistakes can have serious impacts, highlighting the ethical dimension of AI voice tech. We need to test for reliability and carefully consider the potential downsides of inaccurate interpretations.
AI systems should not be static; they need to adapt in real-time. Testing needs to evaluate how the systems learn from ongoing interactions with users, both positive and negative feedback, while continuing to function smoothly. A feedback loop is crucial; users need to be able to correct the system's misunderstandings, allowing the AI to improve over time.
We can't ignore the privacy aspect either. Voice systems often capture very personal data, and testing must focus on ensuring user data remains safe and secure. Systems need to be built to comply with privacy standards and to guard against potential breaches.
Also, we need to recognize that how the system operates can vary across different hardware setups. Testing must involve diverse combinations of microphones, speakers, and other equipment to gauge the impact of hardware on the AI's performance. It's an important reminder that software and hardware are tightly coupled.
Finally, we can't just rely on a single test phase. We need ongoing, long-term testing to monitor the system's performance over time. This allows us to understand if the system degrades or improves and helps us adapt safety protocols as the technology changes. This constant monitoring and evaluation are crucial in the ever-evolving world of AI, allowing us to develop safer and more robust AI voice systems.
Dan Hendrycks' 7 Key Insights on AI Safety Measures at xAI and Their Impact on Voice Technology Development - Independent Auditing Standards Proposed for Voice Technology Companies
A new wave of regulation is being proposed for the burgeoning field of voice technology, centered around independent auditing standards. The goal is to create a more structured approach to ensure AI systems developed for voice recognition and interaction are thoroughly vetted for potential problems. This push for independent auditing is seen as a way to proactively identify vulnerabilities, like security risks or biases, in the algorithms and hardware that power these systems, before they are released for public use. This initiative draws inspiration from the safety-focused research of AI expert, Dan Hendrycks, who has been vocal about the need for rigorous testing and transparent development practices. It’s believed that by introducing independent audits and promoting controlled testing environments, the industry can build more trust and confidence in the safety of AI voice technologies. As these systems become more prevalent in everyday life, the need for transparency and accountability is paramount to address legitimate societal concerns around potential misuse and unintended consequences of advanced AI. Ultimately, this move towards independent auditing is a step towards promoting a more responsible and ethical approach to AI innovation, specifically within the growing sphere of voice technologies.
1. Developing independent auditing standards specifically for voice technology companies is a big deal because it allows for methodical checks of how well these systems work and who's responsible when things go wrong in AI-generated voice interactions. This is particularly crucial as the field of voice recognition grows.
2. Current research suggests that voice recognition systems aren't always accurate for different groups of people. This highlights the need for standards that address any hidden biases in how the AI is trained, ensuring that everyone gets a fair and equal experience when using these technologies.
3. The move towards hardware-based safety measures for voice tech could significantly reduce the chance of malicious actors exploiting the system. This suggests that relying only on software for safety might not be enough, and that hardware limitations might be needed to improve the overall security.
4. Laws and regulations for voice technology often seem to be a step behind the speed at which AI is developing. This makes auditing standards super important for staying ahead of potential risks before they escalate into larger incidents or cases of misuse.
5. Voice systems need to adapt quickly to different ways people speak. Therefore, setting performance benchmarks through independent audits can shed light on areas where systems don't fully grasp various accents or speech styles. This information is crucial for improvement.
6. Transparency in how AI algorithms make decisions becomes even more critical when voice technology touches sensitive fields like healthcare or law enforcement. This emphasizes the need for auditing methods that provide insight into how these AI systems behave in real-world situations, making sure we understand how they're arriving at their conclusions.
7. Part of the suggested auditing standards includes thorough real-world testing, which means AI assistants need to demonstrate that they can handle unpredictable scenarios, not just controlled situations. This is a needed step in evaluating their ability to interact effectively in various settings.
8. Using comprehensive feedback loops in the auditing process can improve the AI's ability to learn and adapt, creating a continuous cycle of improvement that's essential to keep pace with changing user expectations for voice technologies.
9. An unexpected positive of independent auditing is that it could provide a clearer path to integrate knowledge from other fields, like psychology. This could help develop voice technology that's more intuitive and attuned to what users want.
10. Regular audits to check if safety standards are being met can not only reduce risks but also serve as a guide for innovation. This ensures that developers can push the boundaries of what's possible with voice technology while protecting users' experience.
Dan Hendrycks' 7 Key Insights on AI Safety Measures at xAI and Their Impact on Voice Technology Development - Mandatory Circuit Breakers and Kill Switches Required in AI Voice Systems
The discussion surrounding AI voice systems is increasingly centered on the need for mandatory circuit breakers and kill switches. Dan Hendrycks, influential in AI safety at xAI, argues that these physical safeguards are critical. He emphasizes that solely relying on software-based controls isn't enough to protect against malicious use or ensure human oversight. The growing integration of voice AI into our daily routines necessitates a robust safety approach encompassing hardware limitations. Implementing circuit breakers and kill switches could prevent unintended harmful outcomes and foster public trust. This emphasis on hard-wired safety measures signals a shift within the AI field, recognizing that innovation should be accompanied by responsible development practices. It suggests that as AI voice technology continues to evolve, the need for carefully considered safety measures will be paramount.
AI voice systems are becoming increasingly integrated into our lives, and with that comes a growing concern about their safety and potential for misuse. Dan Hendrycks, a leading AI safety researcher, is advocating for a shift in how we design and deploy these systems, emphasizing the critical need for built-in safety mechanisms like mandatory circuit breakers and kill switches.
These circuit breakers, essentially a system's "pause button," would be triggered when the AI encounters unexpected situations or deviations from its intended purpose. This could include instances of misinterpreting user commands or producing outputs that stray from desired outcomes. Their role is to prevent potentially harmful consequences by temporarily halting operations until the issue is resolved.
Kill switches, on the other hand, offer a more immediate and decisive way to halt operation. They become crucial in high-stakes situations, like those encountered in healthcare or legal settings, where the system needs to be quickly shut down if it exhibits problematic behavior. Having a clear way to disable a system can provide crucial peace of mind to users and potentially limit damage in the event of unforeseen issues.
One key concern highlighted by Hendrycks is the vulnerability of many current AI voice systems due to a lack of hardware boundaries. Without these constraints, malicious actors could potentially exploit weaknesses within the system. Incorporating circuit breakers and kill switches as part of the hardware can create a stronger defense against potential threats, enhancing the overall resilience and security of the system.
Another challenge is AI's struggle with understanding the complexities of human language and context. It's not uncommon for AI voice systems to misunderstand nuanced requests or expressions, leading to errors or unwanted outcomes. Designing circuit breakers that trigger when the system lacks confidence in interpreting a user input can help mitigate these issues.
Similarly, biases present in the training data can lead to skewed and potentially harmful outputs. Implementing kill switches that activate when bias detection tools flag problematic responses can help prevent these harmful outputs from reaching users, especially vulnerable populations.
Beyond these immediate safety concerns, Hendrycks emphasizes the importance of circuit breakers and kill switches for fostering a more responsible AI development ecosystem. For example, a system's ability to adapt in real-time is crucial. Circuit breakers can help facilitate this process by temporarily pausing the system during unexpected changes in user interaction or environmental factors, allowing it to reset and adjust before proceeding.
Furthermore, the ethical dimension of AI voice systems necessitates strong safety protocols. Circuit breakers can act as a vital safeguard by halting operations if a privacy breach occurs, minimizing the risk of sensitive information being inappropriately processed or stored.
The issue of accountability is also central to the discussion around AI safety. In the absence of robust safety measures, it can become challenging to determine who is responsible when AI voice systems lead to negative outcomes. Kill switches and circuit breakers can provide a framework for understanding these scenarios and establishing clear lines of responsibility.
We often focus on software-based auditing to monitor AI systems, but this can overlook the technical limitations of the underlying system. Mandatory hardware-based safety mechanisms, like circuit breakers and kill switches, provide a tangible approach to reinforce accountability and complement existing auditing practices.
Hendrycks' push for mandatory safety measures reflects a necessary shift in the AI landscape. It underscores the idea that safeguarding users should be an integral part of development, not an afterthought. By incorporating safety measures at both the software and hardware levels, we can strive towards a future where AI voice technology can seamlessly enhance our lives while prioritizing safety and responsible innovation.
Dan Hendrycks' 7 Key Insights on AI Safety Measures at xAI and Their Impact on Voice Technology Development - Training Data Transparency Rules Essential for Voice AI Development
Developing and deploying AI voice technologies necessitates a renewed focus on the transparency of the training data used to build these systems. Concerns about potential copyright violations and the ethical use of data are driving calls for stricter rules around training data transparency. There's a growing understanding that regulations requiring developers to be open about the data used to train AI models are vital for fostering responsible development.
As voice AI becomes more intertwined with our daily activities, the need for clarity about data authenticity, user consent, and potential biases embedded within training data becomes increasingly urgent. Without this transparency, there are significant risks, including issues with data quality, potential discrimination, and a dampening of public trust. Hendrycks, an expert in AI safety, emphasizes the importance of establishing rules for disclosing training data practices. He sees a lack of such rules as a potential barrier to progress and a threat to the ethical deployment of AI voice technologies.
Legal and ethical concerns regarding the use of data in training AI, specifically voice AI, are becoming increasingly prominent. Experts argue that the lack of transparency around training data sources poses a risk to both creators and users. Media outlets and legal professionals are calling for regulations that mandate transparency regarding the data used to train AI models, particularly in light of copyright concerns and the potential for unethical data use.
Dan Hendrycks, a key figure in AI safety research and advisor at xAI, emphasizes the urgent need to understand the safety implications of AI development, especially for voice technologies that are increasingly integrated into our lives. He highlights the emerging need for a legal framework governing training data for AI systems, a framework that moves beyond the current GDPR and focuses on establishing quality metrics for all AI training datasets.
There's a growing worry about the authenticity, consent, and origin of the data used to train generative AI systems. Many creators are concerned about how their content might be utilized without their knowledge or consent. This issue is further highlighted by the potential enactment of the AI Foundation Model Transparency Act, a bill currently under consideration that could establish federal guidelines for AI training data transparency.
The risks associated with inadequate training data are manifold. Concerns range from poor data quality that degrades AI performance to the potential for algorithmic biases that can discriminate against certain groups. Additionally, the lack of clear guidelines about training data usage could hinder innovation in the field of AI as developers face increasing scrutiny and uncertainty.
To address these concerns, researchers and policy makers are exploring a new governance framework. This framework seeks to regulate the entire lifecycle of AI training data, especially for high-risk AI systems. There's a growing consensus that AI companies should be required to disclose the data used in their model training.
Hendrycks' work underscores the need for safety considerations within AI development. His advocacy for transparency and safety measures makes him a prominent voice in a field grappling with the potential downsides of rapidly evolving technologies. The need for regulations to ensure ethical data handling and responsible AI development is becoming more and more critical as AI systems become integrated into the fabric of society.
More Posts from transcribethis.io: