Experience error-free AI audio transcription that's faster and cheaper than human transcription and includes speaker recognition by default! (Get started for free)
Advancements in AI Text-to-Speech Analyzing the Accuracy of Pronunciation and Intonation in 2024
Advancements in AI Text-to-Speech Analyzing the Accuracy of Pronunciation and Intonation in 2024 - Zero-shot TTS Breakthroughs in Voice Generation
Zero-shot TTS represents a notable leap in AI-driven voice generation, enabling the creation of speech without needing a large dataset of training audio for each voice. This innovative approach utilizes both input text and a designated "prompt" voice, which can be from a different language or style than the input text. While this flexibility is valuable, it also presents difficulties in maintaining the quality of the generated speech and ensuring the generated voice retains the unique characteristics of the original prompt.
Researchers have made headway by utilizing techniques like optimized feature fusion and employing innovative model architectures, such as the diffusion transformer in DiTToTTS. These advancements have paved the way for high-fidelity, multilingual speech synthesis, even without language-specific audio training. However, current zero-shot systems encounter limitations when processing continuous streams of text. This is particularly problematic in applications requiring rapid responses to short text inputs, highlighting an area ripe for continued research.
The trajectory of zero-shot TTS research appears focused on enhancing the flexibility and emotional range of synthesized speech. Future advancements could lead to systems that can better control the style and emotional nuances of generated audio, pushing the boundaries of what's achievable in artificial voice technology.
Zero-shot Text-to-Speech (TTS) represents a paradigm shift in AI voice generation, enabling models to produce speech without needing speaker-specific training data. This means significantly fewer datasets are needed to create a functional model, making the process more efficient. It has been observed that these models can generate speech that remarkably resembles a target voice using just textual instructions, instead of requiring extensive audio samples. This could prove revolutionary for voice cloning and assistive technologies tailored to specific individuals. Intriguingly, even without explicit emotional training, certain zero-shot TTS models show a surprising ability to capture emotional nuances from the input text itself, making them adaptable across a broader range of contexts.
However, there are ongoing limitations. For instance, maintaining consistent intonation and speech quality across extended pieces of text remains a hurdle. When processing beyond the limitations of the training data, zero-shot TTS systems sometimes struggle with maintaining fluency throughout longer dialogues or narratives. The development of sophisticated neural network architectures, such as those employing attention mechanisms, has led to notable advancements in voice quality. These mechanisms help the models emphasize relevant parts of the input text, resulting in more natural-sounding speech. Furthermore, it appears possible to influence the characteristics of generated speech by manipulating the input prompts, allowing users to control whether the voice sounds formal, conversational, or in other specific styles. This aspect of style control is quite promising.
The efficiency of this technology is noteworthy. Some systems can deliver high-quality audio in near-real-time, which is crucial for applications involving live events or interactive experiences. Researchers have been surprised to find that some models can learn from publicly available voice data, leading to an unforeseen capacity to generate less common accents and dialects. However, these models aren't perfect. Users have reported irregularities in pronunciation and stress placement, suggesting that zero-shot TTS should be carefully evaluated before deployment in scenarios demanding precision in linguistic nuances, especially in languages with intricate pronunciation rules.
The emergence of zero-shot TTS brings about ethical considerations, most prominently the risk of voice impersonation and potential misuse in technologies like deepfakes. These risks necessitate a careful examination of regulatory frameworks to mitigate the impact on communication and trust. As with many powerful AI technologies, finding the right balance between innovation and responsible usage is paramount.
Advancements in AI Text-to-Speech Analyzing the Accuracy of Pronunciation and Intonation in 2024 - Cross-lingual TTS Expanding Language Capabilities
Cross-lingual TTS is expanding the reach of AI-generated speech by enabling the creation of natural-sounding audio across multiple languages. These systems aim to seamlessly transition between languages within a single piece of input text, offering a more immersive and engaging multilingual experience. A major challenge addressed by these systems is maintaining consistent speaker characteristics like tone and eliminating any trace of a speaker's native accent while switching languages. Techniques like Dual Speaker Embedding for Cross-Lingual TTS are being explored to tackle this problem.
Using the International Phonetic Alphabet (IPA) and vector-quantized acoustic features has also shown promise in refining pronunciation and intonation in synthesized speech. However, a major hurdle remains in the need for vast amounts of paired text and audio data to train these models effectively. This data requirement presents a significant challenge, especially for languages with limited available resources. Researchers are exploring ways to mitigate this, such as through data augmentation techniques.
The advancements seen in cross-lingual TTS systems hold great potential for expanding access to information and communication across linguistic barriers. Yet, achieving truly seamless and natural-sounding speech across diverse languages continues to require further research and development, particularly in areas like improving pronunciation accuracy and adapting to varied language structures and intonations.
Cross-lingual TTS systems are showing promise in creating a more seamless experience by blending multiple languages within a single output. These systems utilize multilingual datasets, which while beneficial, present a challenge in ensuring pronunciation remains consistent across different languages. It's fascinating that a single voice model can potentially generate speech in multiple languages, potentially making it simpler to create applications that reach a wider audience. This approach simplifies development since it reduces the need for creating individual voice recordings for each language.
Some recent developments show that these systems are becoming increasingly sensitive to phonetic variations, offering the possibility of accurately representing lesser-known or endangered languages, which is critical for their preservation and ensuring more diverse voices within technology. However, it's interesting to note that the way emotion is conveyed through TTS can vary significantly across languages. It seems cultural context can influence how emotional nuances are integrated into synthesized speech, presenting a challenge for developers who aim for consistency in emotional delivery across various languages.
New research suggests that cross-lingual TTS models can unintentionally introduce accentual features from their primary language into a secondary language. This leads to unexpected results when generating speech in languages that require distinct intonation and stress patterns. There's a lot of ongoing work on combining neural networks with phoneme-level translation algorithms to enhance cross-lingual pronunciation accuracy. However, discrepancies still arise due to the limitations of available training data, which can impact the quality of the synthesized output.
As these models become increasingly sophisticated, they are getting better at recognizing and reproducing speaker-specific characteristics from different linguistic backgrounds. However, this occasionally comes at the expense of delivering consistent quality across all target languages. Researchers are actively working on improving the alignment mechanisms in TTS systems. This aims to ensure a better synchronization of speech with silent pauses or other non-speech elements in multilingual contexts, adding a level of complexity to the synthesis process.
Despite significant progress, a lack of dialectal variation in training datasets results in a corresponding lack of representation in the model outputs. This suggests that further data collection and inclusion efforts are crucial if we truly want to achieve cross-lingual versatility and richness in TTS systems. It's also quite intriguing that some TTS models seem to have a learning curve – they become more accurate in pronunciation over time as they are exposed to more input data. This hints at a future where systems can adaptively refine their output based on real-world feedback, improving their performance over time.
Advancements in AI Text-to-Speech Analyzing the Accuracy of Pronunciation and Intonation in 2024 - Speech Style Transfer Customizing Vocal Outputs
Speech style transfer is a growing area within AI text-to-speech, allowing for a greater level of control over the characteristics of synthesized voices. We are seeing techniques like ControlVAE and Diffusion Bridge emerge, offering more precise control over the style of the generated speech. This allows for more creative control in adjusting the characteristics of generated voices, potentially allowing for converting one speaker's voice into another, or even customizing aspects like tone or emotional delivery.
AI models like Variational AutoEncoders (VAE) and Generative Adversarial Networks (GAN) have contributed to the capability of generating a wide range of voice styles and increasing personalization in AI voices. The goal is to achieve more nuanced and human-like speech delivery. While promising, it's important to note that difficulties persist in maintaining a consistent and high quality of speech output, particularly when dealing with extended passages of text. Ongoing research focuses on refining these aspects, hopefully resulting in a future where AI-generated speech is more natural and adaptable to different contexts.
In the realm of AI-driven text-to-speech (TTS), the ability to customize vocal outputs through speech style transfer has become increasingly sophisticated. It's no longer just about replicating a specific voice; researchers are pushing boundaries to create dynamic, adaptable vocal expressions.
One intriguing facet is the capability to blend different speaking styles within a single output. We're seeing models capable of transitioning between formal and informal speech depending on the context of the input text, resulting in more natural-sounding conversations. Further, some models show a surprising ability to detect emotional nuances from text alone, injecting a degree of feeling into the generated voice even without explicit training. This suggests that AI is beginning to grasp the subtle ways language carries emotion.
Furthermore, models are becoming more interactive, capable of adjusting vocal outputs in real-time based on user feedback. This opens up avenues for personalized speech experiences where the system learns and adapts to individual preferences through ongoing interactions. The combination of text and vocal prompts also offers exciting possibilities. We can now generate hybrid voices that incorporate aspects of several different source voices, showcasing the flexibility of this technology.
Interestingly, these models exhibit a type of adaptive learning. They refine their style and intonation over time, revealing a latent potential for improvement through user interactions and feedback. This ongoing learning aspect is captivating. And there's even a push towards multimodal input integration. Researchers are experimenting with combining text and visual cues, such as facial expressions, to fine-tune vocal outputs, further enhancing the authenticity of the speech.
However, alongside these impressive advances, several hurdles remain. One is ensuring consistent pronunciation across diverse speaking styles, particularly when dealing with languages that have vastly different phonetic systems. The inherent capacity of TTS to closely mimic a person's voice also brings up important ethical considerations. The potential for voice impersonation necessitates careful consideration of the ethical implications and the need for regulatory frameworks to prevent misuse.
While TTS models are becoming more adept at understanding the pragmatic nuances of language—how context impacts meaning— there's still much to learn. It's a reminder that achieving truly natural-sounding and contextually appropriate speech style transfer is a complex undertaking. The journey toward perfect speech style transfer is a continuous process of refining and addressing ongoing challenges.
Advancements in AI Text-to-Speech Analyzing the Accuracy of Pronunciation and Intonation in 2024 - Parallel Generation Reducing Response Latency
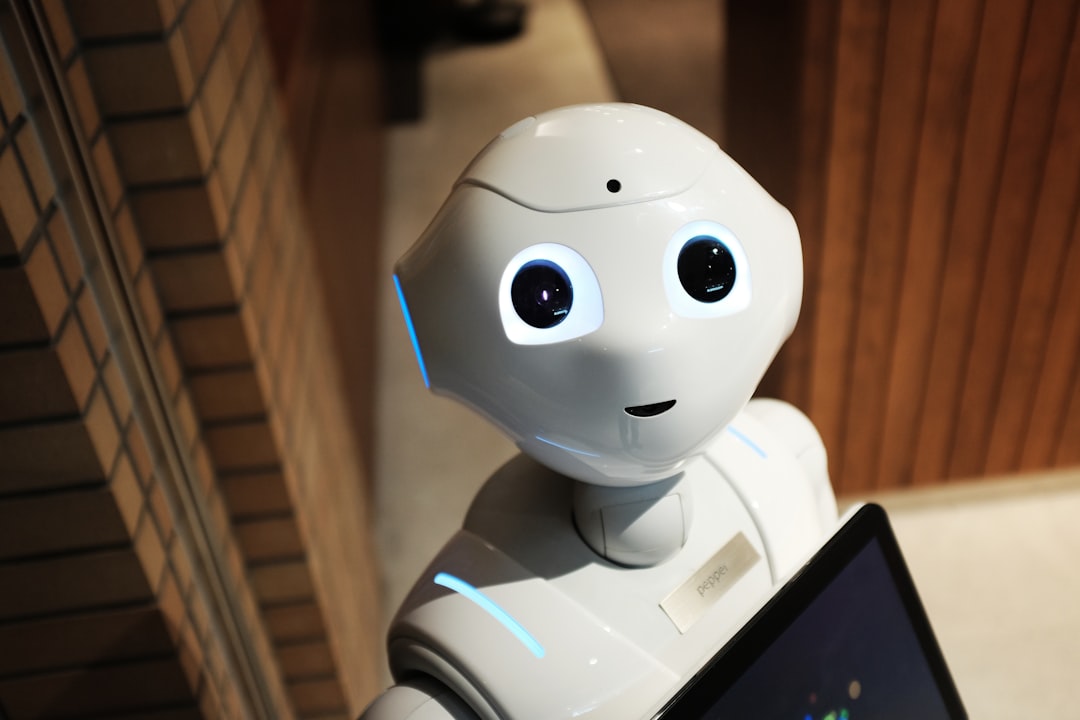
In 2024, a key focus in AI text-to-speech is reducing the time it takes to generate a response, also known as latency. Historically, these systems have faced challenges with speed because they generate a written response first, then convert it to speech. This sequential process leads to delays, especially when used in interactive settings like conversation. However, current research shows that using powerful language models to generate both text and speech at the same time—parallel generation—can effectively reduce these delays. The approach has proven successful in reducing wait times without sacrificing the quality of the generated speech. Techniques like "Assisted Generation" and new models built for generating speech, like diffusion models, are further improving response times. These innovations are significant steps towards creating systems that produce high-quality speech in real-time, which could lead to much smoother and engaging interactions between humans and AI.
AI text-to-speech systems are facing a critical challenge: minimizing the time it takes to generate a spoken response. This latency issue is particularly pronounced in applications that require quick interactions, like conversational AI or real-time translation. Two main hurdles contribute to this: the need to create a written response before a spoken one, and the fact that speech sequences typically take longer to process than text.
Researchers have started exploring parallel generation methods, which involve generating text and speech simultaneously using large language models. The idea is to expand the input and output sequences, allowing both text and speech to be created in parallel. Early tests in question-answering tasks suggest this parallel generation approach not only shrinks the delay, but also keeps the quality of the spoken response intact.
This has led to the development of "assisted generation" techniques which attempt to streamline the sequence of model runs to minimize text generation latency. We're seeing techniques like progressive distillation and classifier-free guidance successfully reduce latency, particularly in diffusion-based speech synthesis models. These advancements are especially useful in AI models that rely heavily on generating speech, like those using Transformer architectures or diffusion decoders.
Interestingly, the integration of speech editing tools within models like the Masked Parallel Transformer is allowing for both improved speech quality and faster response times. The focus now seems to be on streamlining the forward pass of these models – the part of the model that takes an input and produces an output – to achieve faster response times. This is critical for real-time applications where responsiveness is essential.
Overall, there's an exciting push within speech synthesis research to tackle both the speed of generation and the quality of the audio. It looks like 2024 will be a year of significant advancements in this field as researchers are tackling the bottlenecks in AI text-to-speech technology. This continuous improvement in response time and audio quality is essential for the continued success and adoption of AI text-to-speech in various applications. However, we still need to watch out for the potential downsides of pursuing speed above all else. We should carefully consider the potential impact of these new techniques on the quality of the synthesized speech and the potential for unintended consequences. The goal, as always, should be a balanced approach that produces both quality and speed.
Advancements in AI Text-to-Speech Analyzing the Accuracy of Pronunciation and Intonation in 2024 - Noise-resistant Speech Synthesis Improving Real-world Applications
The increasing importance of real-world applications is driving the development of noise-resistant speech synthesis. AI-powered text-to-speech systems often struggle when trained on data recorded in noisy environments, leading to distortions and unwanted noise in the synthesized speech. Researchers are working on ways to improve this, such as using innovative preprocessing steps that clean up the input speech before it's processed by the AI. Techniques like adaptive filtering aim to reduce the impact of surrounding noise, making the output clearer. We're also seeing the development of multimodal speech recognition systems designed specifically for noisy conditions, which is a significant step toward improving how humans interact with AI-powered speech in everyday settings. The ongoing research emphasizes improving the reliability of these systems so that they can consistently produce high-quality speech, even in challenging noisy environments, making them more practical for a wider range of applications. While advancements have been made, challenges remain in achieving consistently high-quality output in a variety of noisy real-world conditions, highlighting a crucial area for future development.
The field of speech synthesis has seen a significant shift towards producing human-like speech, transitioning from older methods to advanced deep learning models. While deep learning has undoubtedly improved the quality of text-to-speech (TTS) systems, a persistent hurdle arises when these systems are trained on audio captured in noisy environments. Often, TTS models that rely on enhanced speech struggle to eliminate distortions and lingering noises, compromising the overall quality of the synthesized output. This issue highlights a key research area—building noise-robust TTS systems that can maintain clarity in real-world scenarios.
It's interesting to see how the push for enhanced human-computer interaction has driven the evolution of TTS. However, noise robustness is equally vital for automatic speech recognition (ASR) systems to function reliably across varied environments. Researchers are diligently working on preprocessing modules to improve speech signals before they're fed into ASR systems, which could potentially minimize noise's negative impact. This involves employing a diverse range of speech enhancement techniques such as comb filtering, adaptive filtering, and methods leveraging hidden Markov models or Bayesian estimation. The goal is to create cleaner audio input that allows ASR to perform more accurately.
One example of recent work in this area is FLYTTS, a TTS system created to deliver rapid, efficient, and high-quality speech generation. It's quite remarkable that this new system can rival the output of established, more complex models in terms of the quality of the generated speech. While the focus on the accuracy of pronunciation and intonation continues to be a significant part of TTS research in 2024, these advancements in noise-robustness are helping to bridge the gap between AI-generated speech and natural human speech.
We're also starting to see the deployment of noise-resistant multimodal speech recognition systems. One notable example is Wavoice, which signifies a critical step toward enhancing user experiences in challenging auditory settings. This focus on improving noise-resistance could lead to more accessible AI-driven applications for a wider range of users. The ongoing investigation into the impact of noise on synthesized speech and the development of models that mitigate it underscore the importance of bridging the gap between idealized training environments and the complex, and often noisy, real-world conditions these systems will operate in. It’s a promising area of research, as it has the potential to improve the usability and accessibility of AI-powered speech technology for everyone.
Advancements in AI Text-to-Speech Analyzing the Accuracy of Pronunciation and Intonation in 2024 - Remaining Challenges in Natural Speech Production
Despite the remarkable progress made in AI-driven text-to-speech, achieving completely natural-sounding speech remains a challenge. One area where improvement is needed is in accurately replicating the subtle aspects of speech, like intonation and rhythm, which are vital for making synthesized speech sound genuinely human. The diversity of human speech – different ways to pronounce words, speech patterns, and even emotional delivery – poses a significant hurdle. Modeling these nuances can be difficult, and current TTS systems often struggle to capture the subtle expressions present in natural human communication.
Furthermore, newer techniques like zero-shot TTS and the ability to generate speech in multiple languages, while promising, reveal shortcomings in maintaining quality across longer pieces of text or when handling different language structures. These limitations highlight the need for ongoing development in how models handle consistency and manage the complexity of diverse languages. Moving forward, it is essential for the field to address these challenges if AI-generated speech is to achieve a level of naturalness that truly resonates with human listeners, fostering a more genuine and engaging experience.
Remaining Challenges in Natural Speech Production
1. **Capturing Emotional Intonation**: While AI-powered speech synthesis has made incredible strides, accurately mimicking the subtle ways humans use intonation to express emotion remains a challenge. The nuances of pitch, stress, and rhythm that convey feelings often get lost in translation, resulting in synthesized speech that can sound somewhat robotic or flat.
2. **Smooth Style Transitions**: Seamlessly switching between different speech styles—from a casual conversation to a formal announcement, for instance—still poses a hurdle. These shifts require dynamic adjustments in tone and pacing that current models sometimes struggle to manage effectively, leading to noticeable shifts in the perceived character of the voice.
3. **Interpreting Ambiguous Language**: Human speech is often peppered with ambiguous expressions whose meaning relies on context. AI models haven't fully grasped these nuances, leading to synthesized responses that may lack the appropriate emotional weight or fail to fit within the larger conversational context.
4. **Maintaining Consistency Over Time**: Keeping speech quality and coherence throughout long interactions is still an ongoing problem. AI-generated voices sometimes exhibit a kind of "vocal fatigue" or inconsistency after extended use, failing to match the natural stamina and consistency of human speakers over time.
5. **Incorporating Non-Verbal Cues**: Effective communication involves more than just words—gestures, facial expressions, and other non-verbal cues play a significant role. AI has yet to fully integrate these elements into speech production, limiting the realism of synthesized output, particularly in interactive scenarios.
6. **Understanding Contextual Language**: Grasping the broader context of conversations is crucial for natural speech. While progress has been made, many systems still fail to adapt their tone or phrasing based on shifts in the conversation, leading to a somewhat disjointed feel.
7. **Accurately Representing Accents**: Replicating regional accents accurately can be difficult for AI models. While some models can mimic specific voices, they might miss the subtle nuances and variations inherent in different dialects, potentially impacting the authenticity of the generated speech.
8. **Modeling Diverse Inflection**: Languages and cultures have unique patterns of inflection and phrasing. Many AI systems haven't mastered these diverse linguistic structures, leading to inaccuracies or awkwardness when switching between languages.
9. **Adaptive Learning from Feedback**: Unlike humans who learn and refine their speech through social interaction, AI models currently lack robust mechanisms for learning from real-time feedback during conversations. This limits their ability to adapt based on user preferences or changing contexts.
10. **Navigating Ethical Considerations**: The ability of AI to closely replicate human voices raises complex ethical concerns, particularly regarding consent and identity. Ensuring responsible use of synthetic speech while navigating the implications of voice cloning adds a layer of complexity to the field, requiring urgent policy considerations.
Experience error-free AI audio transcription that's faster and cheaper than human transcription and includes speaker recognition by default! (Get started for free)
More Posts from transcribethis.io: