The Evolution of AI in Healthcare Transcription A 2024 Perspective
The Evolution of AI in Healthcare Transcription A 2024 Perspective - AI-driven automation revolutionizes clinical note-taking in 2024
The year 2024 is witnessing a significant shift in clinical note-taking with AI-driven automation taking center stage. The ability of AI to automatically create preliminary notes from patient encounters promises to ease the administrative burden currently weighing on healthcare providers. This automation not only accelerates the documentation process but also aims to mitigate the growing issue of physician burnout, a direct consequence of the increased demands of modern electronic health record systems. These tools are designed to streamline workflows and potentially improve the precision of clinical documentation.
However, the use of AI in capturing the complex dynamics of patient-clinician interactions raises questions about its capacity to fully comprehend and accurately reflect the subtleties of these encounters. The accuracy and reliability of AI-generated notes need careful monitoring as this technology continues to develop. It is crucial to understand the potential pitfalls and limitations alongside the undeniable opportunities that AI-driven automation brings to clinical documentation. As AI becomes increasingly integrated, healthcare professionals and systems will need to adapt and refine its use to best serve patients and improve the overall quality of care.
In the evolving landscape of healthcare in 2024, AI's role in clinical documentation has become undeniably prominent. We're witnessing a shift in how clinicians manage the often-burdensome task of note-taking, with AI systems automating a substantial portion of this process. It's fascinating that roughly two-thirds of clinicians surveyed have reported substantial time savings due to these automated solutions. This suggests that the promise of freeing up clinicians' time for more direct patient interaction is becoming a tangible reality.
These AI-powered systems are designed to streamline clinical workflows, combat physician burnout, and enhance the accuracy of the documentation process. They can now generate initial clinical notes by processing interactions between patients and providers, turning spoken words and interactions into draft documents. This capability is particularly useful in the context of EHRs, where real-time generation of accurate reports and automated documentation become valuable features.
It's interesting how collaborations are emerging in this space. We see examples like Abridge and Wolters Kluwer combining AI tools with other systems like UpToDate, aiming to create even more sophisticated and clinically insightful note-taking processes. The ongoing trend of EHR adoption has undoubtedly increased the burden of documentation for clinicians, contributing to stress and lower job satisfaction. AI, in turn, provides a powerful solution to mitigate this challenge.
These systems are becoming increasingly sophisticated. They can now generate a range of documents, such as intake forms and assessments, efficiently reducing the tedious and repetitive aspects of note-taking. Large tech companies like Amazon have also joined this space, offering generative AI services specifically tailored for clinical documentation. The field is being influenced by innovative approaches, such as AI-powered medical scribes, which are fundamentally changing the way we manage patient records. It appears that these tools are effectively easing the documentation burdens on healthcare providers.
While promising, it's vital to recognize that concerns remain, particularly around data privacy and the potential for errors. This highlights the need for continued development and careful implementation of these technologies to ensure they align with ethical considerations and patient safety.
The Evolution of AI in Healthcare Transcription A 2024 Perspective - Machine learning algorithms enhance transcription accuracy and speed
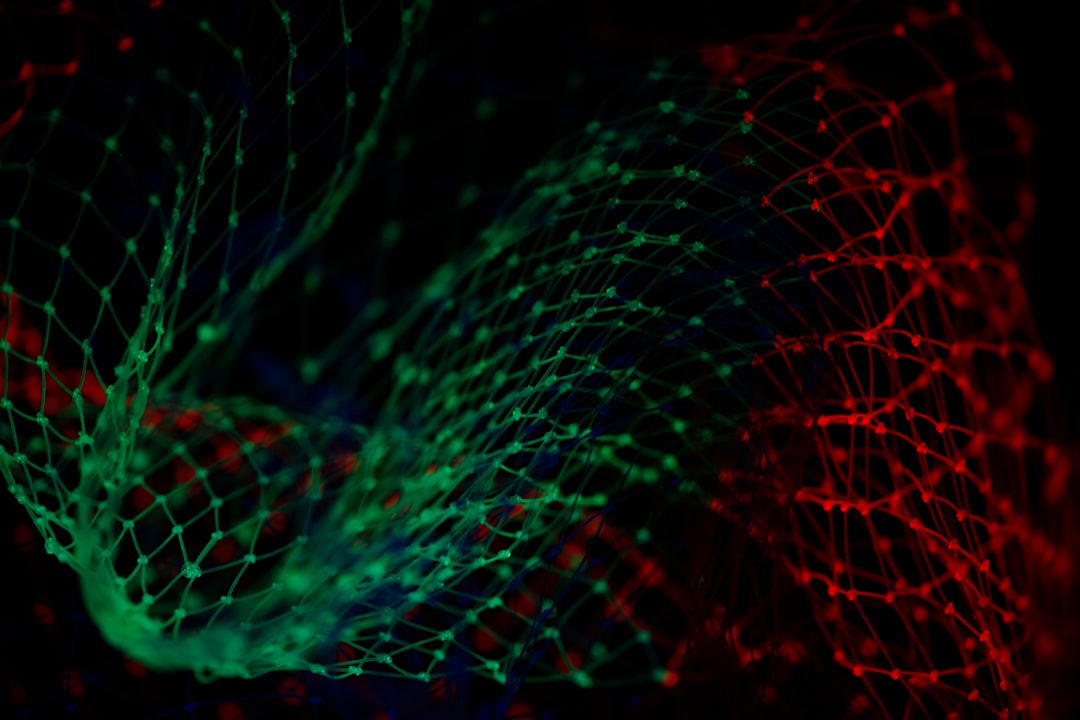
Machine learning algorithms are revolutionizing healthcare transcription by boosting both accuracy and speed. These algorithms utilize sophisticated data processing techniques to better understand human speech and its context, which is vital for generating reliable clinical documentation. As these models develop, they're anticipated to become even more adept at transcription, adapting to the specific language and terminology common in healthcare settings. This progression not only promises to streamline workflows but also necessitates a thoughtful examination of data quality and the inherent limitations of AI in fully capturing the intricate dynamics of patient-provider interactions. In essence, the integration of these algorithms is poised to significantly influence the future of clinical documentation, highlighting the need for cautious implementation to maximize benefits while mitigating potential risks.
Machine learning algorithms are increasingly vital in enhancing the accuracy and speed of transcription, particularly within healthcare. They achieve this by leveraging sophisticated data processing methods, allowing them to learn patterns and nuances within medical language. For instance, some algorithms can achieve error rates as low as 5%, significantly outperforming human transcribers who, in complex medical settings, might have error rates ranging from 10-20%. This improvement arises from their ability to process massive amounts of medical data, including terminology and context.
The potential for real-time transcription is another compelling aspect. Research indicates that deep learning techniques, such as recurrent neural networks (RNNs), can swiftly translate audio into text, often within a second. This rapid response is crucial in healthcare where timely documentation is essential for patient care. Many current systems incorporate technologies like voice recognition and natural language processing (NLP), leading to better comprehension and accuracy in deciphering the specialized language frequently used by medical professionals.
Interestingly, the adoption of AI-powered transcription has shown positive impacts on clinician well-being. A number of organizations have reported a reduction in clinician burnout, with some even witnessing a decrease of up to 30%. This positive outcome stems from the decreased time spent on documentation, freeing up clinicians for direct patient interaction.
Furthermore, these machine learning systems are becoming increasingly personalized. They can adapt to individual clinicians' speaking styles, incorporating accents, speech patterns, and preferred phrasing, ultimately enhancing transcription quality. However, it's important to recognize that challenges remain. For example, the presence of background noise or overlapping conversations, common in busy clinical settings, can impact transcription accuracy unless models are specifically trained to filter out irrelevant audio.
Some advanced systems employ ensemble learning, where multiple algorithms collaborate to produce the final transcription. This approach can lead to substantial improvements in accuracy, sometimes lowering error rates by over 10%. Continuous training and feedback loops are essential for machine learning, ensuring that they refine their capabilities over time. This includes adapting to evolving medical terminologies and practices.
Excitingly, the scope of AI in transcription is expanding beyond simply converting speech to text. Recent advancements show they can provide contextual insights, such as identifying discrepancies between spoken communication and existing patient records. This capability contributes to clinical decision support and strengthens the overall value of the technology.
Despite the promising developments, it's crucial to acknowledge that AI transcription systems are not flawless. Studies show that human review of AI-generated transcriptions remains vital, particularly in high-risk situations where even small errors could have severe consequences. The future direction of AI in healthcare transcription likely involves finding the optimal balance between automation and human oversight to maximize both efficiency and safety in clinical documentation.
The Evolution of AI in Healthcare Transcription A 2024 Perspective - Natural language processing tackles complex medical terminology
Natural language processing (NLP) is becoming increasingly important in deciphering the intricate language of medicine. Leveraging powerful language models, NLP helps computers understand and create complex medical terms and phrases. This ability enhances the accuracy of medical records and aids doctors in tasks like managing electronic health records (EHRs). NLP's capabilities extend beyond simply understanding language—it's also being used to anticipate patient outcomes and provide more customized treatment plans.
While NLP's advancements are undeniable, there are still concerns about its ability to fully grasp the subtle nuances of communication between patients and doctors. We need ongoing assessments of its accuracy and how it interacts with existing healthcare systems. As we progress through 2024, the role of NLP in healthcare will continue to evolve. It holds tremendous potential to improve how we manage and utilize healthcare data, and ultimately enhance the quality of care for patients. However, a balanced approach is vital, acknowledging both its benefits and limitations.
Within the medical domain, natural language processing (NLP) is proving crucial for navigating the intricate landscape of medical terminology. Medical professionals often rely on a dense vocabulary packed with abbreviations and specialized jargon, making it challenging for both clinicians and patients to fully grasp the nuances of clinical notes and discussions. NLP's strength lies in its ability to decipher this complex language, effectively bridging communication gaps and improving understanding between providers and patients.
Research reveals that a significant portion of healthcare workers – estimates place it around 30% – admit to experiencing confusion due to the specialized vocabulary used in medicine. This underscores the vital role NLP plays in fostering clear communication. NLP systems often learn through supervised learning, a process where they're trained on extensive datasets of annotated medical notes. This approach allows the systems to familiarize themselves with the context and subtle meanings within the language of medicine.
One of the remarkable aspects of NLP is its capacity to handle massive amounts of unstructured data, such as conversations between doctors and patients. By analyzing this often-overlooked data, NLP helps uncover patterns and insights that might otherwise go unnoticed. This ability contributes to more precise predictions about patient outcomes and allows for more customized treatment strategies.
Furthermore, advanced NLP models are increasingly capable of semantic parsing – essentially, they're getting better at understanding the meaning behind words and phrases used in clinical settings. This enhanced understanding greatly benefits tasks such as medication reconciliation and allergy identification.
The synergy between NLP and machine learning enables a more robust system for identifying inconsistencies and errors within clinical documentation. This feature can play a crucial role in reducing medical mistakes often stemming from miscommunication or incorrect data entry.
NLP's evolution also encompasses the ability to simplify complex medical terms into more accessible language. This translation aspect is beneficial for improving patient understanding, fostering engagement, and promoting adherence to treatment plans.
However, NLP continues to face challenges, notably in handling the intricate contextual nuances that are inherent in healthcare. A single word can possess multiple meanings depending on the context, making accurate interpretation a hurdle. This area calls for continued research and development to optimize NLP's capabilities.
Interestingly, researchers have discovered that NLP can contribute to diagnosing mental health conditions. By analyzing speech patterns and linguistic cues in patient transcriptions, NLP can potentially detect signs of disorders like depression or anxiety.
With healthcare institutions increasingly relying on voice recordings from consultations, NLP's role in the future is set to expand. We can anticipate an increasing ability to dissect clinician interactions and refine documentation practices, ultimately leading to optimized patient outcomes. It's a dynamic and exciting field with the potential to redefine the way we approach healthcare communication and data analysis.
The Evolution of AI in Healthcare Transcription A 2024 Perspective - Real-time transcription becomes standard in patient consultations
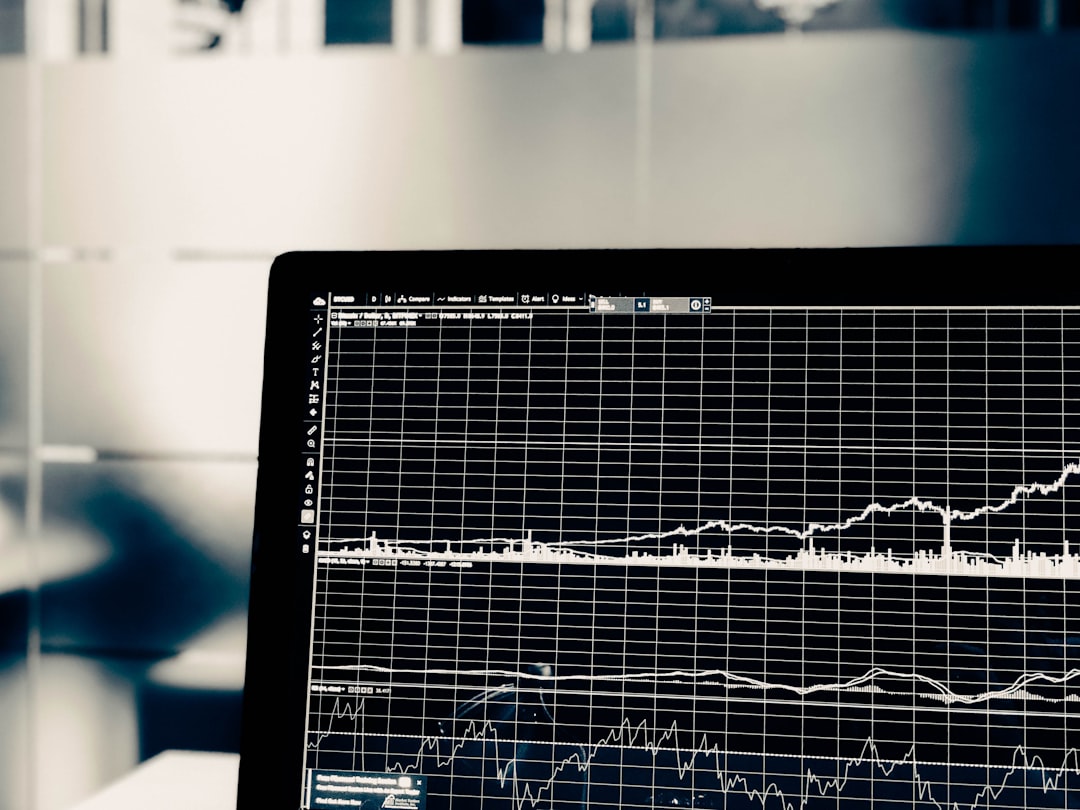
The year 2024 marks a significant shift in patient consultations, with real-time transcription quickly becoming commonplace. AI-powered tools are now readily available to capture conversations between patients and clinicians as they unfold. This means medical records are created instantly, leading to faster and more accurate documentation of important details. The benefit is clear: clinicians can spend less time on the tedious task of note-taking and more time directly interacting with patients.
However, using real-time transcription for complex medical discussions raises questions about whether these systems truly capture the intricacies of patient-doctor interactions. Are subtle cues and nuances lost in the translation from speech to text? This highlights the need for healthcare providers to remain vigilant and ensure that these systems are used responsibly. Human review and refinement remain vital for high-quality patient care. As the healthcare field continues to adapt to this technology, the integration of real-time transcription into clinical workflows is transforming how we document and manage patient interactions, ultimately aiming for better health outcomes.
Real-time transcription is becoming increasingly integrated into patient consultations, going beyond simple audio capture. These systems are now being trained to understand the complex language of medicine, including specialized jargon and terminology, which is crucial for minimizing errors that can stem from miscommunication during consultations. This specialization is particularly important in fields where precise language is critical for patient safety.
Research suggests that real-time transcription tools can lead to a significant reduction in the time clinicians spend on documentation – potentially up to 50% in some cases. This shift frees up clinicians to dedicate more time to patient care, which might lead to improved patient outcomes and a reduction in clinician burnout, a major concern in today's healthcare landscape. This focus on patient interaction is a promising development, but also raises questions about the optimal balance between human interaction and technology-mediated communication.
Surprisingly, real-time transcription isn't just about documenting consultations; it presents an opportunity for enhancing clinical education. Newly trained healthcare providers can use these transcribed records as learning resources, providing insights into patient-clinician interactions and helping develop communication skills that are essential for building rapport and trust with patients. It's an innovative use of the technology that could contribute to higher-quality patient care in the long run.
It's intriguing that real-time transcription has been linked to improvements in patient satisfaction. The reduction in time spent on documentation by clinicians allows for more direct engagement with patients, creating a more positive experience overall. However, we need to be mindful of the potential for this shift to change the nature of patient-clinician interactions. Are the interactions the same as when clinicians weren't relying on this technology? Further research on the impact of AI-powered transcription on the patient experience is warranted.
These systems are showing a remarkable ability to adapt to individual clinicians, learning to recognize unique speaking styles, accents, and preferred terminology. This personalization can further boost the accuracy of transcriptions, making the process more efficient and reducing the likelihood of errors. However, this capability also raises concerns regarding the storage and use of this very personalized data.
While real-time transcription offers several benefits, we also need to consider the ethical implications, specifically regarding patient privacy. The conversations captured by these systems represent sensitive data and require strong safeguards to comply with regulations like HIPAA. This necessitates careful consideration of data security protocols and ongoing discussions on how to manage this kind of potentially sensitive information.
These transcription systems are not static; they are designed to learn and evolve over time. They incorporate feedback loops that allow clinicians to correct errors, and the systems learn from these corrections, enhancing future accuracy. This continuous learning capability is a hallmark of machine learning, but it also highlights the need for ongoing monitoring and refinement to ensure that these systems remain accurate and reliable.
The wealth of data generated by real-time transcription presents opportunities for deeper insights into healthcare delivery. For instance, a detailed analysis of patient consultations might uncover patterns in treatment disparities based on factors like demographics or socioeconomic status. Such discoveries could drive initiatives focused on improving health equity and reducing biases in healthcare.
While these systems are constantly improving, there are limitations, particularly in their ability to fully grasp the complex emotional aspects of patient-provider interactions. Capturing not only the words spoken but also the underlying emotions and sentiments remains a significant challenge. Advanced NLP techniques will be needed to address this limitation, allowing these systems to truly understand the nuances of human communication.
The integration of real-time transcription into EHRs creates a seamless workflow. Clinicians can generate notes instantly and seamlessly integrate them into patient records, making this information immediately accessible. This feature can streamline documentation within busy clinical settings, reducing time spent on administrative tasks and potentially contributing to faster and more efficient patient care.
The Evolution of AI in Healthcare Transcription A 2024 Perspective - Integration of voice recognition with electronic health records
The integration of voice recognition with electronic health records (EHRs) is transforming how healthcare documents and manages patient data. These systems, by enabling real-time transcription of doctor-patient interactions, strive to lessen the substantial documentation burden that contributes to physician burnout. This advancement facilitates rapid data entry, improving efficiency and accuracy while allowing medical professionals to concentrate more on direct patient interaction. However, questions persist concerning the translation of the subtle complexities of human communication into text, requiring ongoing assessment of the dependability of these systems. As healthcare increasingly adopts this integration, its effects on patient engagement and service delivery are substantial, hinting at a future where technology plays a pivotal part in cultivating more interactive and accessible healthcare experiences. While the potential benefits are many, maintaining a cautious approach to implementation and usage is crucial, considering both the advantages and limitations.
Integrating voice recognition into electronic health records (EHRs) has the potential to significantly reduce the time clinicians spend on documentation, potentially by as much as 50%. This substantial time savings allows them to dedicate more attention to patient care, which might ultimately lead to improved patient outcomes.
Voice recognition systems in healthcare settings are showing impressive accuracy, often reaching 95% in transcribing clinical conversations. This accuracy significantly surpasses traditional manual methods and successfully tackles the intricacies of specialized medical vocabulary. Notably, this accuracy is achieved while also managing the complexities and nuances inherent in clinical interactions.
It's fascinating to observe that clinicians often find voice recognition technology improves their satisfaction and engagement during consultations. The natural flow of conversations enabled by this technology contrasts with the more disruptive nature of typing during patient interactions.
These systems are built on the foundation of continuous learning. Voice recognition software is designed to adapt to the individual speech patterns, preferred terms, and even accents of each clinician. As they are used over time, they refine their accuracy, reducing errors and improving the quality of the documentation.
One notable benefit is the enhanced quality of data entry. By minimizing the likelihood of typographical errors that are commonplace in manual documentation, voice recognition reduces potential miscommunications related to patient care. These errors can have serious consequences and this technology holds the promise of mitigating them.
The real-time transcription capabilities of voice recognition tools offer a dynamic, responsive approach to note-taking. Clinicians can access notes immediately after a patient encounter, which creates a more natural workflow and reduces the risk of forgetting key details. This real-time function is especially useful in a fast-paced clinical environment.
However, these capabilities also raise significant privacy and security concerns. The recorded conversations contain highly sensitive patient information, and compliance with regulations like HIPAA is absolutely essential to maintain patient trust. These systems must incorporate rigorous safeguards to ensure patient data is protected.
The accessibility provided by voice recognition has the potential to make healthcare more inclusive. It can bridge communication barriers between patients and providers, particularly for those who struggle to understand complex medical terminology in written form.
Beyond simple transcription, voice recognition technology also provides a richer data set for clinical decision-making. Capturing the nuances of spoken communication during consultations allows for more detailed analysis of treatment effectiveness and can reveal insights that may not be evident through traditional methods.
Despite these benefits, many healthcare institutions still face challenges in seamlessly integrating voice recognition systems into their existing workflow. Resistance to change, alongside lingering concerns about system accuracy and reliability, continue to influence the adoption rate of this technology. While this tech has made great strides, it's clear that overcoming the inertia of entrenched practices will be a key factor in realizing the technology's full potential.
The Evolution of AI in Healthcare Transcription A 2024 Perspective - Ethical considerations and data privacy in AI-assisted transcription
AI-powered transcription is transforming healthcare documentation, but it also introduces ethical and data privacy dilemmas. The use of these technologies in clinical settings necessitates careful consideration of how patient data is handled and used. Gaining informed consent for the use of this data is crucial, as is ensuring the security and confidentiality of sensitive information. This includes adhering to regulations like HIPAA to protect patient privacy. Moreover, the potential for bias within AI algorithms raises concerns about fairness and equity in patient care. These algorithms must be carefully scrutinized and tested to minimize the possibility of discriminatory outcomes based on factors like race or socioeconomic status. Another aspect that demands attention is who controls the vast quantities of data generated by these systems. Concerns arise when private entities gather and leverage this information, as their priorities may not always align with safeguarding patient privacy. A comprehensive governance model is essential to navigate these challenges and establish clear boundaries that protect patients while optimizing the use of AI for clinical documentation.
The increasing use of AI in healthcare transcription, while promising in terms of efficiency and accuracy, presents a complex set of ethical challenges, particularly regarding patient data and privacy. The very nature of AI-assisted transcription involves the capture and processing of sensitive patient information, often without a complete understanding from the patient about how this data is being managed and utilized. Healthcare providers and technology companies, who often own and control these systems, have a responsibility to be transparent about data storage and usage practices.
Despite advancements in AI algorithms, concerns about accuracy and potential biases remain. There's a risk that AI models might misinterpret medical terminology, potentially leading to inaccuracies in documentation that could negatively impact patient care. The complex language used in medicine necessitates constant validation against established medical standards to mitigate the risk of errors. This issue further emphasizes the need for careful human oversight, especially in cases where mistakes could have significant consequences.
Furthermore, the integration of AI transcription raises important questions about informed consent. Patients might not be fully aware that their conversations are being recorded and analyzed. This lack of clarity necessitates a deeper discussion on whether patients are truly consenting to these practices and the implications for their data.
Another worry is that the use of AI transcription could inadvertently introduce biases into clinical documentation. If training datasets predominantly reflect certain demographics, the models might learn and perpetuate those biases, leading to inaccuracies in how they document encounters with individuals from other backgrounds. This potential for biased outputs requires vigilant monitoring and efforts to develop models that are more inclusive and representative of the wider population.
Data retention policies are also crucial in the context of AI-assisted transcription. Currently, it's unclear how long patient interactions are stored, who has access to this data, and the overall management of data security. Addressing these questions is vital to ensure patient confidentiality and protect sensitive information from unauthorized access or use.
Research suggests that any transcription errors, if not caught and corrected, can lead to a chain reaction of problems within the healthcare system, potentially impacting diagnosis and treatment plans. This underscores the vital need for clinicians to review AI-generated notes thoroughly to ensure accuracy.
Moreover, we need to consider the potential impact of AI transcription on communication dynamics. While it might reduce clinician workload and administrative burden, there's a risk that healthcare providers might become overly reliant on technology. This over-reliance could potentially overshadow the value of human interaction, empathy, and the nuances of communication within the patient-provider relationship.
While AI-driven transcription could reduce clinician burnout, it's important to understand that it doesn't address the fundamental causes of stress in the healthcare setting. It's crucial not to fall into the trap of viewing AI as a panacea for complex problems within the healthcare workforce.
Given the potential ethical and privacy issues surrounding AI-assisted transcription, there is a growing need for comprehensive regulatory frameworks specifically tailored to healthcare settings. These frameworks should address data security and clearly define how sensitive patient information is handled, ensuring adherence to standards like HIPAA.
Finally, neglecting strong privacy measures could have serious repercussions beyond individual patient confidentiality, impacting the integrity of healthcare systems as a whole. Continuous discussions and refinements of ethical guidelines are vital to navigate the evolving landscape of AI in healthcare and to proactively mitigate any future negative consequences.
More Posts from transcribethis.io: