Exploring OpenAI Whisper A Deep Dive into Audio Transcription Efficiency
Exploring OpenAI Whisper A Deep Dive into Audio Transcription Efficiency - Training data of 680,000 hours powers Whisper's broad understanding
OpenAI's Whisper model benefits from a truly massive training dataset spanning 680,000 hours of audio. This extensive dataset, gathered from a variety of sources and encompassing multiple languages and tasks, is a key factor in Whisper's strong performance. It's this breadth of data that allows Whisper to handle diverse accents and background noise effectively, and to understand and transcribe speech in a remarkable 98 languages. The sheer volume of data, far exceeding typical academic datasets, is critical in allowing Whisper to generalize well to new and unseen audio examples, even without specialized adjustments. This adaptability, achieved through the massive training data and the open-collection approach used in its compilation, makes Whisper a significant leap forward in automatic speech recognition. It demonstrates the potential of large-scale data in building systems that can effectively process a wide range of audio content.
Whisper's impressive grasp of spoken language stems from its training on a massive dataset spanning 680,000 hours. This diverse collection includes a wide range of audio sources, from podcasts and audiobooks to online videos, exposing the model to a rich tapestry of human speech across various styles and contexts. It's worth considering that such an enormous dataset likely encompasses hundreds of thousands of unique voices, which may explain Whisper's capability to handle diverse accents and speaking styles.
This extensive training, arguably far exceeding typical academic datasets that are limited to a few thousand hours, is a key driver of Whisper's ability to recognize and transcribe over 100 languages. The implication of this wide language coverage is substantial, particularly for fields like media and global communication.
While training on audio was crucial, the incorporation of aligned text proved equally important. It’s likely that this paired data greatly contributed to Whisper's ability to contextualize the spoken word, accurately capturing punctuation and even nuances like sarcasm. The model also takes advantage of a technique called transfer learning. This means that even after exposure to a specific audio domain like medical or legal transcription, its ability to perform those specialized tasks enhances noticeably, revealing a remarkable adaptability to niche applications.
The sheer scale of training this model demands a considerable amount of computational power. This aspect highlights the intricate algorithms involved and challenges the boundaries of existing hardware. Even with such impressive power, Whisper's limitations remain. It is susceptible to errors when handling lesser-known languages or technical jargon, hinting at avenues for future enhancement.
The sheer size and source of Whisper's training data have ignited concerns about privacy and consent. Using audio data without explicit consent raises critical questions, especially when the origins of this data extend to industrial sources. The continuing development of models like Whisper is symbolic of a wider trend in machine learning where bigger datasets and more efficient algorithms fuel advancements. However, the resulting models also complicate evaluating performance and overall reliability.
Exploring OpenAI Whisper A Deep Dive into Audio Transcription Efficiency - Multilingual support for over 400 languages and accents
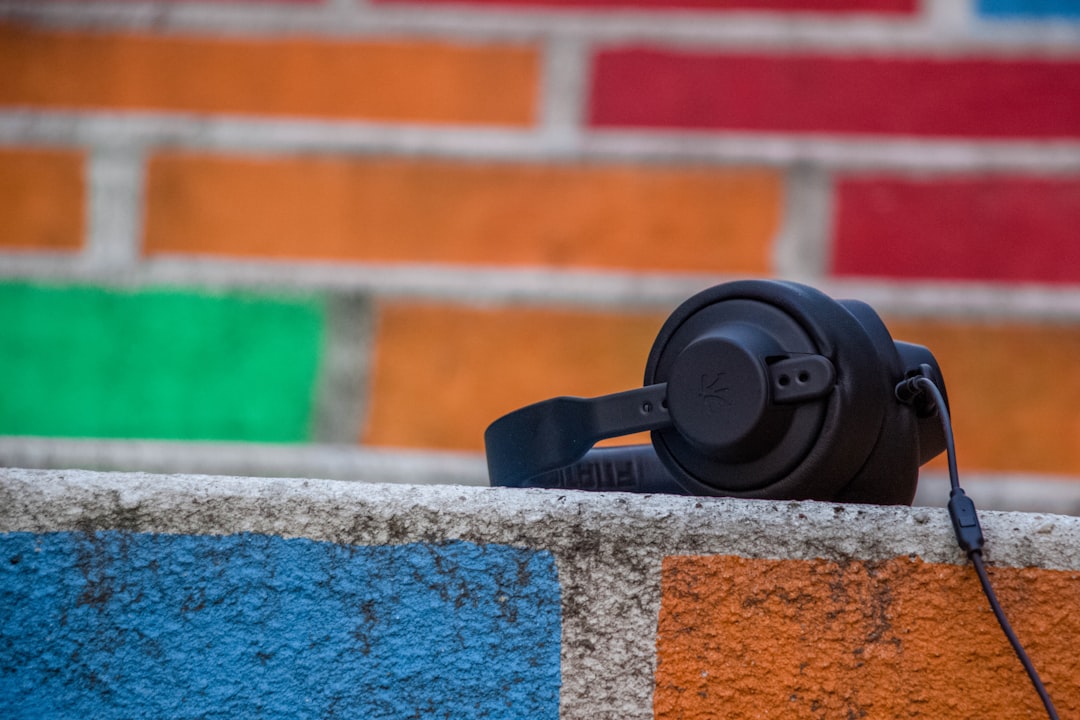
OpenAI's Whisper model boasts impressive multilingual capabilities, handling over 400 languages and their associated accents. This extensive linguistic reach stems from its training on a vast and diverse collection of audio data. The model's exposure to a wide range of speech patterns allows it to adapt to various speaking styles and even filter out background noise, a common hurdle in real-world audio scenarios. Notably, Whisper's developers focused on enhancing its accuracy across diverse accents and specialized language, making it a flexible tool for communication across cultures and technical fields.
While Whisper achieves a high level of multilingual accuracy, it's important to acknowledge that it isn't flawless. It may struggle with less common languages or highly technical jargon, hinting that further development is warranted. Overall, Whisper marks a considerable advancement in automatic speech recognition, showcasing the power of deep learning to handle the complexity of human language across borders and communication styles. It's a testament to the potential of artificial intelligence for global communication and collaboration.
OpenAI's Whisper boasts support for over 400 languages and accents, a testament to its expansive training data and intricate model design. This impressive feat allows it to handle a wide range of regional pronunciations, showcasing its potential to bridge communication gaps across cultures. The sheer breadth of languages and accents recognized suggests a sophisticated language model capable of capturing a diverse array of linguistic patterns. It's interesting that this multilingual prowess isn't solely a product of massive data but also a result of how the language model is structured.
Whisper's ability to cope with noisy audio is promising for real-world use cases. It seems the model has effectively learned to filter out extraneous sounds and prioritize speech, a critical skill for transcription in settings like crowded streets or bustling offices. This suggests an ability to prioritize and parse audio signals even when the recording quality isn’t ideal.
Interestingly, Whisper's proficiency extends beyond simple transcription, potentially incorporating elements of cultural context into its understanding. This hints at the model being able to capture not just the literal words but also the underlying cultural nuances inherent in language, which could prove invaluable in various applications.
Despite its advancements, Whisper, like other AI models, isn't without its limitations. It's prone to propagating errors across languages, which underscores the ongoing need for model refinements, particularly when dealing with the linguistic intricacies of lesser-known dialects. This raises questions about how these errors propagate and how we can prevent it during training.
Whisper's training on diverse audio contexts, like casual conversations versus formal speeches, seems to help the model adapt to different speech patterns. However, it leads us to question how well it manages situations where these contexts are mixed together during transcription. This multi-faceted training and its relation to error propagation is worth exploring further.
While Whisper supports a multitude of languages, there's an observable drop in performance for languages with limited training data or resources. Addressing this disparity is crucial for ensuring fair and equitable transcription across the spectrum of languages. This reinforces the point that while Whisper has impressive capabilities, further refinement is needed to bring less represented languages up to par with others.
Finally, the open-source nature of Whisper and its involvement of the wider community is a fascinating aspect. This continuous feedback loop, where users provide input on model behavior, offers a pathway for continuous improvement. It seems like OpenAI is trying to incorporate human-based correction and validation into a larger model optimization process, which could be a powerful approach to enhance language processing systems.
This multifaceted approach to multilingual and multi-accent transcription is truly fascinating, but it also raises a variety of questions for future study. Whisper’s strengths and weaknesses offer a good example of how language models are evolving, and highlights the complexities of building truly universal language processing systems.
Exploring OpenAI Whisper A Deep Dive into Audio Transcription Efficiency - Generalist approach vs specialized models trade-offs
OpenAI's Whisper, with its generalist approach to audio transcription, exemplifies the inherent trade-offs between broad applicability and specialized performance. Trained on a massive and diverse dataset, Whisper demonstrates remarkable ability to handle a wide range of languages and accents, making it a versatile tool for many different transcription needs. However, this broad training comes at a cost. When dealing with highly specific audio contexts or limited data sets, generalist models can struggle to achieve the level of precision that specialized, fine-tuned models often offer. This suggests a crucial balance – the wider usefulness of generalist models often comes with the sacrifice of finer accuracy compared to more focused systems. Recognizing these trade-offs is becoming increasingly important as we refine and apply audio transcription technology across increasingly diverse applications. The choice between a generalist solution and a specialized one will depend greatly on the specific needs and desired outcome of the transcription process.
OpenAI's Whisper, being a generalist model, can tackle a wide range of audio transcription tasks, which can simplify deployment and reduce the need for multiple specialized systems. However, this versatility comes at the cost of potentially needing a massive dataset, like the 680,000 hours used for Whisper. Specialized models, on the other hand, often excel in very specific areas, requiring less training data that's tailored to their niche.
A generalist model's performance might be more uniform across different situations, while a specialist model might shine in its area of expertise but falter when confronted with anything outside of its typical inputs. This highlights a key trade-off—reliability versus specialized accuracy.
Fine-tuning, a technique for improving model performance, is usually more impactful on specialized models because they're more receptive to domain-specific details. Generalist models, with their broader training goals, might not show as dramatic an improvement when fine-tuned.
It seems that models like Whisper, trained on varied audio and contexts, might demonstrate better performance in real-world scenarios. They learn to handle a wide range of noises and speech styles, unlike specialized models, which may be optimized for ideal audio conditions. This adaptability comes at a price, though—training a generalist model requires substantial computational resources, potentially acting as a barrier for some projects.
While a generalist model's errors may be less predictable due to varying levels of accuracy across languages, the error patterns in a specialized model are often more consistent and easier to understand. This highlights that generalist models embrace inclusivity in design, aiming to serve a broad user base. Specialized models, conversely, are often developed for a particular set of needs, potentially excluding others.
The ethical considerations around data privacy become more complex for models like Whisper, which utilize a huge and varied collection of audio. Specialized models may be trained on more controlled data sources, making compliance a bit easier.
Although generalist models are advantageous for taking advantage of breakthroughs across various fields, keeping specialized models around can still be valuable for tasks where absolute precision is crucial. This suggests that a blended approach, using both specialized and generalist models, might be the most effective in many situations. This 'best of both worlds' approach offers a compelling route forward for improving the accuracy and applicability of speech recognition systems.
Exploring OpenAI Whisper A Deep Dive into Audio Transcription Efficiency - Deep learning techniques behind Whisper's text conversion
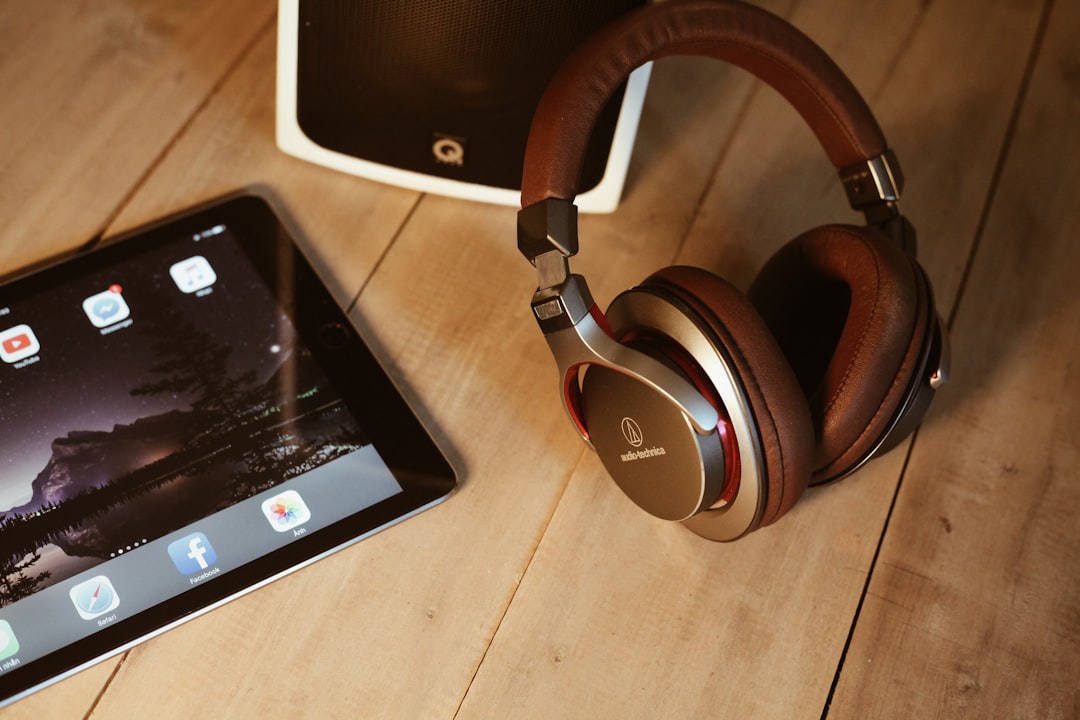
OpenAI's Whisper utilizes advanced deep learning techniques to achieve impressive audio transcription and text conversion. The model's foundation is an encoder-decoder Transformer architecture, which processes audio by converting it into log-Mel spectrograms. This approach allows the model to segment long audio files into manageable chunks and generate accurate transcriptions through autoregressive prediction. Whisper's strength lies in its multilingual capabilities, handling over 100 languages with impressive accuracy. However, this generalist design can also present limitations. Whisper's ability to navigate specific jargon or lesser-known languages can be inconsistent, highlighting a potential trade-off between versatility and specialized performance. The ongoing development of Whisper demonstrates the power of large-scale datasets and sophisticated algorithms, but also emphasizes the need for ongoing improvements to ensure consistency and accuracy across a wide variety of audio sources.
Whisper, OpenAI's creation, is built upon a transformer architecture, a modern deep learning approach that uses self-attention to process audio. This unique design allows the model to grasp the intricate relationships within speech, contributing to its enhanced accuracy compared to older speech recognition systems. Unlike many other models, Whisper takes an "end-to-end" approach, handling the entire audio transcription process—from raw sound to written text—within a single integrated structure. This streamlined approach simplifies the process and optimizes performance in a holistic way.
To bolster its adaptability, Whisper incorporates data augmentation techniques like adding artificial noise, adjusting the pitch, and altering the tempo of the audio during training. These tricks help the model become more robust and resilient to various acoustic conditions encountered in the real world, enhancing its transcription performance in diverse scenarios. The model's multilingual abilities are also aided by language modeling techniques that assess the likelihood of different word sequences. This feature not only transcribes the sounds but also cleverly infers the most probable words in cases of unclear or ambiguous pronunciation, based on the broader context of the speech.
Whisper benefits from the concept of transfer learning, making it readily adaptable to specialized domains like medicine or technology. This ability to swiftly tailor its skills to new contexts is crucial, allowing it to excel in specific areas without needing a complete retraining cycle. The model also employs multi-task learning to enhance its performance in handling multiple languages simultaneously. This approach helps it share features learned across different languages, leading to an overall boost in its capabilities.
Moreover, Whisper has been trained to accommodate a wide variety of speech patterns, including regional accents, slang, and varying levels of clarity in pronunciation. This thorough training equips it to perform well even when faced with speech that differs considerably from standard speech patterns. It's worth noting that the transformer's architecture contains a sophisticated attention mechanism that intelligently focuses on important parts of the audio. This helps the model to prioritize relevant speech segments amidst background sounds, boosting transcription accuracy in noisy settings.
The model's training incorporates aligned text data, which significantly improves its ability to accurately insert punctuation and formatting into the transcription output. This reduces the need for extra steps to correct the final text, leading to cleaner and easier-to-read results. Notably, Whisper is built with continual learning in mind. This implies that as new audio data becomes available, the model can be updated and refined without the need for extensive retraining. This feature paves the way for future improvements and adaptations as speech patterns and needs continue to evolve.
While it's shown promise, it is important to remain aware of the limitations in Whisper's current abilities as with all large language models and to be mindful that further advancements and refinements are likely required to ensure that this technology becomes fully reliable and trustworthy in real-world situations.
Exploring OpenAI Whisper A Deep Dive into Audio Transcription Efficiency - Accessibility for various user groups from engineers to students
OpenAI's Whisper model offers broad accessibility across various user groups, including engineers and students. Its capacity for real-time audio transcription and translation makes it beneficial for individuals with hearing impairments and enhances accessibility for a wider audience. Whisper's ability to streamline audio processing makes it useful for various tasks and projects, from engineering endeavors to student research, improving efficiency and making information easier to manage. While Whisper's generalist nature benefits many, limitations exist when dealing with highly specialized vocabulary or less common languages. These limitations point to a need for continued development in niche applications. In essence, Whisper's potential for fostering inclusivity and providing accessible audio tools is considerable, despite areas that require further improvement.
OpenAI's Whisper, with its ability to transcribe audio across a wide range of languages and accents, offers a unique opportunity to improve accessibility for diverse user groups. While primarily designed as a generalist model, its potential benefits extend beyond just those with hearing impairments. Engineers, for instance, can benefit from Whisper's noise resilience, making it a valuable tool in collaborative environments. The model's ability to tackle some technical jargon, though not perfect, shows promise for streamlining workflows in many disciplines. Similarly, students, particularly those whose native languages aren't English, can use Whisper to access educational materials more readily, potentially lessening the digital divide.
Whisper's capacity for real-time transcription has interesting implications for various learning styles. Visual learners, for example, might find it easier to follow a lecture if they can read the transcription simultaneously. And for auditory learners, its capacity to capture subtle speech patterns and context may be more beneficial compared to a solely written transcript. Furthermore, this real-time transcription feature opens the door to more inclusive collaborations, enabling individuals with hearing impairments to participate in discussions without needing extra assistance.
However, Whisper isn't a panacea. Its limitations, like inconsistencies in handling less-common languages and certain technical jargons, highlight the ongoing need for refinement and data enhancement. Engineers and researchers could leverage Whisper's open-source nature to actively collect and refine its training data, thereby tailoring it to specific technical vocabularies and less represented languages. Ultimately, the ability of models like Whisper to successfully navigate a variety of speech styles and contexts with some degree of accuracy offers valuable feedback loops for researchers interested in critical listening and understanding. This is particularly crucial for students, where nuanced interpretation of complex topics requires deep comprehension and can lead to a greater understanding.
The potential for real-time applications is another aspect that warrants further investigation. Emergency response situations, for example, could benefit immensely from the model's ability to rapidly transcribe crucial information, bridging language barriers and improving communication in critical moments. It's through these real-world applications, and the continuous refinements of the model, that we can see how accessibility, both in terms of usability and knowledge dissemination, can be further enhanced for diverse user groups. However, we must remain mindful of the inherent limitations of generalist models in specialized contexts and the ongoing need to ensure responsible development and implementation of these systems.
Exploring OpenAI Whisper A Deep Dive into Audio Transcription Efficiency - Real-time transcription capabilities via API integration
OpenAI's Whisper, through its API, enables real-time audio transcription, making it a useful tool for scenarios needing immediate audio processing. This functionality allows for continuous audio capture and transcription, where timestamps are added to the output for easier review. The API handles a variety of audio formats, and developers can leverage its capabilities by processing audio in smaller segments, providing flexibility in usage. Whisper's performance in real-time transcription is impressive in many settings but isn't without its flaws. Specifically, dealing with unique jargon or less common languages can sometimes be a challenge, emphasizing the need for further development. The potential of Whisper's real-time transcription capabilities is undeniable, but its journey towards achieving accuracy across a broader range of audio requires continuous improvements. Striking a balance between versatility and the precision of transcriptions remains a focal point in the ongoing development of this technology.
OpenAI's Whisper, through its API, is currently in beta for paying developers and leverages the new GPT-4o model for its audio capabilities. A new model, gpt4oaudiopreview, is expected soon and will accept either text or audio as input to the GPT-4o model. This suggests that the focus may be shifting towards a more versatile model that can manage different input types. Whisper's core strength is its ability to process speech in real-time. It does this by continually capturing audio and combining raw audio bytes from multiple recordings, which is interesting as it provides a unique perspective on how real-time audio can be processed.
Researchers have shown Whisper can transcribe live audio in near real-time, a capability useful for real-time keyword spotting and for building systems that record conversations with timestamps. However, while Whisper benefits from an expansive training dataset and is proficient in many languages, it may not outperform highly specialized models on specific benchmarks like LibriSpeech, which is something to consider when picking a model for a certain task.
Whisper's API accommodates a variety of audio file formats, including common options like mp3 and wav, but imposes a 25MB limit for uploads. Developers can utilize the Whisper API by building a system that processes chunks of audio. The whispercpp library seems to be a common tool in this space. The model even has a lightweight variant, Tiny TFLite, that has been successfully used for real-time transcriptions in desktop applications, which could suggest it's well-suited for specific computing resources.
For larger audio files, users can split them into smaller sections using the pydub library. Current implementations seem to suggest Whisper is a remarkably efficient model, with the ability to use up to 3000 GPUs for performance optimization, which shows that these are computationally intense models that can also be very efficient. It is interesting that the model can scale this dramatically, potentially creating new possibilities for different types of transcription tasks. Overall, early implementations of Whisper demonstrate it has great potential for high-performance audio transcriptions. But as we know with any language model, further enhancements will probably be needed, especially when it comes to dealing with edge cases and highly specific language or technical jargon.
More Posts from transcribethis.io: