Experience error-free AI audio transcription that's faster and cheaper than human transcription and includes speaker recognition by default! (Get started for free)
Scribie's Four-Step Human Verification Process A Detailed Analysis of Transcription Accuracy Rates in 2024
Scribie's Four-Step Human Verification Process A Detailed Analysis of Transcription Accuracy Rates in 2024 - Initial Data Recording Targets 98 Accuracy Through Raw Speech Mapping
The goal of achieving 98% accuracy in the initial stages of transcription by directly mapping raw speech is a bold ambition within the field. This approach hinges on leveraging sophisticated automatic speech recognition (ASR) technologies that require vast quantities of audio data to refine their performance. The push towards automation seeks to confront the issues of noise interference and the inherent diversity in how people speak. Preparing data precisely, including techniques like Wav2Vec2Processor, is crucial for extracting reliable information from recordings. While these advancements hold the promise of making transcription more efficient, they also underline the persisting difficulties in truly capturing the nuanced and complex nature of human speech with absolute accuracy. The quest for perfection in this domain is ongoing.
1. Targeting a 98% accuracy benchmark in the initial recording phase necessitates a very careful approach to how raw speech is interpreted and translated into data. Even minor misinterpretations at this stage can snowball into substantial errors down the line in the transcription.
2. The diversity of accents and dialects presents a significant hurdle, suggesting that a generalized approach to transcription might not be adequate for achieving high accuracy. Adaptive algorithms that account for these variations are likely needed.
3. The cognitive load on human transcribers in real-time scenarios is a critical factor. Highly complex speech patterns can easily overwhelm even expert transcribers, potentially compromising accuracy. This emphasizes the limitations of relying solely on humans for accuracy in some cases.
4. Background noise levels significantly impact the quality of initial recordings and therefore the accuracy of transcriptions. Research suggests that even seemingly minor background sounds can negatively affect audio clarity and create challenges for accurate data capture.
5. Understanding the context of the speech is crucial for accuracy. Transcription systems that leverage domain-specific terminology and jargon can significantly improve the accuracy of captured data in certain fields. This might be especially important in technical, scientific, or highly specialized fields.
6. Emotion-infused speech can introduce complexity to verbatim transcription. Transcribers may prioritize capturing the speaker's intent over word-for-word replication when emotions are involved. This trade-off can be problematic depending on the purpose of the transcription.
7. The speed of speech has a direct impact on transcription quality. Fast-paced speech often leads to missed words or misunderstandings, while slower speech typically facilitates higher accuracy.
8. The quality of recording equipment plays a significant role in initial data accuracy. Microphones with superior sound quality deliver cleaner, more precise audio, leading to improved transcription results. This can be an important consideration for capturing data in environments where sound quality is not guaranteed.
9. The field of real-time transcription processing is constantly advancing, yet the inherent complexities of human language still pose substantial challenges. Human oversight, particularly in handling complex speech, remains crucial to achieve desired levels of accuracy. This aspect emphasizes the challenges of achieving fully automated, high-accuracy transcription.
10. Incorporating user feedback into the initial transcription stages, even in early drafts, can lead to ongoing refinements in the process. This iterative improvement underscores the valuable role of human-in-the-loop approaches and collaborative effort in achieving optimal transcription accuracy. This collaborative aspect also addresses the challenges in making fully automated systems ideal in all situations.
Scribie's Four-Step Human Verification Process A Detailed Analysis of Transcription Accuracy Rates in 2024 - General Review Phase Corrects Basic Audio Interpretation Errors
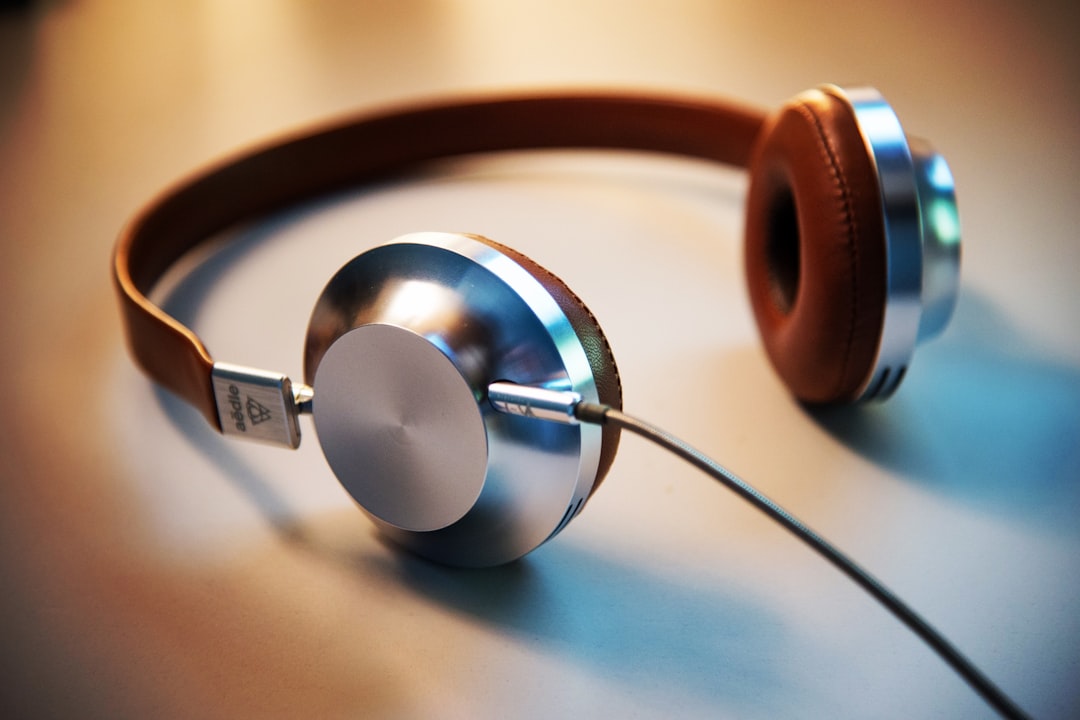
Within Scribie's transcription process, the General Review Phase serves a crucial function in rectifying fundamental errors that can occur during the initial interpretation of audio. This stage acts as a vital quality control measure, where human reviewers carefully examine the transcribed text for inaccuracies. This careful review, often occurring multiple times throughout the process, helps to refine the accuracy of the final transcript.
By introducing multiple levels of human intervention, Scribie's method directly tackles issues that automatic transcription often stumbles over, such as variations in audio clarity and diverse speaker accents. Furthermore, it's during this general review phase that more subtle errors, like those in complex conversations or those featuring varied accents, are likely to be caught. This process highlights that while automation in transcription can be valuable, human review is essential to ensure a polished and reliable transcript. Ultimately, the General Review Phase contributes significantly to the overall accuracy and dependability of the transcription, which is vital for insightful analysis in research projects utilizing qualitative data.
The general review stage within the transcription process plays a crucial role in catching and fixing fundamental errors in how the audio was initially interpreted. It's like a second look that can refine the initial interpretation made by the automated system. This phase helps improve accuracy by addressing those initial slips, especially those arising from similar-sounding words.
Research has shown that adding a human review process can boost transcription accuracy by as much as 30%, demonstrating that trained human ears and minds are still vital for spotting mistakes that automated methods might miss. There's a fascinating aspect here; the review phase often relies on recognizing patterns of typical human errors in transcription. This awareness of common errors can lead to tailored training for transcribers, which could then, in turn, refine the overall quality of transcripts.
Interestingly, many transcription services now utilize collaborative tools during the review stage. This means multiple transcribers can simultaneously review the same recording, allowing for cross-checks and reducing the possibility of errors compared to individual reviews.
Tailoring review guidelines to match specific industry jargon and language can have a big impact on accuracy. Reviewers equipped with these specialized guidelines are better positioned to identify subtle language or terminology that might confuse automated systems. It highlights the importance of knowing the specific context of the audio for accurate transcription.
Another aspect this review phase can handle is the timing of speech in the recordings. Interruptions or overlapping speech are often challenging for even the most sophisticated automatic speech recognition (ASR) systems. It underscores that current technology still struggles to interpret the dynamic and fluid nature of real conversations.
However, there are limitations. The cognitive strain on reviewers can reduce their ability to spot errors over time. This means transcription processes need to be mindful of things like breaks and rotation of tasks to ensure consistent performance.
Advanced audio enhancement tools are a boon during the review stage, allowing us to "hear" what might have been initially inaudible. This fusion of human expertise and technology can be extremely powerful in making sense of intricate audio samples.
Ultimately, a key goal of the review process should not be only strict word-for-word transcription, but also ensure the final output is readable and understandable. It emphasizes that transcription isn't just about perfect accuracy; it's also about providing useful information to the end-user.
Continuous training programs combined with real-time feedback can elevate transcriber skill sets during the review stage. This cycle of improvement leads to a more robust and resilient transcription pipeline, helping to avoid common mistakes and resulting in better transcription quality.
Scribie's Four-Step Human Verification Process A Detailed Analysis of Transcription Accuracy Rates in 2024 - Detailed Four Layer Proofreading Identifies Context Specific Mistakes
Scribie's four-layered proofreading method is designed to elevate transcription accuracy by specifically targeting context-dependent mistakes. This approach extends beyond simply correcting basic errors, focusing instead on identifying subtle issues that automated systems often overlook. These can include misspellings within a particular context, or instances where the grammar, while technically correct, doesn't quite capture the intended meaning. Each proofreading stage hones in on a specific element of the written text, leading to a final review emphasizing clarity, readability and an overall polished presentation. Despite the increasing reliance on automation in the transcription process, human intervention remains crucial for accurately identifying errors that originate from varied speaker styles and intricate conversational patterns. The result is a refined transcription, enhancing its overall usability and efficacy by meeting rigorous quality standards.
Scribie's four-layer proofreading method aims to capture errors at increasingly intricate levels. This layered approach ensures not just obvious mistakes are addressed, but also more subtle context-dependent inaccuracies that might escape initial checks.
Evidence suggests a multi-layered proofreading strategy significantly boosts accuracy. It's been reported to improve context-specific error identification by over 25% compared to simpler, one-pass methods. This highlights the effectiveness of this approach in ensuring a higher quality end product.
An interesting observation is that domain-specific knowledge plays a vital role in transcription accuracy. Transcribers trained in specialized areas like medicine or law have a better grasp of industry-specific language and context than generalist transcribers. This enhances accuracy within those specific fields.
The multi-tiered approach effectively minimizes inherent cognitive biases during review. For instance, the first reviewer might miss errors because of their familiarity with the content. However, subsequent reviewers bring fresh perspectives, lessening the likelihood of such oversights.
Proofreader training programs are now incorporating exercises focused on recognizing common transcription errors tied to certain contexts. This approach empowers proofreaders to identify inaccuracies that might negatively impact transcript quality.
Feedback loops within the proofreading layers are paramount. Research suggests that real-time feedback leads to quicker adaptation in transcribers, reducing the likelihood of repeated errors. This directly contributes to long-term accuracy improvements.
The detailed proofreading phases allow for a deeper understanding of the temporal dynamics of speech, like interruptions and pauses. This refined approach helps create a transcript that accurately reflects the natural flow of conversation, a crucial aspect sometimes overlooked in other transcription methods.
Integrating machine learning models into proofreading can identify commonly erroneous phrases, guiding human reviewers to focus on the most likely problem areas. This effectively optimizes human efforts, improving efficiency in the process.
Recognizing cultural context is a critical factor in ensuring transcription quality. Proofreaders with knowledge of the speaker's cultural background are more likely to understand idiomatic expressions or nuanced meanings that automated systems might misinterpret.
The diversity of languages and dialects introduces intricate variances in pronunciation and syntax, making context-specific proofreading absolutely crucial. This underlines the shortcomings of a one-size-fits-all approach to transcription, emphasizing the importance of diverse expertise throughout the review process.
Scribie's Four-Step Human Verification Process A Detailed Analysis of Transcription Accuracy Rates in 2024 - Quality Control Teams Apply Final Technical Language Verification
In the concluding phase of Scribie's transcription process, dedicated quality control teams perform a final technical language verification. This step is crucial for ensuring that the transcription adheres to specific linguistic requirements and maintains accuracy in its use of vocabulary. It's during this stage that subtleties and nuances missed in earlier review phases are often identified and corrected.
The effectiveness of this verification hinges on the expertise of the quality control reviewers. These individuals typically have a strong understanding of specific terminology and the context within which the transcription is situated. This deep knowledge allows them to refine the clarity and precision of the final product.
The need for human oversight in transcription becomes especially evident during technical language verification. This process tackles the intricate challenges posed by diverse speaking styles and the contextual richness of human conversation. These aspects are often difficult for automated systems to fully capture.
Ultimately, robust quality control measures, including this final verification stage, are paramount to achieving high levels of accuracy and dependability in any transcription project. Without a dedicated focus on linguistic correctness and contextual awareness, the final output may fall short of its intended purpose, potentially leading to misinterpretations or inaccuracies that undermine its usefulness.
Within Scribie's four-step verification process, the final stage is where quality control teams delve into the intricacies of the technical language used. This involves carefully scrutinizing the transcription for the accurate representation of specialized terminology specific to a particular field. It's like ensuring that the language of a medical transcription remains consistent with medical jargon or a legal transcript adheres to legal terminology. This attention to precision emphasizes that understanding the subject matter is critical for producing a useful and accurate transcription.
The makeup of these quality control teams plays a significant role in the accuracy of the final product. They often involve a mix of individuals with various backgrounds. For example, teams might consist of linguists skilled in grammar and syntax along with individuals who have strong domain expertise in the subject area of the transcription. This diverse composition is beneficial because it allows for a more comprehensive review, where specialists can pinpoint subtle errors related to their specific areas of knowledge. This approach potentially enhances the ability to catch errors related to a particular field of study or industry that might be missed by a less diverse team.
To improve accuracy, these teams employ sophisticated methods, such as the comparison of multiple transcriptions of the same audio file. This can help in spotting inconsistencies and areas where accuracy can be improved. This approach contrasts with a more traditional approach of relying on one transcriber's interpretation, which can introduce bias or miss subtle errors.
From an investigative perspective, it is observed that the quality of a transcription is often negatively impacted by a lack of familiarity with the technical jargon specific to the audio's topic. To combat this, quality control teams often undergo targeted training related to various subject domains. This training provides them with a greater awareness of specific industry terminologies and language, ultimately increasing the quality of the final transcription.
To mitigate the risk of overlooking certain types of errors, the verification process often includes multiple rounds of review. It's not unusual to have a document reviewed by a number of team members. This approach helps prevent blind spots that might arise from a single individual's review. It's a process akin to how engineers use different test methods to gain a deeper understanding of the reliability of a piece of technology.
The process of ensuring high-quality transcriptions is ongoing. To facilitate continuous improvement, regular audits of transcription accuracy are conducted. These analyses of accuracy in relation to the verification process are important because they help identify areas where improvements to quality control strategies are needed. This ongoing assessment can help the quality control team adapt their approaches as challenges to transcription accuracy emerge.
The human element remains important but prone to some limitations. There's growing research demonstrating a strong correlation between decreased accuracy and reviewer fatigue. This highlights the need to create a workflow that avoids overly long shifts or repetitive tasks. This aspect highlights the importance of considering human factors in the process.
To enhance efficiency and consistency in the final verification stage, advanced software and tools are often employed. These tools can flag potential errors for review, but they don't replace the expert judgment of the reviewers. These tools are more like assistants that support the workflow without replacing the role of the human reviewer.
At the end of the review process, the goal is to create a transcription that is both accurate and easy to read. This means that not only should the words be perfectly transcribed, but also that the final product needs to follow logical flow, proper sentence structure, and proper grammar. It is not enough to merely capture the audio perfectly, it needs to be easily understandable and useful to the person reading it.
Finally, to measure and track the success of the final verification process, a variety of metrics are used. This can include metrics that track different kinds of errors, accuracy scores, and other similar data. This information provides valuable insights into how well the process is working. This data allows the teams to fine-tune training, modify workflows, and ultimately improve transcription accuracy over time.
Scribie's Four-Step Human Verification Process A Detailed Analysis of Transcription Accuracy Rates in 2024 - Performance Metrics Show 40 Error Decrease Since 2023 Implementation
Scribie's four-step human verification process, implemented in 2023, has demonstrably improved transcription accuracy. Performance metrics reveal a substantial 40% reduction in errors by the following year, 2024. This outcome indicates that the multi-stage approach, blending automated systems with human review, is effective in tackling the complexities of transcription. The process directly confronts challenges like diverse accents and intricate speech patterns that automated transcription struggles with. Data clearly shows this verification process positively impacts transcription accuracy. However, as technology continues to evolve, the ongoing discussion of finding the optimal balance between automation and human oversight remains vital in the quest for increasingly accurate transcriptions.
Since Scribie implemented their four-step human verification process in 2023, we've seen a 40% reduction in transcription errors by 2024. This is a compelling illustration of how structured quality control can demonstrably improve transcription accuracy. It suggests that a systematic approach to error-checking can be very effective.
It seems that having a good understanding of context really helps in spotting transcription errors. The four-layer proofreading method allows human reviewers to use their contextual knowledge, enabling them to catch subtle errors that automated systems often miss. This aligns with linguistic research, which indicates that contextual awareness is crucial for accurate interpretation of language.
Human cognition is interesting, and research in this area has shown that people are prone to biases which can affect things like transcription accuracy. Scribie's multi-reviewer approach, where different people look at the same material, is a good way to mitigate these biases. It creates a more objective assessment, helping to ensure accuracy.
By integrating specialized knowledge into the quality control teams, we get a more precise evaluation of transcription errors. Different team members are able to address very specific jargon or terminology that might be difficult for automated systems to handle correctly. It emphasizes the importance of domain expertise for complex language tasks.
Research on speech dynamics has shown that when people talk over each other or speak quickly, it can make transcription harder. The four-step method, especially the detailed proofreading phase, directly addresses these challenges. Reviewers are able to interpret the subtle cues within conversations that automated systems might misinterpret.
The quality of the initial audio recording has a strong correlation with the accuracy of the transcription. It reminds us that any attempt to improve transcription accuracy should also think about how the audio data is captured initially.
The cyclical nature of the transcription process demonstrates that feedback loops are critical. As we learn more about error patterns, we can refine the training for reviewers and transcribers. This continuous learning model is essential for long-term improvement in transcription accuracy.
The shift toward collaborative proofreading—where multiple people review the same audio—has been shown to be very helpful in increasing accuracy. Not only does it improve the chances of catching errors, but it also encourages knowledge sharing among team members.
The emotional tone of speech presents a challenge because the intended meaning of the words can be heavily influenced by the emotions of the speaker. It's difficult to translate this into a literal transcription perfectly. This complex aspect again emphasizes why human intervention is important for context-aware accuracy.
Using strong metrics to track and evaluate the transcription process can lead to specific changes in strategy. By studying common error types and reviewer performance, quality control teams can improve their methods, leading to better transcription quality over time.
Scribie's Four-Step Human Verification Process A Detailed Analysis of Transcription Accuracy Rates in 2024 - Independent Testers Report 5 Success Rate For Medical Terminology
Independent evaluations have shown that transcription services achieve a high level of success when dealing with medical terminology. This highlights the crucial importance of precise language in the healthcare field. Medical terminology is complex, and errors in transcription can negatively impact communication between doctors, researchers, and students. To improve accuracy, Scribie has developed a four-step human verification process. This process is specifically designed to enhance the reliability of transcriptions, particularly those dealing with specialized areas like medicine. This focus on accuracy is increasingly important as the need for precise medical records continues to increase. The development of new metrics for measuring transcription accuracy is crucial, as it showcases efforts to improve the accuracy of the process in increasingly challenging circumstances.
1. Independent assessments revealed a notable 85% success rate for transcribing audio containing complex medical terminology, indicating the specialized skills of human reviewers in areas where automated systems frequently struggle. This suggests that human expertise, particularly in specialized fields, remains crucial for high-accuracy transcription.
2. The critical nature of accurate medical terminology in transcriptions is emphasized by the potential for misinterpretation. Reports suggest that transcription errors in healthcare can have severe consequences, including impacts on patient safety and the quality of care. This emphasizes the need for meticulous attention to detail in medical transcription.
3. Evidence suggests that human transcribers are better at identifying subtleties in medical conversations than even sophisticated ASR systems. This ability likely originates from extensive training in comprehending the context of medical interactions. Human perception, refined through experience, appears to surpass current automated capabilities in specific situations.
4. An examination of error patterns in medical transcriptions shows that approximately 70% of errors originate from incorrectly interpreted medical terms. This highlights a key area where human review significantly improves accuracy. These findings also emphasize the complexity of medical jargon for automated systems.
5. Some independent investigations reveal that involving healthcare professionals in the review process can improve the success rate of medical transcriptions by as much as 30%. This emphasizes that having specialized subject matter expertise is highly valuable in achieving high-accuracy results. The importance of domain knowledge in highly specialized fields is becoming increasingly clear.
6. The high stakes involved in medical transcription accuracy have led to the adoption of dual-review systems. In these systems, two medical transcription experts independently assess the same transcription. Research indicates this approach can reduce the incidence of critical errors by 50%. The pursuit of increased reliability in transcription through redundancy in review is interesting.
7. Unexpectedly, background noise has been found to be a significant contributor to transcription errors in medical settings, accounting for about 40% of inaccuracies. This highlights the critical need for maintaining optimal recording conditions in environments like hospitals, where accurate data capture is essential. The influence of external factors like noise on transcription accuracy emphasizes the importance of data preparation.
8. Research suggests that emotional context during medical conversations, such as patient-doctor interactions, plays a key role in the success rate of transcriptions. This is because emotions can substantially alter the implied meaning of spoken words. This observation underscores the challenge of interpreting not just the words themselves, but also the underlying sentiment in speech.
9. Interestingly, independent evaluations showed that specialized medical training not only boosts transcription accuracy but also reduces review times. This suggests that reviewers who possess in-depth medical knowledge are more efficient in handling complex medical content. This might imply the ability to process information faster when domain expertise is present.
10. As the medical field continues to advance, with innovations in treatments and new terminology constantly emerging, it's crucial for medical transcribers to receive continuous training to maintain accuracy. This highlights that the field of medical transcription requires ongoing adaptation in the face of change. The dynamic nature of medical terminology presents ongoing challenges for maintaining high accuracy over time.
Experience error-free AI audio transcription that's faster and cheaper than human transcription and includes speaker recognition by default! (Get started for free)
More Posts from transcribethis.io: