Experience error-free AI audio transcription that's faster and cheaper than human transcription and includes speaker recognition by default! (Get started for free)
Understanding AI Cartoon Generation A Deep Dive into Image-to-Cartoon Conversion Quality Benchmarks
Understanding AI Cartoon Generation A Deep Dive into Image-to-Cartoon Conversion Quality Benchmarks - AI Cartoon Quality Benchmarks Through Neural Network Analysis 2024
Evaluating the quality of AI-generated cartoons has seen notable progress in 2024, with benchmarks increasingly sophisticated. Methods like CLIPAGIQA strive to enhance image assessment by incorporating multimodal understanding, though their practical use still presents obstacles. The rise of user-friendly tools such as Vidnoz AI and Fotor's Cartoon Photo Editor demonstrates a focus on improving the user experience and expanding editing capabilities, offering new possibilities for creators. Google's research on the NIMA model is important as it proposes a way to assess both the technical and artistic facets of AI-generated images, potentially shaping future quality benchmarks. However, as these benchmarks mature, it's crucial to remain vigilant about the techniques used to ensure that evaluations are unbiased and effectively reflect the true strengths of different AI systems. The field of AI cartoon generation is dynamic and its evolution hinges on these continuous refinements.
1. The development of neural networks, like GANs and VAEs, has brought about substantial enhancements in AI-generated cartoons, mitigating the common issues of image artifacts previously seen in older systems.
2. AI is now using high-resolution images to super-resolve and transform photos into cartoon versions, preserving intricate details while sharpening the final outcome.
3. The use of perceptual loss functions, which place emphasis on how humans perceive image quality, is increasingly implemented to fine-tune cartoon output, pushing the boundaries of image fidelity.
4. Watermark removal in AI software has gone beyond basic cloning, now utilizing deep learning models trained to reconstruct image sections convincingly, making it hard to pinpoint any manipulation.
5. Style transfer methods provide fine-grained control over cartoon styles, letting users apply specific artistic looks while maintaining the essential features of the original picture.
6. Evaluating AI cartoon quality now often factors in user feedback, acknowledging that the perceived quality can significantly differ between algorithms depending on what users find visually appealing.
7. Studies suggest that utilizing a combination of different neural networks (ensemble methods) can enhance overall image quality by providing a more diverse and thorough analysis of the input image.
8. New datasets specifically designed for AI cartooning are enabling algorithms to better understand and replicate the characteristic elements of cartoon art, moving away from the use of general image data.
9. Metrics traditionally used for assessing photo quality, such as the Structural Similarity Index (SSIM), are being adapted to judge cartoon quality, highlighting the complex nature of evaluating art versus realistic visuals.
10. Implementing feedback loops into AI models allows for ongoing improvements in cartoon generation as they learn from user interactions and preferences, refining the image output with each iteration.
Understanding AI Cartoon Generation A Deep Dive into Image-to-Cartoon Conversion Quality Benchmarks - Precision vs Speed Tradeoffs in Modern Cartoon Generation Pipelines
The pursuit of high-quality AI-generated cartoons involves navigating a constant balancing act between precision and speed within the pipelines that power these transformations. Techniques like GANs and diffusion models have undeniably advanced the quality of cartoon outputs, bringing forth sharper details and more nuanced styles. However, the desire for highly realistic and intricately styled cartoons often comes at the expense of longer processing times. This can create friction for users seeking immediate results, particularly in applications where rapid cartoon generation is essential. On the other hand, prioritizing speed might necessitate compromising on the richness of details and the subtlety of the artistic style. The challenge is clear: how to reconcile the need for efficient cartoonization with the desire for truly high-quality and expressive results. Understanding this ongoing interplay will likely play a crucial role in guiding the future of AI-powered cartoon generation, influencing how users interact with and create in these evolving creative spaces.
In the realm of AI-driven cartoon generation, achieving a balance between speed and precision remains a core challenge. Faster processing times can often lead to compromises in the quality of the resulting cartoon, impacting the realism or fidelity of the output. For example, integrating real-time rendering into the pipeline allows for instant feedback during editing but might result in less intricate details or a less consistent artistic style when compared to methods that process images offline.
Some AI systems employ progressive refinement, where a basic cartoon is initially created and then gradually improved in subsequent steps. While this approach can be effective, it can also introduce inefficiencies if not carefully managed. The enhancements might not always meet user expectations, leading to potential dissatisfaction. Striking a balance between the fidelity of the final cartoon and the computational resources needed to achieve it presents another significant hurdle. High-definition cartoons often demand greater processing power, potentially limiting accessibility for users with limited computing resources.
The development of advanced upscaling algorithms like Super Resolution Generative Adversarial Networks (SRGANs) offers exciting possibilities for preserving details in large-format cartoons. However, they can also introduce new artifacts that require careful correction. Similarly, when AI is used for watermark removal, a trade-off emerges between seamlessly integrating the removed area into the image and the processing time required for flawless output. Rushed methods can often produce unconvincing results.
User-defined stylistic preferences add another layer of complexity to the generation process. When users request specific artistic styles, AI systems must delicately balance these requests with the underlying algorithms designed to ensure consistency across different styles. Unfortunately, many current systems still struggle with intricate textures or blended styles, leading to compromises in overall quality unless additional processing steps are incorporated to refine the outcome.
The increasing focus on perceptual quality metrics can also lead to unintended consequences. While aiming to achieve subjectively pleasing results, aspects like consistency and geometric accuracy, traditionally considered important, may be sacrificed in the process. Finally, while continuous model training using user feedback can undoubtedly enhance cartoon generation, reliance on this feedback loop needs careful management. Over-reliance can inadvertently perpetuate biases in the generated outputs. These intricate relationships between precision and speed require ongoing research and refinement to ensure that the quality of AI-generated cartoons aligns with the diverse expectations of users and creators.
Understanding AI Cartoon Generation A Deep Dive into Image-to-Cartoon Conversion Quality Benchmarks - Technical Architecture Behind Image Stylization Models
The core of AI-powered image stylization, particularly in applications like transforming photos into cartoon-like images, relies on advanced deep learning techniques. Generative Adversarial Networks (GANs) are at the heart of these systems, allowing for the creation of stylized images by cleverly separating content and style information. Models like CartoonGAN and AnimeGAN highlight this process, using intricate autoencoder architectures to achieve this separation. Recent advancements, exemplified by the CartoonRenderer framework, have significantly improved results by using a unique approach called instance-based learning. This approach involves training the model on two sets of images – real-world photographs and cartoon illustrations – which leads to higher quality cartoons that maintain the fundamental meaning of the original photo. Despite these strides, certain challenges persist. Handling high-contrast or visually complex images remains a hurdle for many current AI models in the cartoonization process. The ongoing demand for generating a wider array of cartoon styles presents a continuous challenge to refine and develop the algorithms to better handle the diverse preferences of users while ensuring the fidelity of the final results is maintained, regardless of the intricacy of the input.
AI-powered image stylization, like turning photos into cartoons, increasingly relies on deep learning methods, particularly Generative Adversarial Networks (GANs) or similar architectures. Models like CartoonGAN and AnimeGAN have shown promise in this area, but they face hurdles when dealing with certain image characteristics. A key aspect of these models is their ability to learn from diverse datasets of real photos and the desired cartoon styles. This learning process is crucial for separating content from style, preventing ambiguity in the final output. While some frameworks, like CartoonRenderer, divide the process into three stages—Modeling, Coordinating, and Rendering—within an autoencoder framework, the instance-based approach is proving more successful.
However, these advanced methods still face challenges with high-contrast or complex images. It appears that some fundamental aspects of our visual perception related to edges and textures in cartoons present hurdles for these AI models. It's fascinating that the rise of the cartoon industry has driven research into these specific image processing techniques, not just for style transfer but also for quality assessment. The ability to handle higher-resolution images is a major improvement in current techniques like CartoonRenderer, addressing a limitation in earlier methods.
There's a notable lack of comprehensive studies exploring the diverse applications of 2D cartoon image processing. While we see steady progress in AI-powered cartoon generation, a deeper understanding of the underlying processes is needed. Specifically, multi-scale image processing has gained importance, enabling models to analyze image features across different resolutions. This allows for the simultaneous capture of fine details and broader image structure, improving the final outcome. The application of Neural Architecture Search (NAS) has been a game-changer, allowing for automatic optimization of model architectures. This leads to potentially more efficient and effective models.
Another notable improvement is the rise of content-aware processing. Instead of merely applying a specific cartoon style, these models are getting better at understanding the image content. This means that the cartoon style can be adjusted based on what the image depicts, leading to more natural and intuitive results. This concept of dynamic adaptation is evident in video stylization as well. Models are now capable of maintaining temporal coherence in video cartoons, reducing flickering or other unpleasant artifacts, allowing for smoother transitions across frames.
Fine-tuning with generative adversarial methods is an important strategy, where a model that's been trained on a large dataset undergoes further training on a smaller, curated dataset. This enables the model to tailor its output to specific artistic styles with more precision. Loss functions, the mathematical expressions that guide the learning process, have seen notable improvements. Loss functions inspired by psychology and color perception are improving how models assess the generated outputs. This suggests that it's not just about a technical assessment of image quality, but an attempt to reflect how humans perceive and find artistic merit.
Advanced upscaling methods like NAISR have been integrated, enabling the generation of high-quality cartoons from lower-resolution input. Real-time editing capabilities have also emerged, enabling users to interact with the stylization process in real-time, giving users immediate control over the output. The development of new quality metrics specific to stylized images, like Artistic Structure Similarity Index (ASSI), are an exciting step. These metrics are designed to reflect the characteristics we associate with "artistic" image quality rather than just realism. The future of cartoon generation holds exciting possibilities for innovation and improvement. The convergence of deep learning and advanced image processing techniques offers potential for creating new forms of expressive visuals.
Understanding AI Cartoon Generation A Deep Dive into Image-to-Cartoon Conversion Quality Benchmarks - Common Image Processing Challenges in Cartoonization
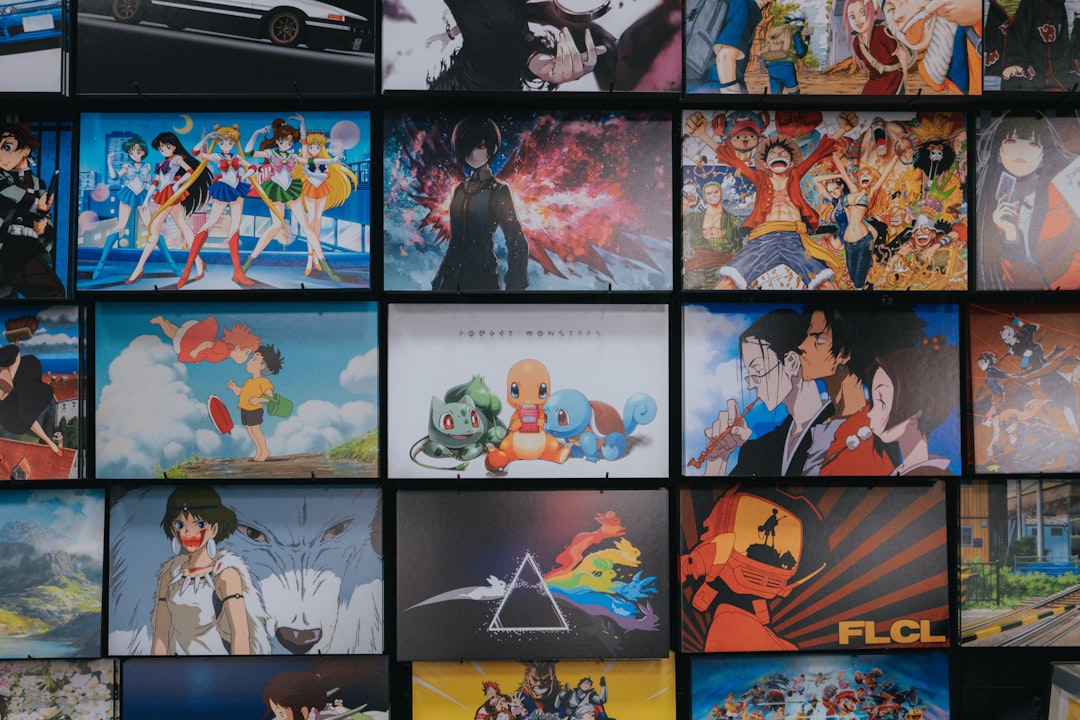
Transforming photographs into cartoon-style images, a process known as cartoonization, involves a complex set of image processing challenges. A key difficulty is finding the right balance between simplifying the visual information while retaining the essential characteristics of the original picture. While AI techniques, especially deep learning, have dramatically improved cartoonization, hurdles remain. Issues like accurately representing intricate details in complex scenes and ensuring smooth transitions between color regions continue to pose a challenge. Furthermore, many algorithms struggle when faced with images that have strong contrasts or high levels of detail.
The diverse artistic styles that users expect add another layer of difficulty. AI systems must adapt and refine their approaches to match a wide variety of preferences without sacrificing the quality of the cartoonized image. Developing models that seamlessly integrate artistic styles and achieve consistent results, regardless of the source image, requires ongoing research and refinement. Striking a balance between achieving fast processing times and generating truly high-quality, visually appealing results remains a critical ongoing pursuit within this field. The field's progress shows that achieving a satisfying level of cartoonization involves navigating a complex path between technical capabilities and artistic expression.
Converting realistic photographs into stylized cartoon images presents a number of intriguing challenges. One persistent issue is maintaining a balance between simplification and detail preservation. While cartoonization aims to reduce visual complexity, it's crucial to retain essential features that convey the core identity of the subject. Overdoing the stylistic changes can lead to the loss of crucial details, compromising the recognition of the depicted subject.
High-contrast imagery poses a unique hurdle for current cartoonization methods. The algorithms often struggle to manage the sharp differences in brightness and color, resulting in unwanted artifacts like halo effects around edges. These artifacts compromise the smoothness and visual coherence of the resulting cartoon.
Generative Adversarial Networks (GANs), a popular approach in cartoonization, often face difficulties achieving consistency in output when multiple artistic styles are involved. Attempts at blending different styles can lead to unexpected and unwanted visual outcomes that might not meet user expectations. This can require extra processing steps for refinement to achieve the desired artistic blend.
Noise reduction is a crucial pre-processing step in cartoonization. Noise present in the input photos can significantly degrade the quality of the cartoon by impacting clarity and detail. Implementing effective noise reduction methods before stylization becomes essential for a high-quality result.
Allowing users to customize the stylistic elements adds another layer of complexity. Catering to individual tastes and preferences can lead to challenges when attempting to generalize across user inputs. Implementing one user's specific artistic preferences can inadvertently reduce the quality of the outcome for another due to conflicting artistic parameters used by the model.
Real-time cartoon generation demands significant processing power. Creating high-quality results on-the-fly can pose bottlenecks, especially when dealing with intricate images that need extensive pixel-level manipulation. This often leads to trade-offs between processing speed and visual quality.
GANs can sometimes exhibit a phenomenon called "mode collapse", where they produce very limited variations of cartoon styles. This highlights the need for diverse and rich datasets for training, which can encourage the model to produce more varied and imaginative cartoon outputs.
Evaluating the quality of AI-generated cartoons based on artistic merit can be tricky. There is still a lack of a widely accepted standard for assessing artistic quality, making it challenging for researchers to establish that their models are producing visually pleasing and satisfying results across diverse artistic tastes.
Recent advancements in neural architecture search are showing promise for improving cartoon generation models. However, a major challenge remains: creating models capable of effectively generalizing across various input types and artistic styles. This remains particularly challenging for unusual or less conventional cartoon styles.
Emerging research on stylized images hints that how we perceive and interpret art could be instrumental in influencing the training process for AI models. This suggests that traditional image quality metrics, which often focus on photorealism, may not be adequate for comprehensively capturing the nuanced aspects of artistic merit in cartoons. This leads us to think about the intersection of AI, image processing, and human perception of visual art, which are areas ripe for further exploration.
Understanding AI Cartoon Generation A Deep Dive into Image-to-Cartoon Conversion Quality Benchmarks - Cross Platform Performance Testing Results on Mobile and Desktop
The increasing complexity of AI-driven image manipulation, including cartoon generation and photo enhancement, necessitates a robust understanding of how these applications perform across different devices. This involves evaluating their capabilities on both mobile and desktop platforms. Performance metrics, often assessed using tools like Geekbench, help developers understand how the CPU and GPU of a device impact the final output. Factors like processing speed and image quality can vary drastically depending on the hardware, affecting the overall user experience. For example, the ability to quickly transform photos into high-quality cartoons could be severely limited on a mobile device with a less powerful processor.
Furthermore, testing across various platforms helps identify and rectify inconsistencies in application behavior, ensuring a seamless experience for users regardless of the device they are using. Tools that facilitate cross-platform testing, such as Xamarin Test Cloud, are essential for identifying potential issues early in the development cycle. Developers need to ensure that the features and performance of their AI applications are consistent across diverse devices to avoid frustrating users with slow processing times, lower-quality outputs, or crashes on specific platforms. While pushing the boundaries of AI image manipulation is exciting, it is critical to optimize for practical implementation. A thorough examination of cross-platform performance provides a path to deliver high-quality AI-powered experiences across the spectrum of user devices.
Cross-platform performance testing has revealed some interesting insights into AI cartoon generation. For instance, mobile devices sometimes outperform desktop computers in generating cartoons quickly, especially when algorithms are designed to leverage limited hardware efficiently. This challenges the common notion that desktops always offer better processing power.
User experiences differ greatly across mobile and desktop platforms. Mobile apps tend to prioritize simplicity and speed, often sacrificing some features present in their desktop counterparts. This highlights a constant trade-off between user accessibility and advanced functionalities.
The computational needs for AI cartoonization vary dramatically between mobile and desktop. Desktops can utilize a wider array of processing resources, often leading to higher-resolution cartoons with more detailed outputs.
Intriguingly, some benchmarking data suggests that while desktops usually excel in highly complex image processing, optimizations in mobile algorithms can result in comparable visual quality for users, prompting questions about the traditional device hierarchy in this domain.
Display variations between mobile and desktop devices can affect how users perceive cartoon quality. Features like color depth and detail can seem more apparent on larger, higher resolution desktop screens than smaller mobile displays.
Recently developed adaptive algorithms allow AI models to adapt their processes based on performance data gathered across various platforms. This dynamic approach leads to smarter rendering, regardless of whether the input image is processed on a mobile device or a desktop.
Performance benchmarks are moving away from simplistic speed measurements and towards more sophisticated evaluations, including user satisfaction scores. This more holistic approach takes into account aspects like perceived cartoon quality and the ease of use across mobile and desktop experiences.
Research indicates that while desktops have fewer performance bottlenecks, they sometimes face longer processing times when dealing with cartoons that incorporate multiple styles compared to mobile devices. These mobile devices might benefit from fine-tuned, application-specific algorithms that are tailored for particular tasks.
Memory management varies greatly between platforms. Mobile systems tend to employ aggressive caching techniques to ensure smooth cartoon generation, leading to quicker load times in some instances compared to more traditional desktop workflows.
Data compression techniques on mobile devices have significantly improved. These methods can reduce file sizes without sacrificing visual quality, resulting in quicker sharing times and less storage use. This has led some users to prefer the output from mobile-based cartoon generation because they can easily share the images without sacrificing a visually pleasing result.
Understanding AI Cartoon Generation A Deep Dive into Image-to-Cartoon Conversion Quality Benchmarks - Impact of Input Image Resolution on Final Cartoon Output
The quality of the final cartoon generated by AI is significantly influenced by the resolution of the input image. Higher resolution images, containing more detailed information, generally lead to more refined and visually appealing cartoon outputs. The algorithms can leverage this detail to more accurately capture subtle features and create more nuanced stylization effects. Conversely, if the original image has a low resolution, the AI might struggle to effectively extract the essential features, resulting in a cartoon that appears blurry or lacks detail, diminishing the overall aesthetic appeal.
However, it's not always practical to rely solely on high-resolution images as input. Many real-world scenarios involve working with images of lower quality. This necessitates that AI models possess the ability to adapt and generate satisfactory cartoon results from lower-resolution inputs. This requires developing sophisticated upscaling and deep learning techniques to bridge the gap between imperfect inputs and the desired high-quality cartoon output. The goal is to achieve a balance where the inherent artistic integrity of the original image is translated into a visually engaging cartoon, even when starting with images that have inherent limitations in their quality or detail. This is a crucial area of research that directly impacts the overall usability and effectiveness of AI-powered cartoon generation tools.
The quality of the input image, specifically its resolution, significantly impacts the final output of AI-driven cartoonization. Higher resolution images provide a wealth of detail, allowing the AI to capture subtle textures and colors more accurately. This results in cartoons that maintain a stronger resemblance to the original image, preserving key features and identity elements. Conversely, lower resolution images can lead to excessive simplification, potentially losing critical information and resulting in a less nuanced final cartoon.
When upscaling images before processing, we encounter interesting challenges. While upscaling can improve the overall appearance, simply increasing resolution without corresponding advancements in algorithm training can introduce artifacts like blurring or pixelation. This suggests that effective upscaling requires both resolution enhancement and a tailored training approach to minimize unintended consequences.
Processing very high-resolution images, like those at 4K, presents a trade-off. While detail and artistic quality improve dramatically, the computational demands increase significantly, making it less efficient for applications needing fast processing. This is a growing concern as real-time cartoon generation becomes increasingly popular, requiring careful calibration between image quality and processing speed.
Another aspect of resolution's influence relates to stylistic consistency. AI models trained on datasets with higher-resolution images generally excel at capturing the subtleties of artistic styles. This implies that the quality of the input data is just as important as the resolution itself in preserving the nuances of the desired artistic features.
There's a phenomenon we can call "resolution dependency" where certain aspects of cartoonization, such as edge definition and color smoothing, are dramatically impacted by the input resolution. This indicates that the AI model needs to be fine-tuned for specific resolution ranges to consistently produce high-quality results.
An intriguing approach involves using both high and low-resolution versions of the same image. By processing both together, the AI can benefit from the fine detail available in the higher resolution while also using a broader contextual approach provided by the lower resolution version. This dual-input strategy has shown potential for enhancing the overall output.
Facial features are particularly susceptible to the limitations of low-resolution input. The AI may misinterpret subtle details, leading to exaggerated or distorted caricatures rather than accurate representations. This can significantly impact user satisfaction, as an accurate portrayal is often a core expectation in cartoon generation.
Noise present in the input image can become a significant obstacle when the resolution is low. It negatively impacts the clarity and aesthetics of the final cartoon. Effective noise reduction techniques applied before the cartoonization process are essential to achieve high-quality results.
Some AI systems employ "super-resolution" techniques to artificially improve the resolution of the input image. This can improve the visual appearance, but it can also create inconsistencies in how details are depicted in the cartoon. This introduces a further layer of complexity in evaluating the final cartoon's quality.
Watermark removal techniques, like other AI-powered tasks, seem to be influenced by resolution. With higher resolution images, algorithms have access to more surrounding contextual information. This enables them to better reconstruct removed areas in a more believable way, resulting in better overall image integrity.
This exploration into the interplay of resolution and AI-powered cartoon generation suggests that future efforts should consider not just enhancing the quality of the input images, but also tailoring the model's training to handle a variety of resolutions and their related impact on the final output.
Experience error-free AI audio transcription that's faster and cheaper than human transcription and includes speaker recognition by default! (Get started for free)
More Posts from transcribethis.io: