7 Key Developments in AI-Powered Live Video Translation Through 2024
7 Key Developments in AI-Powered Live Video Translation Through 2024 - Real-Time ASR Integration Enables 150ms Latency in Video Translation
Real-time video translation has seen a remarkable leap forward with the integration of Automatic Speech Recognition (ASR) systems. This integration has unlocked the potential for ultra-low latency, with some systems achieving a remarkable 150 milliseconds delay. This progress is driven by improvements in both hardware and software, with companies like Speechmatics leading the charge through partnerships like their role on the NVIDIA Holoscan platform.
Furthermore, cloud platforms are refining their speech translation offerings. Tools like Azure's API now allow for continuous translated captions, making real-time understanding during video interactions more seamless. This advancement demonstrates a growing focus on the user experience in live video translation scenarios. Independent efforts like StreamSpeech showcase the potential for highly efficient, real-time streaming ASR that can handle tasks like simultaneous translation with minimal delays.
As this field rapidly evolves, the emphasis on increasing ASR speed and accuracy remains paramount. The pursuit of ever-faster and more precise speech recognition promises to fundamentally alter how AI handles live video translation, particularly in the upcoming year.
Reaching a mere 150 milliseconds of delay in video translation is quite impressive, and it's made possible through clever engineering. These systems use intricate algorithms to pre-process audio data and rely on fast data transfer methods, enabling almost immediate communication with minimal noticeable lag. It's really fascinating how close this gets us to a truly natural conversational experience.
However, getting to this level of speed involves using increasingly complex machine learning models for the automatic speech recognition (ASR) component. These models are constantly being refined by processing mountains of language data across many languages. It's a constant balancing act between speed and accuracy.
One hurdle we face is the quality of the audio. If there's too much background noise or people have different accents, it can really mess with the transcription process and slow things down. This highlights a tradeoff between robustness and real-time performance.
Interestingly, bringing the computing closer to where the audio is captured, using edge computing, helps a lot. Instead of relying solely on cloud processing, we get faster results. This shift seems like a good way to make things faster and more responsive.
Achieving this 150ms target is noteworthy because it's about the same amount of time people take to react in conversations. This means we're getting closer to making video interactions feel quite natural. Imagine being able to instantly understand someone speaking a different language in a video chat – that's the dream!
And the best part? This technology can handle numerous languages simultaneously, opening up communication for more diverse audiences without much delay.
A big part of these advances comes down to improvements in neural network designs. The latest designs can handle processing audio streams in parallel. This means doing multiple things at once, such as recognizing speech and then translating it, all without a noticeable dip in quality.
While we're making huge strides, there's still work to be done. Languages are complex, and some present more challenges than others, thanks to differences in pronunciation, grammar, and regional variations. Model designers must account for these aspects to maintain consistent performance across languages.
It would be incredibly helpful if we could incorporate user feedback into the ASR systems. Having the ability to dynamically adjust these systems based on what people are saying in real time could improve both speed and accuracy.
We're witnessing the 150ms threshold emerging as a benchmark in the field. But researchers aren't stopping there. The race to shave off even more milliseconds continues, driven by the desire to achieve a seamless translation experience that's essentially instantaneous.
7 Key Developments in AI-Powered Live Video Translation Through 2024 - Meta Releases Cross-Language Voice Synthesis for 100+ Languages
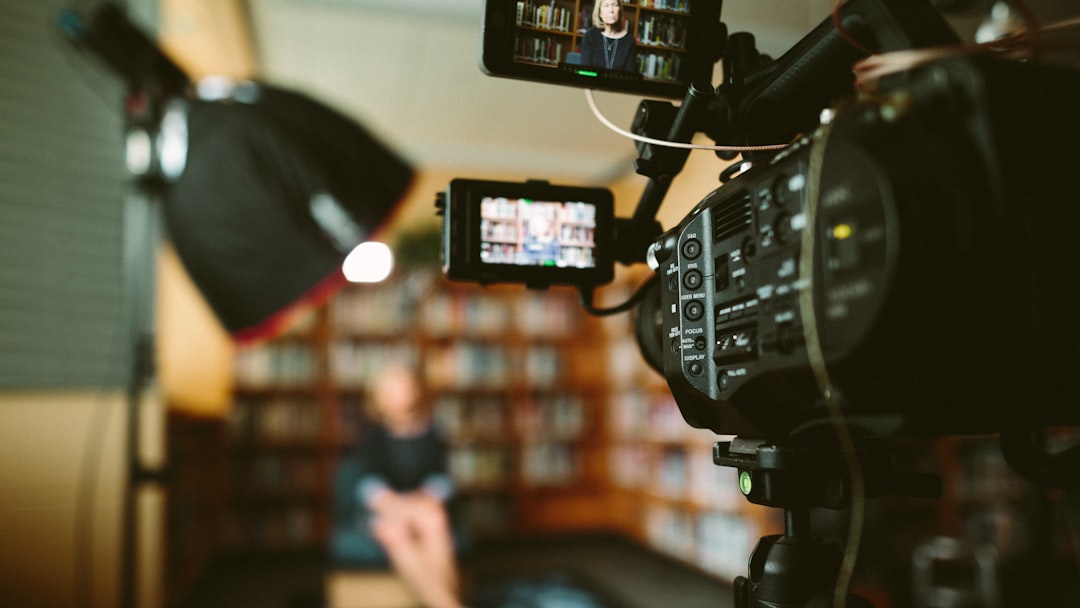
Meta's recent release of a new AI model, SeamlessM4T, represents a significant advancement in the field of cross-language voice synthesis. It can now translate and synthesize speech in over 100 languages, focusing on producing high-quality audio with natural-sounding voices. This model leverages prior efforts like the No Language Left Behind project, expanding its capabilities beyond text translation to encompass a broader range of audio-based communication. The core goal is to break down language barriers, allowing people to interact more easily regardless of their native tongue. SeamlessM4T does this by combining speech recognition, synthesis, and language identification into a unified system.
Importantly, Meta has made SeamlessM4T accessible to researchers through a research license. This decision emphasizes a commitment to fostering open development within the AI community, encouraging researchers and developers to explore its capabilities for various communication applications. While this is an encouraging development for bridging communication gaps, the project still faces hurdles. One of the biggest challenges has been acquiring sufficient high-quality audio data for many languages. Due to the scarcity of available audio data in some languages, the project has even resorted to utilizing resources like religious texts to build up their datasets. This highlights the complex reality of applying AI in language processing, where overcoming data limitations remains a key obstacle to broader access and fairness.
Meta has unveiled a new model capable of generating speech in over 100 languages, aiming to bridge the gap in cross-language communication. This is a significant step, particularly for languages with limited digital resources, as it showcases how AI can handle diverse linguistic structures. At the heart of this development is the SeamlessM4T model, which is designed to handle a variety of tasks within the realm of machine translation, encompassing both text and speech. This builds upon Meta's earlier work with the No Language Left Behind (NLLB) project, which focused on text-to-text translation across 200 languages.
SeamlessM4T goes further by integrating speech recognition, synthesis, and language identification capabilities across an impressive 1,100+ languages. While impressive, we are still left wondering about the accuracy and consistency of the model's performance across such a broad spectrum of languages. Meta, continuing its open-source approach, has released the model under a research license, enabling developers to experiment with it and contribute to its further development. The underlying goal is to facilitate communication by reducing language barriers. This focus on seamless communication is especially relevant with the increasing global interconnectedness seen in today's world.
However, the path to creating such a robust system is fraught with challenges. One of the biggest hurdles was finding sufficient audio data for all these languages. Many existing datasets have a strong bias towards certain languages, leaving many underrepresented. Meta's clever approach to this was to use religious texts, like the Bible, as a source for audio data, as these resources were more widely available in different languages. This is a novel approach and it will be interesting to see how the availability of data impacts the model's overall performance in the long run.
The SeamlessM4T model also tries to preserve the nuances of pronunciation and speech patterns in the translated output. This aspect of voice quality is essential, but also represents a significant challenge. One of the key research directions now will be to see how closely these generated voices can approximate the original speaker's voice style and emotional content. Meta's advances in this field show promise for broader applications of AI in video interactions, making communication across languages potentially smoother and more intuitive. It will be fascinating to observe how this model evolves and integrates into other real-world applications, such as live video translation or language learning platforms. It does appear to be yet another step towards the ultimate goal of enabling frictionless communication for everyone, but it's clear that a lot of work remains.
7 Key Developments in AI-Powered Live Video Translation Through 2024 - DeepL Launches Video Translation API with Context-Aware Processing
DeepL has launched a new API specifically designed for translating video content. A key feature of this API is its ability to consider context when generating translations. This helps improve the accuracy of real-time video translations, especially when dealing with potentially ambiguous language. The API offers translation support for a dozen languages and can output translations in 33 languages, suggesting an attempt to broaden the reach of translated content.
DeepL has also incorporated a context parameter within its API. This allows users to provide additional information relevant to the video, potentially improving the accuracy of the resulting translation. This is particularly useful in scenarios where the translated content might be susceptible to misinterpretation without further context. It's worth noting that including this context data doesn't increase the cost of using the API.
These developments by DeepL represent a move towards more accurate and sophisticated real-time video translations. Whether it significantly impacts the user experience remains to be seen. It's still unclear how well it handles complex or nuanced language, especially when considering regional variations and different accents. Despite these potential issues, the API could have a positive influence on facilitating communication across a wider range of languages and could contribute to making video content accessible to more people around the world. This is certainly a direction that we expect to see the industry move further in.
DeepL has introduced a new Video Translation API that leverages something they call "context-aware processing." This essentially means the system tries to understand the meaning of a phrase not just by looking at individual words, but by also considering the surrounding words and the general topic. This should lead to translations that are more accurate and relevant to the actual content of the video.
The API can currently handle translations from 12 languages into 33, which is a decent starting point but could be further expanded. Notably, they have integrated their DeepL Voice technology, which allows for real-time translation of text in live conversations and video calls. I find this interesting, as the ability to engage in a live discussion while simultaneously getting a translation is useful for cross-cultural collaboration. It shows the intent to go beyond simple subtitling.
A key part of the API is the "context parameter," which allows developers to provide extra information about the content being translated. This can improve the accuracy of translations in cases where there might be ambiguity or multiple meanings. What's interesting about the implementation is that the characters within this context parameter are not included in the pricing, so you can essentially give the system more hints without paying more for it. DeepL has also made it easier to use this feature, by revising their API documentation to be more user-friendly for developers.
Moreover, DeepL has made it easier to manage access to the API. They now allow both free and paid users to manage multiple API keys within a single account. This was implemented in the first quarter of 2024. Interestingly, DeepL has been touting their newest language model as being better than ChatGPT-4, Google, and Microsoft's offerings in terms of overall translation quality. Whether or not that's truly the case remains to be seen, but it's certainly an aggressive claim.
This new API's ability to handle multiple languages simultaneously is quite important in a world where we often need to translate across many languages in a single meeting or live stream. The developers are really striving to make the communication process less of a barrier, and this is a step in that direction.
DeepL, like the other companies in this space, is continuing to develop and refine their AI translation systems. It's exciting to see how much progress has been made in such a short amount of time and the implications for global communication are intriguing. If they can effectively improve the quality and responsiveness of these AI translation tools, it could greatly facilitate collaboration and reduce misunderstanding amongst people who speak different languages. But there's still a long way to go in overcoming language nuances and edge cases, so this is an area we'll need to continue monitoring.
7 Key Developments in AI-Powered Live Video Translation Through 2024 - OpenAI Whisper Large v3 Achieves 95% Accuracy Across 57 Languages
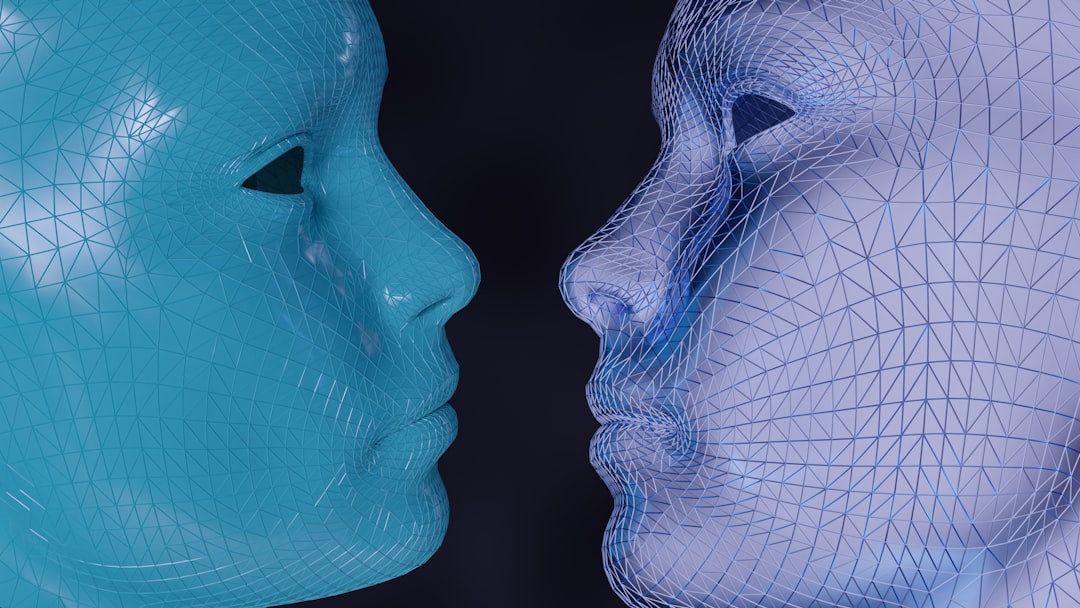
OpenAI's Whisper Large v3 model has achieved a noteworthy 95% accuracy rate in transcribing speech across 57 different languages. This enhanced version of Whisper is designed to handle diverse accents, background noises, and specialized terminology more effectively than earlier iterations, making it more practical for real-world applications. Whisper's remarkable accuracy is attributed to the vast amount of multilingual data it was trained on—over 680,000 hours—and its impressive ability to generalize to new situations without needing specific training. Beyond simple transcription, Whisper is able to produce outputs that include correct punctuation and syntax, suggesting a higher level of understanding of the spoken language. This makes it a promising tool not just for general transcription but also for applications like AI-powered language learning and, importantly, the emerging field of live video translation. By improving the accuracy and speed of automatic speech recognition, models like Whisper help bridge communication barriers across languages.
However, even with these advancements, challenges remain. Languages, with their intricate structures and regional variations, pose persistent obstacles for accurate AI-based understanding. The nuances of speech and context continue to be a focus of development in the field.
OpenAI's Whisper Large v3 has emerged as a noteworthy advancement in automatic speech recognition (ASR), boasting a remarkable 95% accuracy across 57 languages. This level of performance suggests that for many languages, it's on par with human transcription, a testament to the rapid progress in automated language processing. It's particularly impressive because Whisper is designed to handle the complexities of real-world speech, including varied accents, background noise, and even technical jargon, which often trip up previous generations of these models.
A key reason for Whisper's capabilities lies in its extensive training. It's been exposed to over 680,000 hours of diverse multilingual data, collected from the web, effectively providing the model with a very broad understanding of how people speak. That's not all, though. It's also been trained on over 5 million hours of labeled data, allowing it to generalize well in situations it hasn't encountered before. Essentially, it has learned to handle a large array of speaking styles without needing specific examples. This is a common goal in machine learning.
One of the standout features of Whisper v3 is that it generates transcriptions with correct punctuation and syntax, effectively reconstructing the structure of the spoken language. This is quite helpful, as it makes the output directly usable for a wide range of tasks. Its ability to handle conversational nuances, like hesitations or interruptions, further increases its practical utility, particularly in dynamic settings where interactions are less structured.
Already, a variety of tools are incorporating Whisper, including AI-powered language learning platforms. This indicates a growing appreciation for its ability to improve efficiency in tasks like transcription and language education. It has elements that contribute to its high reliability and robustness, keeping it at the forefront of ASR technology. As with all machine learning models, Whisper is continuously being updated, suggesting that we can expect even higher accuracy and performance in the future. It's being put to use in a variety of industries, for anything from transcribing audio from video calls to generating accessible content.
The ability to accurately transcribe audio and video files in multiple languages makes it an exciting development for content creation and accessibility. The potential to make audio and video information more accessible to broader audiences is a compelling application for a technology like this. The development of this model shows the powerful potential of AI in breaking down barriers related to language. But, like other machine learning tools, we must continue to critically examine how it is developed and employed.
7 Key Developments in AI-Powered Live Video Translation Through 2024 - Google Translate Adds Live Camera Translation for 95 Languages
Google Translate has expanded its live camera translation feature to encompass 95 languages, including popular languages like Arabic, Hindi, and Vietnamese. This update offers a more polished user interface, making the translation process feel less abrupt. Notably, it addresses a previous limitation by allowing users to translate text from any of the 100 supported languages, rather than primarily focusing on English. Behind the scenes, Google has been leveraging the PaLM 2 AI model to refine how Google Translate understands and translates related languages. It's also been improving how translated text blends into complex visuals, enhancing the overall naturalness of translated images.
In a related development, Google has also added 110 languages to its translation service in what they are calling their biggest expansion to date. This ambitious expansion could potentially improve communication for hundreds of millions of people around the world. It's exciting to see Google Translate become more inclusive, but it's important to keep in mind that translating between languages with very different structures and nuances presents a continual challenge for AI. The accuracy and true contextual meaning of these AI-powered translations will continue to be a subject of research and improvement in the future.
Google Translate has expanded its live camera translation capabilities to cover 95 languages, including a wide range of commonly used ones like Arabic, Hindi, and Vietnamese. This update isn't just about adding more languages, though. The app now boasts a smoother user experience, with less noticeable jumps or delays during translation. It's a significant improvement over past versions, where the translation process was somewhat clunky.
Previously, Google Translate's camera translation relied heavily on English as the main translation point. Now, it can translate from any of its supported 100 languages, opening up more possibilities for multilingual users. It's fascinating how far the AI model behind it has come. PaLM 2 has played a key role in improving how Google Translate handles languages, especially those that are closely related. For example, it's likely better at translating dialects or regional variations.
Alongside this expanded language support, Google Translate has added 110 new languages to its overall service. They claim it's their biggest expansion yet, which suggests a strong desire to increase the reach of translation services across the globe. This makes me wonder how they plan to handle the quality and accuracy across so many languages in the future. One positive note is that Google's AI seems to have gotten better at merging translations into photos, improving the overall visual quality and blending the text seamlessly.
The Google Lens feature is a nice addition for people who want to quickly search for information using the camera on their phone. While this may seem less related to translation directly, it broadens the usability of the camera beyond simply translating written text. In addition to this, Google Translate has enhanced offline functionality, making translation possible for 59 languages even without an internet connection. This is important for users in areas with limited connectivity or for preserving privacy by keeping data local.
The “Tap to Translate” feature is also quite useful, allowing users to instantly translate text within any app, enhancing accessibility. It's just another example of how translation technology is becoming more integrated into various parts of our digital lives. It's certainly aimed at making communication simpler, and estimates suggest it could potentially help over 614 million more people worldwide, which is a significant impact.
While it seems Google Translate is on the right track, the real-world effectiveness and accuracy still need to be assessed. The challenges that still exist include handling various accents or dialects, regional differences in vocabulary, and even the speed and efficiency of the translation itself. Keeping translation quality high across so many languages with varying writing systems and linguistic structures will certainly be a challenge for the Google team. We'll need to continue to follow the progress and examine how it adapts to the ever-evolving landscape of language.
7 Key Developments in AI-Powered Live Video Translation Through 2024 - Microsoft Teams Implements Universal Live Captions with Voice Cloning
Microsoft Teams has introduced a new feature that provides live captions with real-time translations across multiple languages. This feature currently supports 40 languages, enabling meeting attendees to view captions in their preferred language. A key part of this is the inclusion of voice cloning technology, which allows for more natural-sounding translations by replicating the speaker's voice. Microsoft is calling this new tool "Interpreter" and it's powered by their Azure Cognitive Services, which helps maintain context and quality during translations. While this is a positive development for fostering communication across language barriers, it's also important to consider the challenges of achieving accurate translations for a wide range of dialects and individual speaking styles in real-time situations. It remains to be seen how well it handles diverse accents and speech patterns.
Microsoft Teams has introduced a new feature called "Interpreter" that leverages AI to provide universal live captions with real-time translations. This means meeting participants can see captions in their preferred language, translating from 40 different spoken languages. The system uses voice cloning technology to replicate the natural voice characteristics of the speakers, which is quite impressive from a technical perspective. It’s a testament to how advanced speech synthesis has become. They're using Azure Cognitive Services to power the translation and captioning process, suggesting a focus on quality and contextual understanding. However, to use this feature, you’ll need a Teams Premium subscription.
Interestingly, they can adapt to different languages and accents very quickly, which is pretty cool. Their automatic speech recognition (ASR) component likely uses sophisticated machine learning models to achieve a high degree of accuracy. I imagine these models are refined by processing vast amounts of language data to achieve this. A potential challenge here is ensuring the captions are responsive enough for real-time conversations. Maintaining a short delay, below 200 milliseconds or so, is really important for feeling like you're in a normal conversation.
Of course, this type of feature also raises important privacy questions, especially when it comes to the way voices are cloned. It'll be important to see how Microsoft manages data privacy and user consent for these new voice cloning features, particularly in settings like business meetings. They claim the system learns over time by gathering feedback and monitoring user interactions, potentially allowing the translation and caption quality to improve gradually. I wonder about the long-term cost of running this for businesses. It’s probably not cheap to maintain the servers, APIs, and AI models required for accurate real-time translation at scale.
One interesting question is how well the system handles subtle cultural nuances. Language isn’t just about words; it’s about how we use them in different situations. It will be interesting to see how successfully they capture cultural and idiomatic expressions. Finally, I'm curious how well this will scale as more organizations adopt it. Ensuring good performance across different time zones and languages will be a challenge. If this system can handle the demands of global businesses and continue to improve, it could make team communications significantly easier. It will be interesting to see how effective this is in practice.
Other tech companies like Samsung and Google have been exploring similar technologies, which indicates this is a rapidly growing area. Overall, the development is exciting because it shows how AI can break down language barriers. It certainly helps improve accessibility and inclusion in online meetings, but the field still has many open challenges. This whole area of real-time translation and voice cloning has tremendous potential, but I think it's wise to remain cautiously optimistic about its eventual adoption.
More Posts from transcribethis.io: