Experience error-free AI audio transcription that's faster and cheaper than human transcription and includes speaker recognition by default! (Get started for free)
Step-by-Step Guide Translating Videos Using AI-Powered Tools in 2024
Step-by-Step Guide Translating Videos Using AI-Powered Tools in 2024 - Selecting the Right AI-Powered Translation Tool for Your Needs
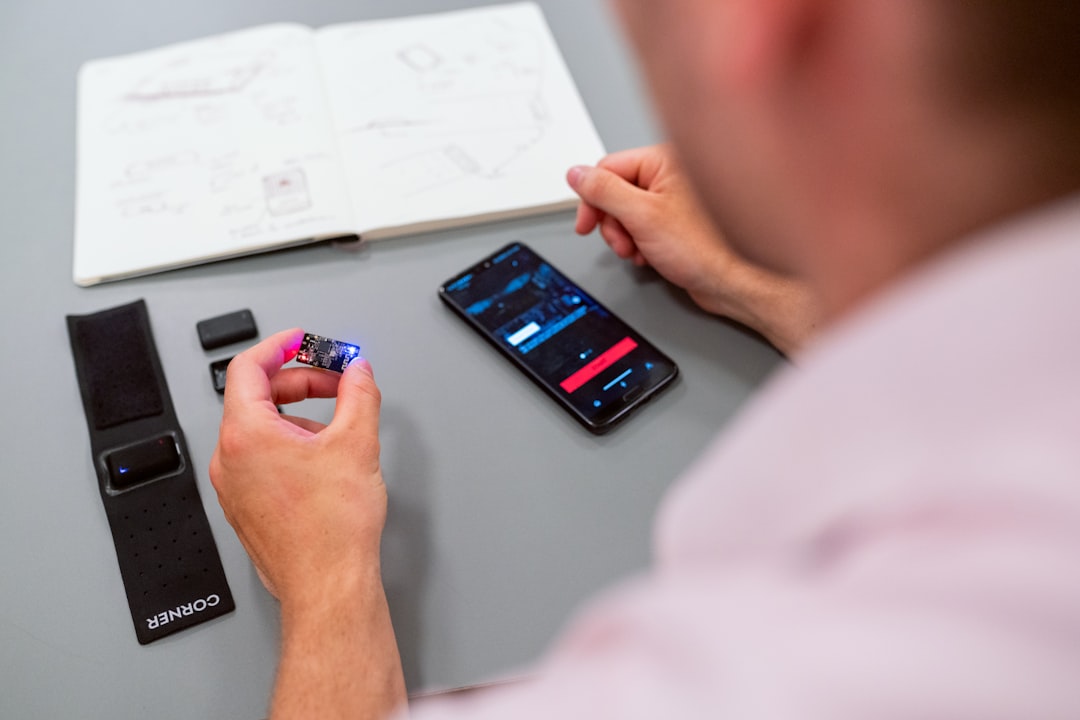
Choosing the appropriate AI-powered translation tool is a critical step in ensuring your video translations are accurate and effective. Factors like the complexity of your project, the languages involved, and desired level of nuance should guide your decision. Some tools, like DeepL or QuillBot, are specifically designed for high-quality translations, focusing on preserving the original meaning and context. On the other hand, tools like Google Translate excel in offering wide language support and ease of use, making them suitable for simpler projects or quick translations.
For businesses and larger projects with more complex needs, there are AI-powered translation platforms such as Transifex and Text United, which offer robust management tools specifically designed for translation workflows. These solutions can handle localization projects across many languages, integrate with existing systems, and streamline the entire process.
The selection of the right AI translation tool is crucial. A poorly chosen tool might result in inaccurate translations, hindering audience engagement and impacting the overall quality of your video's message. Carefully evaluate your requirements and test different tools before committing to one to ensure alignment with your objectives.
The landscape of AI-powered translation tools is quite dynamic, with a growing number of options available. Tools like QuillBot, introduced relatively recently, have gained attention for their translation capabilities. ChatGPT, known for its broader generative AI abilities, is another prominent example, offering more nuanced translations. DeepL has earned praise for its straightforward interface and the quality of its output, while Google Translate remains a popular option due to its versatility and free availability.
Microsoft Translator, on the other hand, caters more towards larger organizations due to its integration features. Transifex, a comprehensive platform, is geared towards managing localization processes in a more holistic way. Text United is a contender focused on providing scalable solutions, and Localize AI and CopyAI offer translation capabilities along with content generation features.
It's interesting to note that businesses, especially those in retail, are increasingly realizing the importance of choosing the right AI translation tool to reach diverse markets. The ideal tool will depend on various factors, with each tool excelling in certain aspects and falling short in others. While we've made great strides in machine translation, finding the perfect balance between automated efficiency and human oversight remains an ongoing challenge. It’s fascinating to observe how these tools are shaping the future of communication and bridging language barriers in various contexts.
Step-by-Step Guide Translating Videos Using AI-Powered Tools in 2024 - Preparing Your Video File for AI Translation
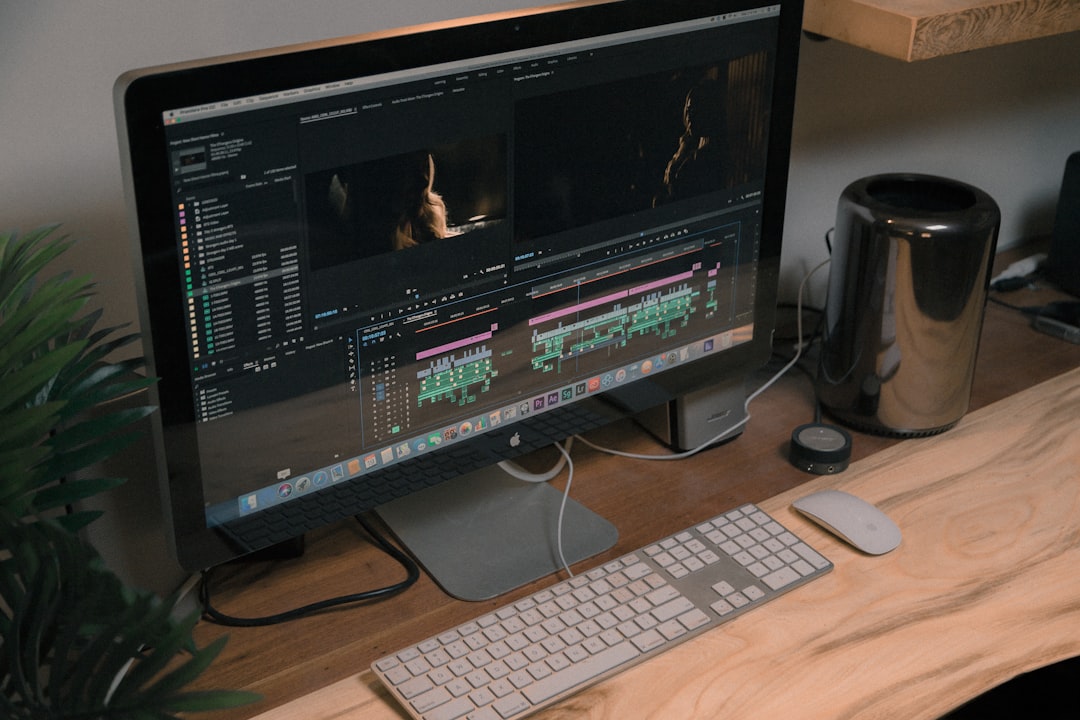
Before you can leverage AI to translate your video, you need to prepare it for the process. This starts with creating a transcript of the audio, which can be generated automatically or done by hand. The accuracy of this initial transcript is key to a successful translation. Once you have the transcript, you'll upload your video file to the chosen AI translation platform. The platform will likely allow you to select from a broad range of languages for your translation. It's crucial to ensure your video file is in a format that's compatible with the platform to avoid complications. This preparation step is often overlooked but is crucial for achieving high-quality, accurate translations and seamlessly integrating translated subtitles or captions into the video, improving efficiency and overall results. While AI tools are capable of producing translations, the initial groundwork of prepping your video can significantly impact their ability to perform well.
When preparing video content for AI translation, it's important to be mindful of several aspects that can impact the quality of the outcome. The video's format and compression method can play a crucial role. Highly compressed formats like MP4 might lose subtle vocal nuances, making it difficult for the AI to accurately understand and translate the speech.
Furthermore, rapid or overlapping speech can pose a challenge to the language model, leading to misinterpretations. AI models rely on context to deliver accurate translations, so fast-paced dialogue or complex sentences can make it harder for them to grasp the intended meaning. Similarly, background noise and audio quality issues can interfere with accurate transcription. Tools that are generally quite effective at transcribing audio might struggle with noisy recordings, which can affect the reliability of the output text.
Interestingly, providing initial subtitles alongside the video can be helpful. This allows for a more structured text representation that improves the quality of the AI training data, leading to potentially better translations. It's important to acknowledge that some languages might prove more challenging than others for AI translation due to nuances in syntax or idiomatic expressions. Tonal languages like Mandarin, for example, present unique difficulties due to the significance of tone shifts in conveying meaning.
Timestamps play a critical role in the proper synchronization of translated subtitles. Inconsistent or missing timestamps can lead to subtitles being out of sync with the spoken audio, potentially causing a jarring viewing experience for the audience. For very long videos, the character limits imposed by most AI translation tools can be a limitation. Dividing the video into smaller segments might be a viable way to ensure comprehensive translation.
It's encouraging that some tools use machine learning techniques to improve over time by incorporating user feedback. Essentially, the more data they process related to similar videos, the better their translation capabilities can become. This suggests that future AI translations can potentially benefit from this iterative process of refinement. However, video content that includes specific cultural references or niche jargon can present difficulties. AI models may lack the contextual understanding to accurately translate these, so supplying a glossary or other supporting information might be necessary.
Finally, the metadata associated with your video file can surprisingly impact the translation process. Providing well-organized metadata, including keywords and relevant descriptions, can be helpful for the AI tools to categorise and translate the content more accurately. This can ultimately lead to a more consistent and meaningful translation of your video.
Step-by-Step Guide Translating Videos Using AI-Powered Tools in 2024 - Generating an Accurate Transcript Using Automatic Speech Recognition
Automatic Speech Recognition (ASR) is a crucial starting point for creating transcripts, essentially converting spoken audio into written text. This step forms the foundation for any AI-powered translation process we're discussing. While ASR can achieve impressive accuracy, it often retains aspects of natural speech, such as hesitations and grammatical errors, which can affect how easy the text is to read. To counter this, techniques like n-best list rescoring and inverse text normalization are used to refine and improve the overall quality of the transcript output. Despite advancements, some difficulties remain, especially when dealing with diverse accents or speech that is impacted by background noise, which can sometimes limit the accuracy of the generated transcript. As ASR technologies continue to mature, finding the right combination of automated processing and human review will likely be key to producing transcripts that are consistently reliable and user-friendly.
1. **Context is King**: Automatic Speech Recognition (ASR) systems are quite reliant on understanding the context of what's being said. If someone uses vague language or specialized terms, even very sophisticated models can struggle. The resulting transcript might miss the mark on the intended meaning.
2. **Noise is a Problem**: The accuracy of ASR can really take a hit in places with lots of background noise. If there are sounds around or people talking over each other, it's tough for the model to separate the main speaker's voice. This can create errors in the final text.
3. **Accents and Dialects**: The effectiveness of ASR varies a lot based on the accents and dialects being spoken. A system trained mostly on standard English might struggle with regional variations. This means that tailoring these systems to specific languages and regional pronunciations is crucial.
4. **Who's Talking?**: Many ASR systems are still figuring out how to tell different speakers apart during a conversation. If people don't speak in ways that make it clear who's who or if they talk over each other, the model may get confused and assign words incorrectly, which messes with the accuracy of the transcript.
5. **Tone Matters**: In languages like Mandarin, the tone of voice makes a huge difference in the meaning of words. ASR models might miss these subtle shifts, leading to errors that impact the accuracy of the transcript. It's a unique challenge for this technology.
6. **The Real-Time Challenge**: Doing speech recognition in real-time adds another layer of complexity. Systems need to be both fast and accurate, which can sometimes lead to compromises in the quality of the transcription as they prioritize speed over more thorough processing.
7. **Learning from Mistakes**: ASR systems often learn and improve over time by using feedback from users. Systems that take into account the corrections people make can gradually get better at transcribing things accurately in the future. It really shows how these technologies are always evolving.
8. **Limits to Length**: Many ASR tools have built-in limitations on how much audio they can process at once. This often forces users to break up longer recordings into smaller chunks, which could make it harder to maintain a consistent flow in the resulting transcripts.
9. **Emotions in Speech**: It can be tough for ASR to accurately transcribe speech when someone is really emphasizing certain words or using strong vocal inflections, since those can change the meaning. This highlights that transcribing meaning, not just the words, remains a big challenge.
10. **Structure Helps**: Giving a system more structured data, like timestamps and who's speaking at what point, can make a big difference in the accuracy of ASR outputs. It allows the system to place parts of the transcript in the right context within the audio, which results in more coherent and useful transcripts.
Step-by-Step Guide Translating Videos Using AI-Powered Tools in 2024 - Applying Machine Translation to Your Video Transcript
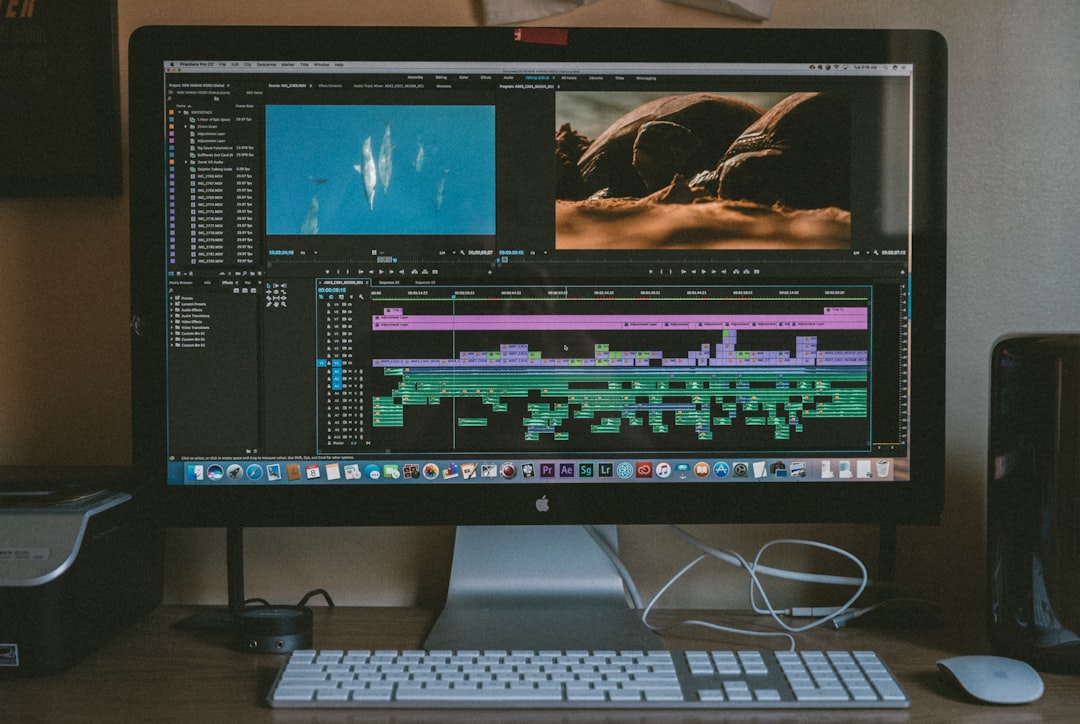
Once you have a video transcript, whether created manually or automatically, you can apply machine translation to it. This step involves feeding the transcript into an AI-powered translation tool to convert it into your desired language. However, it's crucial to understand that these automated tools might not always capture subtle nuances, like cultural references or specific terminology. This means that relying solely on machine translation could potentially lead to inaccuracies, especially when dealing with idiomatic expressions or industry-specific jargon.
To achieve more accurate translations, particularly when handling complex topics, human review is valuable. An individual with knowledge of both the source and target language can ensure the translation conveys the intended message effectively and maintains cultural sensitivity. In essence, striking a balance between utilizing powerful AI tools and incorporating human expertise for context and cultural awareness is paramount to a good result. The ultimate goal is to translate the content accurately while ensuring it aligns with the intended meaning and context for the target audience. While there are significant improvements in machine translation, the technology's limitations remind us that it can still fall short in complex situations.
Applying machine translation to a video transcript, while offering a fast route to multilingual content, comes with its own set of intriguing challenges. While these tools can handle a wide range of languages, the accuracy can vary greatly depending on the specific language pairs involved. For example, translating from English to a language with vastly different grammatical structures might lead to a less accurate result compared to translations between languages that share more similarities.
One recurring issue is the difficulty of grasping subtle contextual meanings or cultural nuances. These tools often stumble when dealing with idioms or culturally specific terms, potentially leading to awkward or unintended translations. It's a reminder that relying purely on automation can sometimes create misunderstandings if not closely monitored by someone familiar with the content and languages.
Another aspect to consider is the relationship between the speed and the quality of the translation. While AI can churn out translations quickly, they often sacrifice some level of detail or nuance in the process. Research shows that slower, more careful translations usually lead to higher-quality results, implying that a balance is needed when relying on these tools. This isn't simply about speed, but about finding a suitable process where speed and accuracy aren't mutually exclusive.
Furthermore, regional differences can also create issues. ASR, the technology that converts audio to text, tends to vary in accuracy depending on the particular dialect or accent being spoken. If an ASR system is primarily trained on standard language variations, it might struggle with colloquialisms or regional jargon, underscoring the importance of training specific models for the different ways people speak.
This is even more pronounced for tonal languages, such as Mandarin, where slight changes in tone can entirely alter the meaning of a word. Current translation tools are still somewhat limited in their ability to handle these subtleties, which highlights a key area for further development in this field.
Interestingly, many machine translation tools are continually learning and improving through user feedback. This iterative approach gradually refines their ability to generate more accurate translations over time. However, there's still a bit of a lag before we see immediate and drastic improvements, highlighting the ongoing need for continuous refinement in these tools.
Another practical limitation comes in the form of character or length restrictions built into many tools. This forces users to break up longer transcripts into smaller pieces, potentially disrupting the flow or context of the overall translation.
Content containing cultural references or specialized terms can also prove challenging, as the tools may lack the proper background knowledge to interpret them accurately. This is where a glossary or background information can be particularly helpful in guiding the translation process.
Lastly, the organization of the metadata can surprisingly affect the overall accuracy. Including relevant keywords and descriptions can help the AI system categorize and interpret the content more effectively, potentially leading to better overall results. The quality of the underlying data and how it's structured really can affect the final translated product, illustrating that even the preparation phase of the video is relevant to the outcome.
In conclusion, while AI-powered tools for translating video transcripts offer a valuable and fast approach to multilingual content, it's crucial to be aware of their limitations. It seems the future of this area is going to involve a careful balancing act between the capabilities of these tools and the critical role that humans can play in ensuring that translation is both accurate and culturally sensitive.
Step-by-Step Guide Translating Videos Using AI-Powered Tools in 2024 - Synchronizing Translated Subtitles with Video Timeline
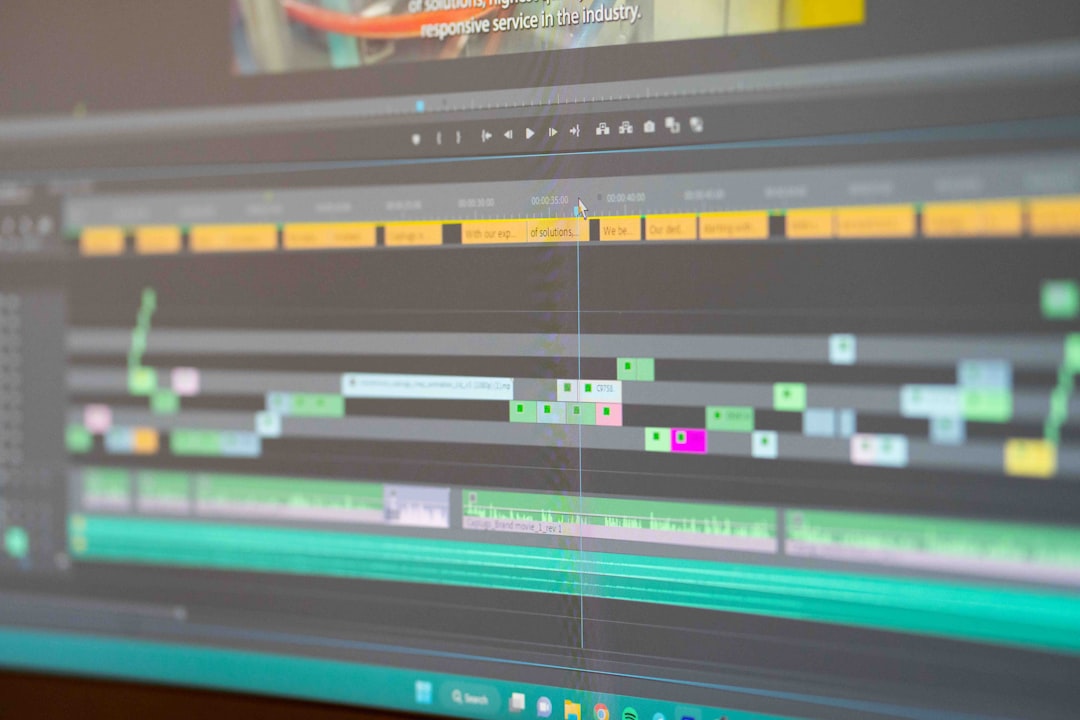
Ensuring translated subtitles align perfectly with a video's timeline is crucial for a smooth and engaging viewing experience. This synchronization process involves carefully adjusting subtitle timings to match the corresponding audio or visual elements. Various tools, like VEED or VLC Media Player, provide ways to manually adjust subtitle placement and timing, allowing for fine-tuning. However, achieving perfect synchronization can be a complex task. This is particularly true when the translated content doesn't match the original timing precisely, or if the original video had inconsistent or missing timestamps to begin with. In the age of AI-powered translation tools, striking a balance between automation and human review becomes important. By carefully addressing these synchronization challenges, we can improve the overall quality of video translations and ensure that the audience has a positive viewing experience. While AI can help with initial translation and synchronization, it's clear that human oversight and intervention are sometimes required to resolve these complexities.
Ensuring translated subtitles align precisely with a video's timeline is a surprisingly intricate task. Each subtitle line requires accurate timestamps to avoid disrupting the viewer's experience. Even a slight delay can make the viewing experience jarring, highlighting the importance of carefully managing the timecodes within subtitle files.
One challenge arises from the variability in how people speak. We tend to speak at an average rate, but pauses, interruptions, or overlapping speech can make maintaining subtitle synchronization difficult. This is particularly true when dealing with spontaneous conversation where the pacing is not predictable.
Many subtitle formats, like SRT files, limit the number of characters per line for easy readability. This can make translating certain phrases or concepts into a new language tricky. We often need to carefully choose our words and even shorten phrases to fit within these constraints without losing the meaning for the viewer.
Further complicating matters are the unique grammatical structures of different languages. Word order can vary considerably, which can make it tricky to keep the subtitles in sync with the original audio, especially when dealing with languages with significantly different grammatical structures.
Moreover, subtitles often require adapting the meaning of phrases rather than just translating word for word, especially when dealing with idioms or slang. Keeping the essence of the original dialogue while adapting for a new culture or language can be difficult, but crucial for keeping viewers engaged.
Another factor to consider is the relationship between subtitles and visual content. Ideally, subtitles shouldn't cover up important parts of the video. We need to position them thoughtfully to avoid disrupting the viewers' understanding of the visuals in the video.
The tools we use to synchronize subtitles are also a factor. There's a range of options available, from free tools to more specialized professional software. Each tool has its own approach to timing adjustments, which directly impacts both how efficiently we can align subtitles and the resulting accuracy.
We also need to consider the average person's reading speed. Subtitles that are too fast or too slow can be difficult to follow, highlighting the importance of correctly adjusting timing.
It's also interesting that we need to think about the emotional tone of the speech and try to match it with the subtitles. Quick, snappy subtitles can help convey humor or urgency, while slower, more deliberate ones can communicate seriousness. If we want to preserve the emotional impact of the original dialogue, we need to consider how we time our subtitles.
It's encouraging to note that many of the synchronization tools utilize machine learning to improve over time. As more users engage with them and provide feedback on synchronization errors, the underlying algorithms learn to better handle these tasks, offering promise for future advancements.
While the field of automatic subtitle synchronization is progressing, the intricacies involved demonstrate the continuous need for innovation and careful development in this area.
Step-by-Step Guide Translating Videos Using AI-Powered Tools in 2024 - Fine-Tuning and Proofreading the AI-Generated Translation
After generating a translation using AI tools, the next important step is fine-tuning and proofreading the output to ensure quality and accuracy. AI models, despite their advancements, often need adjustments to perform optimally for specific video contexts and nuances. Fine-tuning involves refining the AI's understanding through training data or feedback, which can improve its ability to handle complex language, cultural references, and specific terminology. However, even with this, AI sometimes struggles with translating idioms or capturing the intended tone of the original language.
Because of this, human involvement remains crucial. A knowledgeable person, fluent in both the original and target languages, can review the translation and ensure it effectively conveys the intended meaning and preserves cultural context. Striking a balance between leveraging the efficiency of AI translation and the insightful review of a human is essential. This process is necessary to polish the final translation, improving its clarity, accuracy, and alignment with the original intent. Through this collaborative approach, video translations become more effective, avoiding potential misinterpretations that can arise from relying solely on machine-generated text.
1. **Nuanced Language and Context**: Fine-tuning often revolves around capturing the subtle differences in language, like dialects and idiomatic expressions, that automated systems frequently miss. Ensuring the cultural context isn't lost in the translation process often requires a human touch.
2. **Language-Specific Hurdles**: Certain languages inherently present more difficulties due to their grammatical structure or common expressions. For instance, translating from English to a language with a vastly different grammatical makeup like Finnish might result in a less accurate outcome compared to languages that share a more similar structure, like German.
3. **The Speed-Accuracy Trade-off**: The demand for quick translation output can sometimes negatively impact the quality. Research indicates that more careful translation tends to produce more accurate results, highlighting the need to balance speed with thoroughness.
4. **Audio Quality's Influence**: The original audio's quality significantly influences how well the machine translation performs. Poor audio, filled with background noise, can lead to transcription inaccuracies that are further amplified during automatic translation.
5. **Capturing Emotional Context**: Evaluating tone and emotional nuances in speech can be tricky. AI systems often struggle to capture emotional subtleties, emphasizing the need for human review and refinement of the translation to maintain the video's intended tone.
6. **AI's Iterative Learning**: Many contemporary AI translation tools use feedback from human corrections to improve their future output. This ongoing learning process showcases the dynamic nature of AI, but also highlights the need for human reviewers to spot errors that AI systems may not yet recognize.
7. **The Subtitle Character Limit**: Subtitle synchronization is limited by character restrictions, which can lead to shortened translations. The challenge lies in summarizing the meaning efficiently without losing vital information, highlighting the importance of careful word choice.
8. **The Temporal Nature of Speech**: Natural speech often includes irregularities, like interruptions and variable pacing. These irregularities create a challenge when aligning translated subtitles, necessitating detailed adjustments to synchronize timing accurately.
9. **The Value of Metadata**: Interestingly, well-organized metadata can significantly enhance the translation process by providing context that assists AI tools in categorizing and understanding video content more precisely.
10. **Human Oversight: A Necessity**: While AI tools automate a large portion of the translation process, human oversight is still crucial to evaluate the accuracy and cultural appropriateness of the translations, particularly when dealing with specialized vocabulary.
Experience error-free AI audio transcription that's faster and cheaper than human transcription and includes speaker recognition by default! (Get started for free)
More Posts from transcribethis.io: