Experience error-free AI audio transcription that's faster and cheaper than human transcription and includes speaker recognition by default! (Get started for free)
Efficiency vs
Quality Analyzing the Trade-offs in Online Video Compression Tools
Efficiency vs
Quality Analyzing the Trade-offs in Online Video Compression Tools - Video compression artifacts and their impact on visual quality
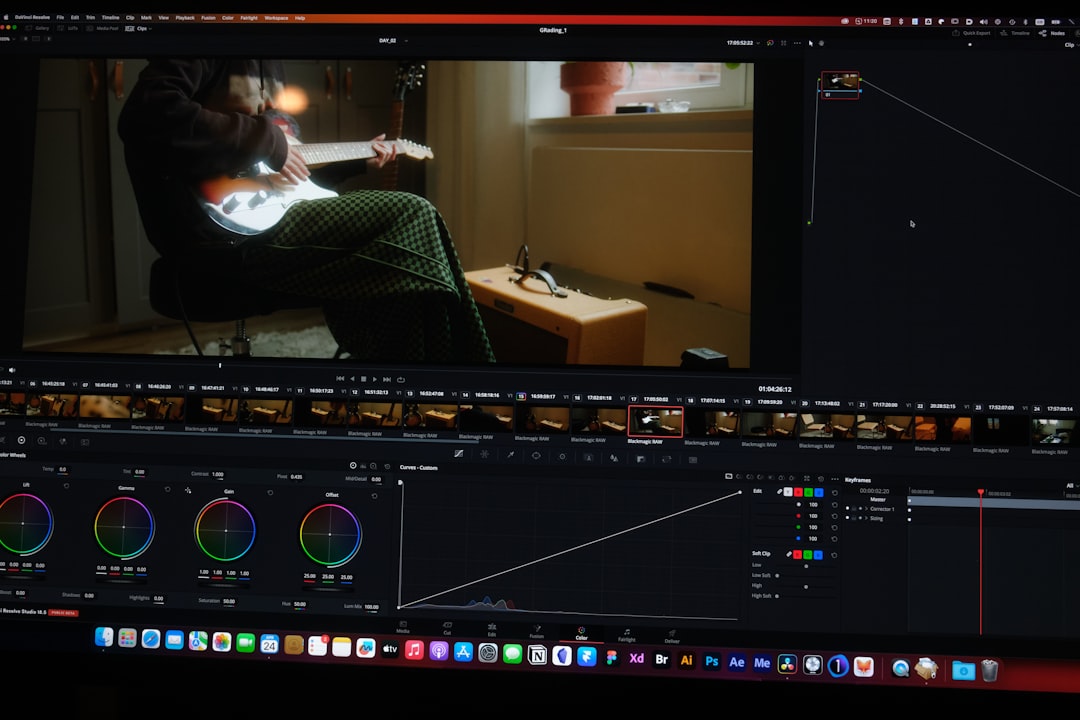
Video compression is a balancing act. On one hand, we want to shrink files so they load quickly. But on the other, we don't want to make the video look like a pixelated mess. These compression "artifacts," the glitches and distortions that crop up, are the price we pay for smaller file sizes. They can be especially distracting when you pause the video, revealing blocky areas, or when motion creates jerky, unnatural movements.
The challenge lies in finding the sweet spot, where compression is efficient enough for quick loading, but doesn't result in a noticeably degraded viewing experience. Choosing the right settings, like the bitrate (which determines the amount of data used) and how the video is broken down into frames (GOP structure), makes a big difference in the quality of the final product.
Unfortunately, while there are ways to try and smooth out these artifacts, they aren't always perfect. Often, they try to fix everything, including areas that don't need it, leading to strange, unnatural-looking results. So finding solutions that can specifically target these artifacts, and minimize the harm they cause, is an important goal for video compression, as we continue to rely on digital content more and more.
The pursuit of efficient video compression inevitably leads to the introduction of artifacts, those visual imperfections that arise from the loss of information during the compression process. These artifacts, like the infamous blocking, banding, and blurring, are unwelcome byproducts of our desire to reduce file size, often sacrificing visual quality in the process. It's fascinating to see how different compression methods, like the widely used H.264 or newer codecs like HEVC and AV1, produce unique artifact patterns. It's almost as if each algorithm has its own signature style.
These artifacts tend to be more pronounced in scenes with rapid motion, where the compression algorithm struggles to accurately capture the fast-changing visuals. Imagine a dramatic chase scene, where the frantic movements of the characters are reduced to a flurry of pixelated edges and smeared backgrounds. This is a classic example of how compression can compromise the richness of the original content.
Furthermore, certain compression settings can heavily influence the visual quality. Pushing the compression ratio too far can result in a barrage of artifacts, including the dreaded "mosquito noise" that blurs edges and obscures important visual details. It's almost as if these artifacts are biting away at the sharpness and clarity of the original image.
Of course, some post-processing techniques, like sharpening or denoising, can partially mitigate the visual impact of these artifacts. However, applying them incorrectly can amplify existing problems, creating a chaotic visual mess. It's a delicate balancing act between cleaning up the image and further distorting it.
The human eye, with its remarkable sensitivity to luminance changes, is more easily distracted by artifacts that affect brightness than those affecting color. This means a slight change in the intensity of light can be more noticeable than a subtle color shift.
While our tolerance for artifacts can vary depending on the content and the context (we might accept some artifacting in a fast-paced video game stream but expect a higher fidelity in a cinematic film), it's crucial to understand that even small adjustments in compression settings can dramatically affect the visual outcome. It's a constant game of balancing efficiency and quality, with the goal of delivering a satisfying viewing experience without sacrificing the essence of the original content.
The ongoing research and development in video compression algorithms is fascinating. Although significant advancements have been made, the trade-off between efficiency and quality remains a key challenge, particularly in minimizing the emergence of unwanted visual artifacts. It's an intricate dance between science and art, where the goal is to find the sweet spot that balances compression efficiency with preserving the integrity of the visual information.
Efficiency vs
Quality Analyzing the Trade-offs in Online Video Compression Tools - The role of bitrate in balancing file size and video fidelity
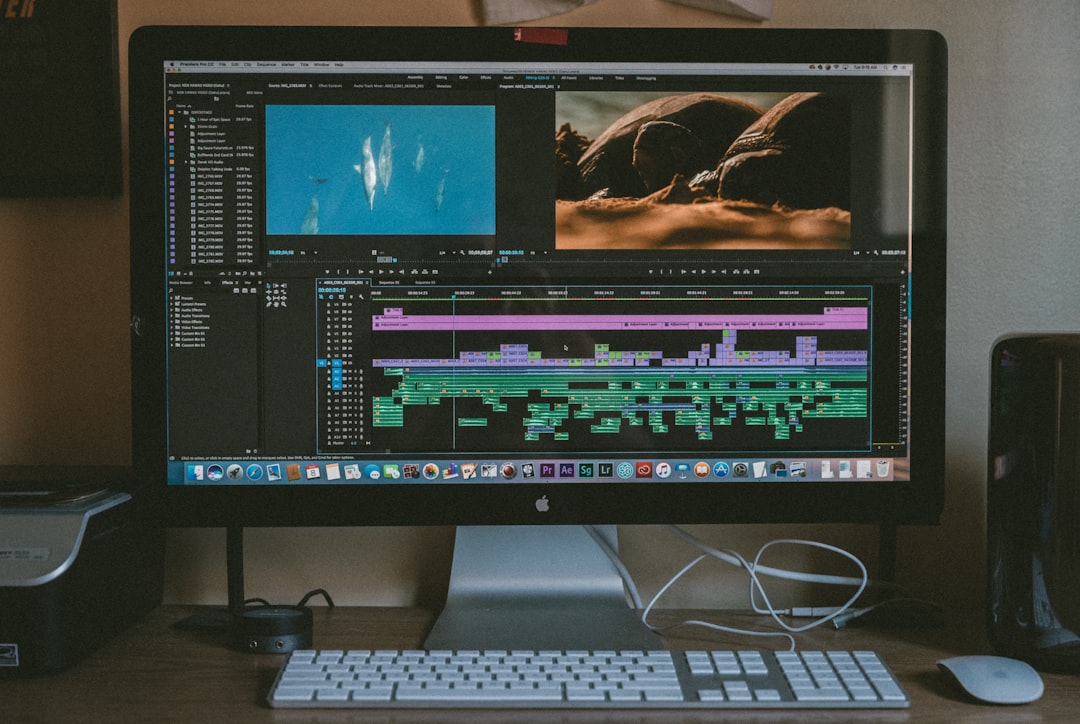
The bitrate, essentially the amount of data used per second for a video, dictates the delicate balance between file size and picture quality. It's a bit like a seesaw: pushing for a smaller file size (lower bitrate) often results in a less detailed, possibly blurry image, while aiming for a sharper, more lifelike picture (higher bitrate) means a bigger file. Thankfully, clever encoding techniques like Variable Bitrate (VBR) are now allowing for some flexibility here. VBR allocates more data to busy scenes with lots of action and less to simpler ones, ensuring that the viewer doesn't notice a drop in quality in important moments while keeping file sizes manageable. However, the quest for smaller files does come with a price. If you reduce the bitrate too much, you can get unwanted artifacts like pixelation or blockiness. So, navigating this balancing act skillfully is key for delivering high-quality videos without losing viewers to buffering or frustrated downloads.
Bitrate is a key factor in the constant struggle between video file size and visual quality. It represents the maximum data allowed per second, with higher rates generally leading to better quality but also larger file sizes.
The relationship between bitrate and perceived quality is complex and can be predicted using models like Shannon's entropy. It's not a linear relationship; doubling the bitrate doesn't necessarily double the quality. This is often seen in subjective assessments of video fidelity.
Variable bitrate (VBR) encoding offers a more flexible approach, dynamically adjusting data transmission based on scene complexity. This can result in smaller file sizes during less demanding parts of the video while maintaining quality during action sequences. This optimization is crucial for bandwidth-sensitive streaming applications.
The choice of compression codec also influences how bitrate affects quality. H.265, for example, can achieve comparable quality to H.264 at half the bitrate, demonstrating technological advancements in compression. However, this also necessitates increased computational power for encoding and decoding.
Research has established that viewers have a threshold for perceiving detail loss. Bitrate reductions below certain levels, usually around 2 Mbps for 1080p video, lead to noticeable degradation in quality. This highlights the need to carefully select settings for specific viewing scenarios.
Adaptive bitrate streaming technology dynamically adjusts the bitrate during playback based on internet speed, optimizing for a smooth viewing experience. This system, however, requires a robust infrastructure to implement effectively.
While reducing bitrate can significantly decrease file size, it often results in clipping and artifacts, particularly in high-motion scenes. This trade-off begs the question: is smaller file size worth a decrease in quality, especially for professional content?
Higher bitrates require more complex encoding, which can increase workflow time, especially for advanced codecs. This means that achieving efficient compression involves more than just file size and visual fidelity but also affects production timelines.
Human visual perception studies suggest viewers prioritize different aspects of video quality depending on content type. For example, viewers might be more tolerant of artifacts in action sequences compared to static scenes, showcasing the subjective nature of quality perception.
Higher frame rates, such as 60 fps, require higher bitrates to maintain visual quality compared to lower frame rates like 30 fps. However, achieving high frame rates presents logistical challenges in both compression and playback. Finding the right balance between bitrate and frame rate is crucial for effective video design.
The constant evolution of compression algorithms and the continued exploration of the intricate relationship between efficiency and quality continue to be key challenges for video compression. Balancing these factors is an ongoing dance between science and art, with the goal of delivering a satisfying visual experience while preserving the integrity of the original content.
Efficiency vs
Quality Analyzing the Trade-offs in Online Video Compression Tools - Comparing efficiency of AV1, VVC, and HEVC codecs
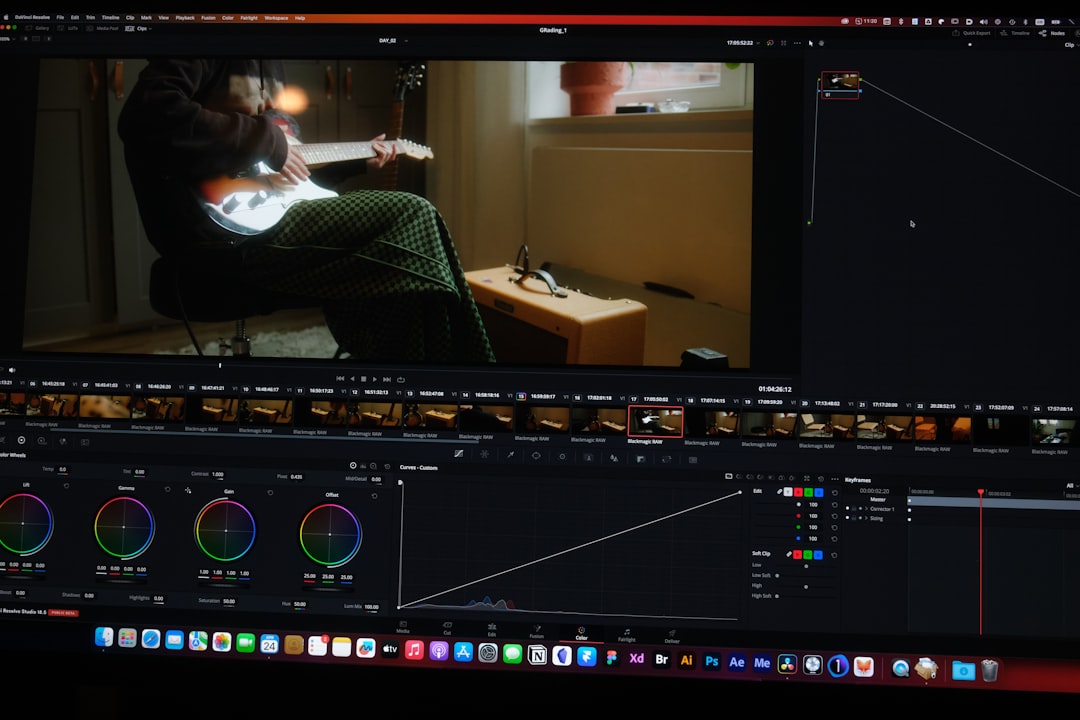
AV1, VVC, and HEVC each offer unique strengths when it comes to video compression. AV1 is a royalty-free option that's particularly strong at reducing bitrates, which is ideal for online video distribution. While it can achieve comparable quality to HEVC, its real strength shines at lower bitrates. VVC, a more recent codec, is designed to handle high-resolution video with incredible efficiency. In specific conditions, it can outperform both AV1 and HEVC, making it a compelling choice for high-quality content. HEVC, a well-established codec, does a decent job but struggles to keep up with the demands of modern online video consumption. Choosing the right codec ultimately comes down to the content itself and the delicate balance between efficiency and quality.
The pursuit of efficient video compression has led to the development of several promising codecs, including AV1, VVC, and HEVC. Each codec brings its unique set of strengths and weaknesses to the table, making the choice for any given scenario a complex one.
AV1, a royalty-free codec, boasts impressive compression efficiency, sometimes achieving up to 30% better results than HEVC at the same quality level. This efficiency makes it an attractive option for streaming services looking to optimize bandwidth without sacrificing user experience. However, AV1 requires significantly more computational power during encoding than HEVC, leading to longer processing times and increased resource usage, which can pose challenges for real-time applications.
VVC (Versatile Video Coding), on the other hand, excels in encoding high-dynamic-range (HDR) content and supports up to 12-bit color depth, making it ideal for high-quality video applications like professional media and entertainment. However, HEVC typically exhibits lower latency in decoding than AV1, which is a critical factor for live streaming scenarios where delays can impact viewer experience.
HEVC, although not royalty-free, continues to be widely adopted due to its robust feature set and its ability to deliver good quality at reasonable bitrates. It offers multiple profiles, including Main, Main10, and others, allowing users to choose a balance between performance and quality based on specific requirements.
While AV1 may be advantageous for lower-bitrate streaming applications, research indicates that HEVC may still outperform AV1 in scenarios with less motion or static backgrounds, demonstrating the importance of considering content context when selecting a codec. Additionally, VVC incorporates features that enhance its resilience to transmission errors, proving useful for applications in unreliable network conditions like mobile streaming in crowded areas or outdoor events.
Ultimately, the decision of which codec to use is a balancing act, weighing factors like compression efficiency, processing demands, latency, error resilience, adoption rates, and licensing complexities. As technology continues to advance, we can expect even more sophisticated codecs to emerge, further refining the trade-off between compression efficiency and visual quality.
Efficiency vs
Quality Analyzing the Trade-offs in Online Video Compression Tools - Multiple Transform Set (MTS) as a middle ground solution
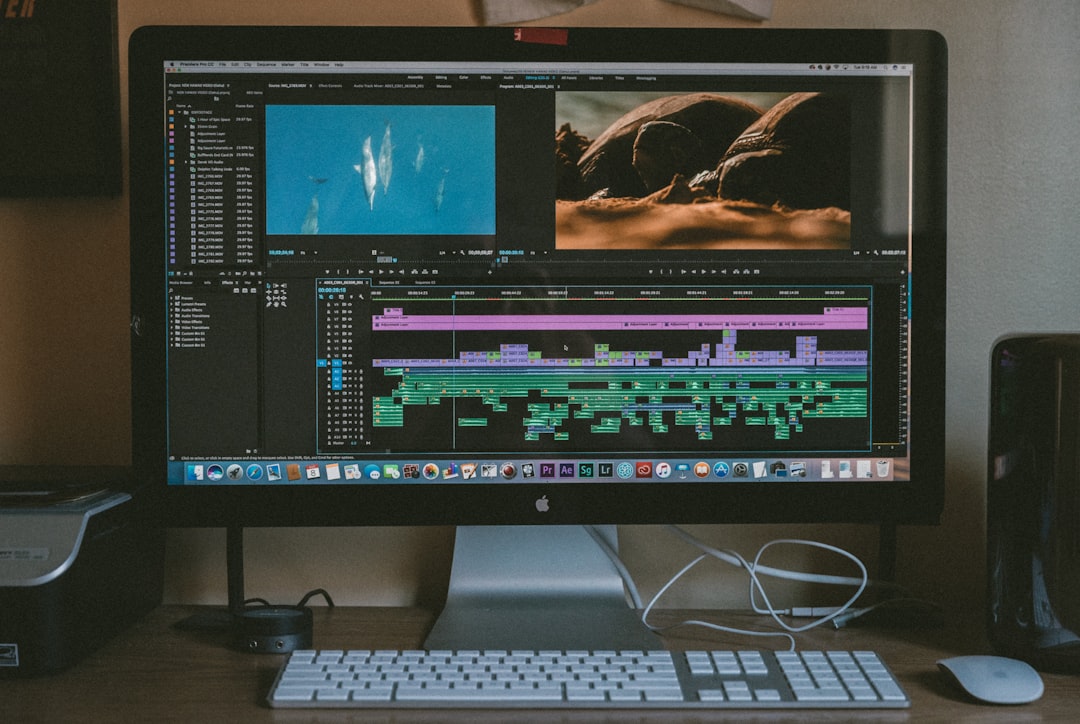
The Multiple Transform Set (MTS) presents a middle ground in video compression, attempting to achieve both efficiency and quality. It does this by using a clever system to choose the best way to break down the video during encoding. This selection process, based on Rate-Distortion Optimization (RDO), helps to compress the video better without sacrificing too much visual quality. MTS is particularly important in the VVC codec, which while providing better compression than its predecessor HEVC, also requires more processing power. With new features like the ability to skip some of the processing steps, MTS can intelligently adjust its strategy depending on the specific video being encoded, preventing significant quality loss that's often associated with very efficient compression. As we demand more from our video streaming experiences, MTS becomes a crucial tool in the quest for efficient, high-quality video without overwhelming our devices.
Multiple Transform Set (MTS) is a fascinating new approach to video compression. It's like a Swiss Army Knife of compression algorithms, employing a range of transforms to handle different types of content and minimize unwanted artifacts. The idea behind MTS is that instead of just picking one compression method, it dynamically chooses the best transform depending on what's happening in the video.
This adaptability makes MTS really good at dealing with videos that have a mix of different types of content, like a scene with lots of movement followed by a still shot. It can focus on compressing the more complex scenes efficiently without sacrificing the quality of the simpler ones.
What's really cool is that MTS uses machine learning to analyze past videos. This means that it can learn from past mistakes and automatically tweak its settings to be more efficient. It's like giving the algorithm a memory so it can get better at compressing videos over time.
Another neat aspect of MTS is that it can encode video in multiple layers. This is great for online streaming because viewers with slower connections can start with a lower quality version and gradually upgrade as their bandwidth increases.
So, MTS isn't just about throwing a bunch of different transforms at a video; it's about using them intelligently and customizing the compression process for the specific content. It's a great example of how video compression is moving beyond the "one size fits all" approach and towards more adaptive and intelligent solutions.
Efficiency vs
Quality Analyzing the Trade-offs in Online Video Compression Tools - Sustainability considerations in video encoding processes
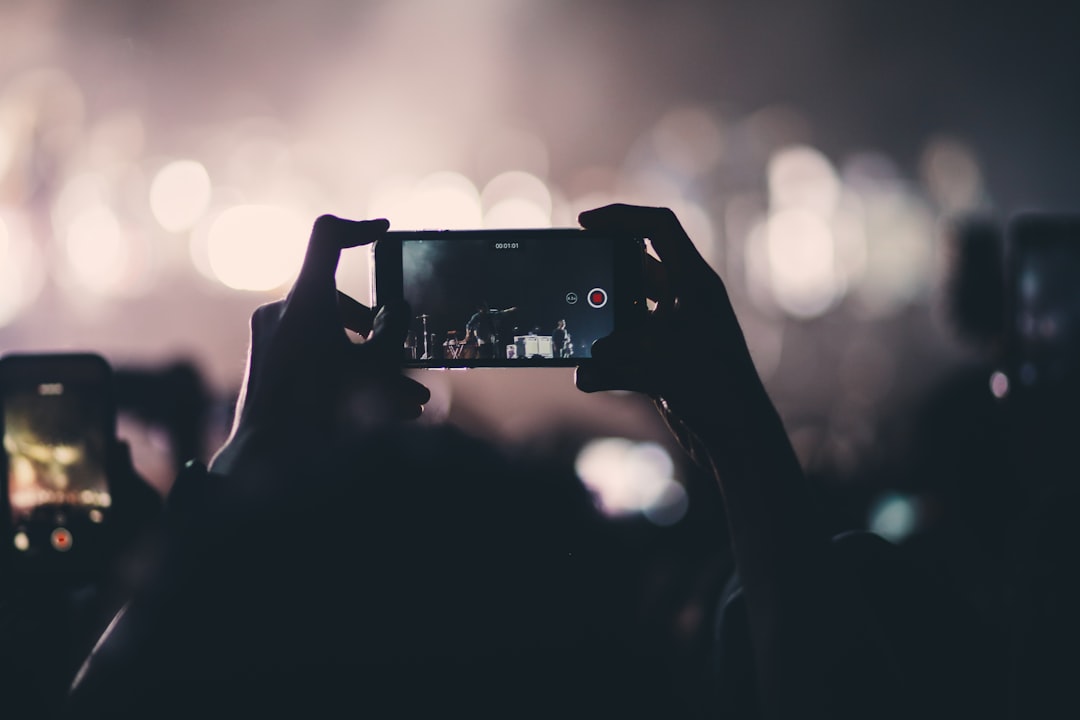
Video encoding is undergoing a shift, where sustainability is no longer a mere afterthought but a core consideration. As online video consumption explodes, so does the energy footprint of streaming services. This has led to a focus on more efficient encoding methods that go beyond just delivering smooth playback. HTTP Adaptive Streaming (HAS) is now being explored as a way to reduce energy consumption by intelligently managing bitrates. This means videos are divided into segments encoded at varying quality levels, providing a bitrate "ladder" that lets viewers select the optimal quality based on their connection speed. This, in turn, minimizes energy wasted on unnecessary data transmission.
Additionally, the development of AI-powered encoding solutions and newer codecs like AV1 and VVC is pushing the boundaries of compression efficiency. These advancements promise to reduce bitrates without sacrificing visual quality, directly translating to lower energy demands for streaming platforms. It's clear that the future of video encoding lies in finding a harmonious balance between delivering a satisfying visual experience and minimizing the environmental impact of our digital content consumption.
It's fascinating to see how the choice of video encoding algorithms impacts energy consumption. Certain codecs, particularly newer ones like AV1, are more computationally intensive, demanding more power during compression and playback. This increased power demand, particularly within large data centers, is a key concern for sustainability. It's a bit like a trade-off - we want the most efficient codecs to reduce file sizes, but the processing overhead can negate some of the benefits.
Adaptive bitrate streaming, a technology that adjusts the video quality based on your internet speed, can also play a role. While it has the potential to make streaming more efficient by reducing server load during peak times, frequent bitrate switching can actually add to resource usage. It's a delicate balancing act - getting it right can be good for the environment, but a poor implementation could actually do the opposite.
The complexity of these codecs also affects hardware. More complex codecs, like AV1, put more strain on encoding hardware, potentially leading to faster wear and tear. This could mean more frequent equipment replacement, resulting in more electronic waste.
The issue of cross-compatibility also needs consideration. While newer codecs like AV1 and VVC offer improvements, they sometimes aren't compatible with older devices. This can lead to users needing to upgrade their hardware sooner than expected, increasing overall resource consumption. It's a chicken and egg scenario - newer technologies can offer benefits, but they can also force older systems to be replaced, contributing to a cycle of consumption.
Another interesting consideration is the preservation of original content quality. While there's been progress, certain compression techniques still struggle to maintain the quality of highly detailed content, particularly 4K and HDR videos. It's almost as if we're losing some of the data we capture, leading to a situation where we're not able to fully take advantage of the increasing resolutions we're capable of.
Machine learning is also starting to play a bigger role in video compression. These AI-based enhancements can dynamically optimize compression techniques, which is great for resource utilization. However, the initial computational demands for encoding can be significant. It's almost like having to do more work upfront to get more efficiency in the long run.
Rate-Distortion Optimization (RDO) is another clever trick. It helps determine the best way to compress the video based on the specific content and scene. While effective, it can lead to inconsistent processing loads, making it difficult to predict how resources will be used.
Finally, we have to consider the cascading effects of reducing file sizes. Smaller videos take less time to load, but they also require less data to transmit. This can be good for the network, but it can also lead to congestion and increased energy consumption in the infrastructure. It's a bit of a paradox - the quest for efficiency can lead to increased overall resource use.
There are also temporal trade-offs to consider. Real-time streaming applications like video conferencing often prioritize low latency, which can compromise video quality. This can put additional stress on networks and bandwidth, highlighting a complex interaction between speed and quality.
Video compression is a multi-faceted problem. Every encoding decision has a ripple effect throughout the entire system. It's a challenge to balance the desire for higher quality, efficient delivery, and sustainable practices, and it will be interesting to see how these issues are addressed as video technology continues to evolve.
Efficiency vs
Quality Analyzing the Trade-offs in Online Video Compression Tools - Strategies for optimizing compression in Video on Demand applications
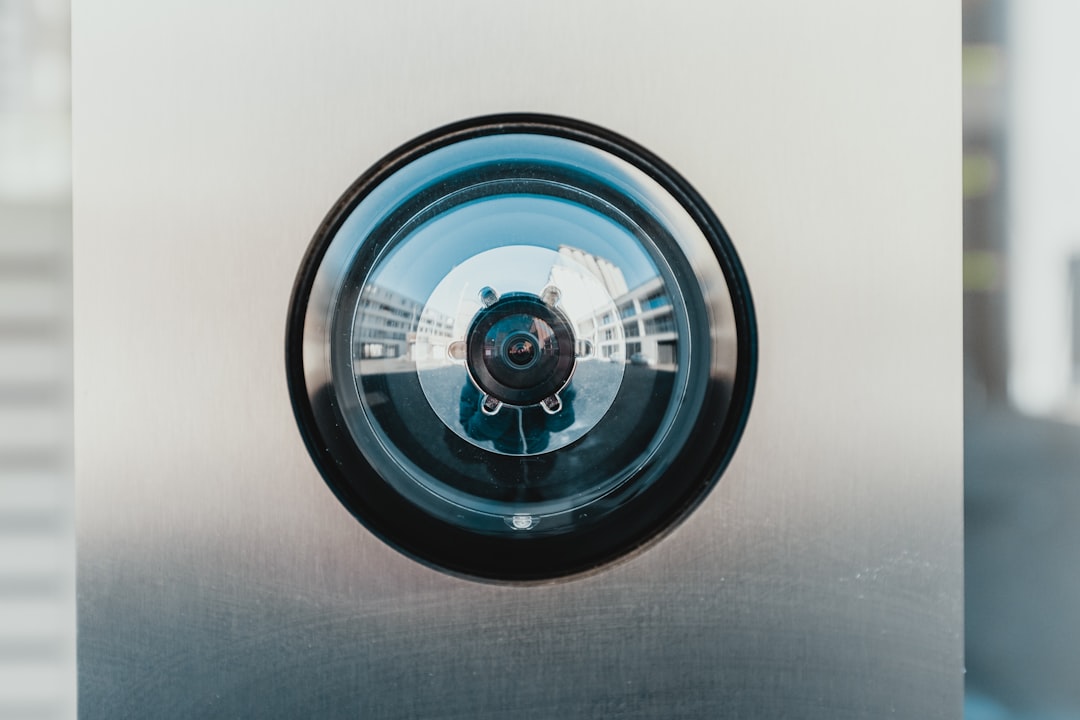
Optimizing compression in Video on Demand (VoD) services is a balancing act between delivering high-quality video and ensuring fast loading times. One approach is using AI to intelligently compress the video, focusing on areas less crucial to the viewer's experience. Advanced codecs like AV1 and VVC allow for better bitrate management, squeezing more detail into smaller files. The way the video is broken down into frames (GOP structure) and how frequently keyframes appear are also crucial, as poorly chosen settings can introduce distracting glitches and artifacts. With the rise of high-resolution video, finding the right compression strategy is essential for keeping viewers happy and content.
Optimizing video compression for Video on Demand (VOD) applications is a complex dance between efficiency and quality. While the goal is to minimize file size for quick loading times, we must also ensure the video looks good. Here's a look at some strategies that researchers and engineers are exploring:
1. **Encoding Time Efficiency**: It’s not just about the final file size; the time it takes to encode a video matters too. New codecs like AV1 can achieve remarkable compression, but they can take significantly longer to process compared to older options like H.264. This time lag presents a challenge for content creators under tight deadlines.
2. **Machine Learning in Video Encoding**: The use of machine learning (ML) in video compression is a game changer. ML algorithms can analyze videos and make smart adjustments to the compression process based on what they see. For example, they can prioritize quality in scenes with a lot of action while applying more aggressive compression to static background scenes. This dynamic approach helps maintain viewer satisfaction while minimizing file size.
3. **Beyond PSNR**: Traditional measures of video quality like PSNR (Peak Signal-to-Noise Ratio) aren't always a good indicator of how a video *actually* looks to a human viewer. New metrics like VMAF (Video Multi-Method Assessment Fusion) are being developed to better capture the subjective experience of quality, leading to more intuitive optimization strategies.
4. **Strategic Bitrate Allocation**: Advanced video compression can leverage "perceptual encoding" techniques, meaning they don't treat all parts of a video the same. They focus more data (higher bitrates) on areas that are important to the viewer, like faces or action sequences, while reducing the data allocated to less visually critical parts. This targeted approach helps maintain a good viewing experience with minimal increases in file size.
5. **Resolutions and Compression Efficiency**: Surprisingly, higher resolution videos don't always mean significantly larger files. Advanced codecs like VVC can sometimes compress 4K video to sizes comparable to 1080p. This is an exciting development as it shows that modern compression can push the boundaries of what we expect in terms of file size.
6. **Heterogeneous Encoding**: Video encoding isn't always a "one size fits all" approach. In some cases, different codecs can be used within the same video stream depending on the content. This means a film with a lot of dialogue might have higher quality encoding for speech-heavy scenes while action sequences might be compressed more aggressively. This tailored approach can lead to a better overall experience.
7. **Rate-Distortion Optimization (RDO)**: RDO is a sophisticated technique that finds the best encoding settings by carefully analyzing the video content. It considers both the potential loss in quality and the gains in compression efficiency, ultimately achieving a balance that can sometimes produce unexpected optimization results.
8. **Content-Aware Encoding**: This method goes beyond simply analyzing the visual elements of a video. It takes into account the type of content. For instance, sports broadcasts often contain data like cards and statistics, which might require sharper quality compared to a simple background scene. This targeted approach can lead to better viewer experiences and smaller file sizes without sacrificing the necessary details.
9. **Temporal Compression**: Video compression often exploits "temporal dependencies", meaning it uses information from previous frames to predict what will happen in the current frame, effectively compressing the video based on the way the content moves. While this can be highly efficient, it also adds complexity to the encoding process and requires considerable computational power for prediction.
10. **Compression's Nonlinearity**: Compression isn't a straight line. Making small reductions in the bitrate can lead to minimal quality loss, but further reductions can quickly introduce noticeable artifacts. This means engineers need to be careful about how much they compress a video and avoid crossing thresholds that would seriously affect the viewing experience.
It's clear that video compression is a continuously evolving field. Researchers and engineers are constantly seeking new and creative ways to achieve the optimal balance between efficiency and quality. As we continue to produce and consume more video content, these innovations will be crucial for ensuring that our video streaming experience remains enjoyable, reliable, and sustainable.
Experience error-free AI audio transcription that's faster and cheaper than human transcription and includes speaker recognition by default! (Get started for free)
More Posts from transcribethis.io: