Experience error-free AI audio transcription that's faster and cheaper than human transcription and includes speaker recognition by default! (Get started for free)
The Evolution of American-to-British English Translation Tools A 2024 Overview
The Evolution of American-to-British English Translation Tools A 2024 Overview - Historical Roots From Punch Cards to Digital Dictionaries
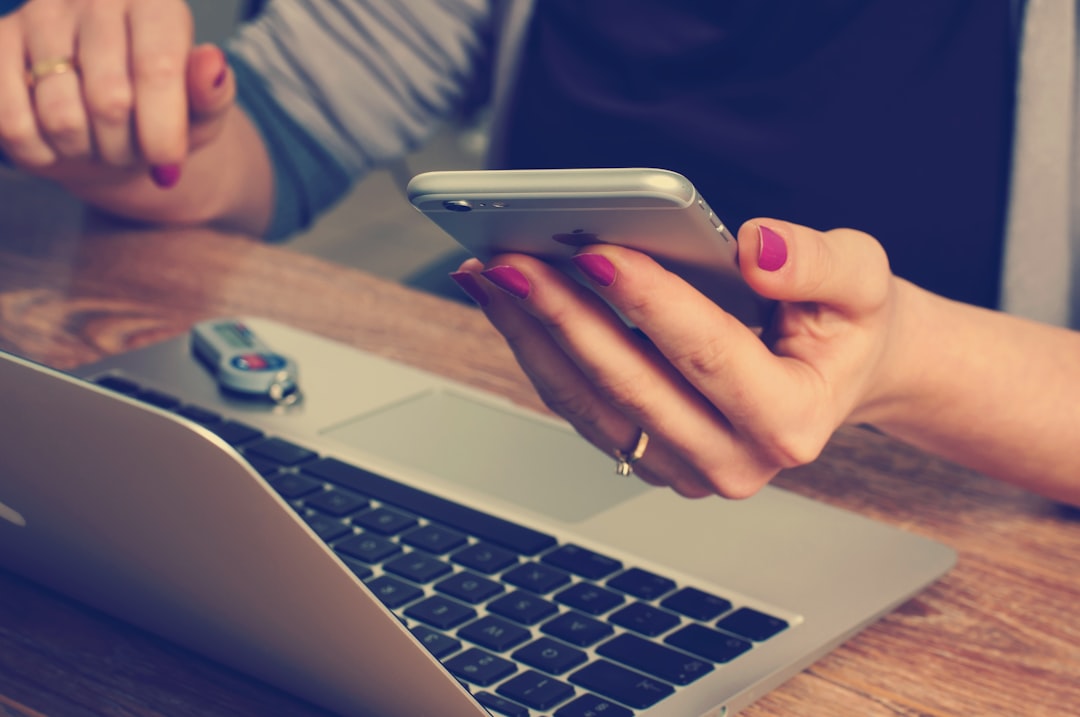
The path from punch cards to digital dictionaries illustrates the close connection between technological advancements and how we record and understand language. This journey began with ancient glossaries and the publication of influential dictionaries like Webster's and the Philological Society's efforts, all of which shaped the ever-evolving field of English lexicography. Hermann Hollerith's punch card system, inspired by earlier textile innovations, marked a turning point in data management and set the stage for modern computing and the digital tools we use today. This historical progression has led to sophisticated translation tools that improve our grasp of American-to-British English, demonstrating the ongoing interaction between technology and language.
The history of dictionaries and translation tools is a fascinating journey that reflects the evolution of technology and its impact on language processing. Early forms of dictionaries, often consisting of glossaries or simple word lists, existed long before the printing press. The Renaissance period saw dictionaries emerge as tools for translating between different vernacular languages, a trend that continues to this day.
The development of punch cards in the late 19th century, initially intended for census data processing, marked a significant advancement in data handling. While not initially conceived for linguistic tasks, their application in early data storage foreshadowed the integration of technology into language processing. The first electronic dictionaries, limited by the computing power of the time, appeared in the 1960s, demonstrating the intertwined relationship between available technology and the advancement of linguistic resources.
Early digital translation tools, often reliant on rule-based systems, faced considerable limitations when dealing with idiomatic expressions, leading to humorous misinterpretations. The introduction of personal computers in the 1980s brought about a democratization of access to translation tools, allowing for a wider range of users.
The advent of artificial intelligence in the early 2000s did not immediately result in a significant leap forward in translation quality. The limitations of these early systems highlighted the challenges in achieving nuanced language understanding. However, user feedback and the utilization of vast databases from multiple sources, including social media and academic publications, have led to significant improvements in translation accuracy. The development of digital dictionaries is an ongoing process that reflects the dynamic interplay between technological advancements, linguistic understanding, and user input.
The Evolution of American-to-British English Translation Tools A 2024 Overview - The Rise of Statistical Machine Translation 2006-2015
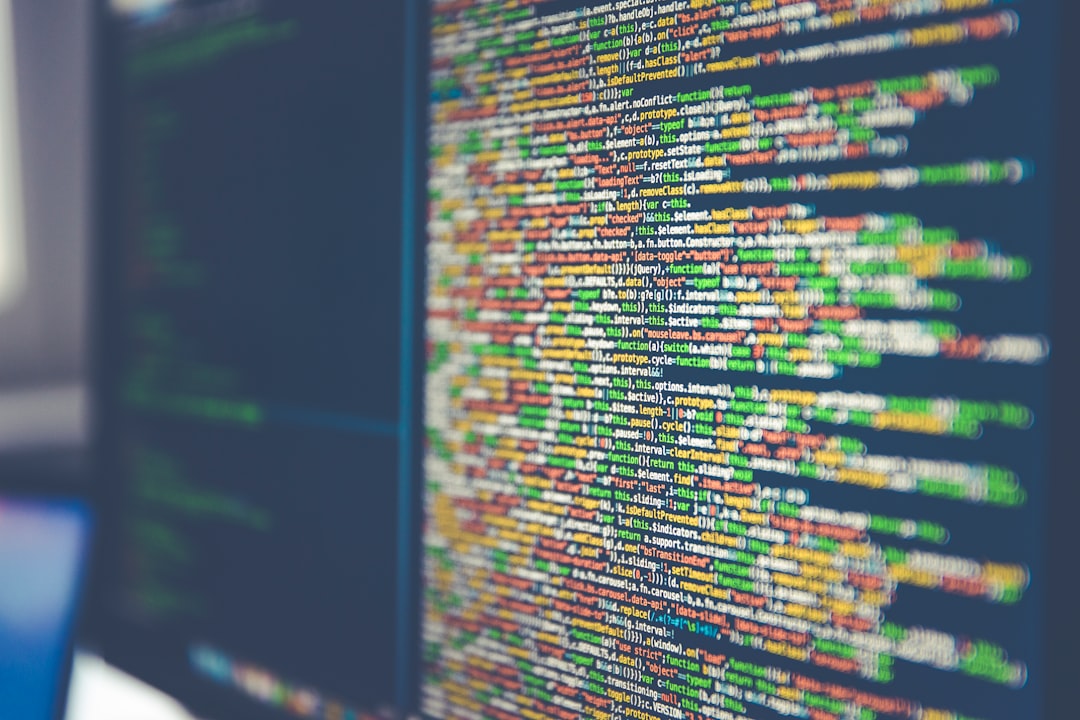
Between 2006 and 2015, statistical machine translation (SMT) rose to prominence as a game-changer in the field of translation. This period marked a decisive departure from rule-based methods, with researchers embracing massive collections of bilingual texts. This shift in focus allowed them to analyze language patterns and enhance translation accuracy in a way that had never been possible before. The influx of data-driven techniques into SMT led to more complex algorithms better equipped to handle the intricate nature of human language. Despite initial doubts about its reliability, advancements in SMT during this time dramatically impacted the adoption of automated translation across industries. By 2015, statistical approaches had become the dominant method, proving the enduring power of collaborative innovation between linguistic theory and technological advancement.
The period from 2006 to 2015 saw a surge in the field of statistical machine translation (SMT) fueled by the rise of data-driven models. This marked a departure from the traditional rule-based systems, which often struggled to accurately capture the nuances and complexities of language, especially when dealing with idiomatic expressions. The introduction of phrase-based translation models, which could translate whole phrases rather than individual words, significantly improved fluency and accuracy. This progress was further fueled by the availability of extensive bilingual datasets like the European Parliament Proceedings and United Nations documents, providing vital training materials for these systems.
During this time, advancements in machine learning techniques like Gaussian processes and discriminative training had a profound impact on SMT, resulting in translations that were more contextual and accurate. This was also a period of intense competition between major technology companies and research institutions, driving rapid advancements in SMT algorithms, resulting in dramatic improvements in translation quality. While these advancements were remarkable, challenges remained, particularly in addressing polysemy – the ability of a word to have multiple meanings. This often resulted in ambiguous translations as SMT systems struggled to identify the appropriate context.
This era saw the standardization of evaluation metrics like BLEU (Bilingual Evaluation Understudy) for gauging the quality of SMT. However, critics argued that these metrics didn't always accurately reflect the nuances of human judgment on translation quality. Despite these limitations, the rise of SMT facilitated collaborative translation efforts on platforms like Wikipedia, showcasing its utility beyond traditional translation services and providing greater access to information across linguistic barriers.
The end of this period marked a paradigm shift as neural machine translation (NMT), which utilizes deep learning architectures, emerged as the new standard. These NMT systems were better able to handle long-range dependencies and contextual information, surpassing the capabilities of SMT. However, it's important to note that SMT, while being largely replaced by NMT, continued to excel in translations between certain language pairs. On the other hand, less-represented languages still lacked sufficient training data, creating a persistent challenge in achieving equitable translation capabilities for all languages. The ongoing quest to address this disparity in language representation remains an important area of ongoing research and development.
The Evolution of American-to-British English Translation Tools A 2024 Overview - Neural Networks and AI A Game Changer for British-American Translation
Neural networks and AI are transforming the field of British-American translation. These new tools rely on sophisticated algorithms that are designed to better understand the nuances of language. While older systems often faltered when faced with idioms and slang, neural machine translation (NMT) has made significant strides by using deep learning to grasp the context of words and phrases. This has led to translations that are more accurate and natural sounding. However, despite the promise of these advances, certain challenges persist. These include ensuring high-quality translations for less commonly used languages and overcoming the difficulties of translating more complex passages. Additionally, the significant computational resources needed to train and operate these systems remain a hurdle. Overall, NMT represents a huge leap forward, but it highlights the ongoing need to balance technological advancements with the complex needs of translation.
Neural networks, specifically those employing deep learning, have shown remarkable promise in understanding and producing text with context in mind, crucial for capturing the nuances of American and British English. Unlike traditional methods that rely on strict rules, neural machine translation (NMT) models learn from extensive data sets, enabling them to identify intricate linguistic patterns and idiomatic expressions that simpler models might miss. This allows for translations that possess a natural flow, mirroring human fluency, due to NMT's multi-layered neural architecture that considers broader contexts for more cohesive results.
The introduction of transfer learning has transformed the training process of neural networks for translation, allowing pre-trained models to be refined for specific language pairings, including those less commonly represented in training data. However, despite these advancements, NMT still faces challenges with polysemy—the phenomenon of words having multiple meanings depending on context. This ambiguity can complicate translations, requiring a nuanced comprehension of the language.
Advanced neural architectures excel at maintaining long-range dependencies within sentences, significantly improving translation quality compared to earlier statistical methods, where context often faded. Incorporating attention mechanisms within neural networks enables these systems to dynamically focus on important parts of the input sentences, boosting accuracy and safeguarding crucial linguistic components.
While neural networks have revolutionized the field of translation tools, critics highlight ongoing challenges in handling cultural subtleties and idiomatic expressions, suggesting human oversight is still vital in high-stakes translation situations. These advanced neural models have dramatically improved accessibility and usability of translation tools, empowering individuals from diverse backgrounds to communicate more effectively across American and British English. Yet, the dependence of NMT systems on massive datasets raises concerns about bias in translation output, as training on unrepresentative data could perpetuate stereotypes or misinterpretations, posing ethical dilemmas for developers.
The Evolution of American-to-British English Translation Tools A 2024 Overview - Adapting to Colloquialisms and Cultural Nuances in 2024
Translation tools in 2024 are facing a crucial challenge: effectively capturing the nuances of colloquialisms and cultural references that exist between American and British English. As globalization intensifies, translation systems need to evolve beyond just translating words, embracing the complexities of slang and regional expressions that reflect cultural shifts. This means recognizing how phrases like "let them cook" have become part of the contemporary lexicon, shaping how we speak and interact. While AI and machine learning are driving progress in translation accuracy and context sensitivity, challenges still remain. These tools often struggle to grasp the subtle meanings behind idioms and generational slang. This emphasizes the need for a "culture-first" approach that goes beyond just language and integrates the cultural fabric influencing communication. This shift towards more inclusive and culturally aware translation tools signals a crucial step in fostering deeper understanding in our increasingly multicultural world.
The evolution of translation technology is fascinating, but it still faces some significant challenges when dealing with colloquialisms and cultural nuances in 2024. One issue is that even within the US and the UK, regional variations exist, making it difficult for translation tools to keep up with the different ways people speak. For example, a phrase that's common in one region might be completely unknown in another, creating potential misunderstandings when trying to translate it.
Another challenge is the constant emergence of new slang and idioms, reflecting current trends in popular culture. These new terms can be very hard to track, and translation tools need to adapt quickly to include them in their systems, or risk falling behind the times.
There's also the issue of semantic nuances, where one word can have completely different meanings depending on the context. Take, for example, the word "biscuit." In the UK, it refers to a sweet snack, while in the US, it often means a more savory, bread-like side dish. These differences can be tricky for AI models, and they need to be able to understand the context of a word to provide accurate translations.
This all comes down to the need for a deeper understanding of local culture. Many colloquialisms are infused with pop culture references, historical context, and humor, making them difficult for AI systems to grasp fully. There's often a need for culturally aware humans to help out, offering insights that automated models can't always pick up on.
Of course, it's also important to consider gendered language. Certain phrases may carry gendered implications, and translation models need to be sensitive to these contexts to avoid perpetuating stereotypes and biases. The goal is to ensure translations are neutral whenever possible.
Emotion and tone are important too. For example, "I'm chuffed" in British English expresses happiness in a specific way. Translating it simply as "happy" misses the informality and affection implied. This highlights the ongoing challenge for translation tools in accurately conveying tone.
It's not just about understanding the language itself, but also the history behind certain phrases. These historical roots can add depth to their meaning, but they are often missed by automated systems. To fully grasp a phrase, a more nuanced understanding of how language evolves is needed.
Modern translation tools often rely on user feedback to improve their algorithms. While this can be helpful in adapting to colloquialisms, it also raises concerns if the feedback is biased in some way.
Phrasal verbs and idiomatic expressions are another challenge. English makes frequent use of these, which can be confusing for translators because their meanings are not literal. For example, "give up" doesn't mean to physically give something to someone, and understanding these expressions is crucial to accurate translation.
Context is also more important than ever. With conversations happening on social media and in other online settings, the context of the language matters significantly in ensuring the translation accurately conveys the meaning.
In short, the ongoing challenge for translation technology is to adapt to the ever-changing nature of language, incorporating regional differences, new slang, cultural nuances, and user feedback to create truly effective translation experiences.
The Evolution of American-to-British English Translation Tools A 2024 Overview - Human-AI Collaboration in Modern Translation Tools
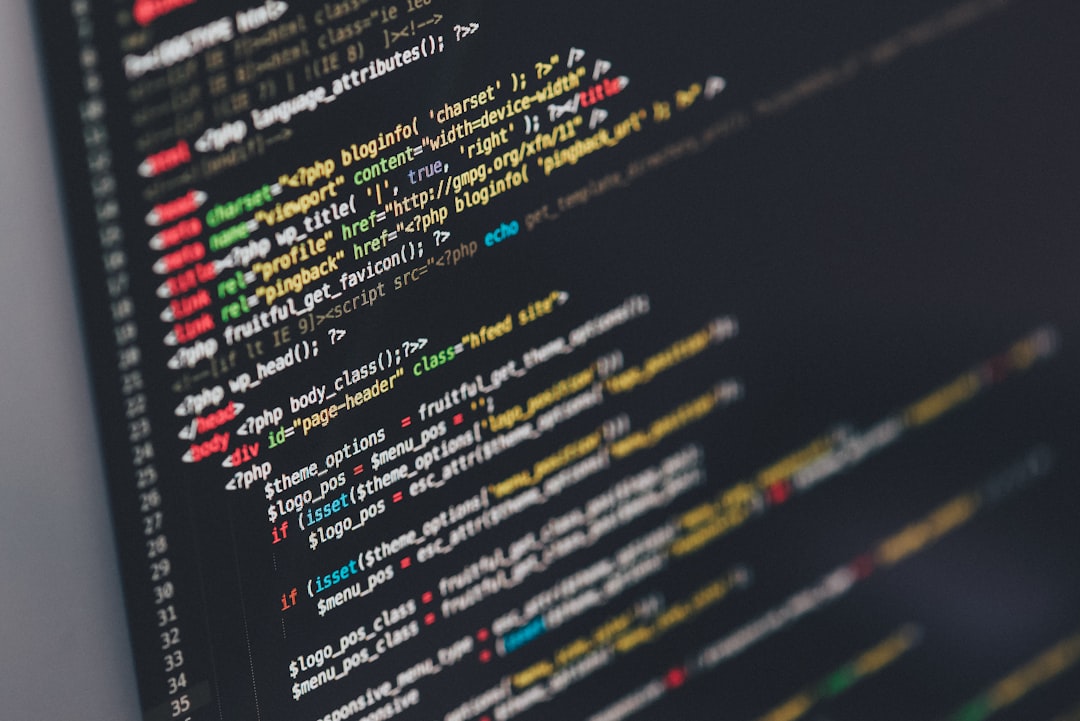
The modern translation landscape is increasingly defined by a collaborative relationship between human expertise and artificial intelligence. This partnership harnesses human creativity and cultural insights, merging them with the efficiency of AI systems to create more nuanced and accurate translations. Despite significant advancements, the unique strengths of human translators, particularly in situations that demand a deep cultural understanding, remain crucial for high-quality outputs. As translation technologies evolve, recognizing and integrating this human element is becoming increasingly important, especially in fields like legal translation, where precision is paramount. The ongoing interplay between machine capabilities and human intuition underscores the necessity for flexible, context-aware systems that honor and reflect the complexities of language.
The world of translation is undergoing a fascinating transformation, with human and AI working together in unexpected ways. It’s no longer a simple case of feeding text into a machine and getting a perfect translation. Now, it’s a collaboration where humans and AI are learning from each other, pushing the boundaries of communication.
For instance, translation tools now leverage the collective intelligence of both humans and AI. It's like a vast team of linguists working together, with AI providing speed and scale, while humans contribute their nuanced understanding of language and culture. This dynamic interplay is particularly evident in the rapid adaptation of these tools to evolving slang and regional dialects.
It’s fascinating to see how translation platforms are using feedback loops to refine their algorithms. When users flag incorrect translations, the AI uses this information to learn and improve, becoming more adept at handling the nuances of human language. This real-time feedback process allows AI to continually refine its understanding of slang, cultural references, and even the subtleties of humor and sarcasm.
And then there’s the intriguing use of cultural context databases. These databases are like massive libraries of cultural knowledge, providing context-sensitive insights that AI-driven systems might miss. This is a crucial step in ensuring that translations are not just accurate but also culturally appropriate, reflecting the diverse world we live in.
However, we're not entirely ready to hand over the reins to AI. There are still certain aspects of translation that AI struggles with, particularly when it comes to intuition and the deeper understanding of context. Human translators excel in these areas, offering their unique blend of cultural awareness and linguistic expertise, especially for high-stakes translations.
One notable challenge for AI systems is polysemy, where a single word can have multiple meanings depending on the context. Here, human intuition plays a crucial role, guiding the AI toward the most appropriate interpretation.
The translation landscape is becoming even more complex with the emergence of blended modeling approaches. These approaches combine the strengths of both statistical and neural methods, allowing for greater flexibility and accuracy. This means that the tools can leverage vast data patterns while also being adaptable to idiomatic expressions and evolving language trends.
Perhaps the most impressive feat of modern translation tools is their ability to instantly adapt to new trends. This is a significant advantage in today’s fast-paced world where slang and colloquialisms are constantly evolving. It's like having a translation tool that can keep up with the latest internet memes!
Some of the most advanced translation systems are even exploring emotion recognition. This is a fascinating development, allowing the tools to identify the sentiment expressed in the original text and tailor the translation accordingly. Imagine being able to translate not just the words, but the emotions behind them!
Of course, with every technological advancement, we must consider potential pitfalls. In the case of AI-driven translation, there is a growing concern about data bias. Because these systems learn from existing data, any imbalances or biases in those datasets can manifest in the translations, creating ethical concerns.
However, there are exciting initiatives to address these issues. Many translation platforms are creating interactive learning environments where users can actively participate in the translation process. This provides a space for valuable human input, allowing AI to learn from user corrections and insights.
The integration of AI into translation tools is revolutionizing our understanding of language and communication. It’s a complex dance between human and machine, a testament to the creative potential of both. The future of translation promises exciting possibilities, fueled by a constant push to bridge the gap between technological capabilities and the nuanced complexities of human language.
Experience error-free AI audio transcription that's faster and cheaper than human transcription and includes speaker recognition by default! (Get started for free)
More Posts from transcribethis.io: