Experience error-free AI audio transcription that's faster and cheaper than human transcription and includes speaker recognition by default! (Get started for free)
The Evolution of Video-to-Audio Conversion Trends and Technologies in 2024
The Evolution of Video-to-Audio Conversion Trends and Technologies in 2024 - AI-Driven Enhancements in Video-to-Audio Conversion
AI is injecting new life into video-to-audio conversion, moving beyond simple extraction to the realm of intelligent audio generation. We're seeing a shift where AI can interpret video and create matching audio based on text prompts, delivering more realistic and synchronized soundtracks. This growing accessibility of generative AI, fueled by advancements in multimodal and small language models, is opening the door for a broader range of users to experiment with these capabilities. While challenges like limited GPU resources and high cloud computing costs persist, the push towards optimized models and efficient data management is ensuring that these advancements remain practically feasible. The net result is a significant leap forward in the quality and flexibility of tools for extracting and creating audio from video, promising a richer and more adaptable future for audio-visual content.
The integration of AI is revolutionizing video-to-audio (V2A) conversion by enabling more nuanced and sophisticated audio experiences. AI models can now interpret the emotional content of videos, tailoring the generated audio to match the visual narrative through dynamically adjusting background music and sound effects. This capability is pushing the boundaries of audio synchronization with visual storytelling.
Significant breakthroughs in deep learning have led to remarkably accurate voice synthesis. AI algorithms can now recreate a speaker's voice with a high degree of fidelity using only a limited number of voice samples. This development has wide implications, particularly in applications where authenticity of voice is crucial. Additionally, techniques like waveform synthesis have significantly improved the quality of audio produced from video sources, making it possible to generate audio that closely mirrors the original audio, even for older videos with poor audio tracks.
AI-driven V2A systems are also surpassing traditional speech-to-text tools in terms of accuracy. They can now transcribe audio from video in real-time by incorporating visual cues and context into their analysis. This contextual understanding enables them to differentiate between words that may sound similar, increasing the overall accuracy of transcription. Further, the flexibility of AI-generated audio allows for easy adaptation to different platforms. Systems can automatically adjust audio levels and formats to ensure optimal playback across a wide range of devices and services.
Moreover, the ability of AI to isolate and refine specific audio components within a video is a notable advancement. This feature facilitates improved audio quality by selectively enhancing dialogue or sound effects, without requiring intensive manual editing. AI is further being implemented to dynamically optimize audio levels during the conversion process. By identifying when a speaker is emphasizing a point, the system can intelligently adjust audio levels to ensure that the emphasis is retained in the final audio output.
This trend toward more sophisticated audio manipulation extends to emotion recognition as well. AI systems can interpret the emotions of the individuals in a video and use this information to generate a soundtrack that reflects the perceived emotional context of the scene. The ability to analyze user data, like viewing habits and engagement patterns, also presents exciting possibilities for optimizing the V2A conversion experience. AI can leverage this data to recommend audio enhancements that improve listener retention and satisfaction. Finally, ongoing development of machine learning-driven quality assessment tools allows developers to systematically measure audio quality based on various parameters. The feedback generated by these tools provides valuable information for improving audio fidelity and the overall user experience within V2A systems, showcasing a continual pursuit of enhancing the field.
The continued development of these AI-driven techniques will likely contribute to a more immersive and personalized video experience, ultimately shaping the future of V2A conversion. However, challenges like resource constraints (such as GPU limitations and the cost of cloud-based computing) continue to impact the pace of advancements in the field, requiring developers to focus on efficient model design and data optimization.
The Evolution of Video-to-Audio Conversion Trends and Technologies in 2024 - Market Growth and Subscription Models in AV Industry
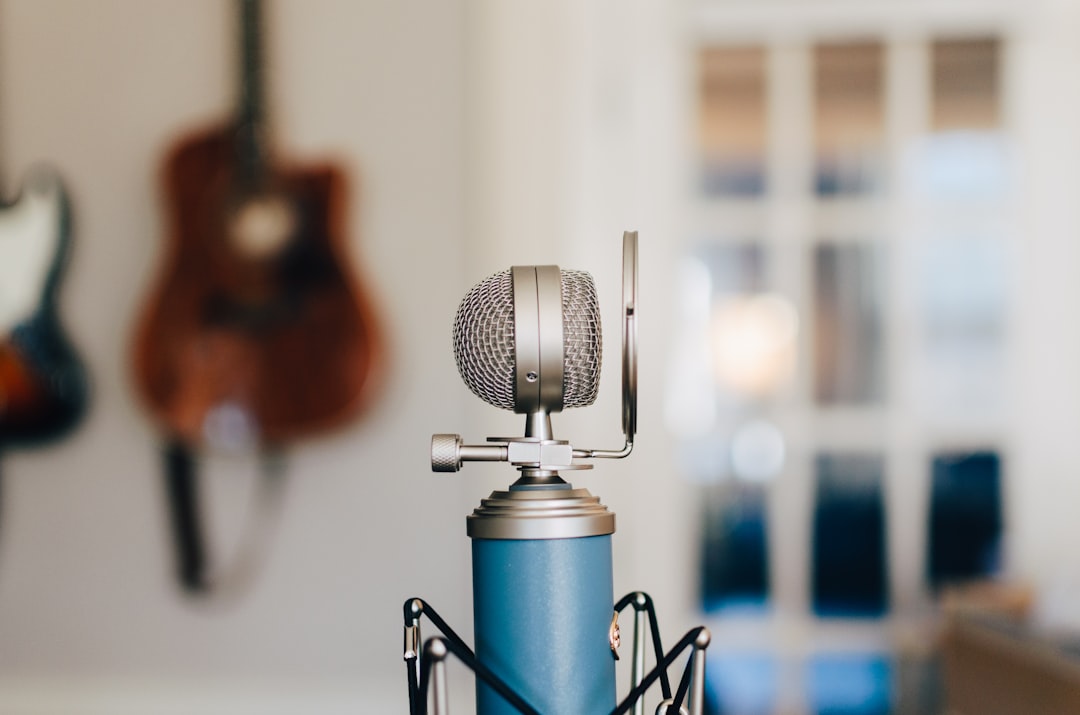
The audiovisual (AV) industry is experiencing a period of substantial growth, fueled by increasing demand and the rise of subscription-based models. Projections indicate the market could reach a massive $660.2 billion by 2029, propelled by a 24.45% compound annual growth rate (CAGR). This expansion is largely attributed to the need for flexible and scalable AV solutions, coupled with the growing acceptance of subscription models like AV as a Service (AVaaS). AVaaS, in particular, offers companies a way to access advanced AV technology without large initial investments, making it an attractive option for many in today's competitive landscape. While the industry is generally experiencing robust growth, the commercial segment has shown signs of slowing down recently. This suggests a shift in market dynamics that necessitates businesses to adjust their strategies and approach to remain competitive. The need to be adaptable and innovative within the evolving AV industry is more prominent than ever before.
The audiovisual (AV) industry is experiencing substantial growth, with projections indicating a market value of over $660 billion by 2029. This expansion is being driven by a surge in demand for flexible solutions, particularly subscription-based models. While some market segments, like professional AV systems, show a more modest growth rate of around 4-5% annually, other analyses paint a more optimistic picture, with estimates projecting a doubling of the market value within a decade, reaching as high as $99 billion by 2031. It's important to note that these projections are varied, highlighting the uncertainty inherent in forecasting future market conditions.
Reports indicate that revenue in the professional AV market is anticipated to climb nearly $100 billion over the next several years, potentially reaching $402 billion by 2028. This rapid growth is influenced by several factors, including advancements in audio and visual display technologies. However, there are signs of market fluctuations. The commercial AV sector, for example, displayed slower growth in 2023, suggesting potential headwinds. These shifts may be connected to the broader economic environment and the evolving needs of businesses and consumers.
The rise of "AV as a Service" (AVaaS) reflects the increasing desire for cost-effective and flexible access to AV technologies. This trend signifies a shift in how organizations procure and utilize AV equipment, moving away from large capital expenditures towards more adaptable and potentially more budget-friendly solutions.
Geopolitical events, such as the COVID-19 pandemic and its aftermath, have also played a role in the evolution of the AV landscape. In Europe, the industry has seen transformations in response to the pandemic's impact, emphasizing the importance of business agility and adaptability to changing circumstances. Ultimately, technological advancements fostering immersive experiences and seamless collaboration across diverse sectors are the core drivers behind the AV market's growth. However, it's crucial to remain aware of economic and geopolitical events that can impact these trends.
There are indications that the sector is in a dynamic phase, with growth rates fluctuating and market segments showing varying degrees of expansion. It will be interesting to see how the industry responds to these evolving conditions and whether the projected growth rates ultimately materialize.
The Evolution of Video-to-Audio Conversion Trends and Technologies in 2024 - Active Noise Cancellation Advances in Audio Extraction
Active noise cancellation (ANC) has seen considerable advancements, particularly in its role for extracting audio from video. This improvement is largely due to deep learning techniques that are now being applied to predict and remove noise, improving the resulting audio's quality. These advances encompass the creation of lightweight ANC systems for a range of earphone types, including earbuds and clip-on models. This miniaturization allows for more practical, real-time processing capabilities, leading to a better user experience, especially in environments with substantial background noise. Furthermore, the field is moving towards more sophisticated algorithms that distinguish between the wanted audio and the unwanted background noise, effectively improving speech clarity and the overall quality of sound extracted from videos. This refinement of ANC highlights its value not just in consumer-facing technologies like earphones, but also across a wider array of sectors like telecommunications and the production of hearing aids. While there's progress, it's worth acknowledging that the ultimate effectiveness of these ANC approaches depends heavily on the complexity of the noise environment and the nature of the desired sound. Ongoing research and development are likely to yield further enhancements, potentially leading to even more seamless and robust noise reduction in diverse audio extraction scenarios.
Active noise cancellation (ANC) has moved beyond simply using sound-absorbing materials. It now involves actively processing audio signals to identify and remove unwanted noise. Recent improvements leverage deep learning to predict not just the intensity, but also the phase of noise, making it much better at eliminating noise. This is particularly impressive when dealing with complicated, overlapping sounds.
This technology has made its way into various earphone designs, from the familiar in-ear and earbuds to clip-on styles. Each of these types uses built-in ANC features to evaluate performance. Even the automotive industry has adopted ANC. Hyundai's implementation of a road noise ANC system for electric vehicles, starting in 2018, was driven by the need to address the quieter engine noise common in EVs. We've seen steady advancements in active headsets, a key market for ANC technology, since the early days of the technology (patented in the 1950s). One interesting example is the use of complex computational models, such as a 10-layer dilated convolutional neural network on a field programmable gate array, to implement real-time ANC systems for in-ear headphones.
The progress in ANC is intrinsically connected to improvements in digital signal processing. This field has seen continuous development for the past 75 years and has provided a solid foundation for many of the algorithms that underpin ANC systems. These algorithms are continually refined to increase the signal-to-noise ratio, leading to better user experiences across diverse applications like telecommunications and hearing aids. The impact of ANC extends to various industries, tackling the problem of high background noise in various environments. This has clear benefits for applications like speech recognition, enhancing intelligibility and clarity.
Researchers have also integrated elements of soft computing and more sophisticated signal processing techniques to improve ANC systems. These approaches help fine-tune how the technology handles various noise profiles, further enhancing its performance. However, the desire for real-time processing can introduce delays, which can be problematic for users in areas like gaming or live performance where exact timing is critical. Current ANC research also focuses on creating more individualized listening experiences. Using machine learning, ANC can adapt to user preferences, tailoring audio to diverse contexts. ANC systems can now be used alongside other sensory information, such as visual data, to extract audio more accurately. This multimodal approach enables ANC to respond dynamically to changes in the environment and user interactions.
We've also seen breakthroughs in echo cancellation technologies. This reduces the impact of background conversations or noise during recordings, which is incredibly valuable in noisy urban areas or busy workplaces. Miniaturizing ANC for devices like smartphones has, however, posed new challenges, especially regarding battery life and managing heat. These are crucial considerations for mobile devices that need to remain compact. It's notable that the development of standardized testing methods for ANC is an ongoing endeavor, making it easier to benchmark the performance of different ANC solutions. Incorporating real-time user feedback into ANC systems represents a positive step towards creating ANC solutions that better meet the needs and preferences of users. Ultimately, these continuous improvements aim to provide more refined noise cancellation that seamlessly adapts to different contexts and listening experiences.
The Evolution of Video-to-Audio Conversion Trends and Technologies in 2024 - Rise of Video-to-Audio Conversion as a Service
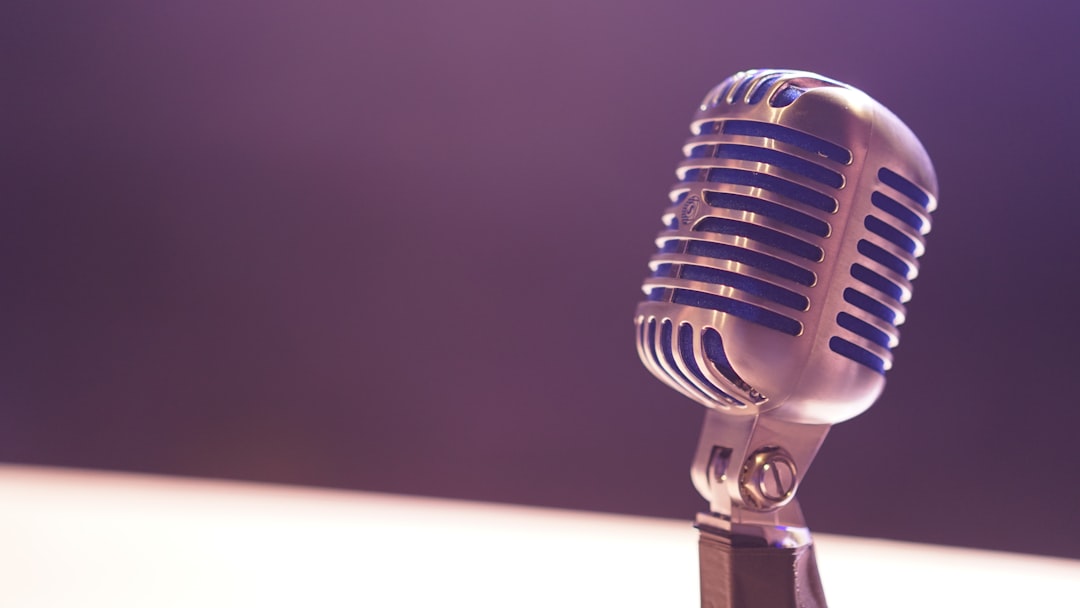
The increasing prevalence of video-to-audio conversion offered as a service signifies a shift in how we interact with audiovisual content. The demand for swift and efficient video-to-audio transformations is fueled by the constant advancements in AI and the growing need for convenient media access. This trend is further supported by the expanding use of subscription models within the AV industry. These models provide businesses with the opportunity to utilize cutting-edge AV tools without substantial upfront capital expenses. However, the rapid expansion of these services also brings forth complexities. Maintaining consistent audio quality across diverse video formats and effectively managing vast quantities of data are key challenges. The industry faces a balancing act between accelerating growth and ensuring the reliability and quality of the technology. Going forward, service providers must address these challenges to stay competitive and cater to the ever-evolving expectations of users, ultimately shaping the future of audio extraction and manipulation.
The landscape of video-to-audio conversion is rapidly evolving, fueled by a growing market projected to reach over 9.4 billion dollars by 2026. This growth is driven by the increasing demand for audio content across various platforms, like podcasts and audiobooks. It's fascinating how quickly machine learning is impacting this field. We're seeing the emergence of real-time audio translation services, catering to multilingual audiences and making video content much more accessible globally.
Furthermore, recent advancements have enabled the reconstruction of audio from videos that previously lacked complete or even partially intact audio data – a truly remarkable development in digital media processing. The accuracy of voice cloning, a feature incorporated into many video-to-audio conversion services, is reaching astonishing levels. These services can now synthesize voices that incredibly closely mimic not just the pitch and tone but also the unique nuances of a specific speaker. This level of realism raises important ethical and copyright concerns, something researchers will need to keep an eye on.
Despite the impressive progress, the performance of AI-driven audio extraction systems isn't always perfect. The quality of the original video, especially the resolution and compression, can significantly influence the fidelity of the converted audio. Low-resolution or heavily compressed video files often result in a notable drop in the quality of the audio output after conversion. This suggests that there's still room for improvement in the robustness and adaptability of the algorithms.
We're also witnessing the rise of a new generation of startups focusing on converting specialized video content into high-quality audio, such as educational videos. This trend aligns with the wider shift towards remote learning and the need for on-the-go education. Even media companies are now strategically employing video-to-audio conversion for purposes beyond just entertainment or education. They're recognizing the potential SEO benefits of audio versions of their content, as audio can enhance search engine rankings and improve discoverability.
One of the most unexpected developments is the speed with which video-to-audio conversion is becoming embedded into mobile applications. Some surprisingly user-friendly apps allow individuals with minimal technical experience to conduct complex audio editing tasks on their smartphones in a matter of minutes. It's a sign that this powerful technology is quickly becoming democratized.
The next generation of video-to-audio algorithms are now incorporating natural language processing (NLP) techniques. These methods enable a better understanding of situational context within a video, which can be crucial in determining the emotional tone of the generated audio. It's a step towards ensuring that the generated audio is more naturally aligned with the video content.
As the video-to-audio service ecosystem expands, we are also seeing the emergence of concerns regarding potential misuse and piracy of synthetic audio content. To combat these threats, developers are increasingly embedding watermarking technologies into the generated audio to help prevent the unauthorized distribution of audio created through these services. The coming years are likely to see even more developments in this arena, raising both exciting and potentially troublesome new challenges for creators and users alike.
The Evolution of Video-to-Audio Conversion Trends and Technologies in 2024 - Next-Generation Codecs for Efficient Audio Extraction
The pursuit of efficient audio extraction from video is driving the development of next-generation audio codecs. These new codecs tackle the growing need for high-quality and immersive audio, particularly within the ever-expanding realm of streaming and broadcast media. Codecs like AuroCX, designed with streaming in mind, are showcasing impressive improvements in scalability and efficiency, surpassing some limitations of existing formats. Similarly, projects like Google's SoundStream, which utilizes neural networks for audio compression, demonstrate an increasing reliance on AI-driven approaches to enhance the entire process.
Meanwhile, formats like MPEGH 3D Audio and Dolby AC4 are specifically geared towards providing a richer listening experience in broadcast environments, highlighting a broader shift away from codecs traditionally used for cinematic purposes. The synergy between these new audio codecs and the expanding use of AI for audio extraction further underscores a key trend: the ability to dynamically adjust and enhance audio quality in real-time is becoming more prevalent, even when dealing with noisy or otherwise less-than-ideal recording conditions. This trend is not just about improving the everyday listening experience but is crucial for various industries requiring high-fidelity and precise audio capture and manipulation. The continuing development of these next-generation codecs is central to optimizing bandwidth utilization and ensuring high audio fidelity across various platforms, revealing that innovation in codec development remains a critical area of focus.
The field of audio extraction is seeing a surge in innovation with the development of next-generation codecs. These codecs are increasingly leveraging machine learning techniques to intelligently adapt to the nuances of video content. For instance, they can now better detect and respond to variations like a speaker's emphasis or shifts in background noise, providing a more refined audio output.
We're also observing a significant jump in compression efficiency with newer codecs like AAC and Opus. They're capable of achieving compression rates that are up to 50% better than older formats such as MP3. This is especially beneficial in situations where bandwidth is limited, like when streaming over mobile networks, resulting in a noticeable improvement in audio quality.
Many of these modern codecs are moving towards support for multichannel audio formats. This can deliver a more immersive soundscape for viewers by accurately recreating spatial audio, a development that holds potential for both the film and gaming industries.
What's particularly interesting is the growing ability of advanced codecs to adapt in real-time. They're becoming more responsive to changing conditions, such as fluctuations in network speeds or constraints on a user's device, and adjust their coding strategies accordingly. This adaptive behavior is a notable advantage, maintaining audio fidelity without the need for manual intervention.
Another interesting facet is the increased use of perceptual coding. These codecs leverage our human hearing limitations, effectively removing frequencies that are typically inaudible to us. This allows for more efficient packing of audio data, resulting in smaller file sizes without sacrificing the perceived sound quality.
HEVC, a codec already playing a significant role in video compression, is being complemented by advancements in Versatile Video Coding (VVC). VVC holds the promise of even greater efficiency, delivering higher audio quality at lower bit rates. If this potential is realized, it could significantly alter how we distribute audio and video content online.
The applications for advanced codecs are expanding beyond simple audio extraction. Some of the latest techniques can even translate the spoken language in videos into different languages in real-time. This exciting capability has implications for global accessibility, allowing people to enjoy content regardless of the language it was originally created in.
We're also witnessing the development of codecs specifically designed for augmented and virtual reality applications. These are critical for experiences where low latency and high audio precision are crucial to maintain the alignment between visual and auditory cues. This type of optimization is essential to prevent users from feeling detached or disoriented during immersive VR or AR experiences.
The emergence of machine learning-enhanced codecs has led to a rise in adaptive bitrate streaming. With this technology, the audio quality during playback dynamically adjusts based on a user's current network conditions, ensuring a consistent and high-quality audio experience even when network connectivity is unstable.
Finally, the competition among codec developers is pushing the boundaries further. Some are striving to achieve what they call 'lossless compression'. Essentially, this means that the audio extracted from a video source can be perfectly reconstructed, opening up new possibilities for archival and restoration technologies. It's a fascinating development with the potential to revolutionize how we preserve and restore audio-visual content for future generations. The ongoing advancements in codecs are continuously transforming the audio extraction landscape, improving the quality of the audio we experience from video content and revealing new applications for this rapidly evolving technology.
The Evolution of Video-to-Audio Conversion Trends and Technologies in 2024 - Integration of Audio Analytics in Conversion Processes
The integration of audio analytics into video-to-audio conversion processes is a significant trend emerging in 2024. It signifies a move beyond simple audio extraction to a more nuanced approach that leverages the information embedded within the audio itself. This allows conversion systems to gain a deeper understanding of the audio content, which in turn can be used to refine the quality and purpose of the output audio. For example, audio analytics can help optimize the fidelity of converted audio, tailor it to match audience preferences, and even use emotion recognition to ensure a better alignment with the visual narrative in the source video.
While the integration of these capabilities holds a great deal of promise, there are challenges to be addressed. Handling the wide variety of audio formats and the vast amounts of audio data involved in large-scale conversions remain obstacles. Effective algorithms and robust data management systems are needed to ensure the smooth implementation of audio analytics across the field. As the technology progresses, we can anticipate an increasingly important role for audio analytics in enriching the user experience and creating a stronger connection between video content and its audio counterpart. It seems likely that this area will be one of growing importance for audio-visual content moving forward.
The integration of audio analytics into video-to-audio conversion processes is revealing exciting possibilities for enhancing user experience and content creation. Research suggests that tailoring audio experiences based on individual preferences can substantially boost listener engagement, with some studies showing retention rates increasing by as much as 70%. This highlights the powerful impact sound design can have on our psychological response to content.
Beyond simple personalization, the ability to analyze the emotional tone of speech within videos is becoming increasingly crucial. Audio analytics can now dissect dialogue and match audio characteristics to the mood of a scene, ensuring the generated audio enhances the storytelling experience. It's fascinating how this technology can refine the narrative impact of dialogue, moving beyond basic voice reproduction.
This same technology can also be leveraged to improve accessibility for individuals with hearing impairments. By using real-time audio transcription and adaptation, tailored listening experiences can be generated based on user feedback. This opens up the potential to personalize the audio experience for those with varying hearing capabilities, fostering inclusivity in video content.
Furthermore, the data generated through audio analytics can be used for predictive modeling, giving content creators valuable insight into audience preferences. Analyzing historical listening patterns can allow businesses to predict future trends and better align content creation with audience interest, offering the potential to improve content efficacy and engagement.
Interestingly, this capability can extend to identifying signs of 'audio fatigue'—a phenomenon where audience engagement drops due to prolonged exposure to similar audio profiles. By monitoring listener interaction and engagement metrics, audio analytics can adjust audio elements in real-time, keeping users engaged and retaining attention. This suggests a future where audio landscapes are dynamic, automatically adapting to the listener's shifting preferences.
Moving beyond emotion recognition, voice synthesis is evolving to capture the nuances of context within a conversation. This capacity for contextual understanding allows for the creation of audio tracks that possess greater emotional depth, particularly in formats like audiobooks and podcasts where narrative and character development are central.
Algorithms are getting better at not just isolating specific sounds but also understanding the importance of those sounds within the context of the video. This allows for audio enhancement where essential components, such as dialogue, are dynamically elevated above background noise. This is especially valuable for genres heavily reliant on a strong narrative where clear audio is fundamental to understanding the story.
The integration of real-time audio analysis within video conversion pipelines leads to dynamic adaptive audio systems. These systems react to shifts in the emotional content of a video and make corresponding adjustments to the audio, transforming static audio into interactive, engaging experiences.
Studies show a strong positive correlation between users' satisfaction and audio conversion processes incorporating real-time analytics feedback loops. This ability to dynamically adjust audio based on content or audience response enables a continuous optimization of the audio experience.
The ongoing development of machine learning algorithms in audio analytics has reached a point where algorithms can automatically suggest audio enhancements based on historical user interactions. This could significantly impact content production workflows, potentially reducing creative bottlenecks and allowing creators to focus on the bigger picture. While the potential is exciting, it is worth noting this approach may still be in its nascent stages and will require significant development before it has widespread, seamless implementation.
Experience error-free AI audio transcription that's faster and cheaper than human transcription and includes speaker recognition by default! (Get started for free)
More Posts from transcribethis.io: