Experience error-free AI audio transcription that's faster and cheaper than human transcription and includes speaker recognition by default! (Get started for free)
The Evolution of AI-Powered Sound Effects Removal A 2024 Analysis
The Evolution of AI-Powered Sound Effects Removal A 2024 Analysis - Neural Networks Revolutionize Audio Processing Software
The integration of neural networks is dramatically altering the capabilities of audio processing software. We are seeing notable improvements in real-time manipulation and the detailed analysis of sounds. This technology is instrumental in speech recognition systems, forming the basis of virtual assistants and transcription software. Moreover, advances in deep learning techniques have led to better audio signal processing across a wider range of applications including speech, music, and environmental sound. Although progress exists in creating audio with AI, the field of audio synthesis using generative models still lags compared to image or text generation. Despite the positive trends, challenges remain, particularly related to the substantial computational resources required to process raw audio data in neural networks. This highlights the ongoing tension between the immense promise and the limitations that still exist in AI's role within audio technology.
The integration of neural networks into audio software has revolutionized the field by enabling a new level of sophistication in real-time sound manipulation and analysis. This is particularly evident in applications like speech recognition, powering features seen in voice assistants and transcription tools. Deep learning techniques have propelled improvements in handling various audio signals, whether it's human speech, musical instruments, or environmental noises. While the creation of audio through generative AI, like synthetic speech or music, shows promise, its progress still trails behind the advancements seen in image and text generation.
We are seeing the emergence of innovative AI-powered audio solutions, like the tools being developed by Adobe, which focus on enhancing audio quality in videos by effectively tackling issues like wind noise or microphone placement inconsistencies. Similarly, software like iZotope's Neutron 2 showcases the potential of AI, providing features like a "track assistant" capable of automatically identifying instruments and suggesting appropriate audio settings.
However, this increased performance comes at a price. Processing audio within neural networks frequently involves using raw time domain audio, providing detailed information but requiring substantial computational resources. iZotope's RX 6 exemplifies the direct application of AI in audio processing through its capability to isolate dialogue from complex background noise.
The exciting potential of combining AI with music production has unlocked new avenues for creative exploration. This includes possibilities like advanced music remixing and composing, opening up a vast array of potential for artists and producers. The surge in interest and innovative ideas around AI in audio processing suggests a very bright future for the merging of these technologies. Despite the exciting possibilities, concerns remain about the resource demands of neural network models, which may limit access for smaller studios or individual creators due to the need for significant computing power.
The Evolution of AI-Powered Sound Effects Removal A 2024 Analysis - Market Growth Signals Rising Demand for AI Audio Recognition
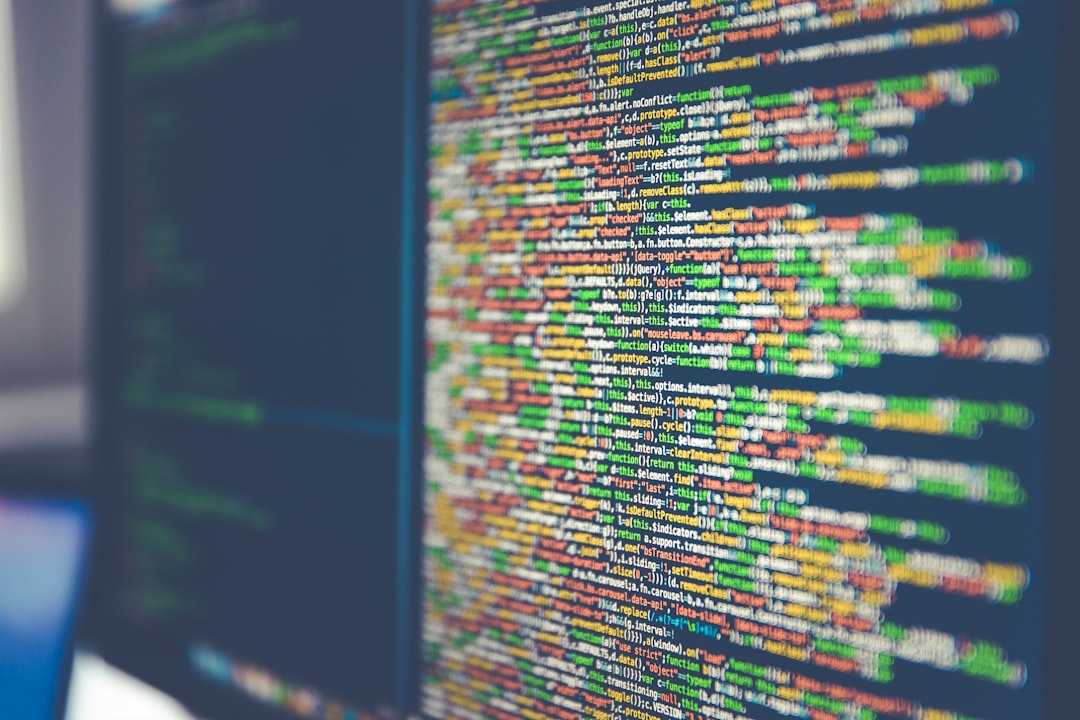
The expanding AI audio recognition market reflects a surge in demand, driven by technological progress and the rising use of smart devices. Predictions indicate a significant growth trajectory for the overall speech and voice recognition market, potentially reaching hundreds of billions of dollars by 2030, demonstrating a substantial rate of expansion year-over-year. Features like voice biometrics and AI-powered virtual assistants are becoming increasingly integrated into our daily routines, shaping a rapidly evolving audio recognition landscape. While this growth is encouraging, the reliance on substantial computing power to process audio data raises concerns about the accessibility and potential environmental impact of these technologies, especially for smaller developers or researchers. This blend of promising growth and inherent challenges creates a fascinating and complex stage for the future development of AI audio recognition.
The expanding market for AI audio recognition is quite compelling. Various market research reports suggest a strong upward trajectory, with projected compound annual growth rates (CAGRs) consistently exceeding 20% over the next few years. This signals a growing appetite for these technologies across different industries. It's encouraging to see that, under controlled conditions, these AI systems can achieve impressive accuracy levels exceeding 95%. This suggests that there's a real potential for these systems to revolutionize tasks like automated transcription and customer service interactions.
However, real-world scenarios present a different challenge. AI algorithms are becoming more sophisticated at disentangling and enhancing overlapping sounds in complex audio environments. This is critical for applications like post-production and broadcasting where clarity and intelligibility are paramount. Furthermore, the increasing popularity of voice-based interfaces, where it's reported that over half of users prefer vocal commands, fuels a demand for more robust audio recognition technologies. This demand incentivizes continuous innovation from developers to meet the evolving needs of users.
It's fascinating how AI audio recognition is finding its way into new domains. Healthcare, for example, is exploring the use of AI systems to analyze patients' vocal patterns for early disease detection. This kind of cross-disciplinary application highlights the versatility of the technology. Recent studies have also shown that reducing background noise in audio can significantly boost the performance of AI audio recognition systems, sometimes by as much as 30%. This points to the crucial importance of good sound quality for real-world implementation.
There are also some critical points to consider. Many AI models rely on publicly available audio datasets, raising concerns regarding data privacy and usage rights, particularly as these models become integrated into commercial products. The rise of real-time audio processing tools has spurred demand in areas like online gaming and live streaming, where seamless AI integration enhances the overall user experience. But this growth also presents the risk of a saturated market, forcing companies to focus on creating unique features to stand out. Otherwise, there's a risk of becoming outdated as competition intensifies.
With the global deployment of smart devices continuing at a rapid pace, the need for robust audio recognition will only increase. This creates a fiercely competitive landscape, driving companies to invest heavily in research and development to stay ahead of the curve. It's clear that the future of AI audio recognition holds both exciting opportunities and critical considerations for researchers and developers alike.
The Evolution of AI-Powered Sound Effects Removal A 2024 Analysis - Automated Audio Categorization Transforms Editing Workflows
Automated audio categorization is reshaping how audio editing is done, allowing creators to focus on their content rather than getting bogged down in the technical details. Software like Adobe Premiere Pro now includes tools that automatically recognize different audio elements like dialogue, music, or sound effects, offering specific controls tailored to each category. This helps streamline the editing process considerably. Similarly, tools designed for post-production, such as Auphonic, are increasingly automated and able to simplify tasks like removing unwanted silence, enhancing audio quality with less manual intervention. This automation, driven by recent advancements in deep learning, leads to more accurate audio classification, but questions remain about the adaptability of traditional classification methods to handle the diverse audio tasks encountered in different workflows. While these tools show promise, the industry grapples with balancing the accessibility of these systems with the substantial resources required to run them. This is a major factor in determining how these innovations impact the broader field of audio editing going forward.
Automated audio categorization is reshaping how we approach audio editing. By utilizing multiple neural network layers, these systems are becoming increasingly adept at differentiating between overlapping sounds—a significant improvement over traditional methods, especially helpful in sound design and music production. The ability to analyze and categorize audio in real-time is another exciting development. Editors can now receive immediate feedback, allowing them to adapt and refine audio during the editing process, a feat previously unimaginable.
There's a growing emphasis on contextual awareness in these systems. By considering surrounding sounds and past data, the categorization process becomes more accurate, benefiting the overall workflow. Furthermore, some models are adopting adaptive learning principles, continually refining their ability to categorize audio based on user feedback and interactions. This continuous improvement over time promises to boost the precision of these systems.
The integration of multiple data modalities is another interesting area. Combining audio categorization with video and text can create a richer editing experience. Editors can gain a more complete understanding of the audio-visual context, ensuring audio choices effectively enhance the overall narrative. Tools like latent semantic analysis are also being applied. This allows the models to identify deeper meanings and relationships within the audio itself, going beyond simply tagging sounds to achieving a more profound understanding of their context within a piece of media.
The applications of automated audio categorization extend beyond traditional media. Healthcare is using these tools to monitor patient vocalizations, suggesting its adaptability across different fields. But alongside these advancements come challenges. The training of these models requires massive amounts of audio data, which can be a barrier to entry for new developers. Acquiring high-quality, diverse datasets is often a major hurdle.
The datasets used for training can also introduce biases into the system's analysis. This concern arises in critical fields like criminal justice and surveillance, where the fairness and reliability of AI-driven audio analysis are crucial. And, while automated audio categorization increases efficiency, it also raises questions about job displacement within the audio editing field. It's important to consider how to balance the potential benefits of automation with the need for human creativity and quality control in the creative process. Striking that balance will be crucial as this technology matures.
The Evolution of AI-Powered Sound Effects Removal A 2024 Analysis - Edge AI Expands Its Footprint in Audio Applications
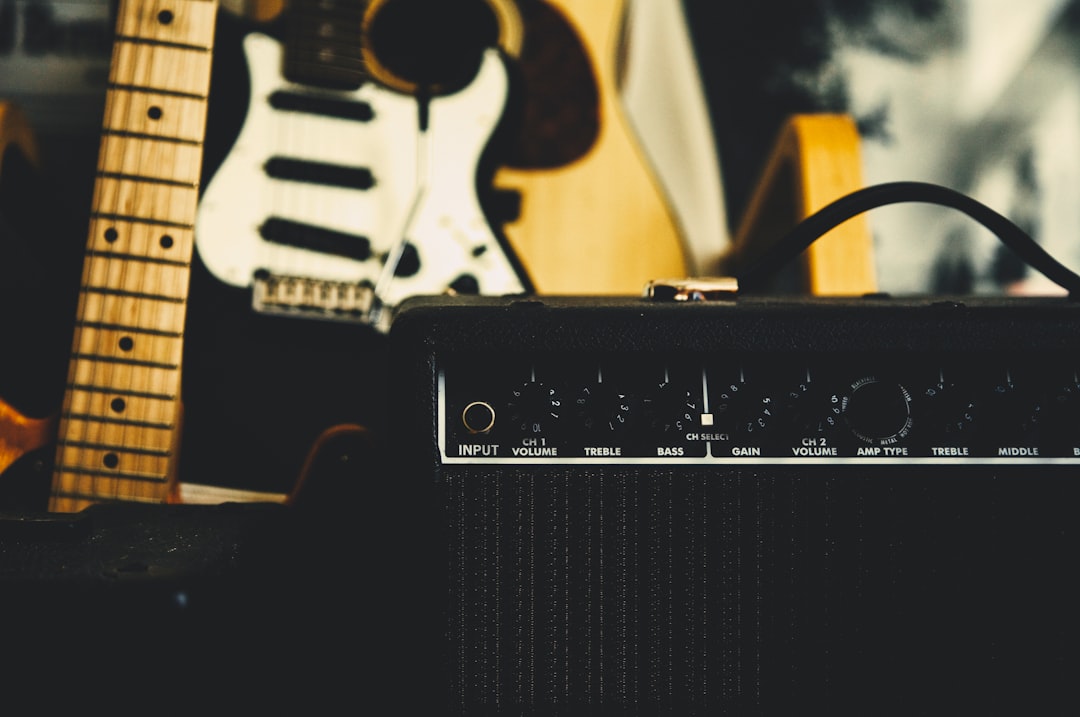
The integration of Edge AI into audio applications is gaining momentum, bringing processing power closer to the source of audio data. This approach reduces dependence on cloud-based services, thereby improving data security and minimizing delays in real-time audio processing. This localized processing is crucial for applications needing quick response times and personalized experiences, such as those found in smart speakers or noise cancellation headphones. The development of increasingly sophisticated AI algorithms is allowing devices to perform complex audio tasks directly, without constant connection to a central server. This means devices can have more intelligence built-in, leading to improvements in features like sound effect removal or voice recognition. However, the increasing complexity of these algorithms also raises questions about resource demands and whether all users will have access to these advancements. While Edge AI promises a more efficient and user-centered audio landscape, its wider adoption will need to address potential obstacles related to device hardware limitations and energy consumption.
The integration of edge AI into audio applications is leading to some fascinating possibilities, particularly concerning real-time processing and responsiveness. One of the most noteworthy aspects is the ability to handle audio data with minimal delay. This is a crucial aspect in scenarios like live audio streaming or interactive gaming, where even minor delays can have a disruptive effect on user experience. It's also interesting how edge AI allows systems to dynamically adapt to the user's environment. For instance, noise cancellation features can automatically adjust based on the surrounding audio, resulting in better overall audio quality regardless of the conditions.
Edge AI's emphasis on local processing also brings about significant advantages regarding data privacy. Because the audio data isn't being transmitted to a remote server, the potential risks associated with data breaches are reduced, keeping sensitive audio information within the user's control. It's also notable that the adoption of edge AI isn't necessarily confined to high-performance devices. We're seeing increasing compatibility with more readily available and affordable hardware, opening the door for a wider range of devices to utilize advanced audio processing.
Further, edge AI can employ algorithms trained to enhance audio quality automatically. This is a significant step forward because it means that users can enjoy the benefits of professionally adjusted sound without needing a deep understanding of audio engineering. This increased accessibility, a trend seen in other areas like image editing, helps democratize audio production by removing many of the technical hurdles that might have previously limited users. This can be especially useful for creators in fields like music production or sound design who might not have access to high-end equipment or specialized knowledge.
The impact of edge AI on music production and sound design is a compelling area of research. The ability to generate more complex soundscapes with fewer resources can be a game changer. Edge AI can assist in creating new sound effects and help users streamline the creative process, from concept to final output.
Of course, the advantages of edge AI also come with certain limitations. While reducing latency is a benefit, it's still constrained by the computational capabilities of the devices themselves. Powerful audio algorithms might demand substantial computing resources, which could pose a challenge for devices with limited processing power. Edge AI algorithms are increasingly employing contextual awareness, adapting to different audio cues in the surrounding environment. This means systems can change their behavior in real time, differentiating between a noisy environment and a quiet setting, for example.
It's not just about entertainment. Edge AI's potential applications extend to fields like telecommunications and healthcare. For instance, we might see improved clarity in phone calls or the ability for doctors to leverage AI to analyze patient voice patterns for potential health indicators.
While the future of edge AI in audio applications remains bright, we must consider these inherent limitations and how they will shape its adoption and deployment. It's fascinating to see how the field continues to evolve and potentially revolutionize our everyday interactions with sound.
The Evolution of AI-Powered Sound Effects Removal A 2024 Analysis - User-Friendly Tools Democratize Sound Effects Removal
The ability to remove unwanted sound effects is becoming more accessible to a wider audience due to user-friendly tools. AI-driven applications, like those offered by Adobe and Meta, are simplifying audio manipulation. Adobe's updated AI features, for instance, give users the means to remove distracting background sounds and reverberations with minimal technical skill. Open-source initiatives like Meta's AudioCraft project further expand access by allowing users to create audio and music from straightforward text descriptions. While these developments are promising, there's ongoing debate about their lasting effect on the music industry. Some question the long-term impact on the value of traditional audio expertise and the importance of originality in music creation. The democratization of sound effect removal via these tools raises intriguing questions about the future landscape of sound design and audio production.
The increasing user-friendliness of sound effects removal tools is a notable outcome of AI advancements. We're witnessing a shift where these tools are becoming more intuitive, able to discern between desired audio and unwanted noise through sophisticated machine learning techniques. These algorithms analyze audio characteristics and adapt well to different sound environments, showing promise in enhancing audio clarity.
Historically, high-quality sound effect removal was a specialized skill requiring both expertise and expensive software. But now, with accessible tools, anyone can achieve professional results without needing extensive training. This accessibility is a democratizing force, widening participation in audio editing and sound design.
Many current tools also excel at real-time audio processing, which is a game-changer for applications like online gaming and live streaming. This instantaneous feedback loop allows for on-the-fly adjustments, refining sound and improving the user experience.
Furthermore, these tools are gaining the ability to understand the acoustic context of a recording. They can sense changes in the environment, such as a sudden increase in background noise, and adapt their parameters to maintain audio clarity. This contextual awareness is a step beyond basic noise reduction.
Some of these tools also employ adaptive learning methods. They gather insights from how users interact with the tools and use that data to improve future suggestions for sound modifications. This personalization tailors the user experience to individual workflows, leading to greater efficiency over time.
Interestingly, these tools are not just for musicians. Their applications extend into areas like healthcare, where they can enhance audio from patient consultations or help with voice analysis for diagnostic purposes. This cross-application potential is an encouraging indication of their versatility.
We are also observing a trend towards multimodal integration. Some advanced tools are starting to blend audio with visual cues from videos, allowing for sound adjustments that better align with the visual narrative. This leads to a more seamless and impactful audio-visual experience.
Another notable aspect is how AI is helping automate audio workflows, removing mundane tasks that previously consumed a significant amount of time. By automating tasks, these tools allow creators to spend more time on the creative elements of audio editing, ultimately shortening project completion times.
A critical area to consider is data security and privacy. Many of these tools favor local processing on the user's device, reducing cloud reliance and thus minimizing the risks associated with data breaches when dealing with sensitive audio.
However, despite their advantages, AI models in these tools can still be susceptible to bias, especially if the training datasets are not representative of diverse voices. This can affect performance across various speaker types, requiring creators to be aware of potential limitations in these technologies.
The Evolution of AI-Powered Sound Effects Removal A 2024 Analysis - Deep Learning Algorithms Enhance Sound Analysis Capabilities
Deep learning algorithms have substantially improved the capacity for analyzing sound, fundamentally altering how we perceive and manipulate audio data. These intricate algorithms enable more refined sound detection, paving the way for enhanced categorization and the ability to process complex audio environments in real time. This improvement is particularly evident in applications like healthcare, where deep learning methods are being utilized to analyze respiratory sounds for improved diagnostic accuracy. Furthermore, the connection between deep learning and audio recognition is driving the development of more intuitive automated tools. However, it's important to acknowledge the significant computational resources needed to execute these models and the rising concerns regarding data privacy. As this field progresses, resolving these issues will be vital for ensuring responsible advancement. The intersection of deep learning and sound analysis is generating a broad range of possibilities, hinting at a future brimming with potential in this constantly evolving field.
Deep learning algorithms are becoming increasingly sophisticated, allowing for a level of sound analysis previously unattainable. They can now distinguish fine details within sounds, like separating individual instruments in a complex mix in real-time, a task once needing meticulous manual manipulation. This ability is transforming how we handle background noise. Deep learning algorithms can adapt noise cancellation in response to changes in the surrounding environment. This means headphones or smart speakers can adjust their noise reduction dynamically during a conversation or while streaming audio without requiring user input.
Traditionally, audio processing methods struggled with handling overlapping sounds. Deep learning changes this, enabling improved separation and clarity in cluttered audio environments. This is invaluable in industries like post-production and broadcasting where audio fidelity is crucial. This granular level of sound processing is made possible by convolutional neural networks (CNNs). These networks mimic the human auditory system, showcasing the technology's potential to replicate biological hearing processes.
While impressive, these algorithms still face limitations in adapting to varied real-world scenarios. They might perform flawlessly in controlled settings but encounter challenges in situations where audio characteristics change significantly. The increasing reliance on AI for sound enhancement in commercial audio software also raises questions about the future role of traditional audio engineers. Their expertise, once vital for sophisticated audio manipulation, may become less central as AI systems handle more of these tasks.
Deep learning algorithms are extending beyond just removing noise and separating sounds. They've also shown promise in interpreting human emotions through voice analysis. This creates new applications in fields like customer service and therapy, where vocal nuances can provide valuable insights into emotional states. Some researchers are exploring the use of generative adversarial networks (GANs) to create synthetic audio that's virtually indistinguishable from real recordings. This suggests a future not just for removing sound but also for the creation of new sounds tailored to specific artistic purposes.
The impressive capabilities of these algorithms are tied to the vast quantities of training data needed to achieve optimal performance. However, this reliance on large datasets can introduce biases reflecting the data they were trained on. This is especially important in applications designed for diverse user groups, where fairness and accuracy are paramount. Efforts to enhance sound quality using deep learning highlight a crucial area of research: explainability. Understanding how these algorithms make decisions about sound processing is critical for establishing trust and transparency in AI audio applications.
Experience error-free AI audio transcription that's faster and cheaper than human transcription and includes speaker recognition by default! (Get started for free)
More Posts from transcribethis.io: