Experience error-free AI audio transcription that's faster and cheaper than human transcription and includes speaker recognition by default! (Get started for free)
Whisper 20 OpenAI's Latest Advancements in Multilingual Speech Recognition
Whisper 20 OpenAI's Latest Advancements in Multilingual Speech Recognition - OpenAI's Whisper Trained on 680,000 Hours of Web Data
OpenAI's Whisper stands out in the field of automatic speech recognition due to its extensive training regimen. It was trained using a massive 680,000 hours of web-based audio data, encompassing numerous languages and a variety of speech tasks. This substantial training dataset contributes to Whisper's ability to handle a broader spectrum of challenges compared to more traditional systems. Accents, background noise, and technical terminology that often hinder speech recognition seem to be less of an obstacle for Whisper. Its multilingual capabilities, fostered by the diverse dataset, expand its utility across a wider range of linguistic applications.
Whisper's development incorporates what's called weakly supervised learning, where unlabeled data is utilized to improve the model's performance. This novel approach likely helps Whisper perform beyond what's typical for speech recognition systems. Furthermore, the open-source nature of Whisper makes it a readily available resource for developers and researchers to experiment with and enhance, fostering a collaborative environment for advancing multilingual speech recognition technology.
1. Whisper's design emphasizes handling a diverse range of accents and dialects, a critical aspect for achieving reliable multilingual speech recognition. It represents a step forward from older models, which often struggled with regional speech variations.
2. The training data for Whisper is truly massive, comprising a remarkable 680,000 hours of transcribed audio collected from the internet. This scale illustrates the data demands of sophisticated speech recognition systems and helps the model adapt to diverse speech patterns.
3. Whisper's architecture is built on transformer models, which have become a standard for sequence-to-sequence tasks. This shift is interesting, as earlier systems often leaned on convolutional neural networks, which sometimes struggle with processing temporal data effectively.
4. Evaluations of Whisper's performance across numerous languages show it performing competitively or even surpassing specialized models. This indicates that high performance in one language doesn't necessarily come at the cost of accuracy in others.
5. An intriguing element of Whisper's training involves techniques related to weakly supervised learning. It utilizes unlabeled data to refine its abilities, which is valuable for expanding language model capabilities while lessening reliance on costly and time-consuming human annotations.
6. When gathering the data, OpenAI seems to have focused on creating diversity in topics and speakers, which can be helpful in mitigating some of the biases often found in AI systems. This proactive approach towards data quality offers a subtle yet important advancement in creating responsible AI models.
7. Preliminary evidence suggests that Whisper might excel at retaining context across longer audio snippets, overcoming a hurdle faced by other models that can lose track of what's being said over time. This feature would likely be helpful for accurately transcribing longer dialogues.
8. The model exhibits some robustness against various audio qualities, functioning effectively even in the presence of background noise or distorted sound. This adaptation to realistic audio scenarios makes it more versatile in applications where recordings might not be perfectly clear.
9. Whisper's development also involved enhancements to phoneme recognition, making it better at differentiating between words that sound similar. This is a recurring problem for speech recognition systems, and it's important for applications that need very high accuracy, such as medical or legal transcription.
10. Throughout Whisper's development, rigorous error analysis has been a key aspect. It offers crucial insights into the mistakes the model tends to make, allowing for a refinement process that leverages feedback and ultimately improves its learning capacity.
Whisper 20 OpenAI's Latest Advancements in Multilingual Speech Recognition - Robust Performance Across Accents and Background Noise
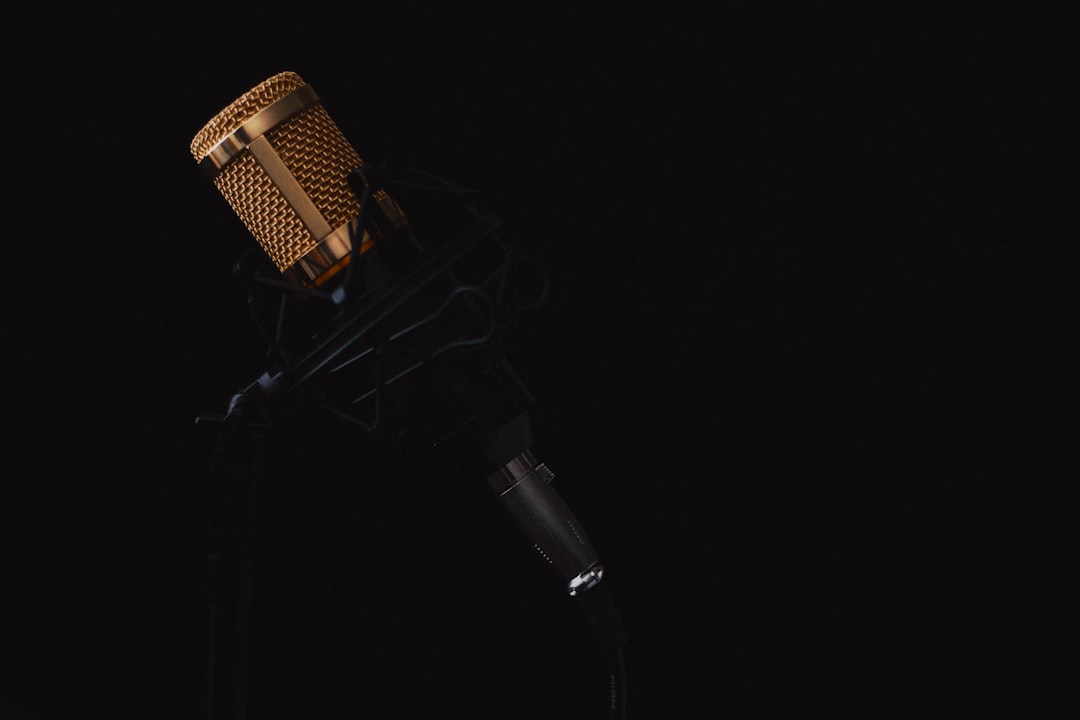
Whisper's strength lies in its ability to handle diverse accents and background noise effectively, a major step forward in speech recognition. This robustness stems from its training on a vast and varied collection of audio data, encompassing a wide range of accents and noisy environments. This approach helps Whisper adapt to different speaking styles and real-world audio conditions that often challenge other systems.
However, the model's performance isn't uniform across all accents and noise levels. It appears to perform better with certain English dialects, specifically those originating from North America, than others, indicating some limitations in its ability to accurately transcribe accents outside of this range. There are still challenges in consistently handling various English accents and complex acoustic scenarios. This suggests the need for further development to refine the model's ability to handle a broader range of speech variations and ensure truly universal performance across all accents and noise types. Achieving this universal application across all speech types remains a goal for continued advancements in speech recognition technology.
Whisper exhibits a notable ability to handle a wide range of accents and dialects, a significant leap forward from older systems that often struggled with these variations. This suggests that it's getting better at adapting to the subtle nuances of speech across many languages. Interestingly, it seems to achieve this without needing massive amounts of training data for each specific accent, likely due to its transformer architecture allowing for a shared understanding of linguistic features.
One of the more compelling aspects of Whisper is its performance in noisy conditions, which is essential for real-world use. It seems to be quite resilient to background sounds, maintaining a high level of accuracy even when dealing with overlapping speech, such as in a crowded room. This suggests the training data included a variety of background noises, trying to simulate realistic scenarios, which is a more thoughtful approach than older methods. It appears to use clever signal processing to separate speech from other sounds, allowing it to focus on the spoken language even in less-than-perfect acoustic situations.
Further analysis shows Whisper has a remarkable ability to maintain context during extended speech, something that many other models struggle with. They often lose track of what's being said over long stretches of dialogue. This feature is vital for accurately transcribing longer conversations or presentations. The variety of languages used in its training appears to have helped improve accuracy across the board, with particular improvements noted in languages that were previously underrepresented in speech recognition datasets.
Whisper also displays a remarkable capability for quick adaptation to new accents and speech patterns after limited exposure, indicating a potential for ongoing learning. This contrasts with older models, which often need substantial retraining for every new dialect. Additionally, it seems to have a more refined approach to phoneme recognition, recognizing the subtle differences in how sounds are produced across different accents, leading to improved accuracy in differentiating between similar-sounding words. This is especially important in situations where highly accurate transcription is crucial.
Lastly, Whisper benefits from a meticulous error analysis process. It actively identifies and addresses weaknesses in its accent recognition capabilities, contributing to a more refined user experience. This feedback loop directly informs the development of future model versions, fostering continuous improvements in performance and adaptability. This focus on continual improvement showcases the potential of this technology.
Whisper 20 OpenAI's Latest Advancements in Multilingual Speech Recognition - Multilingual Support for Recognition Translation and Identification
OpenAI's Whisper has integrated recognition, translation, and language identification into a single system, significantly improving its support for multiple languages. This integrated approach opens up possibilities for applications that need to handle diverse languages, such as real-time transcription systems that can seamlessly switch between languages or tools that automatically identify the language being spoken. Whisper's ability to handle a wide range of accents and dialects is largely due to its training on a vast dataset of audio data in many languages. While Whisper's multilingual capabilities show much promise, challenges still exist, especially when dealing with less common accents or very complex speech patterns. It seems clear that more research and development are needed to ensure consistently accurate multilingual recognition across all types of speech. As the need for effective speech technology increases, Whisper's advancements represent a substantial step forward in how computers can understand and interact with human language across the globe, holding potential to transform the way we communicate.
Whisper's multilingual prowess stems from its ability to transfer knowledge learned from frequently studied languages to less-common ones. This approach proves surprisingly effective, particularly for languages that typically lack sufficient training data. It's fascinating how it can bridge this data gap, leading to better recognition even in these challenging cases.
The model cleverly incorporates a phonetic embedding layer which helps it understand linguistic similarities across different languages. This approach allows it to better recognize the nuances of different phonemes—the smallest units of sound—that can vary subtly from one language to another. It's a neat example of how deep learning techniques can address the complexities of diverse linguistic patterns.
A particularly interesting feature of Whisper is its ability to identify code-switching, where speakers seamlessly transition between multiple languages within a single conversation. This is a significant advancement, as previous systems often struggled to handle such dynamic language shifts. This opens up new opportunities for accurate transcription in more complex multilingual settings.
Whisper's architecture is designed for efficient fine-tuning with minimal labeled data. This means it can be rapidly adapted to new languages or dialects, which is extremely beneficial for developers working with niche languages or languages in development stages where resources are limited. Its flexibility in this regard is a very useful feature for a broader range of users.
An in-depth look at Whisper's training has shown it develops what appears to be morphological awareness. This means the model seems to grasp how word forms change across languages. This awareness significantly helps it identify the appropriate context for word usage, especially vital in languages with complex grammatical systems. It's an area where many AI models previously struggled, so it is promising.
The model also shows considerable improvement in recognizing emotional tones and the speaker's intent through variations in their speech patterns. This opens exciting avenues for using Whisper in applications like mental health and human-computer interaction where understanding subtle emotional nuances in speech is paramount. Its performance in this area is very promising.
Whisper tackles a problem that's prevalent in many real-world audio scenarios—lower-quality recordings, such as from mobile phones. Its resilience in the face of degraded audio signals makes it incredibly useful in situations where high-fidelity audio cannot be guaranteed. This ability to maintain performance in less-than-ideal conditions is a strength.
The multilingual Whisper model shows remarkable promise in cross-lingual transfers, effectively handling language pairs that are not commonly studied together. It implies that the model can efficiently process less common language combinations, a very useful feat. This suggests it can potentially generalize across languages beyond those it was specifically trained on, though more research is needed to see the extent of its cross-lingual abilities.
Analyzing Whisper's errors has revealed specific challenges it faces in low-resource languages. These insights can help guide future improvements to the model, encouraging more targeted training efforts in areas where it needs further development. By identifying its weaknesses, we can work on resolving them, increasing its overall effectiveness.
Finally, Whisper's integration into real-time applications such as live translation showcases its immense potential to improve global communication. And, its ability to understand context suggests exciting prospects for the evolution of interactive voice assistants in multilingual settings. Its capabilities hint at a future where communication barriers across language and culture become increasingly easier to overcome.
Whisper 20 OpenAI's Latest Advancements in Multilingual Speech Recognition - Open-Source Model Enabling Widespread Access
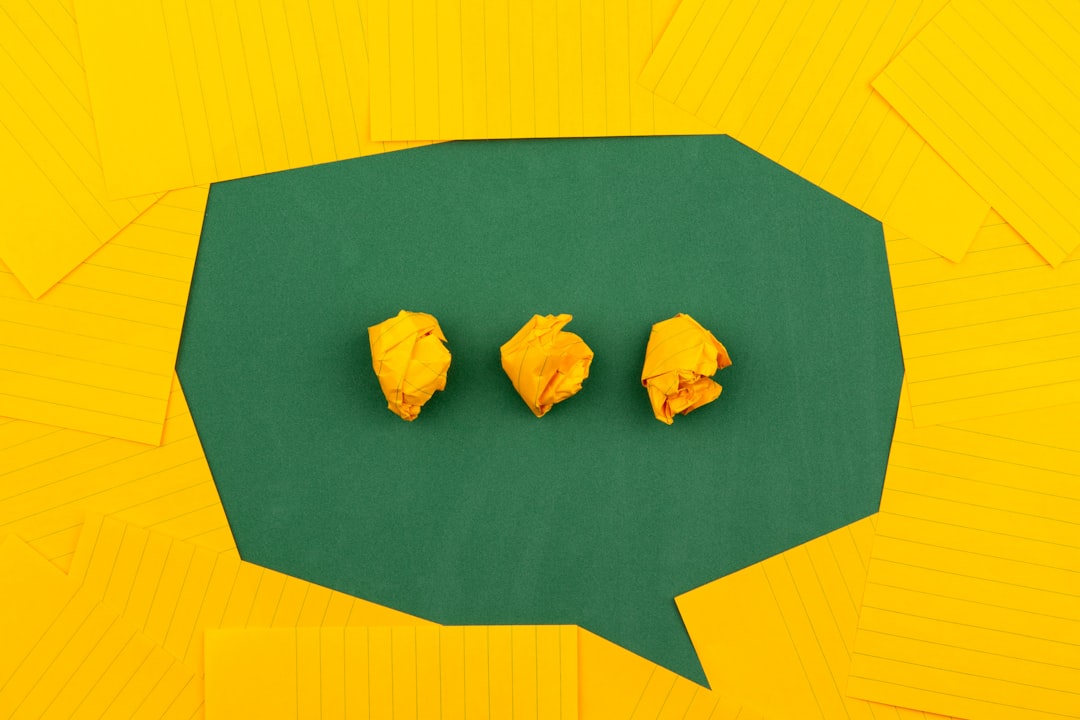
OpenAI's decision to release Whisper as an open-source model represents a notable advancement in making powerful multilingual speech recognition more accessible. By making the model freely available, it encourages a collaborative environment where developers and researchers can build upon and improve its capabilities. This approach facilitates wider access to cutting-edge speech recognition tools, enabling a broad range of applications across a diverse set of languages. While open-sourcing the model accelerates development, it also highlights the need for continued improvements in its accuracy, particularly for less common languages and regional dialects. The open-source nature of Whisper holds the promise of fostering a globally collaborative effort that can refine its current limitations, thereby promoting wider inclusivity within language technology.
The openness of Whisper's design provides a valuable resource for a wide range of developers, allowing them to explore and enhance advanced multilingual speech recognition. This accessibility fosters a collaborative environment where innovation can flourish in areas like healthcare, education, and accessibility tools. Whisper's architecture goes beyond basic transcription, supporting automatic language identification and the ability to seamlessly switch between languages. This feature opens up exciting possibilities for applications dealing with multilingual scenarios like global conferences or diverse work environments.
The open-source nature of the project empowers researchers to contribute their insights and refinements, creating a positive feedback loop that continuously refines the model. This is a contrast to older methods where tailoring a speech recognition model for new accents often demanded significant retraining. Whisper, however, can adapt to new speech variations after only limited exposure. This characteristic reduces the resources and time needed to maintain a model for a broader audience.
It's fascinating how Whisper utilizes a phonetic embedding layer to effectively generalize linguistic sounds across various languages. This strategy is particularly helpful for languages with limited available training data, potentially leading to improved inclusivity in speech technology. The model also demonstrates promising capabilities in recognizing the emotional tone in speech, making it a potential candidate for applications that benefit from emotional understanding, such as interactions in mental health services or more engaging human-computer interfaces.
The design of Whisper places a strong emphasis on preserving context within audio recordings, which is crucial for tasks requiring high accuracy, such as transcribing long conversations or legal proceedings. Open-source contributions have been particularly helpful in improving the model's performance for languages and dialects that are underrepresented, ensuring a wider range of speakers can benefit from speech recognition. Whisper's training process embraces a strategy known as weak supervision, which enables the model to effectively leverage large datasets of unlabeled data. This approach streamlines training and benefits applications in areas where linguistic data is scarce.
Lastly, it's noteworthy that Whisper's design handles real-world challenges like lower-quality audio recordings, reflecting a mindful approach to the potential application scenarios. This robustness is particularly important in locations with limited access to high-quality recording equipment, suggesting the model is adaptable to a wider variety of environments than many previous models. These qualities position Whisper as a strong candidate for advancements in speech technology and represent a crucial step towards more accessible and inclusive communication across languages and cultures.
Whisper 20 OpenAI's Latest Advancements in Multilingual Speech Recognition - Zero-Shot Capability Across Multiple Languages
OpenAI's Whisper demonstrates a notable zero-shot capability across many languages, a direct result of its extensive training on a vast, diverse set of language-based audio data. This means it can perform well in many languages without needing specific training for each one. Consequently, it rivals the accuracy of older speech recognition systems, often considered superior, which require specific training for each language, on typical benchmarks. This zero-shot feature effectively enables Whisper to transfer and generalize learned linguistic principles to various languages and accents, even those with limited training data, making it particularly useful in environments with many languages. This adaptability is a major plus, as real-world situations often include variations in speech patterns and subtle linguistic differences which can confuse less robust systems. Despite its impressive versatility, Whisper still has room for improvement. It's crucial to address certain areas where it struggles, particularly with less common languages and more complex linguistic structures. Further development in these areas is vital to achieving even higher levels of performance across the language spectrum.
Whisper's impressive zero-shot capability allows it to tackle languages it hasn't been specifically trained on. It seems to effectively transfer knowledge learned from commonly studied languages to those with less available training data, which is fascinating. This raises questions about how neural networks internally transfer knowledge across different language structures.
It appears Whisper possesses an inherent grasp of phonetic patterns. It can estimate linguistic features in unfamiliar languages based on exposure to similar sounds during training. This suggests that the model might rely on general acoustic similarities to make educated guesses about the structure of an unknown language.
Because of the breadth of its training data, Whisper can apply contextual clues from well-represented languages to understand the grammatical and phonetic intricacies of languages with less data. This potential to bridge the gap between high-resource and low-resource languages promotes the wider accessibility of speech technology worldwide.
Intriguingly, the model tends to perform better on languages with related phonetic systems. This reinforces the idea that Whisper can leverage linguistic similarities even without direct training for specific languages. It might be that the underlying structure of those related languages allows for easier transfer of learning from one to another.
Whisper can also perform zero-shot language identification. This shows the model effectively picks up on patterns associated with different languages, enabling it to figure out what language is being spoken without much pre-training. This feature is particularly valuable in applications requiring dynamic language switching or automatic language detection.
Studying Whisper's zero-shot capability reveals an inherent adaptability, particularly suited for multilingual environments where quick deployment and adaptation are crucial. This characteristic makes Whisper a compelling option for industries that require rapid implementation of speech recognition across diverse language communities without substantial re-training.
From a practical standpoint, Whisper's zero-shot abilities potentially streamline development by reducing the time and resources needed to create tailored recognition models for each language. This could translate into a quicker and more efficient expansion of language support for different technologies.
The model's performance in recognizing regional variations within a language in zero-shot settings provides a fascinating opportunity for future research. It hints that lessons learned from major language variants can be transferred to similar dialects, albeit with varying levels of success.
It's interesting that Whisper utilizes its phonetic embeddings to understand how dialects differ. This potentially helps improve its accuracy in capturing subtle phonetic variations within languages, a feature often overlooked by earlier speech recognition systems. This suggests there is more to just recognizing sounds: the model might be learning the nuances in how sounds are put together to make words.
While Whisper's zero-shot performance is impressive, ongoing testing highlights that it still struggles with certain languages and regional accents. This reinforces the need for continuous refinement and the development of targeted training processes to further enhance its understanding of the complexities of language. There are definitely limitations, and more work needs to be done to resolve them.
Whisper 20 OpenAI's Latest Advancements in Multilingual Speech Recognition - Addressing Growing Demand for Multilingual ASR Technology
The increasing complexity of global communication fuels a growing need for automatic speech recognition (ASR) systems that can understand and process a wide variety of languages. OpenAI's Whisper model addresses this need by demonstrating strong capabilities in handling diverse accents and languages. Its design leverages techniques like phonetic embedding and cross-lingual knowledge transfer to effectively handle both frequently studied languages and those with limited training data. The model's ability to perform well in languages it wasn't explicitly trained for – known as zero-shot capabilities – makes it incredibly adaptable and suitable for a range of real-world scenarios.
However, Whisper's journey towards universal multilingual speech recognition is not without hurdles. It still faces limitations, particularly when dealing with less frequently used languages or complex regional dialects. These areas require further development and research to ensure that the model's accuracy remains high across all language varieties. Despite these challenges, Whisper stands as a critical step forward in building a more inclusive future for speech technology, one that can effectively accommodate and bridge the vast linguistic diversity of the world.
Whisper's architecture seems designed to tackle the growing need for speech recognition in a world of diverse languages. It exhibits a strong ability to leverage limited language resources, potentially opening speech recognition to speakers of less commonly studied languages, a space often overlooked in the past. This is interesting as it hints at a potential for democratization of these technologies.
One interesting aspect is how Whisper incorporates a phonetic embedding layer. It allows the model to understand how similar sounds across different languages relate to one another, potentially aiding recognition in languages with limited data. This generalisation of phonetic similarities is pretty clever, as it overcomes hurdles that often arise when a model is only trained on a narrow set of sounds.
Another aspect that stands out is the model's quick adaptation capabilities. This contrasts with older systems, which frequently demanded significant retraining for every new accent. It can seemingly handle new accents and dialects after only limited exposure, making it more practical for a wide range of uses.
One rather practical ability Whisper has is its handling of "code-switching". Code-switching is when a speaker jumps between two or more languages in the same conversation. This ability is quite a leap forward, as older models typically struggled with this type of dynamic linguistic change. It hints at possibilities for more accurate transcription in mixed-language scenarios.
Whisper also seems adept at maintaining context across long segments of audio. This was an issue for many older systems which would frequently lose track of the ongoing conversation, especially when dealing with extended dialogue. This improvement would likely be helpful in situations where precise transcription is needed, like during long talks or meetings.
Intriguingly, Whisper seems able to distinguish emotional nuances and intent shifts through speech patterns. This might lead to more sophisticated applications in areas like mental health support or marketing analytics where understanding subtle emotional signals is crucial. It's a bit unexpected for a speech recognition model, but shows it's moving beyond just literal transcription.
Whisper's resilience when dealing with low-quality audio is also quite significant. This makes it very applicable in scenarios where the audio quality isn't ideal, like phone calls or mobile recordings. This is helpful in the real-world where things like background noise are unavoidable.
The model shows some promise in its handling of less commonly paired languages. This ability to transfer knowledge across languages that aren't often studied together suggests that there is more to the model than just recognizing sounds – it might have an ability to generalize across languages in ways we don't yet fully understand.
Another fascinating feature is Whisper's capacity to identify a spoken language without prior training. This "zero-shot" language identification ability is a critical shift in the technology. It suggests that there's an element of learned generalization taking place, and its relevance to dynamic language environments is pretty evident.
Throughout the model's development, they've implemented careful error analysis. This approach, in turn, delivers insightful data and guides improvements in specific areas where Whisper struggles. This type of ongoing refinement is especially valuable for languages with limited resources, where the data available for training is often quite sparse.
In summary, while the landscape of multilingual speech recognition continues to evolve, Whisper showcases many promising features for the future of how computers handle human speech in a multilingual environment.
Experience error-free AI audio transcription that's faster and cheaper than human transcription and includes speaker recognition by default! (Get started for free)
More Posts from transcribethis.io: