The Rise of AI-Powered Video Editing A Deep Dive into VEEDIO's Capabilities
The Rise of AI-Powered Video Editing A Deep Dive into VEEDIO's Capabilities - AI's Impact on Video Editing Workflow
Artificial intelligence is rapidly altering the landscape of video editing, impacting workflows in significant ways. AI's capacity to automate repetitive tasks is streamlining the editing process, freeing up editors to focus on more creative aspects of their work. This automation leads to increased efficiency and, ultimately, faster turnaround times for projects.
Beyond speeding things up, AI can analyze video footage to identify crucial segments and moments. This ability to recognize key elements helps editors quickly generate initial edits, providing a strong foundation for further refinement. The ability to automate the creation of edits is a noteworthy development and represents a significant time-saver in production.
The introduction of generative AI capabilities adds another layer to the impact of AI on editing. Now, creators can personalize the style and feel of their edits using AI tools, adding an element of individual creative expression to the process. This can contribute to greater flexibility and potentially a new wave of stylistic video content.
As the integration of AI into video editing tools advances, it's clear that its role will only grow. The future likely holds even more innovative applications and opportunities for creativity within the video production landscape. It will be interesting to observe how these tools will evolve and continue to shape the world of video editing.
The integration of AI into the video editing landscape has brought about a notable shift in how workflows are managed. We're seeing a move towards automating routine tasks, such as color grading and audio synchronization, leading to potential time savings for editors. Some studies even suggest a reduction in editing time of up to 75%. This allows editors to dedicate more time to the creative aspects of their work, pushing boundaries rather than getting bogged down in technical minutiae.
Beyond automation, AI algorithms are being used to analyze the emotional content of footage, providing insights into the potential impact on audiences. This capability helps editors curate content that resonates better with viewers, potentially enhancing engagement rates. Further, AI can now automatically generate subtitles and translations for videos, which can help significantly expand a video's reach across different linguistic markets.
It's also intriguing how AI is influencing decision-making during the editing process. Some software now suggests edits based on established trends and viewer data. This function can help creators align their content with what's popular, albeit it also raises interesting questions about originality and creative control. Some AI tools can even create a kind of digital storyboard by analyzing the footage and scripts, giving editors a head-start on visualization before even delving into the bulk of editing.
Furthermore, AI excels at maintaining visual consistency within long projects by keeping track of visual details and ensuring stylistic coherence. This is crucial for complex productions that may span multiple edits or editing teams. Another fascinating development is the application of speech recognition technology. AI can isolate and tag specific phrases or key moments within the audio track, streamlining the retrieval of information during the editing process.
The role of machine learning is especially promising. AI can adapt to individual editing styles over time, observing preferences and suggesting edits that align with an editor's particular approach. However, the emergence of AI also creates challenges. As certain tasks become automated, it raises questions about the evolving nature of roles within video production teams and the necessary skill sets for future editors. While efficiency is increased through automation, there is also a concern that an overreliance on AI tools may lead to a homogenization of style and potentially diminish the 'human touch' that is crucial for impactful storytelling. The ongoing development of AI for video editing raises questions about the delicate balance between efficiency and creativity. It will be interesting to observe how these trends continue to shape the landscape in the years to come.
The Rise of AI-Powered Video Editing A Deep Dive into VEEDIO's Capabilities - VEEDIO's Automatic Scene Detection Technology
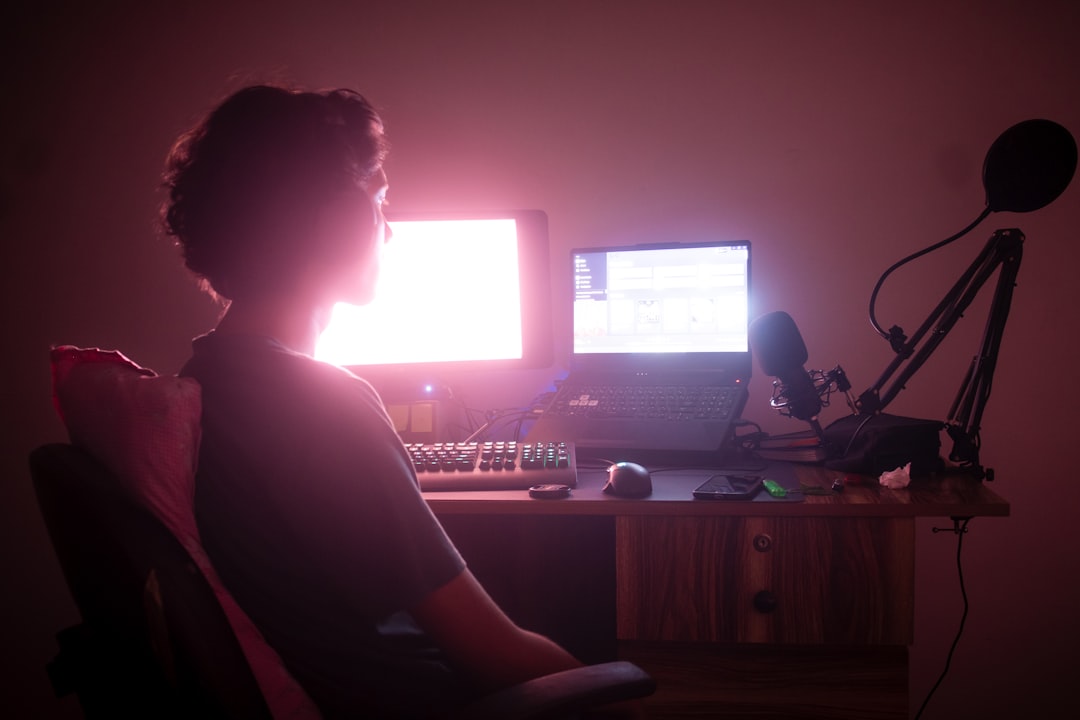
VEEDIO's Automatic Scene Detection is a feature built on AI algorithms that analyzes video frames to pinpoint where scenes change. It looks for things like cuts, fades, or dissolves, which indicate the start or end of a scene. This ability to automatically detect scene breaks is quite useful. It boosts how well we can understand and organize the video content itself. This kind of AI-driven scene recognition has the potential to revolutionize how we edit videos, making the organization of video footage much simpler. While this technology offers efficiency and speed, it also brings into question the importance of a human editor's creativity. As these AI-powered tools grow in sophistication, the challenge of ensuring that a unique, artistic viewpoint isn't lost in the midst of automation becomes a central issue. The ongoing integration of AI into video editing raises important questions about striking a balance between efficiency and maintaining a personal creative touch. How future video editors navigate this changing world, and find that balance, will be an interesting area to watch unfold.
VEEDIO's Automatic Scene Detection Technology leverages AI algorithms to analyze video frames, pinpointing scene transitions like cuts, fades, or dissolves, which define individual scenes. This capability significantly improves the analysis of video content, achieving a level of accuracy in interpreting visuals previously unseen. AI-powered scene recognition is reshaping video editing, streamlining the categorization and organization of footage.
It's interesting how VEEDIO's platform seamlessly integrates this technology. Their system allows users to generate professional-quality videos easily through a user-friendly interface, utilizing drag-and-drop functionalities. While their text-to-video feature, allowing video generation from textual input, shows the potential of AI, it also raises the question of the impact on the creative process. One can wonder whether it encourages a shift towards a more formulaic approach to content creation. Future innovations, like real-time editing for live events, promise to revolutionize the video editing landscape, although concerns regarding latency and bandwidth management are not to be discounted.
The scene detection technology goes beyond simply identifying breaks between segments. It also highlights key frames within each scene, facilitating the creation of quick visual storyboards. This helps in planning edits before embarking on the more detailed stages. The technology's capacity for adaptive learning is intriguing; it adapts to individual user preferences, constantly refining its capabilities based on feedback and edits. Furthermore, the multi-layered analysis, considering aspects like color grading and composition in tandem with transitions, allows for a more nuanced understanding of a scene's impact. This depth of analysis, along with the ability to integrate audio cues and dialogue, potentially bridges the gap between visual and audio narratives.
However, certain aspects warrant closer examination. VEEDIO generates metadata for each scene, providing insights into content and audience engagement, but the reliability of these insights and their potential for bias remains an open question. The system seamlessly integrates with other VEEDIO tools like audio synchronization and color grading, providing precise markers, which should expedite the editing process. But what level of manual control is available? It also supports a wide range of video formats, including 4K and VR, which demonstrates adaptability but also highlights the need for ongoing testing to ensure its effectiveness across diverse platforms.
The technology can draw upon historical data, applying insights from previous projects, presumably leading to refinements in its scene recognition. This learning approach can be helpful, although it also could lead to a homogenization of editing styles if not handled cautiously. The system offers the capacity for backup and recovery, allowing quick reverts to previous versions, which is beneficial in large-scale projects. But questions arise regarding the system's capacity to retain these edits long-term, particularly if significant changes are implemented over time. While these developments in automatic scene detection are undeniably impressive, it’s essential to also explore potential downsides. The reliance on automated systems might shift the burden from conceptualization to refinement, potentially creating a homogenization of content if not consciously addressed. Continued investigation is needed to fully understand the technology's capabilities and limitations in shaping the future of video editing.
The Rise of AI-Powered Video Editing A Deep Dive into VEEDIO's Capabilities - Natural Language Processing in Video Captioning
Natural Language Processing (NLP) is fundamental to the automated creation of video captions, essentially transforming visual content into written descriptions. AI systems, often using deep learning techniques like encoder-decoder networks, are trained to extract key visual information from videos and translate that information into natural language captions. This automated process offers a significant improvement over traditional methods, enabling real-time caption generation and boosting accuracy. The increasing importance of AI-driven video captioning tools underscores the broader trend towards greater accessibility and engagement across various video platforms. While this technology offers numerous benefits, it also presents concerns about the potential for standardization of style and the need for a careful balance between automation and human creativity within video editing workflows.
Natural Language Processing (NLP) plays a crucial role in automatically generating descriptive text from video content, going beyond simple transcription. It aims to capture the essence of a video by understanding context, including subtleties like sarcasm or emphasis, aspects that are often missed by traditional captioning methods. This deeper understanding allows for a more nuanced and engaging viewing experience.
NLP-powered video captioning relies heavily on deep learning, particularly encoder-decoder models. These architectures are designed to extract key visual features from the video and then translate them into natural language captions. While this process appears straightforward, it involves complex tasks like understanding the visual narrative and mapping it to a coherent textual description.
The process of video captioning, with NLP at its core, fundamentally involves understanding what is happening in a video and then translating that visual information into a textual format. This translation process requires sophisticated algorithms to interpret both the visual and auditory elements of the video to generate appropriate and informative captions. Deep learning approaches have significantly surpassed traditional methods in achieving this goal. They have enabled real-time captioning and generally improved the accuracy and quality of generated captions.
Video captioning powered by NLP provides numerous benefits across various domains. It is used for subtitling, making videos accessible to a wider audience. It's employed in video surveillance systems for automatic event logging and analysis. It even plays a role in enabling human-machine interaction, where visual content is translated into a language that machines can comprehend.
Online platforms such as YouTube, Twitch, and Instagram are increasingly emphasizing the importance of accurate video captions. These platforms recognize the benefits of captions for improving accessibility, particularly for viewers with hearing impairments. They also play a role in boosting engagement, as captions can provide valuable context and help retain viewers who might be watching in noisy environments. Several tools, including Adobe Express, now provide AI-powered captioning features, automating the transcription of audio and allowing users to refine the captions for accuracy.
Essentially, the task of video captioning can be viewed as a mapping problem. The challenge is to effectively connect visual features extracted from a video directly to corresponding textual descriptions. This endeavor highlights the inherent complexities of integrating computer vision and NLP seamlessly, as it requires both systems to work in harmony.
The evolution of AI-powered video captioning has transformed content creation and consumption. Videos are becoming more accessible to a wider audience, including those who are deaf or hard of hearing. This accessibility leads to improved audience engagement and understanding. It’s reshaping how content is experienced, pushing the boundaries of communication through video.
The blending of NLP and video generation represents a rapidly expanding area of AI research. Its potential applications span numerous fields, from education and entertainment to scientific research. This convergence is leading to the development of more sophisticated video-related technologies, continually refining how we both create and interact with video content. However, like all technologies, this rapid advancement does have potential downsides and unforeseen consequences. As this area continues to develop, it's important to consider both the benefits and the potential challenges that may arise from the integration of NLP into video generation and editing tools.
The Rise of AI-Powered Video Editing A Deep Dive into VEEDIO's Capabilities - Machine Learning for Content Optimization
Machine learning is increasingly vital for refining video content. By analyzing how viewers interact with videos, machine learning algorithms can offer insights that improve content quality and relevance. This data-driven approach helps creators understand what resonates most with their audience, leading to videos that are more engaging and retain viewers longer. However, relying too heavily on these automated processes can be problematic. There's a risk of creative homogenization, where videos begin to look too similar, and the distinctive creative touch that makes storytelling compelling can be lost. As machine learning capabilities advance, it becomes increasingly important to find a balance between the efficiency it provides and the need for authentic, individual expression in video content. Striking this balance is crucial for the future of video creation.
Machine learning is increasingly being used to refine content strategies by analyzing how viewers interact with videos on platforms. This real-time feedback loop allows creators to adjust their editing choices based on what resonates most with their audience, essentially blurring the lines between creating and optimizing content. It's fascinating how we can now make changes on the fly, guided by what viewers are responding to.
The capacity of machine learning to process vast quantities of video data allows for sophisticated predictive analysis. By examining past video performance, algorithms can anticipate trends and suggest topics likely to attract a larger audience. This ability to foresee what might work is quite a change for content planning, allowing creators to make more informed decisions. However, it also raises the question of whether this approach leads to more formulaic content or a new kind of creative expression that caters to predictive trends.
Machine learning also lets us study the effectiveness of various video styles. For instance, it can determine which color palettes or pacing resonates best with certain demographics, providing insights that help enhance the emotional impact of a video. This is a powerful way to better target audiences, but it also begs questions about how far we should go in tailoring our work for specific demographics. Is it possible to personalize content too much and end up sacrificing the broader appeal of a video?
NLP tools are useful for gauging viewer sentiment from comments and feedback. This kind of analysis goes beyond simple viewership counts and helps editors understand how their content is perceived emotionally, a significant advancement compared to traditional feedback methods. It's intriguing how well NLP can capture the nuanced ways in which people respond to a video, but the reliability and accuracy of these analyses should be carefully scrutinized. It also poses the question of what level of bias might be built into NLP systems, and how this could influence creative decisions.
ML can automate A/B testing on video edits by analyzing audience responses to different versions in real-time. This accelerates the process of feedback and allows creators to quickly determine which edits are most effective, potentially shortening the iterative editing process significantly. While this is clearly a useful time-saver, it's important to be mindful of the potential for it to reduce experimentation and potentially lead to a 'lowest-common-denominator' approach to content. It's always a balancing act to optimize for engagement without compromising the originality and boldness of the creative vision.
With machine learning, we can now delve into the emotional response of viewers, gauging their level of engagement at different points in a video based on both visual and auditory cues. This deeper understanding of viewer experiences is a shift from simply looking at basic viewership statistics. The capability to connect with audiences on an emotional level is interesting, but raises questions about how this data might be misinterpreted or used in potentially manipulative ways. There's also a need to find ways to ensure the data is ethical and transparent, avoiding issues of privacy and misuse.
Machine learning algorithms can identify patterns in viewing habits across multiple platforms, allowing creators to personalize their content for different audiences based on their overall viewing history. This cross-platform approach creates the potential for finely-tuned audience engagement, but it also necessitates consideration for issues of privacy and data ownership. It's also an area where there's a lot of potential for both benefit and misuse. It's imperative to consider these larger issues along with the obvious advantages of tailoring content more specifically for individual users.
Machine learning is also useful in helping detect potential copyright violations within video content, offering a chance to identify possible issues before publication. This can protect content creators from legal issues while simplifying compliance procedures. However, the efficacy and accuracy of these AI-driven copyright detection tools need careful evaluation. The potential for misidentification or false positives is an important concern, as it could have serious consequences for creators.
AI systems capable of continual learning can adapt to an editor's preferred style, fostering a more personalized editing experience. Over time, these systems become better at suggesting edits that match an editor's particular approach. This dynamic learning capacity is an exciting feature, but we must also understand the long-term consequences. Is it possible that these AI-driven suggestions could stifle creativity and lead to a more homogenized style of video editing?
The increasing use of machine learning for content optimization raises vital questions about originality and the role of creativity. While these tools are certainly powerful, there's a danger that creators might be steered towards producing more formulaic content, challenging the very notion of artistic expression in a digital media landscape. It's vital that we develop a better understanding of how these tools can be used thoughtfully and responsibly, to ensure the benefits of AI are enjoyed without compromising the unique perspectives and expressions that are integral to creativity.
The Rise of AI-Powered Video Editing A Deep Dive into VEEDIO's Capabilities - Real-time Rendering and Effects Application
Real-time rendering, a technology that's significantly improved by faster processors and advanced software, is changing how video effects are created. This allows editors to see their changes immediately, which is vital for a quick workflow in video production. AI has become increasingly important, enhancing visual effects with realistic compositing and streamlining processes that were previously very time-consuming. Neural rendering, an AI-based approach, is helping to produce remarkably realistic graphics, but also raises questions about how much creative control artists will have when AI takes on more of the workload. Real-time rendering's use is spreading quickly, and the question of how to balance efficiency with a unique, personal creative touch is becoming more important.
Real-time rendering has progressed significantly thanks to advancements in processing power and software, particularly GPUs capable of handling millions of calculations per second. This allows for immediate visual feedback during the editing process, eliminating the need for lengthy rendering delays. This ability to see effects instantaneously changes how we edit and allows for more fluid creative iterations, similar to the way architects can see designs evolve in real-time.
However, this speed often comes at the cost of visual quality. Editors must weigh the convenience of instant results against potential loss of detail, especially when using intricate effects that might benefit from offline rendering for optimal quality. Finding that sweet spot between speed and fidelity is a constant challenge.
To manage the demands of real-time rendering, various techniques are employed. Managing spatial resolution using mipmapping and level-of-detail rendering allows textures to be displayed efficiently. For smooth visuals, motion blur can be approximated, conserving processing power while maintaining a sense of motion.
Software often utilizes standardized protocols like OpenGL or DirectX for cross-platform compatibility. This enables real-time rendering engines to function seamlessly across different hardware configurations, making them more accessible to creators.
Some cutting-edge real-time rendering leverages neural networks to predict and apply lighting based on the scene's geometry. This clever approach significantly reduces computing load, producing effects that would typically require intense calculations. It is fascinating how we can use AI to improve aspects of the rendering process.
Interestingly, real-time feedback affects how viewers engage with the content. Studies indicate that instant visual feedback significantly influences viewers' perception and emotional responses to a video. The immediate nature of real-time effects can potentially heighten audience engagement, making it a tool that has a psychological dimension.
Virtual reality applications, which need smooth visual experiences at 90 frames per second to avoid making users feel ill, are heavily dependent on real-time rendering. This highlights the need for optimized techniques that ensure seamless visuals and prevents motion sickness, a serious design concern.
Real-time rendering engines can also adapt dynamically to performance changes. They adjust settings based on the current state of the system, ensuring that editors have a consistent experience even with scenes that become increasingly complex—a valuable feature in complex productions.
The algorithmic foundation of real-time rendering isn't limited to video editing. These techniques are also utilized in fields like architecture, automotive design, and game development, demonstrating the technology's broader applicability across different areas. This multi-disciplinary nature of the underlying techniques helps us see how advancements in one field can create opportunities in others.
Looking ahead, real-time rendering is likely to play a major role in interactive video content. We may see narratives where viewers' choices directly influence how a video unfolds, altering the traditional approach to editing and potentially redefining how stories are told. This new form of storytelling holds a great deal of promise and will undoubtedly lead us to re-examine how video content is produced and consumed.
The Rise of AI-Powered Video Editing A Deep Dive into VEEDIO's Capabilities - Ethical Considerations in AI-Assisted Editing
As AI integration into video editing becomes more sophisticated, ethical considerations are paramount. The ability to generate realistic yet potentially deceptive content, known as deepfakes, poses a significant threat to truth and individual identity. This capability necessitates thoughtful discussions around responsible AI development and the implementation of safeguards against the misuse of these technologies for malicious purposes like misinformation or identity theft.
Another crucial concern lies in the potential for AI algorithms to amplify existing societal biases. The data used to train these systems can contain inherent biases that are then reflected in the AI's outputs, potentially leading to unfair or discriminatory outcomes in video editing decisions. Ensuring that AI systems are trained on diverse and representative datasets is essential to mitigate this risk and promote inclusivity and fairness.
Furthermore, the growing role of generative AI in video editing raises questions about originality and artistic control. While these technologies can unlock new levels of creativity, they also introduce the risk of creating a more homogenous style of video content. It's essential to carefully consider the balance between automated efficiency and the preservation of unique artistic expressions. Striking this balance will be key to avoiding the potential for a decline in the distinct, human element that makes compelling narratives. The evolving landscape of AI-powered video editing thus necessitates careful consideration of these ethical implications, prioritizing both technological advancement and responsible innovation.
The increasing integration of AI into video editing brings about a range of ethical considerations that we must carefully examine. One of the key concerns is the possibility of AI systems inadvertently perpetuating biases embedded within the data they're trained on. This can result in the unintentional reinforcement of stereotypes or the exclusion of specific groups within the content, raising concerns about fairness and equitable representation in video editing.
Another challenge is navigating the complex world of intellectual property when AI generates or modifies video content. The use of existing media for training AI models can lead to questions about copyright ownership and potential infringements, creating a need for clearer legal frameworks regarding AI-generated content. The capabilities of AI also raise the issue of content manipulation, where aspects of videos can be altered, including expressions and context, potentially without the knowledge of the viewers. This is particularly concerning in contexts where accurate and trustworthy information is paramount, such as news and politics.
The growing reliance on AI-powered editing tools poses a potential risk of diminishing the uniquely human aspects of storytelling. Editors might lose some of the nuanced perspective and creative choices that contribute to compelling narratives, potentially leading to a more uniform or homogenized style of video content. Furthermore, the use of advanced machine learning in AI often requires substantial data collection from users, raising important privacy concerns. It becomes critical to implement ethical data management strategies to ensure the responsible use of this data and to safeguard user privacy.
One aspect that requires careful consideration is the transparency of AI's decision-making process. Many AI systems operate as what are known as "black boxes", where the rationale behind their recommendations or outputs isn't easily understood. This can create difficulties for editors when attempting to justify content decisions or analyze why specific suggestions are made. The ongoing shift toward AI-powered editing, while enhancing efficiency, could also lead to changes in the employment landscape within the video editing industry. The ethical consequences of potential job displacement need careful examination and potentially a thoughtful approach to workforce adaptation.
The ability to manipulate video footage through AI gives creators unprecedented power to shape narratives, which raises questions about the reliability and trustworthiness of presented information. As AI evolves, current regulations may struggle to keep pace, leading to ambiguity regarding ethical guidelines for content creators and platforms. Another area of concern lies in the capability of AI to analyze viewer data and optimize content for emotional responses. This can lead to increased viewer engagement, but it also has the potential to be misused, for example, by exploiting viewer emotions for commercial gain without appropriate transparency. These are just some of the significant ethical challenges that we are facing with the growing use of AI in video editing. They represent a significant set of challenges that we must actively address to ensure that this powerful technology is used responsibly and ethically. It will require careful and continuous scrutiny as the field progresses.
More Posts from transcribethis.io: