Experience error-free AI audio transcription that's faster and cheaper than human transcription and includes speaker recognition by default! (Get started for free)
Sociotechnical Perspectives Bridging AI Development with Societal Impact in 2024
Sociotechnical Perspectives Bridging AI Development with Societal Impact in 2024 - AI Ethics Boards Collaborate with Community Leaders on Responsible Development
In the evolving landscape of artificial intelligence, AI ethics boards are actively engaging with community leaders to navigate the intricate social and ethical questions surrounding AI development. This shift underscores the need to connect the developers of these technologies with the individuals and groups directly impacted by them. By fostering dialogue and incorporating community perspectives, these collaborations aim to ensure AI systems are designed with a focus on equity, fairness, and transparency. The goal is to bridge the divide between technological advancements and the values and concerns of society.
The rapid pace of AI progress highlights the urgency of developing a robust framework for responsible development. These collaborative efforts strive to achieve this by incorporating community insights into the earliest stages of AI system design and implementation. This emphasis on community engagement recognizes that the equitable integration of AI into society requires careful consideration of its impact on various communities. Essentially, it emphasizes the crucial role of community input in building a future where AI serves societal needs and upholds shared values of fairness and justice.
In numerous regions, AI ethics boards are increasingly playing a vital role in policy conversations. This surge in attention underscores a growing awareness of the potential for AI to have far-reaching impacts on society, both positive and negative. This realization is prompting greater engagement with communities during AI development, acknowledging that local experiences and viewpoints shape how these technologies are received and used.
Research points towards a strong correlation between involving community members in the decision-making process and fostering greater transparency in AI development. This increased openness can help to mitigate the skepticism that often accompanies novel technological advancements. Interestingly, it appears that companies proactively integrating ethics boards into their operations experience fewer instances of public criticism and regulatory challenges, highlighting a clear practical benefit of incorporating ethics into AI projects.
The shift in perspective suggests that collaborations between developers and community leaders are crucial not only for complying with regulations but also for creating innovations aligned with societal values. Public forums and consultation initiatives, especially those that seek input from underrepresented groups, are becoming key methods for capturing insightful viewpoints that traditional AI development models might overlook. The composition of ethics boards themselves has also evolved. They now often include experts from a variety of backgrounds, ranging from sociology to legal domains, leading to more comprehensive AI guidelines that weave technical specifications with broader societal implications.
Emerging evidence indicates that neglecting community engagement in AI projects poses a heightened risk of facing legal hurdles or adverse public relations situations for businesses. Establishing open communication channels between AI engineers and community groups helps in the development of ethical standards that are flexible and adaptable to real-world effects. This stands in contrast to rigid, static guidelines that may not adequately address unforeseen consequences. Furthermore, many organizations are harnessing community feedback to create best practices for AI implementation, suggesting a broader shift towards more adaptable and responsive project management methods in the tech world.
Sociotechnical Perspectives Bridging AI Development with Societal Impact in 2024 - Integrating Human Factors in AI Governance Frameworks
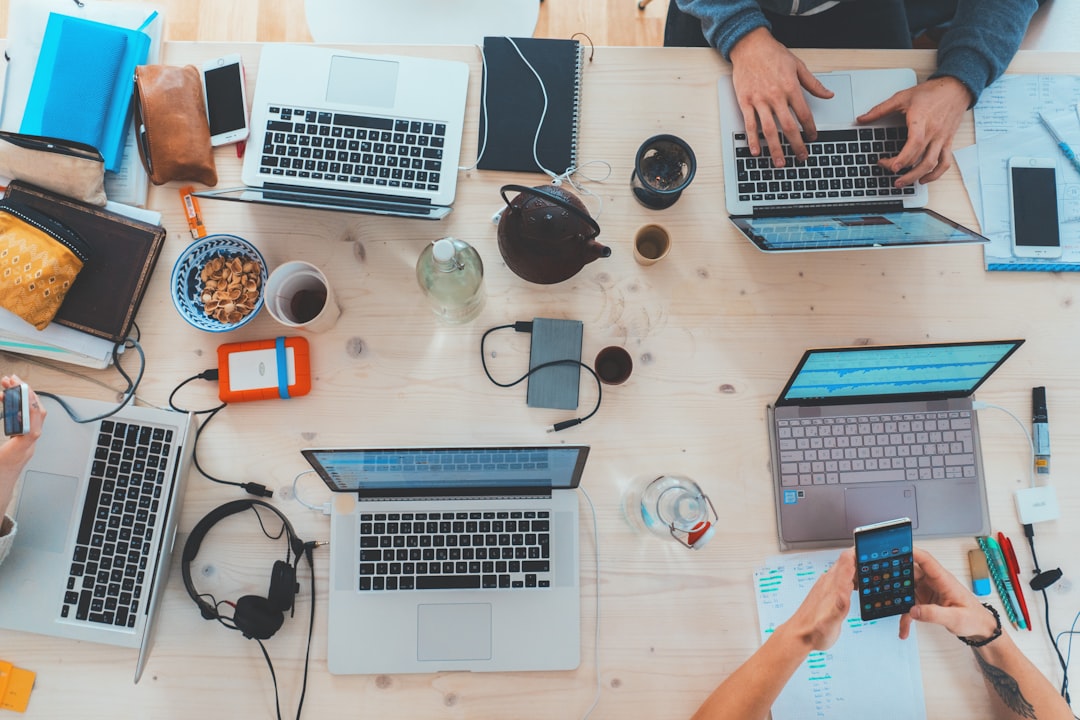
Integrating human factors into the governance of AI systems is crucial for navigating the complex relationship between technology, society, and ethical considerations. This involves recognizing that AI, while offering potential benefits, can also exacerbate existing societal biases and inequalities if not carefully managed. A key aspect is understanding how AI systems interact with the social world, necessitating a collaborative effort among policymakers, AI developers, and the communities affected by these technologies.
As AI becomes more deeply embedded in our daily lives, the need for a sociotechnical approach to governance becomes even more apparent. This approach emphasizes the importance of considering the broader societal context within which AI is developed and deployed. This includes recognizing the diverse ways individuals and communities will interact with these systems, and the potential for unforeseen consequences.
To address this challenge effectively, AI governance frameworks must incorporate insights from the humanities and social sciences. This interdisciplinary perspective helps ensure that ethical considerations are prioritized throughout the AI development lifecycle. Moreover, it facilitates a more nuanced understanding of the social impacts of AI, leading to greater transparency, accountability, and ultimately, the development of AI systems that are truly aligned with societal values and human needs. This human-centered approach ensures that the focus remains on building a future where AI empowers and benefits all of society, not just a select few.
Considering human factors within AI governance frameworks can significantly improve how people interact with and use these systems. This is particularly crucial in safety-critical fields like healthcare and aviation, where user errors, a major cause of technology malfunctions according to research, can be minimized through user-centered design.
Research suggests that users are more receptive to AI in critical applications, such as autonomous vehicles, when their ethical worries and expectations are addressed early in the design process. This suggests a growing understanding that AI needs to align with human values to be accepted.
Involving diverse teams that include behavioral scientists and psychologists during AI development can lead to a better user experience, improving the likelihood that people will adopt and trust new technologies. This interdisciplinary approach recognizes that human behavior is a key factor in determining AI's success.
However, neglecting human factors in AI governance can lead to systems that disproportionately impact vulnerable groups. Studies have shown that marginalized communities often face more difficulties using poorly designed systems. This highlights the need for a more equitable approach to AI development.
We're starting to see more emphasis on understanding cognitive biases in how humans make decisions. This is essential for designing AI that can effectively support human operators, especially in high-pressure scenarios. It's increasingly apparent that AI systems need to account for how people think and react.
Incorporating human factors not only addresses ethical concerns but can also make AI projects more financially efficient. Companies prioritizing usability often see improvements in productivity and lower training costs. There's a clear link between good human-AI interaction and increased efficiency.
Interestingly, AI governance structures that prioritize human factors have been linked to better compliance with data protection laws. This might be because human-centered approaches often emphasize transparency and user consent. This is a counterintuitive and insightful relationship worthy of further research.
By incorporating psychological principles into AI design, we can reduce resistance to adopting new technologies. Systems that match human behaviors and motivations tend to lead to more positive user experiences. It's not enough to just build AI; it needs to be designed to fit with how people naturally interact with the world.
Studies show that companies involving users in feedback loops during AI development can minimize bias in AI algorithms. This leads to more fair outcomes across different demographics, demonstrating the benefit of a more inclusive design process.
Finally, evidence is growing that integrating ergonomic principles into AI design can enhance the physical quality of human-AI interaction. This makes technology more accessible to people with varying abilities, creating a more inclusive environment. By designing for all users, we can create AI that truly benefits everyone.
Sociotechnical Perspectives Bridging AI Development with Societal Impact in 2024 - Organizations Implement Sociotechnical Approaches to Translate AI Principles into Practice
Organizations are increasingly recognizing the need to align AI development with societal values and are turning to sociotechnical approaches to achieve this. These approaches integrate human and social considerations into the design and implementation of AI systems, ensuring that a diversity of viewpoints are factored in from the beginning. This is vital as AI increasingly influences key decision-making processes impacting daily life, from traffic flow to resource allocation. Understanding how different communities perceive and are affected by AI is critical, revealing the need to move beyond purely technical considerations when crafting AI governance frameworks. A sociotechnical lens emphasizes the importance of equitable and responsible AI deployment, leading to systems that are more aligned with community needs and values. This shift towards more holistic AI governance helps build a foundation for AI systems that benefit all members of society, not just a select few.
Organizations are increasingly realizing the importance of incorporating a sociotechnical lens into their AI development and deployment processes. This means going beyond just the technical aspects of AI and considering the broader social context in which these technologies operate. We're seeing a growing number of examples where organizations are striving to translate abstract AI principles, like fairness and transparency, into tangible, practical steps. It's fascinating to observe how this is manifesting in different ways.
One interesting observation is that many organizations find that fostering a culture of open dialogue around AI ethics leads to more engaged and collaborative work environments. It seems that getting teams to think critically about the social implications of their work can actually boost team spirit and encourage a sense of shared responsibility. This suggests that incorporating sociotechnical considerations might not just be the 'right thing to do', but also a path to a more positive workplace experience.
Another intriguing aspect is that by taking a more holistic view that considers both the technical and social factors, organizations appear to be improving their ability to anticipate and manage challenges. A sociotechnical approach seems to equip them with a more comprehensive understanding of the potential impacts of AI systems, enabling them to be more adaptable when things don't go as planned. It's as if they are better prepared to handle the inevitable bumps in the road during complex projects.
Furthermore, we're seeing some organizations build sociotechnical principles into their training programs. The idea is that by embedding these concepts into how employees learn and develop, they can make better, more informed decisions, especially when navigating the complicated ethical dilemmas that can arise within complex AI systems. Early evidence suggests that this approach can lead to faster and more accurate decision-making within these contexts.
The application of sociotechnical approaches is not just limited to internal organizational dynamics. Organizations embracing this perspective are also finding themselves better prepared to engage with external stakeholders. This translates to stronger, longer-lasting partnerships with community groups, industry partners, and other stakeholders. This increased trust and collaboration is crucial in fostering a shared understanding of the benefits and risks associated with AI technologies. It also promotes a greater sense of collective ownership over the future of AI.
There's also a growing body of evidence to suggest that when organizations involve diverse teams and stakeholders in the AI development process, they are better equipped to avoid unintended biases in AI outputs. This is a critical aspect of responsible AI development. The insight here is that having diverse perspectives involved in the design process seems to help reduce the risk of AI systems perpetuating or exacerbating existing inequalities.
A curious and potentially powerful outcome of embracing sociotechnical approaches is that organizations appear to be better positioned to navigate the increasingly complex regulatory landscape surrounding AI. We are seeing fewer instances of regulatory pushback and smoother compliance processes in companies taking a sociotechnical approach. This indicates that regulatory bodies may be recognizing the value of this holistic approach.
Interestingly, a sociotechnical focus seems to lead to greater innovation, with some organizations seeing a notable increase in the number of new products and services developed. Perhaps it's because this broader perspective allows them to see new opportunities and potential applications for AI that might otherwise be overlooked.
In addition, organizations that integrate sociotechnical approaches seem to achieve cost savings through increased efficiency in project management and resource allocation. This makes sense, as it suggests that anticipating and mitigating risks upfront can lead to reduced costs in the long run.
Finally, an area that merits further study is the observation that organizations adopting sociotechnical principles appear to respond more quickly to unforeseen challenges and crises. They seem to have the capacity to adapt strategies faster than their counterparts who don't focus on sociotechnical aspects.
While still a developing field, the evidence suggests that sociotechnical approaches offer a valuable pathway for organizations seeking to both leverage the potential of AI while minimizing the risks and ensuring a more equitable and beneficial integration of AI into society. It's a reminder that technology, especially powerful technologies like AI, cannot be considered in isolation from the human world and the values that shape it.
Sociotechnical Perspectives Bridging AI Development with Societal Impact in 2024 - AI's Transformative Impact on Energy and Transportation Systems
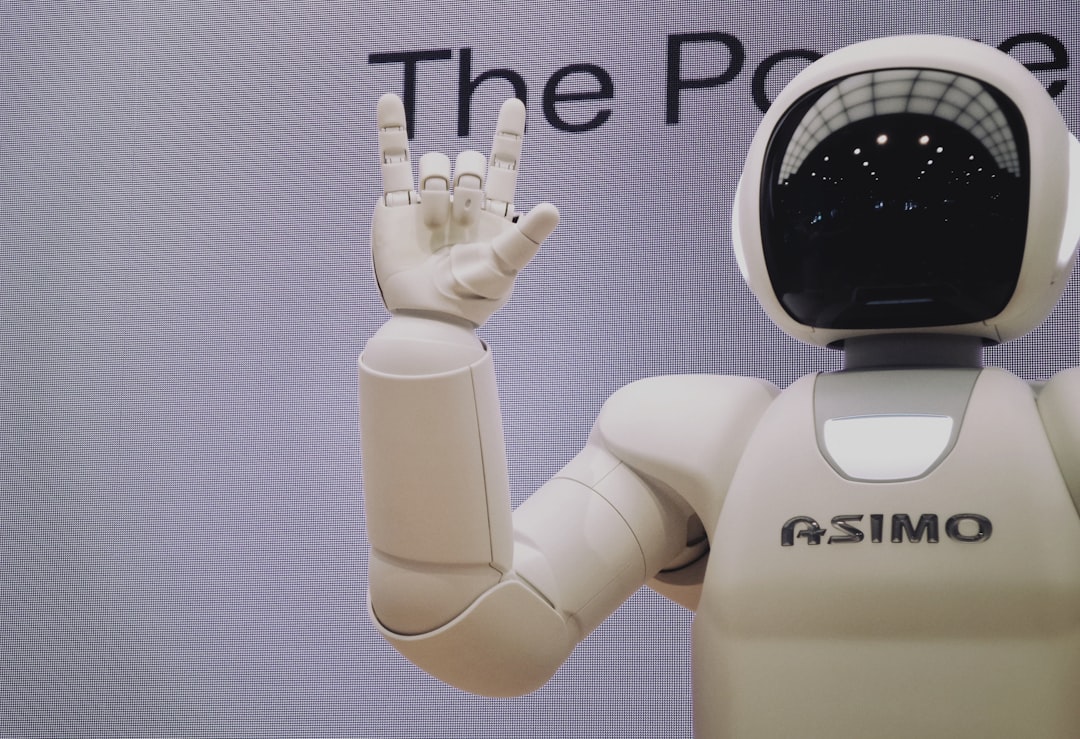
AI's emergence within energy and transportation systems is prompting a fundamental shift in how we approach these crucial aspects of modern life. AI's ability to optimize energy distribution and manage traffic flow is leading to increased efficiency and, potentially, greater sustainability. The focus on reducing carbon emissions and improving resource management is encouraging a reassessment of how we power and move through our world. However, the rapid integration of AI also presents challenges, particularly the problem of "dataset shift." This occurs when real-world conditions differ from the data AI systems were trained on, potentially leading to flawed decision-making within these critical systems. The example of autonomous vehicles clearly demonstrates how AI is not just a technological enhancement but a catalyst for fundamental change in transportation and urban planning. Given the significant implications for society, it is vital to adopt a sociotechnical perspective. This means considering the social and ethical dimensions of AI's impact alongside its technical capabilities. Only through a holistic understanding can we ensure that the benefits of AI are widely shared and do not worsen existing inequalities.
Considering AI's influence on established systems like energy and transportation offers a fascinating lens through which to understand its broader societal impact. A key challenge for AI in transportation is the concept of "dataset shift." This occurs when real-world situations change in ways not captured in the AI's initial training data, which can impact its decision-making. This is particularly relevant in dynamic environments like traffic flow or weather patterns.
The potential development of artificial general intelligence, or AI that mirrors human cognitive abilities, raises intriguing and, at times, unsettling questions about its impact on society. It's crucial to approach this potential with a degree of caution and a clear focus on the ethical implications. However, AI also has considerable promise for improving sustainability. It can be a critical tool for reducing carbon emissions in industries such as transportation and for aiding energy transitions to cleaner sources.
The emergence of autonomous vehicles is a significant example of AI's transformative capabilities in the transportation sector. These systems push the boundaries of vehicle autonomy, fundamentally reshaping our understanding of transportation networks and their management. Further, incorporating AI into smart cities has the potential to address various urban challenges, such as optimizing traffic flow and resource management, potentially increasing urban sustainability.
It's important to recognize the sociotechnical aspects of AI development. We need robust discussions that bridge the gap between AI technology and society. This requires considering the roles and responsibilities of those involved in AI's development, and it emphasizes the necessity of a collaborative approach among researchers, developers, policymakers, and the communities that will be affected.
Thinking about how AI influences fundamental societal institutions like law and democracy highlights the ethical considerations that must guide its development and implementation. There's an ongoing need to ensure that AI development and deployment align with fundamental societal values.
Ongoing research continuously explores how AI can be implemented in urban environments. This exploration focuses on addressing various facets of city living, while also acknowledging the challenges associated with integrating such advanced technology into complex and existing infrastructure. Researchers are constantly working to identify how AI can effectively integrate into urban systems to offer real-world benefits while mitigating potential downsides.
Sociotechnical Perspectives Bridging AI Development with Societal Impact in 2024 - Machine Learning Algorithms Reshape Public Resource Allocation Strategies
Machine learning algorithms are increasingly reshaping how public resources are allocated, impacting everything from traffic flow to social services. These algorithms can analyze vast amounts of data to identify patterns and optimize resource distribution, potentially aligning public services with specific community needs more effectively. This shift offers opportunities to improve efficiency and effectiveness in resource management, but it also raises important questions. One key concern is the potential for these algorithms to inadvertently perpetuate or exacerbate existing societal biases. Ensuring fairness and equity in the application of these technologies is crucial. Additionally, there's a growing emphasis on the need for transparency and explainability in how these algorithms work and how decisions are made. To build trust and ensure the public good is prioritized, it is vital to foster collaboration between developers, policymakers, and communities. Ultimately, a balanced approach is needed – one that integrates technical advancements with ethical considerations and a deep understanding of how these systems affect people's lives. Successfully integrating machine learning into public resource allocation requires a sociotechnical approach that considers both the technical potential and the social impact of these tools.
Machine learning algorithms are showing promise in refining how public resources are distributed. By analyzing large datasets and identifying previously unseen trends, governments can potentially allocate funds more strategically based on real-time information and predictions, leading to potentially more efficient spending. It's been noted that public agencies using these algorithms report an increase in citizen satisfaction with services, presumably because these systems are better able to understand and address community needs.
Research suggests machine learning algorithms might even help mitigate biases in resource distribution. By examining historical data, these systems can potentially expose existing inequities and alert decision-makers to make fairer choices. This technology also seems to offer cost savings, with organizations experiencing reduced operational expenses through optimized resource allocation and waste reduction. However, a major concern remains that machine learning models can inadvertently amplify existing biases if not carefully monitored and adjusted, potentially worsening existing social inequalities.
Interestingly, in some cases, machine learning has sped up critical responses in public services, such as emergency services, by quickly recognizing and reacting to high-demand situations. This highlights a beneficial aspect of using these systems. But, we must acknowledge that many local governments may lack the necessary technical staff and knowledge to fully implement these algorithms. This creates a barrier between the technology's potential and practical implementation.
Some areas have integrated machine learning models with public feedback systems to increase participation. These initiatives have shown promising results in improving community-driven projects, highlighting the potential for enhancing democratic processes. Furthermore, machine learning algorithms could boost transparency in resource allocation by providing data-driven explanations for funding choices. This data-driven approach could increase trust and accountability.
An unexpected consequence of adopting machine learning for resource allocation is a shift towards a culture of innovation and collaboration within organizations. We're seeing increased interdisciplinary collaboration as people from different fields work together to address complex social issues, a possibly positive societal impact of implementing these AI algorithms. While there are challenges, like bias, expertise gaps, and the need for careful implementation, machine learning algorithms appear to be a powerful tool for improving public resource allocation, provided they are thoughtfully and responsibly deployed.
Sociotechnical Perspectives Bridging AI Development with Societal Impact in 2024 - Sociologists and AI Researchers Join Forces to Challenge Tech Narratives
In 2024, the field of artificial intelligence is witnessing a growing partnership between sociologists and AI researchers. This collaboration seeks to challenge common assumptions about technology by emphasizing the importance of a sociotechnical approach. By considering the social context in which AI is developed and deployed, this perspective underscores the need to incorporate diverse perspectives when examining the impact of AI on society. This collaboration aims to address issues like bias and inequity within AI systems, arguing that a more equitable and responsible framework for AI development is critical. Sociological insights are becoming more crucial in shaping AI policies that prioritize the needs of the broader society, ensuring that innovations in AI align with human values and actively involve community perspectives. Fostering a deeper understanding of how technology interacts with society through interdisciplinary efforts is essential for guiding conversations about the future of AI and its place in our daily lives.
The intersection of sociology and AI research is revealing valuable insights into the societal implications of artificial intelligence. Traditionally, AI development has often been focused on technical aspects, overlooking the complex social dynamics that influence how technology is used and perceived. By bringing sociologists into the fold, researchers are starting to uncover how ingrained biases can subtly influence AI systems and their outcomes. These biases aren't just about the data itself, but also reflect how humans make choices and interact with the world, something not always captured in standard AI modeling.
Moreover, this collaboration is pushing for a deeper understanding of how different groups within society interact with AI. Sociologists are providing a crucial lens to examine how AI impacts marginalized communities, highlighting disparities in access and benefit that might otherwise be overlooked in purely technical evaluations. Understanding these dynamics is becoming critical for creating AI systems that are equitable and just.
Researchers are also finding that sociology's focus on narrative and qualitative data can greatly improve communication around AI development. By employing narrative approaches, researchers are better able to explain how data is interpreted and decisions are made, bridging the gap between technical processes and public understanding. This is further reinforced by a shift towards quantifiable metrics for community engagement, allowing designers to better gauge social impact alongside technical performance.
This interdisciplinary dialogue is challenging some core assumptions within AI development. It's becoming clear that human behavior is far less predictable than many AI models assume, and that a greater understanding of social context is needed to build genuinely effective and user-friendly AI systems. Sociologists' contributions are extending into policy discussions as well, influencing recommendations for regulations that are more aware of technology's limitations and human variability.
Further, ethnographic methods are being embraced to observe and analyze real-world interactions with AI. This allows researchers to capture nuanced user experiences in natural settings, leading to a more accurate reflection of actual human needs within the technology's design. Intriguingly, this partnership has also highlighted the fact that resistance to AI adoption is often rooted in historical inequalities, highlighting the need for trust-building initiatives within AI rollout strategies. The end goal of these efforts is the creation of shared frameworks for analyzing sociotechnical interactions, frameworks that could be fundamental for constructing truly fair and beneficial AI systems that serve all of society, not just a select few. This type of systemic understanding is crucial for developing AI that truly meets the needs of people and communities.
Experience error-free AI audio transcription that's faster and cheaper than human transcription and includes speaker recognition by default! (Get started for free)
More Posts from transcribethis.io: