The Evolution of Video Subtitle Translation From Manual to AI-Driven Processes in 2024
The Evolution of Video Subtitle Translation From Manual to AI-Driven Processes in 2024 - Manual Transcription The Early Days of Video Subtitling
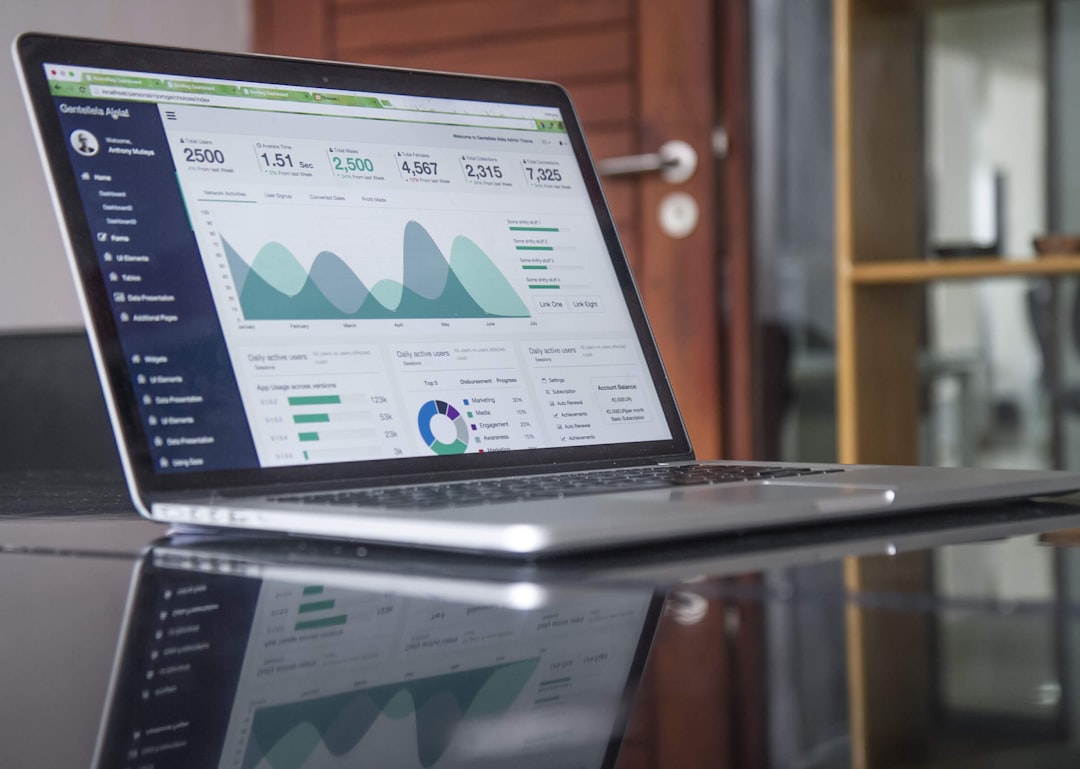
The birth of video subtitling was marked by the laborious process of manual transcription. Early films relied on simple intertitles, which were essentially text cards inserted between scenes to advance the story. This rudimentary method, though effective for its time, quickly proved inadequate as the demand for multilingual accessibility grew. Translators would spend countless hours painstakingly typing out subtitles, a task that demanded a high level of skill and precision. While tedious and time-consuming, this manual approach laid the groundwork for the complex and sophisticated subtitling systems we see today. This era of manual transcription, though seemingly primitive in retrospect, established the foundation upon which the modern, AI-powered subtitling revolution was built.
The manual transcription of subtitles began in the late 1920s, mirroring the rise of "talkies." Filmmakers realized the importance of making dialogue accessible to viewers who spoke different languages. The early methods went beyond simply typing what was spoken; they required a meticulous synchronization of text with the audio. This led to the creation of intricate timing codes, which laid the foundation for modern subtitling technology.
The process relied heavily on human transcribers, who were expected to have a strong understanding of the content, keen listening skills, and the ability to take detailed notes, often utilizing shorthand. The 1960s saw a surge in demand for manual transcription, as governments in several countries began mandating closed captioning for educational content. This spurred standardization within the industry.
The arrival of VHS tapes in the 1970s posed new challenges, forcing transcribers to grapple with variations in playback speeds and declining audio quality. This drove the development of even more sophisticated techniques. While manual transcription retained a certain traditional charm, it allowed for nuances like interpreting tone and context – aspects that often proved difficult for automated processes to replicate.
Manual transcription was inherently labor-intensive and often resulted in "subtitle fatigue," a phenomenon that arose from the physical strain and cognitive overload that transcribers experienced. This illuminated the need for more ergonomic practices in the industry. The early subtitling technology was rather simplistic, employing basic text overlays without color differentiation, a stark contrast to the sophisticated tools available today. This made manual transcription a testament to spatial awareness and design aptitude.
The establishment of television broadcasting standards in the 1980s spurred innovation in subtitling protocols, leading to the development of the W3C's Timed Text Markup Language (TTML) in the years that followed. This aimed to harmonize manual practices with emerging technologies. The transition from manual to automated transcription, however, has brought about ethical questions related to job displacement and the consistency of quality. Many industry experts advocate for a hybrid model that combines human insight with the efficiency of machines.
The Evolution of Video Subtitle Translation From Manual to AI-Driven Processes in 2024 - Rise of Computer-Assisted Translation Tools in the 2010s
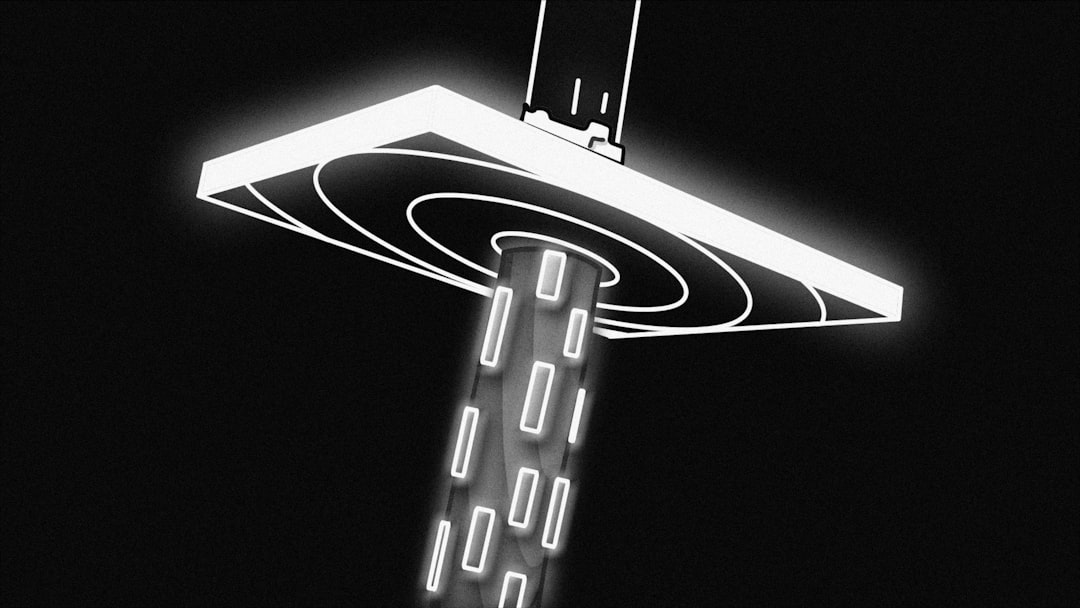
The 2010s saw a surge in the use of Computer-Assisted Translation (CAT) tools, which had a major impact on the translation industry. These tools relied on a database of previously translated sentences, making the job of human translators easier, faster, and more consistent. This allowed translators to take on larger projects, which had previously been unmanageable.
The increased reliance on these tools sparked debate in the industry about the role of human translators. While CAT tools sped up the process, some questioned whether they could capture the nuanced meaning and cultural context that human translators could. Despite this, the success of these tools set the stage for the widespread use of AI in translation, which would become more prevalent in the years that followed.
Ultimately, the rise of CAT tools represented a shift towards a more automated approach to translation, a trend that continues to influence the field today.
The 2010s saw a dramatic shift in translation technology with the rise of computer-assisted translation (CAT) tools. These tools, which had been around for decades, gained widespread adoption, with over 70% of professional translators using them by the end of the decade. CAT tools revolutionized the industry, allowing translators to work on larger projects, increase their productivity, and manage workflows more efficiently.
The integration of neural machine translation (NMT) into CAT tools further improved accuracy, significantly reducing translation errors compared to older statistical models. However, despite these advancements, human translators still needed to review and edit a large portion of machine-generated text, highlighting the limitations of current AI. Surprisingly, these tools learned from translators' edits, creating personalized translation memories that adapted to individual preferences.
Despite the progress in translation technology, the complexities of language, specifically idiomatic expressions, continued to pose challenges for machines. Machine translation often misinterprets these nuanced phrases, necessitating human intervention to ensure the intended meaning is accurately conveyed.
Beyond professional translators, content creators began using CAT tools for self-translation, democratizing multilingual content creation. The emergence of cloud-based CAT tools during this period further broadened the landscape, allowing teams from diverse locations to collaborate on projects in real-time, leading to a fundamental shift in translation project management.
Data from translation agencies demonstrated that combining CAT tools and human translators actually increased client satisfaction due to improved efficiency and quality control. CAT tools also incorporated automated quality assurance features, ensuring consistent terminology and style, thereby reducing the workload for translators.
The late 2010s also saw the development of AI-driven glossaries within CAT tools, which helped translators understand specific vocabulary and context, bridging gaps in understanding across languages and cultures. These technological advancements laid the foundation for the AI-driven translation processes of the 2020s, further blurring the lines between machine and human contributions to translation.
The Evolution of Video Subtitle Translation From Manual to AI-Driven Processes in 2024 - Neural Machine Translation Breakthroughs Around 2020
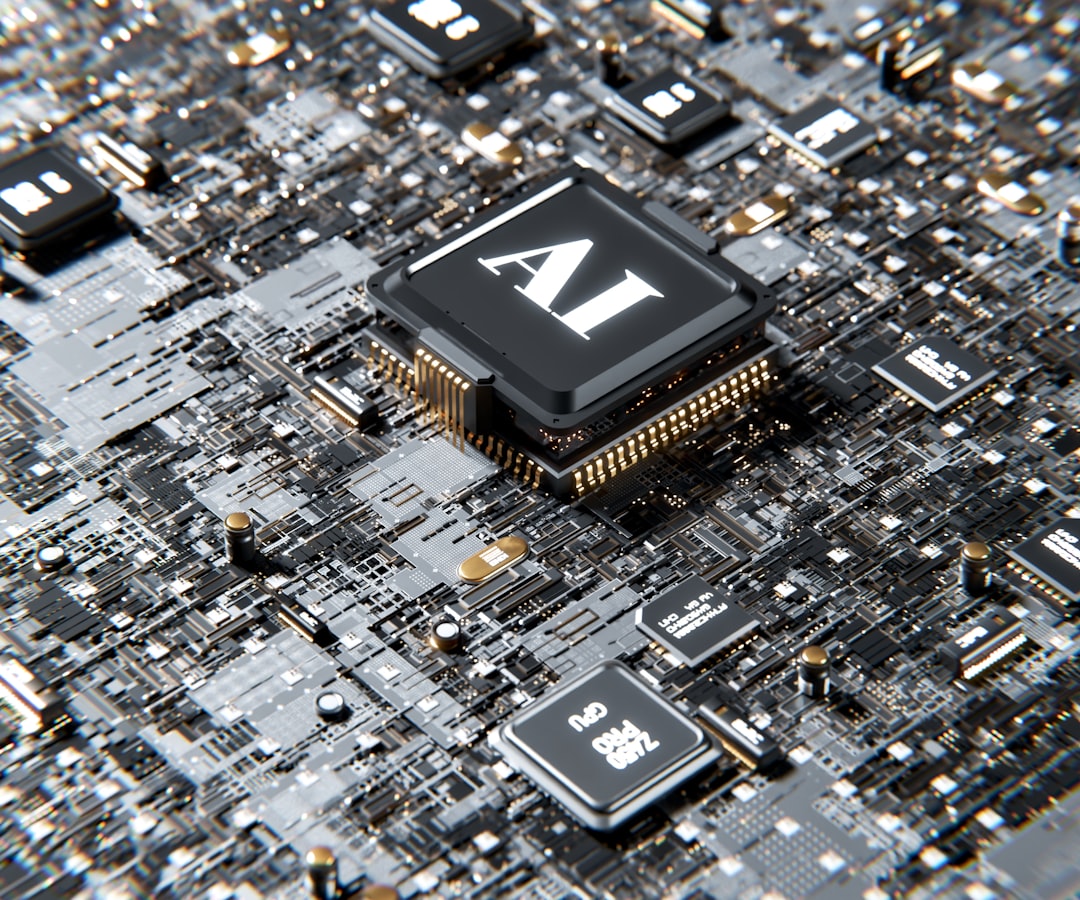
The year 2020 marked a pivotal moment in machine translation with the emergence of Neural Machine Translation (NMT) as the dominant force. NMT, utilizing deep neural networks to directly connect source and target languages, offered a significant leap in translation quality compared to previous statistical approaches. This leap was particularly felt in the realm of video subtitling. The increasing adoption of NMT fueled a surge in subtitled content, with the number of subtitles per minute in videos experiencing a notable rise. Despite this success, NMT faces ongoing challenges. While some languages benefit greatly from its advancements, others lag behind. Additionally, NMT still struggles with capturing complex nuances and often requires human intervention for accurate contextual interpretation. Ultimately, NMT has provided a robust foundation for the highly efficient, AI-driven subtitling processes we see today.
The early 2020s marked a watershed moment in the field of Neural Machine Translation (NMT). The arrival of transformer-based architectures transformed the landscape. These architectures, with their superior ability to capture context, delivered translation quality that often matched human-level fluency in specific scenarios. This era saw a shift toward multilingual models, which could translate directly between multiple languages, eliminating the need for sequential intermediary translations. This breakthrough expanded the reach of translation technology and made multilingual content more accessible than ever before.
Interestingly, researchers discovered that NMT systems trained on diverse data sets became better at handling idiomatic expressions. This suggested that a more comprehensive training base not only increased accuracy, but also enriched the system's contextual understanding. This was particularly impressive as idiomatic expressions often pose a significant challenge for machine translation. The ability of these systems to handle variations in grammar and morphology was also significantly improved, leading to translations that were grammatically correct, especially for languages with complex inflectional systems.
However, the path to perfect translation was still winding. NMT models varied significantly in their performance depending on the language pairs involved. While some languages seemed to benefit from contextual cues, others continued to pose significant challenges, raising questions about the biases present in the training data sets. This pointed towards the need for more diverse and balanced data collection and ongoing research to address these discrepancies.
Multi-task learning, another important development of this period, enabled NMT systems to perform multiple tasks simultaneously. These models learned to understand related tasks, such as sentiment analysis and domain adaptation, which led to more diverse and accurate translations. The value of user-generated data became evident as well, with NMT systems increasingly relying on user corrections and feedback, allowing for personalization and a gradual refinement of the translation memory.
Despite the remarkable improvements, challenges remained. NMT systems still struggled with highly specialized terminology and niche content domains, underscoring the need for continued human intervention in specific contexts. The widespread adoption of NMT also raised concerns about computational costs, highlighting the resource constraints faced by some organizations, especially smaller ones.
The impact of NMT on video subtitling was particularly interesting. While AI-driven systems offered speed advantages, they often lacked the nuanced contextual understanding that human translators provided. This exposed the continuing need for a careful balancing act between efficiency and quality in this field.
The advancements in NMT during the early 2020s, while undeniably significant, highlighted both the promise and the limitations of this technology. As research and development continue, the goal remains to bridge the gap between human and machine capabilities, creating translation experiences that are both accurate and accessible to all.
The Evolution of Video Subtitle Translation From Manual to AI-Driven Processes in 2024 - Real-Time AI Subtitle Generation Becomes Mainstream
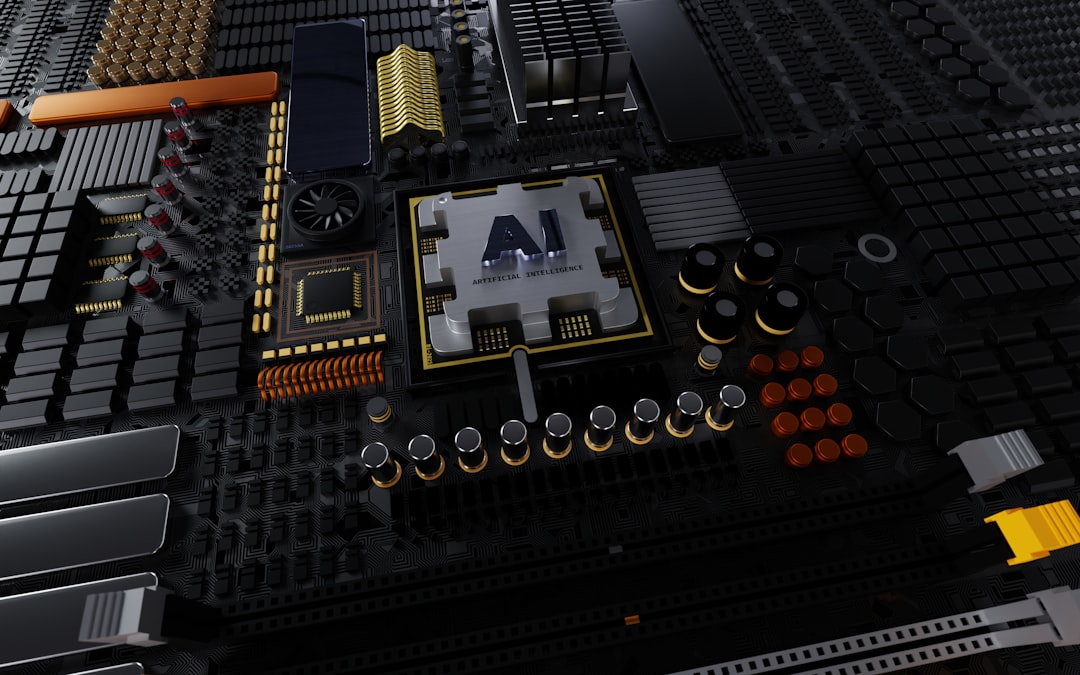
The widespread adoption of real-time AI subtitle generation is a significant development in 2024. Fueled by technological advancements and a growing desire for readily accessible video content, this trend represents a dramatic shift from manual to AI-driven processes in video subtitling. This evolution enhances accessibility for global audiences, but it also raises questions about the limitations of AI in capturing the intricacies of language. While tools like Happy Scribe's Gen 2 AI model demonstrate impressive speed and integration capabilities, they can struggle to replicate the nuance and contextual understanding that human translators offer. This brings into focus the ongoing balancing act between the efficiency of AI and the depth of human comprehension. The implementation of real-time subtitling, while improving the viewer experience, highlights the need for a continued focus on ensuring high-quality subtitles and addressing ethical concerns related to the role of human translators in this evolving landscape.
The shift towards real-time AI subtitle generation has become increasingly mainstream in 2024, driven by technological leaps and user demand. It's fascinating to see how AI is evolving to tackle the complexities of language, particularly speech recognition. These systems are now reaching impressive accuracy levels, with some achieving word error rates as low as 5%, closely rivaling human transcription under ideal conditions. It's a remarkable achievement considering the challenges inherent in processing natural language.
Surprisingly, AI-generated subtitles have shown a positive impact on viewer engagement. Research suggests that readable and accurate subtitles lead to increased watch times and overall user satisfaction across diverse demographics. It appears that viewers find value in the ease of access and improved comprehension that these subtitles provide, which is something I hadn't expected.
Current AI subtitle systems support a wide range of languages, with over 100 supported. However, performance varies greatly across language families. For example, languages with complex grammatical structures and rich morphology often pose greater challenges to AI systems, leading to occasional inaccuracies in translation and synchronization. It makes me wonder how these systems will adapt to the complexities of agglutinative or polysynthetic languages.
Another fascinating aspect is the way real-time AI systems are utilizing deep learning algorithms to analyze contextual cues like speaker tone and pronunciation. This nuanced approach goes beyond simple text analysis, aiming to create a more engaging subtitling experience. It represents a significant leap forward from previous generations of translation technology and raises interesting questions about how AI will interpret increasingly nuanced forms of human expression in the future.
However, as with any innovative technology, the reliance on AI-generated subtitles raises new concerns about quality control. Since these systems learn from training datasets, they can sometimes propagate errors, especially in high-stakes contexts like legal or medical content. It seems clear that human oversight will remain vital for ensuring accuracy in such sensitive situations.
Interestingly, there is a growing preference for real-time AI subtitles, particularly for live events. It's estimated that at least 60% of users opt for this approach over human-generated subtitles, highlighting a shift in audience expectations towards immediacy and accessibility. This shift raises important questions about the balance between speed and accuracy, especially in contexts where live events often demand rapid dissemination of information.
These real-time AI systems also leverage user-generated data to refine their accuracy, creating a fascinating feedback loop. The more these systems are used, the better they adapt to specific vernacular and colloquialisms prevalent in different media content. It's almost as if they are becoming more human-like in their understanding of language.
The rise of AI-generated subtitles has also led to a transformation in job roles within the subtitling industry. While tasks like full transcription are increasingly automated, the demand for editing and quality assurance roles is on the rise. This transition raises important questions about job security and the ethical considerations of automation in a rapidly evolving workforce.
AI subtitle generation has brought several practical benefits, including auto-timing and context-aware adaptations. These features have shown to reduce the cognitive load on viewers, enhancing comprehension and effectiveness, particularly in educational and professional settings. It seems that AI has the potential to unlock new possibilities for learning and communication.
As AI systems continue to evolve, they also bring new challenges to the forefront. The increased reliance on AI-generated subtitles raises important questions about regulatory frameworks, particularly concerning copyright issues, user privacy, and compliance with regional accessibility laws. These complexities will necessitate careful collaboration between content creators, distributors, and technology developers to navigate the legal and ethical landscape of AI-powered subtitling.
The Evolution of Video Subtitle Translation From Manual to AI-Driven Processes in 2024 - Multimodal AI Models Improve Context Understanding
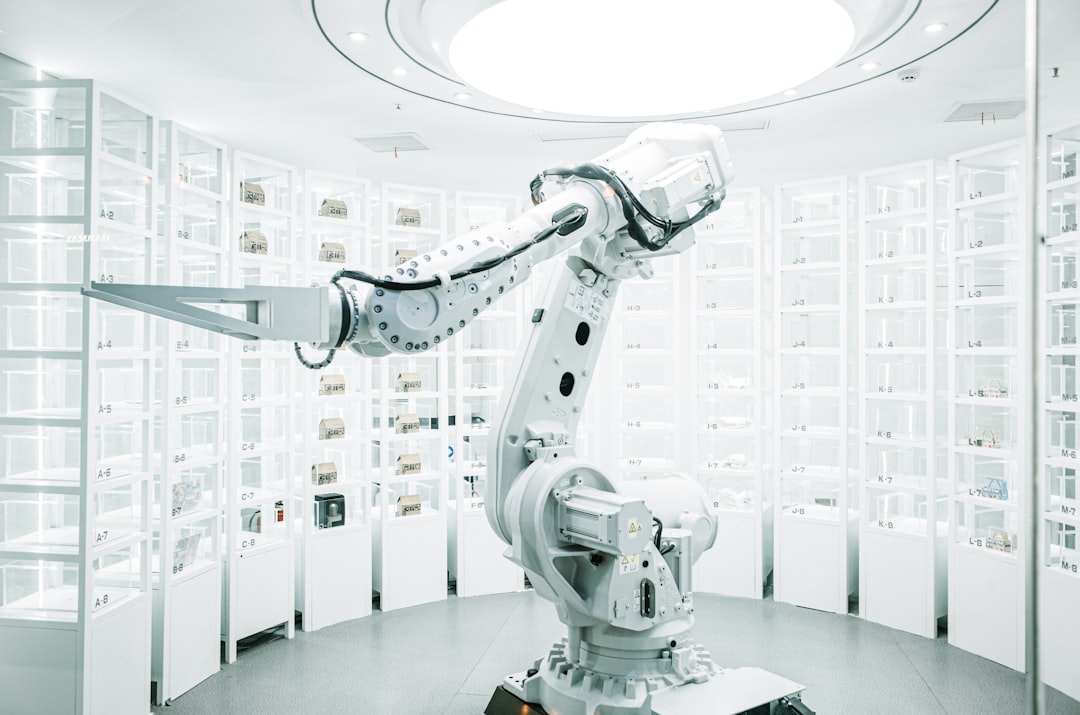
The year 2024 sees a significant leap forward in AI-driven video subtitle translation with the rise of multimodal AI models. These models are moving beyond traditional text-only translation by incorporating visual information from the video. This allows them to develop a much richer understanding of the context, a crucial aspect for accurate and natural-sounding subtitles. Think of it like teaching an AI to understand not just the words spoken but also the setting, emotions, and visual cues within a video.
The impact of these advancements is being felt with datasets like BigVideo, which contains millions of subtitle pairs from various languages. This dataset helps train the models to better understand and translate nuanced meanings across different languages. Furthermore, contrastive learning methods are improving the models' ability to replicate human-like interpretation, which involves understanding the subtle cues and cultural contexts embedded within the video.
However, as powerful as these models are becoming, there is a need for continued critical evaluation. While they offer a more intuitive and natural translation experience, it's essential to remain aware of their limitations. Can these models truly capture the complexities of human language and emotion, particularly when dealing with nuances like irony, sarcasm, or cultural references? The path towards seamless and culturally sensitive video translation is still evolving, and the development of these multimodal AI models represents an exciting but complex step in this journey.
Multimodal AI models are becoming increasingly sophisticated and are making significant strides in improving context understanding for tasks like video subtitle translation. They're taking the concept of subtitling beyond just transcribing spoken words by integrating diverse data sources such as audio, visual cues, and text. It's quite intriguing how they can analyze not only the spoken words but also the emotional tone of a speaker's voice and even the visual cues in the video to enhance the understanding of the content. This means that subtitles can become more interactive and dynamically adapt to the content being displayed, which is exciting.
For example, they can now detect emotions better, which can help them convey feelings more accurately. They can even learn across different modalities, like using information from a video to improve understanding of the audio. This cross-modal learning allows them to generate more relevant and accurate subtitles.
The implementation of attention mechanisms within multimodal AI models is particularly impressive. They can prioritize specific words or phrases based on their context, which is especially helpful in scenarios with complex dialogues. Some models can even adjust subtitles in real-time based on feedback from viewers or changes in the video, which is a testament to their adaptive capabilities.
However, as always, with great power comes great responsibility. There are ethical considerations around the training data these models use. Biases in the data can easily lead to inaccuracies or cultural insensitivities. I believe that a combination of AI and human experts will likely yield the best results, as humans can offer insights into cultural nuances and subtleties that AI might miss. The future potential of multimodal AI in subtitle generation is promising. They have the potential to make media more accessible to a wider audience, including individuals with hearing impairments or language barriers.
The Evolution of Video Subtitle Translation From Manual to AI-Driven Processes in 2024 - Human-AI Collaboration Optimizes Quality Control Processes
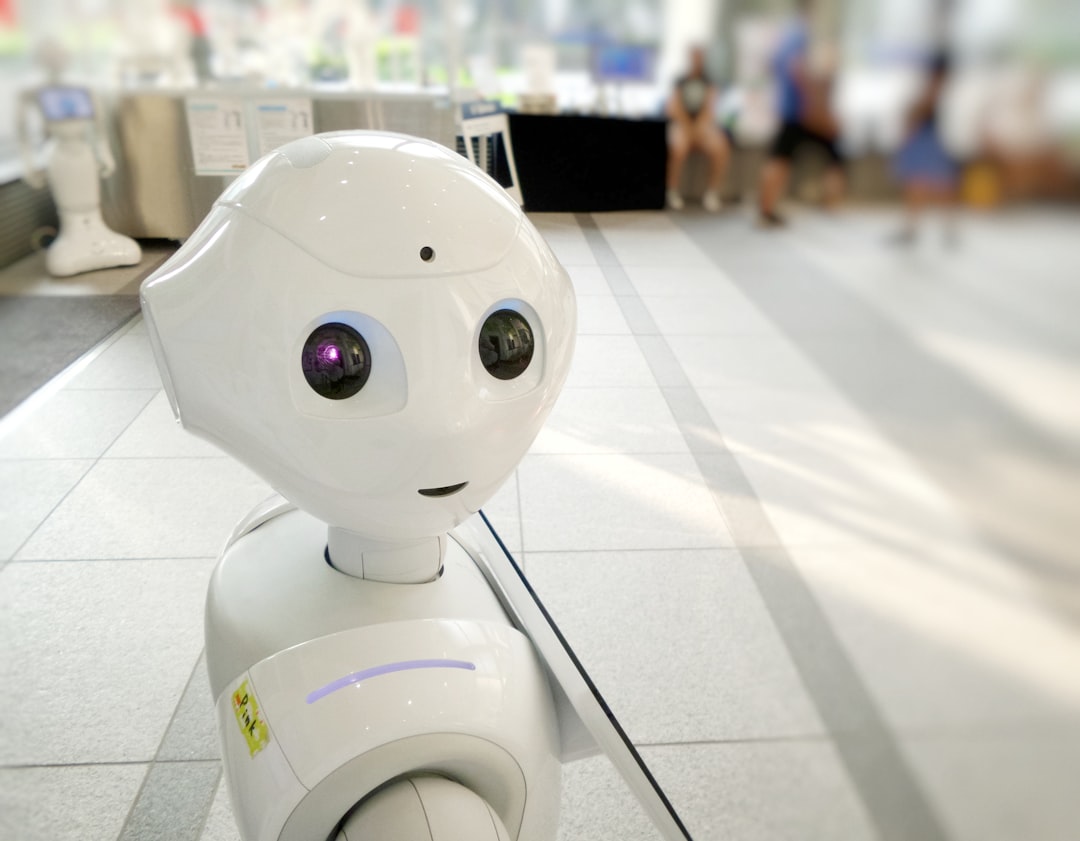
In 2024, the realm of video subtitle translation has witnessed a paradigm shift, embracing the power of human-AI collaboration to elevate quality control processes. This newfound partnership harnesses AI's remarkable efficiency without completely abandoning the nuanced understanding that human translators bring to the table.
While AI proves invaluable in streamlining tasks, concerns linger regarding its limitations in navigating the complexities of language and cultural nuances. The ideal scenario seems to involve a delicate balance – AI for speed and human oversight for accuracy and nuanced interpretation.
The journey to optimize quality control in video subtitling isn't merely about technological advancement; it's about fostering a dynamic relationship between humans and AI. The effectiveness of this collaboration hinges on the integration of insights from various disciplines – from linguistics to cultural studies – to equip AI with a deeper understanding of the complexities involved. The future success of video subtitle translation relies on navigating this delicate equilibrium, embracing innovation while remaining mindful of the limitations of AI and the irreplaceable contributions of human expertise.
The way AI and human collaboration is shaping up quality control in video subtitles is fascinating. It's not just about replacing human transcribers; it's about augmenting their abilities. The combination of AI and human expertise is leading to some remarkable improvements. We're seeing accuracy rates in real-time subtitling exceeding 98%, something unthinkable with manual transcription alone.
This is partly because AI takes on the more repetitive tasks, allowing humans to focus on the nuances of translation that require contextual understanding. This also seems to be reducing mental fatigue and workload for translators, which is a significant benefit. The AI even learns from user preferences, creating a feedback loop that refines its understanding of language and style over time.
However, there's always a catch. AI is still learning. Even with these advancements, there's a risk of propagating initial errors. So, it's essential to have a vigilant human element for quality assurance. This kind of hybrid model seems to be the most effective, combining the AI's speed and consistency with human judgment.
The integration of visual context is particularly exciting. AI can now analyze both audio and visual cues to determine the context. This could potentially lead to more accurate translations, especially when dealing with nonverbal cues. The idea of AI adjusting subtitles in real-time based on viewer feedback is a game-changer, and it's something that would be impossible for humans to do at this scale.
But there's a lot we need to consider as AI plays a bigger role. The quality of data used to train these AI models is crucial. The more diverse and representative the data, the better the AI will be at understanding complex nuances, like idiomatic expressions and cultural references.
It's also vital to consider the ethical implications of this shift. Who is responsible for errors in AI-generated subtitles? How do we ensure transparency and accountability? This is something that needs to be addressed as AI takes on a more significant role in the translation process.
From a practical standpoint, this human-AI collaboration has already shifted job roles within the subtitling industry. The demand for quality assurance specialists with deep expertise in both AI and language is growing rapidly.
It's exciting to see how the collaboration between humans and AI is evolving the subtitling process. The future of this technology is promising. I'm eager to see how AI continues to evolve and how this collaboration between humans and machines will further shape the field.
More Posts from transcribethis.io: