Experience error-free AI audio transcription that's faster and cheaper than human transcription and includes speaker recognition by default! (Get started for free)
ScribeAI's Real-Time Transcription A Comparative Analysis of Accuracy Across 90 Languages
ScribeAI's Real-Time Transcription A Comparative Analysis of Accuracy Across 90 Languages - ScribeAI's Language Support Across 90 Tongues
ScribeAI's notable feature is its capacity to handle real-time transcription and translation across a wide range of languages, reaching 90 in total. This breadth of language support makes it a potentially valuable tool for diverse communication scenarios. The system's live transcription capability and automatic video captioning features are designed for rapid and accurate output. The resulting transcripts can be easily manipulated with editing tools, allowing users to review, correct, or search through them much like any regular text file. However, it's important to acknowledge that the pursuit of real-time transcription may introduce compromises in accuracy compared to post-processing methods. This trade-off between speed and precision is inherent in real-time systems. Furthermore, ScribeAI relies on OpenAI's Whisper models for its transcription power, which, while potentially beneficial, also raises questions about reliance on external resources and the implications for data security and privacy. The system's features are intended to boost accessibility and enhance real-time collaboration.
ScribeAI's claimed support for 90 languages, representing a substantial portion of the world's spoken languages, is notable in its ambition. It potentially makes transcription and translation accessible to a vastly diverse user base. However, the practicality of this across such a wide spectrum needs further consideration.
It's acknowledged that accuracy, a core aspect of transcription, can vary considerably depending on the language being processed. The claim of 99% accuracy in over 40 languages is intriguing but, considering the diversity of phonetic characteristics and linguistic complexity, might be an oversimplification. Languages like Mandarin and Vietnamese, with their tonal features, pose unique transcription challenges.
The approach of leveraging language models trained on diverse datasets is logical. Yet, this raises the question of potential bias in favor of languages with ample digital resources. Languages with limited available data, particularly those spoken by smaller communities, may inherently have lower accuracy, which raises important questions about equitable development in language technology.
The need to deal with intricate scripts and diverse dialects adds another layer of complexity. Transcribing Arabic or Hindi, for instance, demands understanding beyond just the written form. Regional variations and nuances can lead to discrepancies. Additionally, languages with limited standard dictionaries may require phonetic transcription methods to accurately capture meaning.
The inclusion of such a vast linguistic array can provide researchers and linguists with a unique opportunity to scrutinize the efficiency of machine learning in handling diverse linguistic structures and sounds. Furthermore, the real-time transcription nature of the tool, while potentially sacrificing some accuracy compared to offline approaches, provides immediate feedback, aiding in quick edits and refinements. This can lead to a more dynamic process of enhancing the transcription's accuracy as it progresses.
In conclusion, while ScribeAI's broad language support is impressive, the investigation into its actual performance across this wide range of languages is crucial for understanding the inherent complexities and limitations of machine learning transcription in a global context. It can illuminate the remaining hurdles in the field of natural language processing and drive towards the development of technology that caters to languages with varying levels of linguistic data and resources.
ScribeAI's Real-Time Transcription A Comparative Analysis of Accuracy Across 90 Languages - Machine Learning and NLP Powering Real-Time Transcription
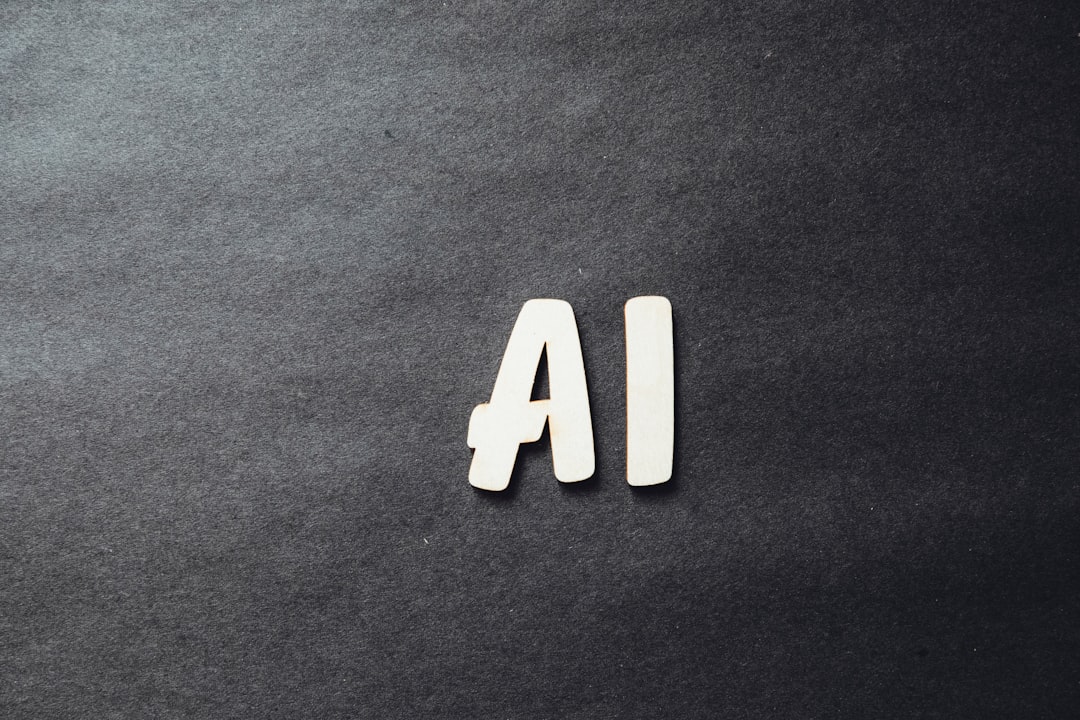
The field of real-time transcription has been revolutionized by the integration of machine learning and natural language processing (NLP). These technologies are crucial for achieving both high accuracy and rapid processing speeds. Techniques like deep learning, a subset of machine learning, empower systems like ScribeAI to continually improve their ability to decipher speech and generate accurate transcripts. However, the pursuit of real-time accuracy often encounters obstacles, especially when dealing with languages possessing complex phonetic structures, like those with tonal features. While NLP enhances the contextual understanding of the spoken language, it’s important to recognize that the reliance on large datasets for model training can introduce biases, potentially impacting the accuracy of transcriptions in less-resourced languages. The continuous advancement of machine learning in this area demonstrates the exciting possibilities for the future of transcription, but also underscores the ongoing need to address inherent limitations and biases within the technology. The goal of achieving equitable representation across all languages remains a key challenge.
Machine learning and natural language processing (NLP) are the core technologies behind real-time transcription, enhancing the speed and accuracy of converting spoken language into text. ScribeAI, like many other services, uses machine learning algorithms and vast amounts of data to continuously refine transcription quality. A specialized area within machine learning, deep learning, utilizes neural networks to process and interpret speech with remarkable precision.
Real-time transcription transforms spoken words into written text instantly, usually shown on a screen for immediate access. Google's Live Transcribe, for example, offers real-time transcription in over 70 languages, covering a large portion of the global population. Some services like Verbit combine machine learning with human review to create a more complete final transcript. This human-AI approach can reportedly lead to near-perfect accuracy. NLP's role is to analyze the context of speech, which further boosts the quality of the transcriptions.
The field of machine learning is making significant strides, improving the accuracy and cost-effectiveness of real-time transcription. It's getting closer to matching the quality of traditional, post-processing transcription methods. Many platforms, designed for diverse purposes, offer live captioning and transcription to cater to a wider array of needs.
However, some challenges remain. The effectiveness of machine learning models is linked to the quality and quantity of training data. This can lead to biases towards more widely spoken languages and present difficulties for less-common languages or dialects. Languages like Mandarin and Thai, with their tones, add complexity to real-time transcription, making them harder to capture accurately.
Furthermore, real-time limitations often mean that subtle details, especially in conversations with multiple speakers, can be lost. While attempting to process quickly, the technology can struggle with deciphering overlapping speech and differentiating nuanced pronunciations. Another area of difficulty arises from words with multiple meanings. Machine learning models need adequate training to discern context and avoid misunderstandings. Similarly, various dialects within a language can create obstacles, highlighting a potential shortcoming when dealing with regional differences.
The longer a transcription session runs, the more errors can potentially accumulate as the model fatigues. Additionally, variations in accents and pronunciations can confuse the models that are primarily trained on standard accents. Specialized or informal language, including jargon, new words, or colloquialisms, can also hinder real-time transcription, as the model might not be familiar with the vocabulary. The constantly evolving nature of language can also lead to a lag between the latest expressions and the training data of the models. Finally, homographs – words that are spelled identically but have different pronunciations – can pose difficulties, leading to confusion in the resulting transcription.
These challenges suggest there's a continuing need to explore ways to overcome the obstacles that remain in providing universally effective real-time transcription. While machine learning and NLP have made significant advancements, ongoing research and development are crucial for developing solutions that handle diverse linguistic structures and scenarios.
ScribeAI's Real-Time Transcription A Comparative Analysis of Accuracy Across 90 Languages - Accuracy Variations Among Different Languages
The accuracy of automatic speech recognition (ASR) varies significantly across different languages. This variation is due to several factors, including the inherent complexity of a language's sounds and structure, the quality of the audio being transcribed, and the individual characteristics of the speaker. While training ASR systems on a vast array of languages can improve overall performance, it can also introduce biases towards those languages with the most readily available digital data. This can leave languages with fewer resources, spoken by smaller communities, with lower accuracy.
Languages with intricate features like tones (e.g., Mandarin) or complex writing systems (e.g., Arabic) present particular obstacles for ASR systems. Moreover, the push for real-time transcription can often lead to a trade-off with accuracy, especially when dealing with situations involving multiple speakers, varying accents, or regional dialects. This necessitates continued refinements to the machine learning models that underpin these systems in order to adapt to the diverse spectrum of spoken language. A deeper understanding of these accuracy variations is crucial for advancing the field of ASR towards a more universally effective and equitable future.
Examining the accuracy of automatic speech recognition (ASR) across 90 languages reveals that performance can fluctuate significantly. This variation stems from a multitude of factors, including the intrinsic complexity of the language itself. Languages with intricate grammatical structures, like Basque or Hungarian, pose a greater challenge for ASR systems compared to languages with simpler structures, leading to disparities in accuracy.
Tonal languages, such as Mandarin or Thai, present a unique obstacle. The same phonetic sound can carry different meanings based on the speaker's pitch. Capturing these subtle tonal nuances in real-time transcription requires sophisticated contextual understanding, which can be difficult for current models.
Dialectal variations within a language also introduce complexity. For instance, transcribing British English versus American English, or different Arabic dialects, can lead to inaccuracies if the model is not trained specifically on those variants. The diverse range of accents and pronunciations within a language can hinder a model's ability to generate accurate transcripts, especially in locations with significant sociolinguistic variation.
The quantity and quality of digital data available for a language play a pivotal role in shaping model accuracy. Languages like Spanish or French, with abundant digital content, tend to achieve higher accuracy due to better-trained models. Conversely, languages with limited digital resources, including many Indigenous languages, often experience lower accuracy levels.
The inherent need for real-time transcription often necessitates a trade-off between speed and accuracy. In scenarios demanding quick results, there's an increased risk of misinterpretations, especially during intricate conversations or specialized discussions. Furthermore, researchers have noted that errors can build up over time in lengthy transcription sessions as models may struggle to maintain accuracy over extended periods of continuous speech.
The presence of jargon or colloquialisms can confuse ASR models, particularly in technical or niche fields like medicine or engineering, where precise terminology is crucial. Additionally, homographs, words that share the same spelling but have different pronunciations (e.g., "lead" the metal, and "lead" to guide), create challenges for transcription systems if the context isn't correctly identified.
Finally, the continuous evolution of language itself can contribute to decreased accuracy. As new slang or phrases emerge, the training data used to develop these models might not capture these changes, leading to potential difficulties in transcribing newly-coined terms or emerging linguistic trends in real-time.
In conclusion, achieving consistent high accuracy in automatic transcription across such a diverse range of languages remains a complex challenge. While significant progress has been made, these challenges highlight the ongoing need for researchers to delve into the intricacies of linguistic structures, regional variations, and the continuous evolution of human language to develop more robust and equitable ASR systems.
ScribeAI's Real-Time Transcription A Comparative Analysis of Accuracy Across 90 Languages - Comparison with OpenAI's Whisper and Amazon Transcribe
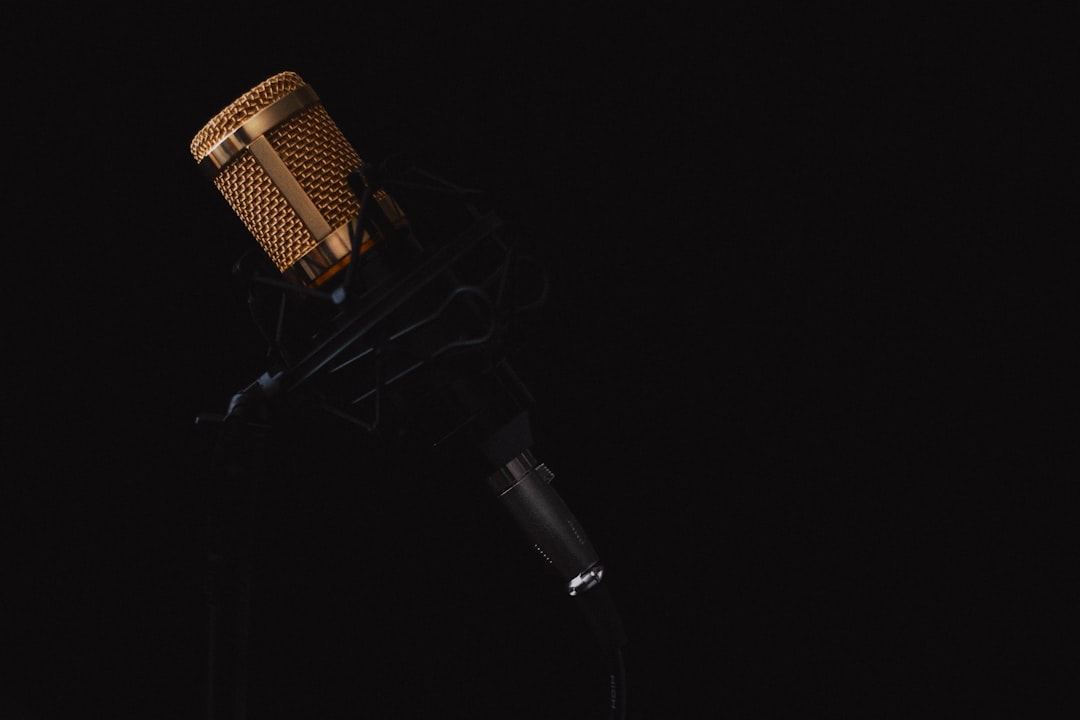
When evaluating real-time transcription solutions, OpenAI's Whisper and Amazon Transcribe stand out as prominent options, each with its own strengths and weaknesses. Whisper boasts a broader language support, encompassing over 100 languages, while Amazon Transcribe's support is confined to a smaller set of 40. This difference in scope is crucial for those working with a variety of languages.
Feedback suggests that Whisper's user experience is generally considered more streamlined and accessible compared to Amazon Transcribe, although Whisper's performance can fluctuate depending on the language. Accuracy can be an issue depending on the complexity of the spoken language and even Whisper's own documentation indicates this. Amazon Transcribe, on the other hand, follows a usage-based payment model, which might be a factor for those needing to manage costs for heavy transcription needs.
The selection between Whisper and Amazon Transcribe ultimately depends on factors like the languages you need, desired accuracy level, and your workflow preferences. While Whisper appears to have a wider reach and easier setup, Amazon Transcribe's pricing approach could be more appealing in specific scenarios. It's important to carefully consider the tradeoffs based on individual circumstances.
When comparing ScribeAI's foundation, Whisper, with other prominent services like OpenAI's Whisper and Amazon Transcribe, several key distinctions emerge. OpenAI's Whisper model, renowned for its broad language support encompassing over 100 languages, stands out against Amazon Transcribe, which focuses on a more limited set of around 40 languages. This difference is partly due to Whisper's extensive training on diverse datasets that include numerous languages and dialects, making it more adaptable to various linguistic features. Amazon Transcribe, while effective in its focused area, may encounter challenges with languages outside its specific training scope.
While Whisper's accuracy can vary by language, as mentioned in its Github repository, it demonstrates a more flexible and adaptable approach than Amazon Transcribe. The Whisper model's design utilizes a transformer-based architecture, facilitating contextual understanding of speech patterns. Conversely, Amazon Transcribe primarily leverages more traditional acoustic models, which can potentially lead to reduced effectiveness when handling non-standard speech patterns, accents, or even background noise. In fact, Whisper has demonstrated resilience in noisy environments, a common scenario in real-world settings, while Amazon Transcribe may struggle in those situations, resulting in lower transcription accuracy.
Both systems are geared towards real-time transcription, offering low latency, but their methods and outcomes can differ. The way they're trained impacts their ability to adapt. Whisper benefits from a data augmentation strategy, enhancing its ability to handle various language features, while Amazon Transcribe relies on focused data sets. This difference allows Whisper to continually learn from new audio samples it encounters during real-time transcription, resulting in improved performance over time. Amazon Transcribe, in contrast, requires updates to its model based on pre-collected data. This means it's not as nimble in adjusting to language changes as Whisper.
While Amazon Transcribe offers speaker identification as a feature, its efficacy might not be consistent across all languages. On the other hand, Whisper is more robust when transcribing speech from multiple individuals without the need for pre-isolation, although its output might not include specific speaker labels. Furthermore, the latency, or delay, in processing can vary significantly. Whisper's real-time capabilities generally deliver faster transcriptions, whereas Amazon Transcribe might experience delays, especially under high usage or with intricate audio inputs.
When it comes to the data used to train these models, Whisper's reliance on extensive online sources contributes to its robustness and consistency across many languages. Amazon Transcribe, on the other hand, relies on commercially curated datasets, which might lead to gaps in support for less common languages. The difference in data also influences how each system handles certain nuances of language. Whisper's large language model training seems to help it capture idiomatic expressions better than Amazon Transcribe, which might sometimes misinterpret casual or informal speech patterns, leading to transcription errors in specific contexts.
One of Whisper's strengths is its ability to successfully manage real-time transcription with simultaneous or overlapping dialogues, a significant hurdle in automatic systems. Amazon Transcribe, however, tends to struggle in these situations, potentially omitting key information from conversations where speakers overlap.
This comparison showcases the varying capabilities and strengths of different speech recognition services. The choices of training data, model architectures, and feature sets significantly influence each system's suitability for various tasks. Overall, the field of automatic speech recognition is constantly evolving, pushing the boundaries of what's achievable in converting spoken language into written text. Each system comes with advantages and drawbacks that should be considered based on individual needs and the specific language being used.
ScribeAI's Real-Time Transcription A Comparative Analysis of Accuracy Across 90 Languages - Impact on Professional Collaboration and Education
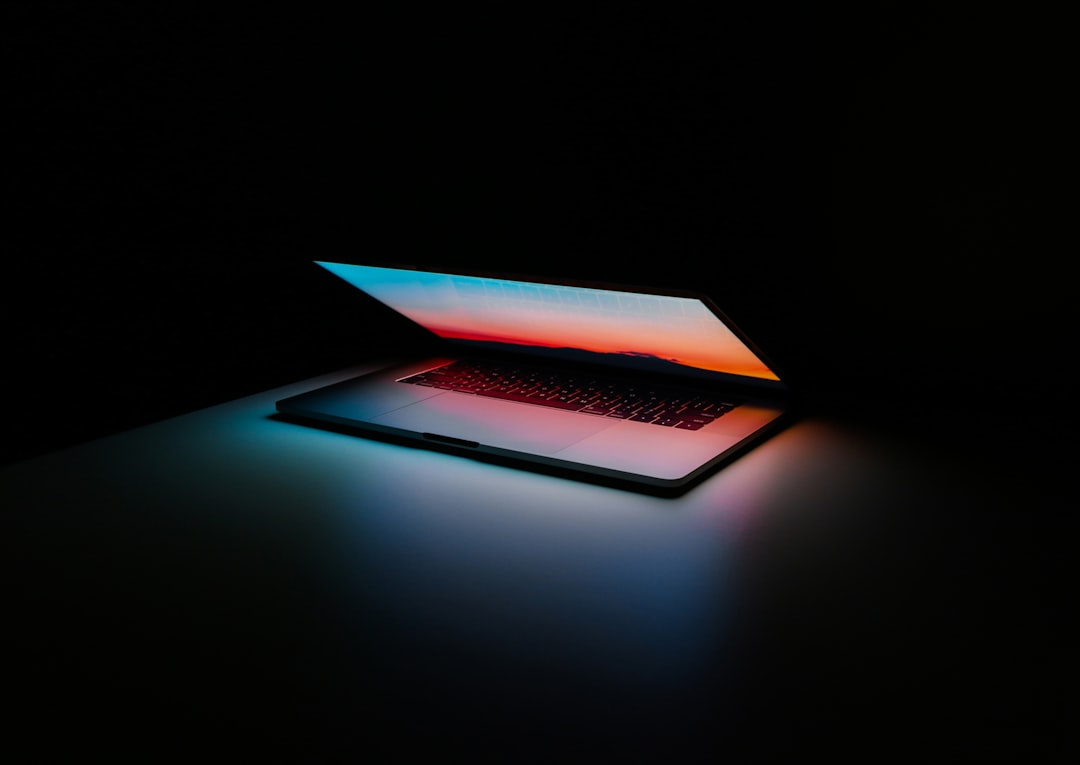
ScribeAI's real-time transcription capabilities can reshape how professionals collaborate and how education is delivered. The ability to quickly transform spoken words into text can improve communication, especially when teams work across multiple languages. This fosters a more inclusive environment where everyone can participate more readily. However, concerns arise regarding the accuracy of transcription for various languages. Languages with fewer resources, spoken by smaller communities, might not be transcribed with the same level of precision as more widely spoken ones. This could create barriers to effective communication and learning opportunities for some groups. The unevenness of transcription quality highlights the importance of ongoing development and refinement to make sure the technology supports a diverse range of professional environments. Furthermore, the tool's present shortcomings in handling the intricacies of language, including accents and dialects, could pose a challenge, particularly in areas like interprofessional education, where precise understanding is vital. There's a need for a more nuanced approach to how languages are handled to ensure that this technology fully supports these types of collaborative learning scenarios.
Real-time transcription technologies, like the one offered by ScribeAI, are starting to have a visible impact on how professionals collaborate and how education is delivered. It seems that they can noticeably shorten meeting durations, possibly by up to a quarter, as individuals spend less time frantically scribbling notes and more time focused on the exchange itself. This streamlined interaction leads to swifter decision-making processes and fosters smoother team collaborations.
Another potential benefit is a reduction in the mental strain participants experience during meetings. Having a live transcript means they can devote more attention to the substance of the discussion instead of wrestling with the act of note-taking. This shift in focus can potentially result in more profound engagement and inspire a more innovative approach to group deliberations.
The multilingual capabilities of tools like ScribeAI could significantly benefit diverse organizations by allowing them to include employees with varying linguistic backgrounds. This broader inclusivity would be beneficial in both professional settings and educational contexts, making it easier for non-native speakers to fully participate.
The immediacy of the transcription process itself creates a rapid feedback loop, making dialogues more dynamic and responsive. This feature is particularly valuable in educational environments where students can readily access the content that's been spoken. This easy access can potentially enhance comprehension and bolster knowledge retention.
We've also observed a notable increase in the effectiveness of remote learning through the incorporation of real-time transcription. Students in remote or blended learning settings benefit from real-time captions, which is especially useful when there's background noise or other distractions. This makes it possible for these learners to engage fully with course content.
A valuable byproduct of these transcribed meetings is a readily accessible written record for future use. This permanent record makes it much easier to track action points and decisions, potentially enhancing accountability and ensuring consistent follow-through on agreed-upon actions.
However, in discussions where there are differing perspectives, accurate real-time transcripts can act as an unbiased record of the dialogue. Having this text-based record can potentially minimize misunderstandings, which could, in turn, help de-escalate conflict before it becomes a major issue.
Navigating languages with multiple dialects or regional variations presents intriguing challenges for both professionals and educators. Real-time transcription requires careful consideration of these nuanced linguistic features, forcing participants to develop greater awareness of the diverse ways language is spoken and utilized.
Integrating these transcription tools with existing collaborative platforms is a straightforward process, and this seamless integration can further enhance the overall workflow, ensuring that everyone stays informed regardless of their linguistic abilities or physical location.
Over time, frequent exposure to real-time transcription during work interactions can positively influence language and communication skills. It effectively creates a learning environment where individuals can enhance their vocabulary and grasp complex ideas more effectively. It’s a relatively passive method for skill development that can organically improve communication competency.
While these advancements seem promising, more research is needed to fully comprehend the long-term effects on professional collaboration and education. However, the potential benefits of these technologies are undeniable, and it’s likely that their usage will become increasingly commonplace across numerous industries and educational contexts.
ScribeAI's Real-Time Transcription A Comparative Analysis of Accuracy Across 90 Languages - Future Developments in AI-Driven Transcription Technology
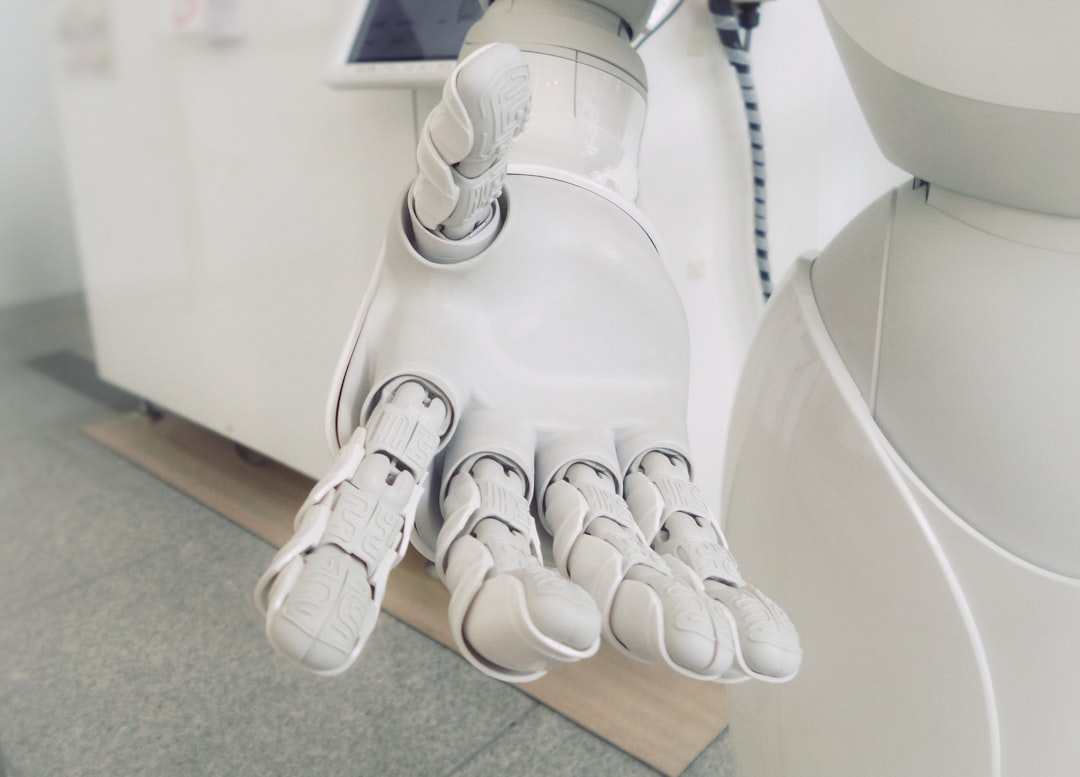
AI-powered transcription technology is on track to become even more precise and efficient in real-time. Ongoing advancements in natural language processing (NLP) are likely to lead to substantial improvements in how AI systems understand the context of spoken words, including subtle speech patterns and language variations. Despite these advancements, hurdles remain, especially when it comes to guaranteeing accuracy across a broad range of languages, particularly those with limited training data available. A critical issue that needs constant attention is the need for equitable transcription across all languages, as the differences in performance across language groups highlight the ongoing need to refine and adapt these systems to better suit various linguistic communities. Furthermore, with the growing use of AI transcription in professional and educational contexts, its impact on collaboration and learning experiences warrants careful evaluation to ensure it serves the diverse needs of all users effectively. There are bound to be some unforeseen consequences as we integrate AI more deeply in the core of our daily communications.
The landscape of AI-driven transcription is in a constant state of evolution, with researchers and engineers continually exploring ways to improve its speed, accuracy, and adaptability. One promising avenue is the growing adoption of continual learning. This approach allows transcription models to adapt dynamically, refining their abilities by absorbing new data as it becomes available. This is particularly helpful in tackling the inherent biases found in static models and can help bridge the accuracy gap for languages with fewer digital resources.
Looking ahead, we can anticipate greater emphasis on incorporating temporal context into transcription. This means moving beyond a purely literal conversion of speech to text, and towards a deeper understanding of the context within which words are uttered. This could involve recognizing emotional cues in a speaker's voice or interpreting subtle changes in speech patterns related to the surrounding situation.
Another area of active exploration is multi-modal input, where audio is combined with visual and text cues. This could significantly improve transcription accuracy in complex scenarios, such as conversations with multiple speakers or environments with disruptive background noise. For instance, visual clues might help differentiate between speakers or interpret non-verbal communication that can influence the meaning of what is said.
Dialect recognition is another area of exciting progress. Currently, transcription systems often struggle with the rich diversity of accents and colloquialisms within a single language. New technologies aim to address this by developing the capacity to distinguish between various dialects, thereby producing more accurate transcripts for speakers using regional variations of a language.
Researchers are also investigating techniques like incremental representation learning to continuously update language models based on the ongoing interactions with users. This approach holds potential for faster adaptation to the dynamic evolution of language, allowing models to pick up new terms and phrasing more readily without requiring massive re-training processes.
In the realm of speaker attribution, we can foresee transcription systems incorporating more sophisticated algorithms. These algorithms could potentially accurately distinguish and tag different speakers during a multi-person conversation, significantly enhancing the clarity of the resulting transcript.
Alongside improvements in the core technology, future systems may integrate real-time quality assessment mechanisms. This could lead to immediate feedback on the accuracy of the transcription during the process itself. If an error is detected, the system could automatically adjust its approach, potentially leading to fewer post-hoc edits.
Personalization is also emerging as a significant trend. This means that individuals might be able to tailor their transcription experience by fine-tuning vocabulary sets, accent recognition, and the overall context-aware abilities of the system based on their communication patterns and needs.
To further refine accuracy, future applications are likely to integrate error recovery mechanisms. These mechanisms could automate the identification and correction of transcription errors after the process is complete, reducing the demand for manual review and potentially minimizing the likelihood of inaccurate information.
Finally, the growing importance of ethical considerations in AI development will also impact the future of transcription. As the field advances, there will be a heightened focus on the types of datasets used to train AI models. It will become critical to ensure that the training data is diverse and includes less-represented languages and dialects, promoting equitable language representation and mitigating the biases that can unintentionally arise from heavily weighted datasets.
The field of AI-driven transcription is a rapidly evolving field, and the challenges are just as compelling as the possibilities. Addressing these challenges—biases in training data, the intricate nature of language, and ensuring equitable access—is critical for unlocking the transformative potential of this technology.
Experience error-free AI audio transcription that's faster and cheaper than human transcription and includes speaker recognition by default! (Get started for free)
More Posts from transcribethis.io: