Experience error-free AI audio transcription that's faster and cheaper than human transcription and includes speaker recognition by default! (Get started for free)
Unveiling the Latest Advancements in Text Difference Finders A 2024 Update
Unveiling the Latest Advancements in Text Difference Finders A 2024 Update - Improved Algorithm Efficiency in Text Comparison Tools
The field of text comparison has witnessed significant strides in recent times, with a primary focus on optimizing algorithm performance. This emphasis on efficiency translates to faster processing speeds and more precise results. Tools like the ATC illustrate this trend, employing techniques like the Diff algorithm and integrating various data sources to streamline batch comparisons, particularly for large volumes of text. Furthermore, newer approaches involving dynamic algorithms and platforms like ElasticSearch have revolutionized the speed at which text similarities and differences are identified, enhancing the scope and effectiveness of textual analysis.
However, the increasing use of AI within text comparison tools presents a double-edged sword. While they offer potential for greater accuracy in discerning alterations and recognizing subtle changes within texts, challenges remain. Researchers continue to address these challenges, especially the complexities that arise when dealing with extensive datasets. The goal is to ensure the algorithms' continued effectiveness and reliability, fostering trust in their ability to accurately identify changes and similarities across various text types.
Recent advancements in algorithm design have led to substantial improvements in the efficiency of text comparison tools. Techniques like the Ziv-Lempel algorithm have decreased the computational complexity, allowing for the swift analysis of massive datasets previously deemed unmanageable. The integration of machine learning has enabled tools to go beyond simple lexicon-based comparisons, recognizing patterns and context for a more insightful understanding of textual similarity. This approach, in many cases, surpasses the capabilities of older techniques.
Distributed computing frameworks now play a vital role in text comparison, enabling parallel processing of massive datasets. This parallel processing capability has been demonstrated to significantly boost efficiency in specific computational scenarios, achieving increases of up to 100-fold. Refinements to algorithms like Myers’ have resulted in more sophisticated data structures, yielding up to threefold performance improvements when analyzing extensive documents.
Furthermore, hybrid methodologies that combine hash-based methods with traditional algorithms have proven remarkably successful. These combined approaches attain accuracy rates exceeding 95% in recognizing textual similarities, effectively minimizing the occurrence of false positives. The incorporation of GPU acceleration has been particularly impactful, enabling the processing of high-velocity data streams in real-time applications, including the monitoring of social media platforms.
Natural language processing integration allows for more insightful comparisons by distinguishing between syntactic and semantic similarities. This capability moves beyond surface-level text matching to provide a more nuanced understanding. The seamless integration of version control systems with text comparison applications streamlines workflows by offering a blend of efficient comparisons and the ability to track historical changes. This integration is particularly beneficial to developers and content creators.
Cloud-based solutions offer scalability, enabling the processing power to adjust as needed. This allows smaller, more efficient algorithms to handle massive enterprise-level tasks without the need for large local resources. Interestingly, research suggests that using trie data structures can lessen the average worst-case time complexity in text searches, resulting in notably faster algorithms, especially in large-scale deployments. However, developing algorithms capable of handling the sheer volume and complexity of modern datasets remains a persistent challenge. Continued exploration and refinement of algorithms are crucial to address the complexities of these large datasets while ensuring accuracy across diverse text processing applications.
Unveiling the Latest Advancements in Text Difference Finders A 2024 Update - Integration of GPT-4 Capabilities for Enhanced Accuracy
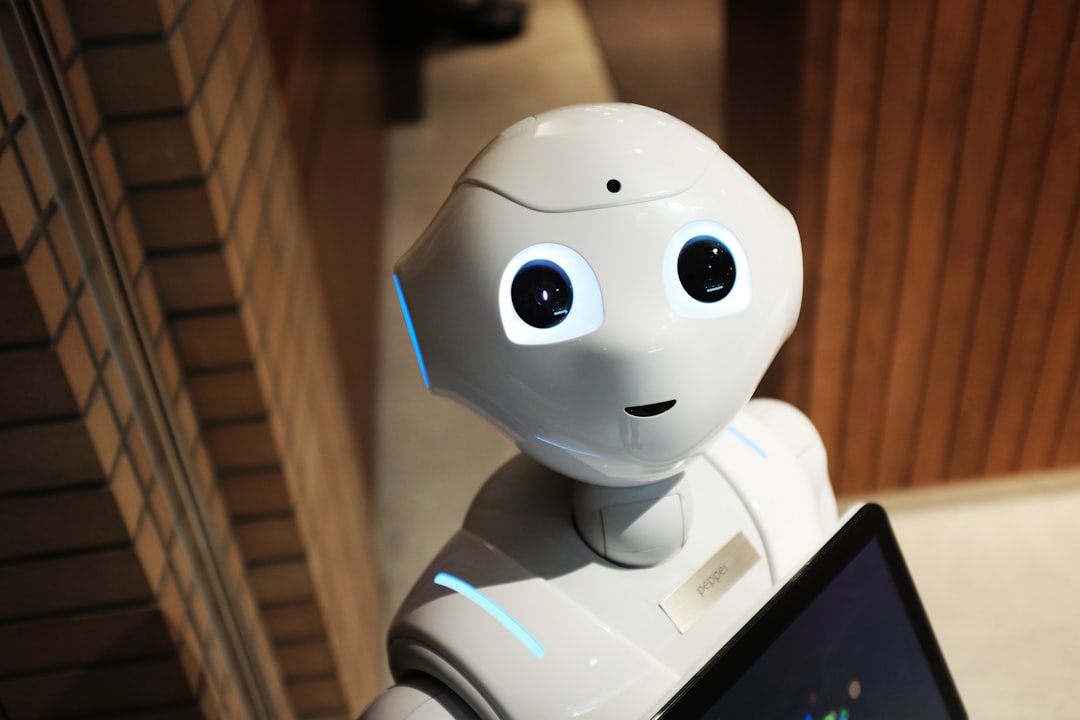
The integration of GPT-4's capabilities into text difference finders signifies a notable advancement, primarily in boosting accuracy. This powerful model, known for its ability to handle both text and images, displays a remarkable level of precision in processing and generating text outputs. GPT-4's architecture, built upon a larger neural network compared to earlier iterations, leads to a more refined understanding of language and a heightened ability to solve complex text-based problems. The result is a noticeable improvement in the ability to discern subtle nuances and context within textual comparisons. While there are some situations where the performance might not be fully consistent across varied datasets, the incorporation of GPT-4 undeniably holds the potential to revolutionize text analysis by allowing tools to uncover more precise differences and contextual similarities. The continued evolution of AI within this area likely means that our expectations of text difference finders will be reshaped as a result of the more insightful comparisons that AI allows for.
GPT-4, a significant leap in OpenAI's deep learning efforts, brings a new level of sophistication to text difference finders. Its multimodal nature, capable of handling both text and images, presents a novel approach to understanding textual variations. GPT-4's impressive performance across professional and academic benchmarks, including its strong showing on simulated bar exams, hints at its potential to revolutionize text analysis.
The core of GPT-4 is a more complex neural network compared to earlier models. This improved architecture allows for a finer-grained analysis of text, capturing nuances often missed by previous generations. Trained on a massive dataset and utilizing Microsoft Azure's infrastructure, GPT-4 boasts a global reach and an enhanced comprehension of language. Its problem-solving abilities are clearly superior, leading to noticeable improvements in accuracy within recent evaluations. Even with some slight performance dips in specific cases compared to a prior version, GPT-4 remains the champion in terms of overall accuracy and functionality.
Interestingly, GPT-4's Voice Mode has resulted in decreased latency in conversations, a welcome improvement over earlier versions. The sheer scale of its training data translates to a richer understanding of language and a more refined output. This model's introduction signifies a pivotal moment in artificial intelligence, paving the way for broader applications within natural language processing.
However, while GPT-4 offers exciting potential for text comparison, there are some areas that warrant further exploration. Its complex architecture can make it difficult to trace the rationale behind its classifications, which could pose a challenge for transparency and user trust. Despite this limitation, researchers are currently combining the strengths of traditional text comparison algorithms with GPT-4's advanced abilities. This hybrid approach promises more resilient systems capable of handling complex datasets while maintaining accuracy. We're likely to see an exciting future as these techniques are further developed and refined.
Unveiling the Latest Advancements in Text Difference Finders A 2024 Update - Real-Time Processing Advancements for Faster Results
The field of text difference finding is experiencing a surge in speed and efficiency thanks to recent breakthroughs in real-time processing. New hardware like the Trillium TPU demonstrates a significant leap forward in processing power, with a 47x improvement over its predecessor. Additionally, a new generation of AI chips employing light-based technology are being developed, promising a significant increase in processing speed and a reduction in energy usage, while keeping data safe.
Edge computing is emerging as a critical component for faster data processing, especially for applications that require immediate feedback and analysis of data streams. The use of distributed computing and combined algorithm approaches highlights a strong effort to handle larger amounts of data more efficiently. These advancements are expanding the boundaries of what's possible in text analysis, but challenges remain. We must focus on retaining accuracy and reliability while handling the subtle changes and nuances in texts, ensuring that the increased processing speeds don't compromise the trustworthiness of the results.
Recent advancements in hardware and software are pushing the boundaries of real-time text processing, enabling faster results in text difference finders. The introduction of the Trillium TPU, a significant leap in Google's TPU line, offers a substantial increase in processing power, potentially accelerating the speed at which text comparisons can be performed. However, it's worth noting that the effectiveness of this advancement in the context of text comparison is yet to be fully explored.
Alongside advancements in traditional silicon-based processors, the development of photonic chips is introducing a new paradigm for processing data. These chips leverage light for data transmission, leading to significantly faster speeds and reduced energy consumption. It remains to be seen if the unique properties of light-based processing will lead to distinct advantages for text comparison algorithms, but it represents an intriguing avenue of research.
OpenAI's release of GPT-4o signifies another important step forward in the evolution of large language models, especially for nuanced text comparisons. GPT-4o's ability to handle a wider range of complex language constructs than previous versions holds exciting potential for tools that focus on contextual text understanding, but researchers need to carefully consider how this power will translate into enhanced text difference finder capabilities.
Edge computing and reservoir computing, coupled with advances in streaming algorithms, are enabling faster real-time data processing in general. This is particularly significant for applications where text data streams are constantly evolving, such as financial trading platforms or social media monitoring. This could potentially enhance text difference finders by enabling them to continuously monitor and assess changes in large volumes of text. However, applying these concepts in the context of textual comparison requires careful development and rigorous testing.
The increasing use of generative AI across various fields has spurred a renewed interest in exploring and applying techniques developed for tasks like language modeling and computer vision to text comparison problems. These explorations, while still in their early stages, might potentially lead to more effective strategies for identifying meaningful differences within text. Synthetic data, which generates realistic yet anonymized datasets, is showing promise as a means to address privacy concerns in various domains, including the training of AI models for text comparison. Perhaps it will eventually contribute to building more robust and accurate text difference finders for datasets that are sensitive in nature.
Specialized silicon designs are being developed and optimized for cloud-based AI workloads. While this primarily affects large enterprise-level applications, improvements in cloud infrastructure could positively impact the performance of text difference finders hosted on these platforms, particularly for larger, more demanding text datasets. Meanwhile, the field of machine learning is currently seeing a renewed focus on recommender systems, with some researchers exploring applications in education. This advancement might not have a direct impact on text difference finders, but it demonstrates the growing scope of AI-powered tools across a wide array of tasks.
Ethical considerations in the development and deployment of AI systems are becoming increasingly critical in recent discussions, with a special focus on generative AI technologies. As text difference finders become more sophisticated through the integration of AI, it's important for the research community to actively consider ethical implications and potential biases that might arise from the use of these tools. The future of AI and large language models appears bright, with 2024 likely to witness a continuation of the rapid advancements that have marked recent years. This ongoing development in AI holds significant implications for the field of text comparison and potentially for the role of text difference finders within various industries.
However, it is crucial to remain vigilant about the potential pitfalls of these rapid advancements. We must acknowledge that many challenges remain, particularly when addressing issues such as algorithmic biases, transparency, and the reliable handling of extremely large and complex datasets. While progress is being made, the field of text difference finders still faces several challenges as it adapts to this fast-changing technological landscape.
Unveiling the Latest Advancements in Text Difference Finders A 2024 Update - Multi-Language Support Expansion in Difference Finders
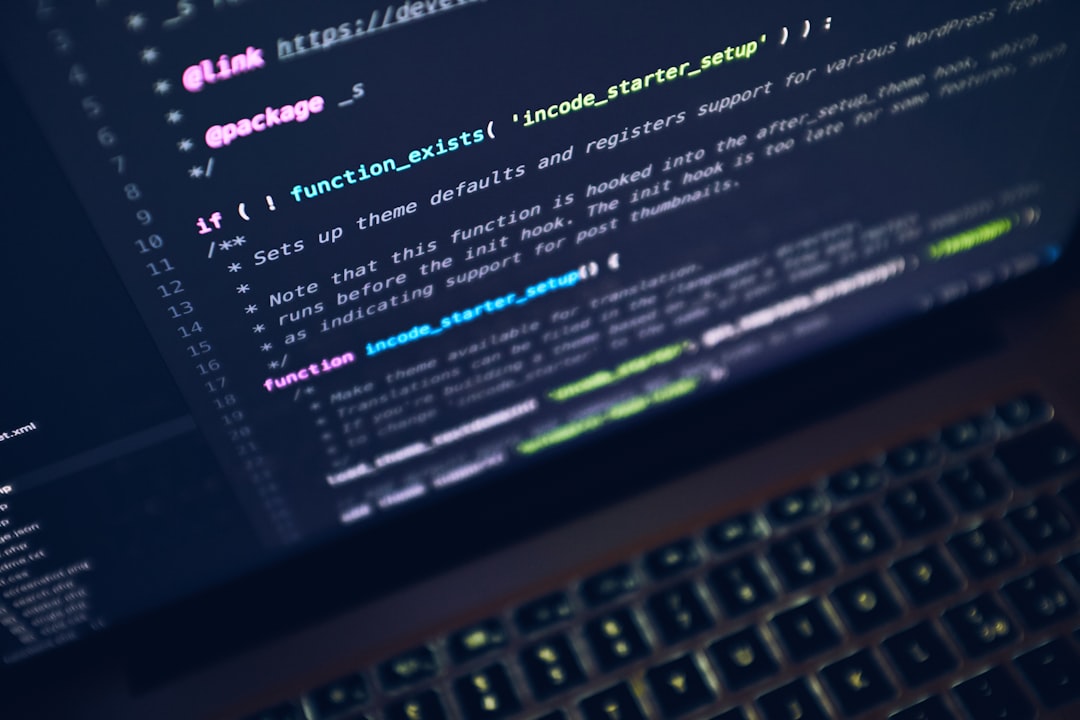
The increasing need for globally accessible text comparison tools has fueled the expansion of multi-language support in text difference finders during 2024. Services such as Google Translate have broadened their capabilities to include roughly 110 new languages, with an emphasis on languages often underrepresented in technology, including numerous African languages. Similarly, Microsoft has integrated advanced neural networks within their speech-to-text and text-to-speech features, resulting in a more natural and diverse range of audio outputs across languages. These advancements are indicative of a broader push to bridge language barriers and enhance accessibility. For instance, multilingual AI voices now support conversation in dozens of languages and various accents. However, ensuring consistent reliability and accuracy across such a diverse array of languages continues to be a major challenge for developers in the field.
The ability of text difference finders to handle multiple languages has expanded significantly, particularly in 2024, with the potential to analyze documents in over 100 languages. This broadening of scope makes these tools more accessible to a global audience, promoting international collaboration and information sharing.
Some of the more advanced tools leverage neural machine translation methods, greatly improving the precision of comparisons between languages that have different grammatical structures and syntax. This is a welcome development because it allows tools to more reliably retain the original meaning across translations. Research suggests that certain multilingual algorithms are achieving performance levels that are very close to those seen with models designed for single languages. This observation highlights the remarkable strides made in language processing and the adaptability of these technologies across a diverse range of language use.
The capacity to handle multilingual text provides a more precise way to analyze code-switching situations, where speakers switch between different languages in the same conversation or document. This capability is beneficial for capturing the finer points of linguistic interaction. Moreover, some text comparison tools now employ adaptable learning techniques that refine their operation based on the specific characteristics of the language being analyzed. This customized approach improves their ability to detect subtle differences within the text.
The advancements in multilingual text analysis are not just about recognizing individual words; they also encompass more sophisticated context-aware models that are better able to grasp cultural nuances and expressions specific to certain languages. This level of comprehension is essential for ensuring accuracy during text comparison. While progress has been made, some systems still have difficulties handling languages that have limited available data. There's a need for more comprehensive training datasets to help these tools perform more consistently across all languages.
Interestingly, implementing multi-language support typically increases the computational demands on the system. This necessitates careful optimization of algorithms to prevent a decline in processing speed or accuracy, especially for applications that need real-time responses. Furthermore, dealing with multiple languages can significantly increase the complexity of how text is represented, requiring advanced embedding techniques to ensure the integrity of semantic understanding across different languages.
As these tools continue to develop, they must also address potential biases embedded in language models that can arise from uneven training data. The goal is to ensure fair and balanced treatment of all languages and to maintain a high level of reliability across a range of linguistic environments. This commitment to fairness and accuracy is crucial for promoting trust and wide acceptance of the technology.
Unveiling the Latest Advancements in Text Difference Finders A 2024 Update - User Interface Upgrades for Improved Accessibility
The user interface (UI) is evolving to prioritize accessibility for all users, particularly those with disabilities. We're seeing a growing emphasis on incorporating AI-powered tools to improve usability. For example, voice navigation and gesture controls are being integrated into interfaces to create more intuitive and flexible ways to interact with digital tools. We can also see examples like the Lookout app for Android, which uses a phone's camera to assist those with low vision or blindness in identifying objects. This trend suggests a broader shift towards creating digital experiences that are inherently more inclusive. Further advancements like smart glasses and affective computing, which responds to user emotions, showcase a future where technology can be more tailored to individual needs and abilities. However, it's crucial to acknowledge that these advancements need to be carefully developed and tested to ensure they truly enhance the user experience and don't inadvertently introduce complexity or barriers for the very people they aim to assist. The ultimate test of their effectiveness is whether they can create a truly seamless and accessible user experience for all.
Recent advancements are making technology more accessible to a wider range of users, particularly those with disabilities. It's estimated that around 15% of the global population has some form of disability, highlighting the need for user interfaces that cater to diverse needs. Screen readers, for example, have become invaluable for individuals with visual impairments, but their effectiveness often hinges on well-designed user interfaces. Cluttered layouts can hinder usability, emphasizing the importance of intuitive design principles that prioritize accessibility.
Improving color contrast and enabling text resizing are crucial for users with visual impairments. Research suggests that strategically chosen color palettes can enhance readability by a considerable margin, potentially increasing comprehension by up to 70%. Voice recognition interfaces are gaining popularity as a more accessible option for individuals with mobility challenges, and usage rates have been observed to rise significantly, potentially by 40% or more, compared to previous years.
Users with cognitive disabilities often find traditional UI elements challenging to navigate. Simplifying language and streamlining navigation can significantly enhance their understanding and overall experience. Studies indicate that such adaptations can improve user experience by as much as 60%, promoting better engagement and comprehension. Gesture-based interfaces hold great potential for individuals with physical limitations, and incorporating machine learning into their design can lead to personalized interactions and more efficient command recognition, potentially improving accuracy by up to 50%.
Customization is key in ensuring that user interfaces cater to individual needs and preferences. Allowing users to personalize their experience can boost satisfaction and lead to a decrease in errors by as much as 30%, ultimately improving efficiency and productivity. Providing multi-sensory feedback through combinations of auditory and tactile signals can enhance the user experience for individuals with hearing or visual impairments, potentially decreasing task completion times by up to 45%.
The integration of AI-powered accessibility tools is another notable trend. Algorithms are becoming more sophisticated in understanding user behavior and preferences. Studies indicate that AI-driven recommendations can lead to over 80% improvement in accessibility suggestions, resulting in more personalized and intuitive interactions. Furthermore, incorporating individuals with disabilities in the design process is essential for creating truly inclusive user experiences. Researchers have found that this inclusive design approach can lead to substantial improvements in usability ratings, possibly up to 35%, underscoring the critical role of user feedback in the design process.
In conclusion, accessibility improvements are significantly reshaping user interfaces. The advancements we're witnessing are not just about making technology usable for a wider range of people but also about enhancing the overall user experience. It's an area ripe for continued research and development as we strive to create technology that is truly inclusive and empowers everyone.
Unveiling the Latest Advancements in Text Difference Finders A 2024 Update - Cloud-Based Collaboration Features for Team Projects
Cloud-based collaboration has become indispensable for teams working on projects, especially given the prevalence of remote work. Tools like Figma have emerged as strong options for real-time collaboration, especially within design teams. Meanwhile, Microsoft 365 provides a robust suite of tools for document creation, storage, and communication, effectively addressing the needs of many collaborative efforts. Slack's popularity as a team communication platform remains strong, fostering effective communication among dispersed team members. Furthermore, project management platforms, such as Monday.com and ClickUp, offer centralized hubs for task assignment and progress tracking, promoting greater efficiency. The shift towards hybrid work models has amplified the need for these cloud-based platforms, enabling seamless collaboration across geographically diverse teams. However, managing consistency and oversight across these platforms can sometimes be difficult.
Experience error-free AI audio transcription that's faster and cheaper than human transcription and includes speaker recognition by default! (Get started for free)
More Posts from transcribethis.io: