Experience error-free AI audio transcription that's faster and cheaper than human transcription and includes speaker recognition by default! (Get started for free)
7 Key Differences Between US and UK English in AI-Powered Translation Tools
7 Key Differences Between US and UK English in AI-Powered Translation Tools - Spelling Variations in AI Translations
AI translation tools face difficulties when confronted with the diverse spelling variations between UK and US English. Tools often struggle to accurately differentiate between spellings like "colour" and "color," leading to inconsistencies in the translated output. Furthermore, understanding the nuances of how words are borrowed and used in different phrases poses a significant obstacle for AI. British English's tendency towards maintaining traditional spellings, in contrast to American English's preference for simplification, further complicates this challenge for the AI system. These differences in spelling and their impact on meaning are significant and require careful attention to produce translations that are both accurate and reflect the intended context. Successfully adapting to these spelling distinctions is a key component to achieving higher quality translations.
AI translation tools often encounter difficulties when dealing with the diverse spelling conventions present in British and American English. This stems from their reliance on large, pre-existing datasets which might not equally represent all regional variations, leading to a lack of consistency in the output. For example, British English frequently utilizes more 'u's in words like "colour" and "favour," compared to the simplified "color" and "favor" favored in American English. Unless explicitly trained otherwise, AI models might default to the more common American spelling, sometimes overlooking the regional nuances.
Some spelling discrepancies arise from historical linguistic developments, such as "defense" in American English versus "defence" in British English. AI systems may fail to grasp these context-dependent distinctions without additional cues or training. Interestingly, the user's location can sometimes influence the outcome. A user in the UK might receive a translation with British spellings for the same source text as a user in the US, showcasing that the location aspect is considered by certain tools.
Hyphenation styles also contribute to translation challenges. Words like "realize" (US) and "realise" (UK), or the inconsistent treatment of compound words, complicate AI's translation efforts. Some platforms attempt to rectify these issues by integrating user feedback or collaborative error reporting systems. These features concentrate on improving the model's accuracy and responsiveness to regional spelling inconsistencies over time. However, this approach might not always be effective, especially with subtle nuances or regional idioms.
The ability of AI to consistently capture and reproduce regional idioms, where spelling variations exist (such as "analogue" and "analog"), remains a hurdle. Failing to capture these variations can significantly distort the original meaning. It's also worth noting that AI spell-checkers may not be fully up-to-date with the latest linguistic changes. For instance, the ongoing debates on gender-neutral language have not yet comprehensively impacted the way AI handles spelling.
The common reliance on a uniform, general-purpose dictionary in automated translation tools often restricts the AI's ability to adapt to industry-specific jargon or terminology that has its own distinct spelling variations. Lastly, English spellings influenced by other languages or cultures, such as "plaza" (common in both US and UK), can pose a challenge for AI, particularly if its training data lacks sufficient diversity. This underscores the need for AI systems to be exposed to a wider array of linguistic patterns and regional variations to enhance their ability to capture the intricate nuances of global English.
7 Key Differences Between US and UK English in AI-Powered Translation Tools - Vocabulary Differences Challenging Machine Learning
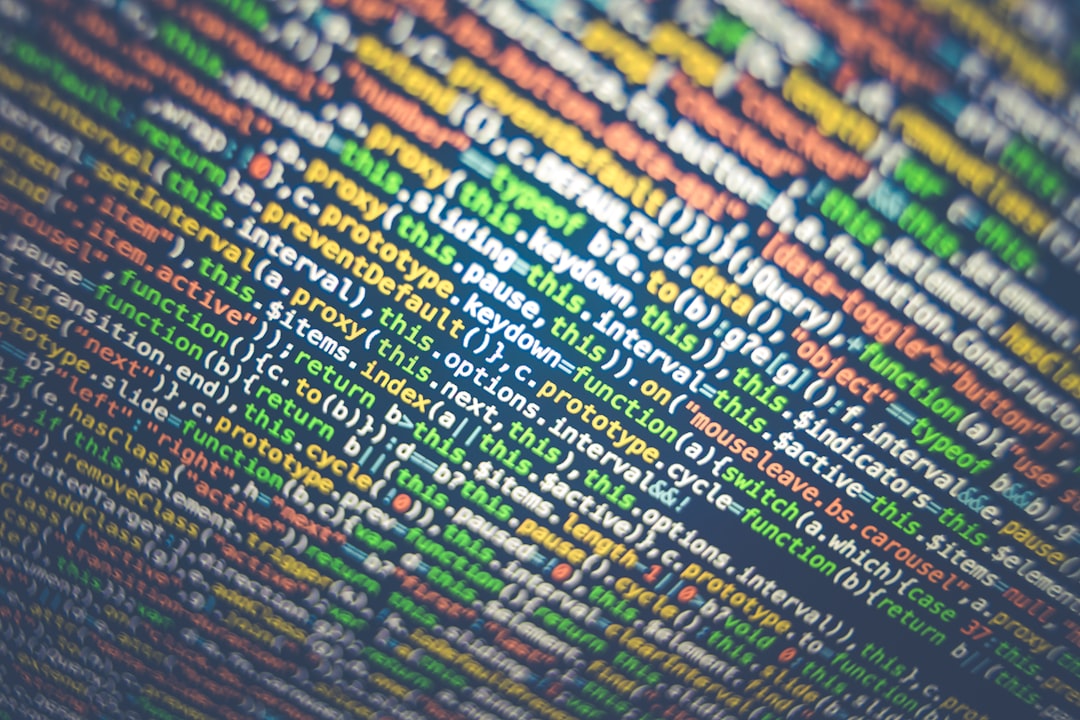
Machine learning models face difficulties when handling the diverse vocabulary found in US and UK English. This challenge arises because the same objects or concepts can have different names in each dialect. For instance, the front of a car is called a "bonnet" in the UK but a "hood" in the US, while the rear storage compartment is referred to as a "boot" in the UK and a "trunk" in the US. These variations extend beyond just objects, affecting how everyday phrases and even sentence structures are formed. For example, while the UK might say "at the weekend," the US typically says "on the weekend." These differences pose a barrier for AI translation tools, which need to accurately understand the context and regional preferences to bridge the gap between US and UK English speakers and ensure accurate and meaningful translations. Without this capability, AI may struggle to create translations that are truly effective for cross-dialect communication.
AI translation tools face a significant hurdle when it comes to handling the diverse vocabulary that exists between US and UK English, even within specific domains. For instance, a term like "lorry" in British English translates to "truck" in American English, presenting a challenge for ensuring accurate and contextually relevant translations, especially in professional settings.
It's estimated that over 80% of everyday English words have variations between US and UK English. This necessitates the ability of AI systems to dynamically adjust their vocabulary based on the intended audience, but many current AI models still rely on fixed training datasets that don't consistently capture these evolving differences.
Certain British English expressions, like "bob's your uncle" or "cheeky," have no direct equivalents in American English, making it difficult for AI to capture the intended meaning while also maintaining a sense of regional authenticity.
The frequency of specific word usage can also vary greatly between the dialects. For example, while "boot" in British English refers to a car's storage area, it's typically understood as footwear in American English, leading to potential confusion for AI translation systems.
Words with multiple meanings ("polysemous") also create further complications. Take "biscuit," for instance: in British English, it means what Americans call a "cookie," while in American English, "biscuit" refers to a savory bread-like food typically served with breakfast.
AI often misinterprets phrasal verbs that are more common in one dialect than another. For example, "take out" might suggest "to remove" in American English, while "take away" is the more common phrase for that action in British English. This can lead to unintended alterations in the meaning of the translated text.
Local dialects and regional slang add another layer of complexity. Terms like "gutted," which informally means "very disappointed" in British English, aren't readily understood by AI when translating for an American audience, highlighting the need for a more nuanced understanding of context.
The influence of technology on language is also noteworthy. Words like "smartphone" are widely understood, but terms like "app" (short for application) can have slightly different connotations depending on cultural context.
A large portion of UK English vocabulary maintains words with French origins—like "mum" versus the American "mom"—resulting in potential subtle differences in familial communication that AI could misinterpret without the proper context.
Finally, AI tools often struggle with the differing levels of formality present in US and UK English. For example, the casual "I'll ring you" in British English is equivalent to "I'll call you" in American English. Failing to understand this distinction can significantly impact the overall tone and style of communication.
7 Key Differences Between US and UK English in AI-Powered Translation Tools - Grammar Nuances Affecting Natural Language Processing
Grammar, with its subtle variations and structures, significantly impacts the success of Natural Language Processing (NLP), especially when navigating the complexities of US and UK English. AI systems must be adept at discerning these nuanced grammatical differences, including variations in tense usage and sentence structures, as these subtle shifts can dramatically alter the meaning conveyed. Failing to recognize these grammatical intricacies can lead to translations that miss the intended context, potentially hindering clarity and fluency in communication. This is especially true when considering the nuances of explicit versus implicit meaning, a challenge for both human language learners and the AI attempting to process it. NLP algorithms need to become more sophisticated to adequately interpret these subtle variations. Moving forward, AI-powered translation tools must incorporate a deeper understanding of these grammatical nuances to achieve more accurate and contextually relevant translations across different English dialects.
Computers interacting with human language, a field known as Natural Language Processing (NLP), relies on algorithms to understand, interpret, and generate human communication. However, the subtle variations within language, specifically grammar, pose a notable challenge. These nuances are critical for conveying precise meaning and enriching interactions, making them harder for computers to grasp. This is especially evident when dealing with language learners who might struggle to distinguish between explicit and implicit meaning.
One way to illustrate the complexity is by comparing synonyms, idioms, and homophones. These seemingly similar terms carry distinct connotations and interpretations, emphasizing the importance of fine-grained understanding in communication. NLP uses techniques like syntactic analysis, breaking down sentences into smaller units (phrases or dependencies) to try to parse and comprehend human language. Additionally, semantics, which explores meaning, is at the heart of NLP research, trying to disentangle the intricate layers of meaning hidden within language. Dependency grammar provides a model for understanding how the words in a sentence relate to each other, influencing the overall interpretation.
Now, consider the differences between US and UK English. They diverge in various ways, including spelling (like "color" vs. "colour"), vocabulary (such as "truck" vs. "lorry"), and grammar itself. These nuances can create hurdles for AI-powered translation tools. For instance, UK English often uses single quotation marks for direct speech ("'Hello'") while US English uses double marks ("Hello"). This stylistic difference alone can impact how a text is perceived.
Similarly, certain grammatical structures are utilized differently. For example, the use of the present perfect tense ("I have just eaten" in UK vs. "I just ate" in US). Another example is the treatment of collective nouns, where UK English sometimes considers them plural ("The team are winning") while US English usually treats them as singular ("The team is winning"). The choice of prepositions also varies, like "different from" vs. "different than".
Furthermore, AI struggles with context. The word "football" has entirely different meanings in both dialects, highlighting the need for context-sensitive translation. The frequency and use of passive vs. active voice, date formats (day/month/year vs. month/day/year), contractions ("haven't" vs. "hasn't"), and even diminutive forms ("telly" vs. "television") add to the complexity. AI has to be trained to capture these nuances. Cultural references further complicate the issue. Idioms rooted in British customs can be totally lost on an American audience, making it crucial for AI systems to grasp the cultural context that impacts grammar usage.
Overall, achieving high-quality translations requires AI to bridge these grammatical gaps effectively, which is an ongoing challenge in the world of NLP. It emphasizes that simply relying on large datasets isn't enough, especially if they aren't representative of the full spectrum of regional and cultural language features. It's a testament to the intricacy of language and the need for AI to evolve with a deeper understanding of these linguistic subtleties.
7 Key Differences Between US and UK English in AI-Powered Translation Tools - Punctuation Rules Impacting AI Interpretation
AI's ability to accurately interpret text is heavily influenced by punctuation rules, especially when dealing with the distinct styles of US and UK English. American English generally places punctuation marks inside quotation marks, regardless of whether they relate to the quoted part. British English, on the other hand, positions punctuation outside the quotes if it isn't part of the quoted material. This disparity can be a source of errors and confusion for AI translation systems, particularly when handling dialogue or text excerpts. Furthermore, the placement of commas and periods differs significantly between the dialects, further adding to the challenges of AI interpretation and leading to potential inconsistencies in translated outputs. To ensure accurate and coherent translations across dialects, AI-powered translation tools need to account for and effectively manage these differences in punctuation usage. Overcoming these challenges is key to producing translations that are clear, accurate, and contextually appropriate.
When AI systems process text, variations in punctuation between US and UK English can create hurdles. For example, UK English sometimes places punctuation outside closing quotation marks, especially if the punctuation isn't part of the quoted content itself, whereas US English usually keeps punctuation inside. This difference in placement can subtly alter meaning or emphasis, which AI might not always discern accurately.
The inclusion or exclusion of the Oxford comma (the comma before the final conjunction in a list) is another area of divergence. American English strongly favors it, while it's less common in British English. If an AI model isn't trained to understand this, it may misinterpret the relationships between items in a list, potentially causing errors in translation.
Furthermore, question mark placement within quotations creates a challenge. British English places the question mark outside closing quotes if it isn't part of the quote, whereas US English generally keeps it inside. This can create confusion for AI trying to interpret the structure of questions within translated text.
Interestingly, the use of ellipses (those three dots indicating a pause or omitted text) also varies. UK English often includes a space before and after each dot, while US English tends to treat it as a single character without spacing. AI models that haven't been trained on this subtle difference may struggle to interpret the intended pauses or omissions accurately.
Compound words and hyphenation are also problematic. The way compound words are treated can differ depending on region. For instance, "high-quality" in US English is often seen as "high quality" in British English. AI models must learn to adapt to these changes to avoid alterations in meaning within translations.
Bracket usage in formal writing tends to lean towards round brackets in UK English, contrasting with more varied usage in US English. AI systems may encounter difficulties correctly interpreting this style variation if not adequately trained.
Another issue is the way comma splices are perceived. While comma splices (where two independent clauses are joined solely by a comma) are sometimes acceptable in British English, especially in less formal settings, US English typically requires more robust punctuation. AI systems need to balance their understanding of this, lest they misapply rigid rules in a way that alters the intended stylistic effect.
Quotation marks themselves carry distinct conventions. The use of double quotation marks in American English versus single in British English, though a seemingly small distinction, can change how AI perceives dialogue or attribution. This needs to be considered for effective interpretation.
The punctuation of conjunctions like 'and' also varies. British English sometimes omits the comma before "and" in a series in less formal situations, while US English typically includes it. This subtle change can affect how AI analyzes sentence structure during the translation process.
Finally, punctuation choices can heavily influence the overall tone of a written piece. AI systems that don't account for the context provided by punctuation can potentially misinterpret formality levels, resulting in translated text that sounds too formal or too casual for its intended audience. This highlights the importance of contextual awareness in AI's understanding of the written language.
It's clear that the nuances of punctuation play a significant role in cross-dialect AI translation. AI models need to be trained extensively on a wide range of text examples to be able to reliably navigate these variations and accurately interpret and generate text that accurately reflects the intended style and meaning.
7 Key Differences Between US and UK English in AI-Powered Translation Tools - Pronunciation Variances in Speech Recognition
When examining how pronunciation differences affect speech recognition, we find notable variations between American and British English that pose challenges for AI translation tools. For example, the way vowel sounds are produced in words like "lot" and "bath" differs significantly, potentially causing confusion for AI models unless they are specifically trained on these distinctions. Similarly, the pronunciation of "th" sounds varies considerably, with British English typically producing a clearer articulation while American English often exhibits a softer pronunciation. These phonetic differences, when combined with the diversity of regional accents within both dialects, present significant obstacles for achieving accurate transcriptions. AI systems may struggle to correctly interpret and transcribe speech patterns due to these variations. To improve accuracy, it's essential for speech recognition systems to be trained on comprehensive and diverse datasets that represent both American and British pronunciation styles. This allows them to better recognize the nuances of each accent and develop a more robust understanding of the different ways English is spoken.
Here are ten interesting points about how pronunciation differences can affect speech recognition, especially when AI-powered translation tools try to understand the variations between US and UK English.
1. **Vowel Sounds**: The way vowels are pronounced can be quite different between American and British English. For example, the "a" in "dance" or the "o" in "hot" might sound distinct, which can cause problems for AI because it might not recognize the different regional pronunciations.
2. **Emphasis and Tone**: The stress put on different syllables in words can vary between the two dialects. Consider the word "advertisement," where British speakers might stress the third syllable, while Americans usually stress the second. AI systems trained primarily on one type of accent may find it challenging to handle these shifts in emphasis.
3. **Glottal Stops**: Some British accents, like Cockney, use a glottal stop instead of the "t" sound in words like "butter" (it sounds like "buh-er"). Speech recognition systems can struggle to understand this change, potentially misinterpreting or incorrectly transcribing the word.
4. **Connecting Sounds**: In casual speech, especially in American English, sounds at the end of words often blend into the next vowel. For instance, "I want to" can become "I wanna". AI systems that haven't encountered this kind of casual pronunciation may struggle to transcribe it correctly.
5. **Pronouncing the "R"**: Many British accents don't pronounce the "r" at the end of words ("car" might sound like "ca"). This contrasts with American English, where the "r" is typically pronounced. AI may struggle with this variation, leading to possible errors in translation.
6. **Pronunciation of Specific Words**: Some words have different pronunciations between the two dialects, like the word "schedule", which uses a "sh" sound in British English and a "sk" sound in American English. This can lead to the AI mistaking one for the other.
7. **Words with Same Spelling, Different Pronunciation**: There are cases where words are spelled identically but pronounced differently. For instance, "lead" (to guide vs. the metal). If AI focuses only on spelling, it might miss the intended pronunciation, and wrongly connect the meanings.
8. **Training Data Bias**: Many AI models are primarily trained on data from American English, creating a potential bias that makes them less good at recognizing British pronunciations. This can cause them to favor US accents and struggle with UK dialects.
9. **Informal Speech and Slang**: Casual phrases like "gonna" (going to) or "wanna" (want to) are often used in both dialects but can be tricky for AI to understand if it hasn't been specifically trained on these informal pronunciations. The translations could end up sounding odd or out of place.
10. **Understanding the Surrounding Words**: Sometimes, AI can get confused because the pronunciation of a word might depend on the words surrounding it. For example, "I'm here" and "I'm hear" sound very similar. AI needs to be capable of understanding the entire context in order to infer the right pronunciation.
Figuring out how to handle these pronunciation differences is important if we want to improve AI's ability to interpret and translate between US and UK English in real-world situations.
7 Key Differences Between US and UK English in AI-Powered Translation Tools - Collective Noun Treatment in Language Models
Language models, particularly those powering AI translation tools, face a challenge when dealing with collective nouns in US and UK English. British English allows for both singular and plural verb forms with collective nouns, depending on whether the emphasis is on the group as a single entity or its individual components. In contrast, American English generally uses singular verb forms for collective nouns, resulting in a simpler grammatical approach. However, this simplification can lead to issues if the intended emphasis is on the individuals within the collective, rather than the group as a whole. This divergence in treatment highlights the complexities of AI translation. For truly accurate and natural translations, it is imperative that AI systems understand the context and subtle nuances of collective noun usage across different dialects of English. Failure to adapt to these complexities may lead to inaccurate translations and a diminished understanding of the intended message. The ability of AI to successfully discern and adapt to these linguistic differences is essential for improving communication across diverse variations of the English language.
Here are 10 points about how collective nouns are handled differently in language models, which might pique the interest of those studying AI-powered language translation:
1. **Singular vs. Plural Treatment**: British English often treats collective nouns like "team" or "family" as plural (e.g., "The team are winning"), while American English typically uses the singular form (e.g., "The team is winning"). This difference can trip up AI if it's not explicitly trained to recognize it.
2. **Cultural Nuances in Usage**: The way collective nouns are treated isn't just a grammatical quirk; it reflects cultural views on groups and individuals. British English often emphasizes the group as a whole, which can confuse AI if it doesn't grasp the cultural context.
3. **Regional Variations in British English**: Even within British English, some regional dialects have their own quirks when it comes to collective nouns, adding another layer of difficulty for AI that relies on broad generalizations instead of localized data.
4. **Challenges in Cross-Dialect Communication**: If AI is mostly trained on one dialect, it might misunderstand collective nouns in cross-dialect communication. For example, a British user talking about a sports team could trigger an error in an AI model mainly designed for American English.
5. **Impact on Meaning**: How a collective noun is treated can strongly impact the meaning of a sentence. AI that doesn't understand this can produce translations that completely miss the intended message.
6. **Limitations of Training Data**: Many AI models are trained on data that doesn't fully represent the varied grammar of British English, including the flexible ways collective nouns are used. This can lead to biased results that favor American conventions.
7. **Constantly Changing Language**: Collective noun treatment isn't static; it changes as society evolves. Nouns that were traditionally plural might shift to singular and vice versa. AI models need regular updates to keep up with these changes.
8. **Formal vs. Informal Context**: The treatment of collective nouns often depends on whether it's formal writing or casual conversation. AI needs to understand the context to apply the correct grammatical form.
9. **Subtle Impact on Tone and Style**: Choosing between singular or plural can subtly change the tone of a sentence. AI that overlooks these nuances might generate translations with unintended changes in style or formality.
10. **Issues with Combined Data**: Some language models combine data from various sources, which might have inconsistent ways of handling collective nouns. This can lead to confusion and errors in AI-generated translations. Making sure the training data is consistent is important for ensuring accurate translation.
7 Key Differences Between US and UK English in AI-Powered Translation Tools - Date Format Distinctions in AI Data Processing
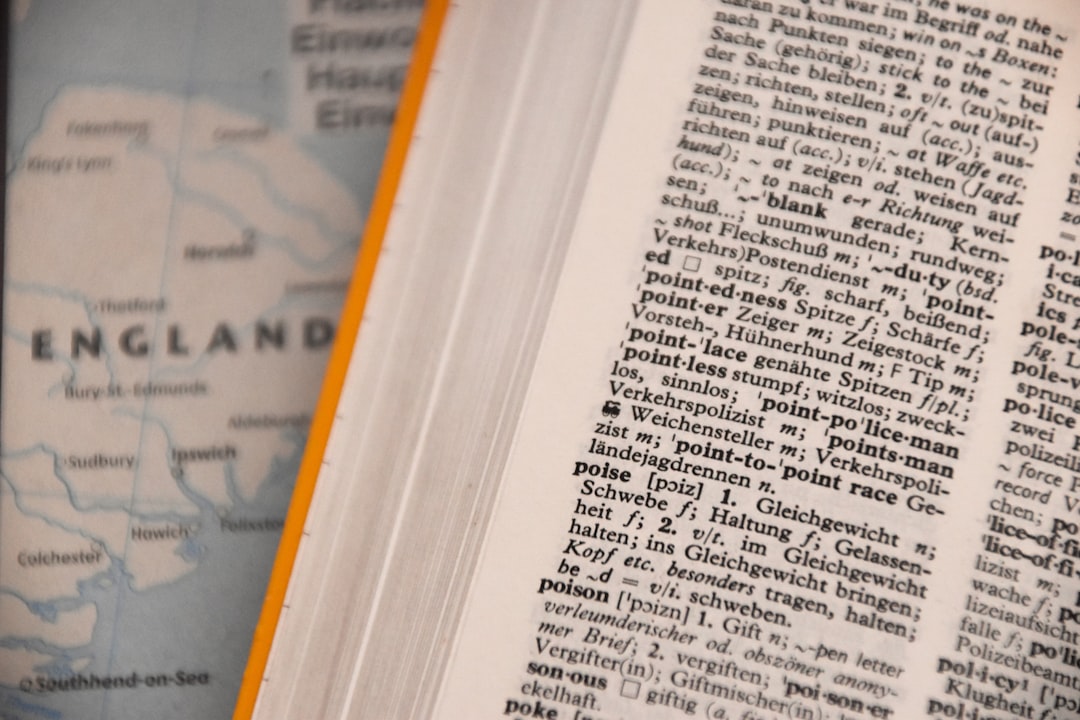
AI systems processing data often encounter challenges due to differing date formats across languages and regions, particularly when dealing with US and UK English. The US commonly uses the MM/DD/YYYY format (month/day/year), whereas the UK employs the DD/MM/YYYY format (day/month/year). This simple difference can have significant consequences for AI data analysis, potentially causing misinterpretations and skewed results if not properly addressed. For example, if an AI system trained primarily on US data encounters a UK date, it might misinterpret the order of the numbers, leading to incorrect analysis. This issue becomes increasingly important as datasets are often globally sourced and shared. Understanding these date format conventions is thus crucial for ensuring that AI systems can accurately interpret and process data from diverse sources, promoting the integrity of any insights drawn from it. If AI fails to adapt to this distinction, it can lead to inaccurate analysis and flawed conclusions in fields reliant on correct chronological order.
Here's a rewrite of the text about "Date Format Distinctions in AI Data Processing" in a similar style and length, focusing on the complexities and challenges:
When AI systems process data, particularly in a global context, variations in date formats can introduce significant complexities and potential challenges. For instance, the way dates are written in the US (MM/DD/YYYY) differs from the UK standard (DD/MM/YYYY). This seemingly simple distinction can lead to AI misinterpreting a date like 04/05/2024, producing unexpected results.
One of the major hurdles is that AI systems often rely on context to figure out the date format. In documents lacking clear clues, the AI might make incorrect assumptions, which can have serious implications in areas like finance or legal matters where precision is crucial. Imagine the confusion if a financial transaction was misdated due to a wrong interpretation of the format.
Furthermore, when users input dates, inconsistencies in their formatting can confuse the AI. If the AI is primarily trained on US data, it may automatically assume the MM/DD/YYYY format, overlooking valid UK dates. This can lead to errors and potential miscommunications in scenarios like online bookings or scheduling.
This becomes even more challenging when building applications meant for a global audience. These systems need to effortlessly adapt to various date formats, ensuring the AI can understand user input while producing output that is clear and sensible to the recipient, regardless of their location or standard date practices.
A contributing factor is that many AI models are largely trained on US-centric datasets. This can result in a lack of exposure to diverse date formats found elsewhere. This bias can lead to errors in translating or processing text from sources utilizing British English or other date formats.
Moreover, numeric date representations introduce ambiguity. For example, '02/03/2024' could refer to February 3rd or March 2nd depending on the context. This creates uncertainty that AI systems need to carefully consider and potentially resolve through context clues or additional input.
If AI incorrectly interprets a date, that error can cascade through related systems. Imagine an automated scheduling system that incorrectly understands a deadline because of a wrongly formatted date – this can create a series of subsequent issues across the application ecosystem.
To help resolve these challenges, the way AI applications are designed is critical. User interfaces need to incorporate methods to clearly establish date format preferences. Without such features, relying on the AI to infer it can be problematic and lead to user frustration.
These challenges are even more pertinent in heavily regulated sectors like healthcare or finance where using specific date formats is mandatory. AI systems that aren't trained to recognize and adhere to these requirements could put organizations at risk of violations.
Lastly, the standards for date formats are in a state of continuous evolution as more regions become integrated with digital communication. AI systems need consistent updates to keep pace with these changes, ensuring their accuracy and relevance in a rapidly changing digital landscape.
Experience error-free AI audio transcription that's faster and cheaper than human transcription and includes speaker recognition by default! (Get started for free)
More Posts from transcribethis.io: