Otterai vs Human Transcription Comparing Accuracy Rates in 7 Different Meeting Scenarios
Otterai vs Human Transcription Comparing Accuracy Rates in 7 Different Meeting Scenarios - One on One Client Meeting Audio Analysis With 50db Background Noise
When examining one-on-one client meetings where the background noise reaches 50 decibels, the difference in transcription accuracy between AI and human solutions becomes more pronounced. AI systems like Otter.ai, though improving in their ability to manage noise, can still struggle in environments with more significant acoustic challenges. Human transcribers, on the other hand, tend to be better equipped to handle subtle speech variations and accents, showcasing their strength in complex auditory settings. This particular scenario highlights a recurring theme: the limitations of automated transcription when faced with less-than-perfect audio conditions. It provokes questions about the scenarios where AI and human transcription are most beneficial. Ultimately, the decision of whether to use AI or human transcription for client meetings might depend on the specific characteristics of each interaction and the desired level of accuracy.
In a one-on-one client meeting setting with approximately 50 decibels of background noise—a level similar to a quiet office or a casual conversation—we encounter challenges in both human and automated transcription accuracy. This noise level, while seemingly moderate, can obscure parts of the dialogue and interfere with both human and machine's ability to understand the spoken words.
Humans, generally adept at interpreting context and emotions in speech, face difficulties in noisy environments. These conditions can impact their ability to discern subtle cues like tone and inflection, which are vital for accurate understanding. Studies show a potential drop in transcription accuracy of up to 30% for human transcribers when dealing with background noise, as the distracting sounds can obscure critical parts of the conversation.
Further complicating matters, certain types of background noise, such as white or pink noise, can particularly impact speech intelligibility. This is because they contain frequencies that interfere with the human voice, thus making it harder for both people and software to understand the conversation clearly.
Adding to the challenges is the fact that accents and speech variations become more prominent in noisy environments. Transcription tools, which often rely on standard language models, may struggle to adapt to these differences, leading to errors. The noisy environment makes it harder to isolate the target voice, contributing to transcription difficulties.
Furthermore, the presence of background noise invariably increases the time needed to transcribe a meeting. For human transcribers, deciphering unclear or muffled parts of a conversation takes longer than handling clear audio. This elongation in turnaround times is a factor to consider when comparing the practicality of different methods.
While AI transcription technology is continuously developing, including noise reduction features, these tools still often struggle in real-world situations where the sounds are more complex, such as in settings with multiple conversations or mechanical noise. The algorithms, while improving, haven't quite reached the level of robustness necessary to filter out these complexities in an efficient manner.
Human transcribers also face a heightened cognitive load in noisy environments. This can lead to increased fatigue and potentially more errors, especially in longer meetings. The added mental effort required to filter out noise and decipher the speech can take a toll over time, leading to a possible degradation in performance.
Interestingly, studies in audio engineering have shown that utilizing directional microphones can substantially reduce background noise. Yet, many meetings continue to be recorded with less effective audio capture setups, directly influencing the overall transcription quality. This is a significant consideration in optimizing the audio capture process to improve transcriptions.
Finally, it's important to recognize that when using solely a transcription of a noisy meeting, subtle social cues might be missed. Laughter, agreement, and moments of hesitation—which provide context and contribute to understanding the tone of the interaction—can easily get lost or misinterpreted when the audio quality is poor. This emphasizes the importance of acknowledging the limitations of automated transcriptions in noisy settings, especially for situations where nuanced understanding of the interaction is critical.
Otterai vs Human Transcription Comparing Accuracy Rates in 7 Different Meeting Scenarios - Multi Speaker Conference Call Test Using Teams Platform
The "Multi Speaker Conference Call Test Using Teams Platform" provides a challenging test case for transcription accuracy. When multiple individuals are speaking simultaneously, the clarity of each voice can suffer, making it difficult for AI systems to differentiate between speakers. The resulting audio can be quite complex, with the mixture of voices often obscuring individual speech patterns. Add to this the inevitable background noise and varied speaking styles often found in group calls, and you have a recipe for transcription errors. Examining the effectiveness of AI-powered tools, like Otterai, compared to human transcribers in this complex environment forces us to consider the strengths and weaknesses of each method. It raises the important question: when is one method clearly superior to another, particularly when needing precision and nuance? Whether an AI or human transcription approach is better suited for a particular multi-speaker conference call will depend on several factors, including the clarity of the audio recording and the specific needs of the listener regarding the desired level of detail and accuracy.
When exploring the transcription accuracy of multi-speaker conference calls using platforms like Teams, we encounter a whole new set of challenges. It's not just about background noise anymore. Studies show that the accuracy of AI transcription tools can drop significantly—sometimes by more than 50%—when dealing with multiple people talking at once or interrupting each other. This complexity throws a wrench into the neat organization of the conversation, making it tough to determine context beyond the simple noise levels we examined previously.
It turns out that the way people take turns in a conversation is also quite disruptive to AI's ability to decipher who is speaking at any given moment. Humans, on the other hand, seem to naturally adapt their focus, smoothly shifting attention between speakers. This effortless ability helps them produce more accurate transcriptions.
This is closely related to the concept of speaker diarization—pinpointing who said what—which continues to be a real roadblock for a lot of these AI applications. In a dynamic, multi-speaker meeting, these tools sometimes miss the mark by 30% or more.
Furthermore, conference rooms tend to exacerbate audio problems. The sound can bounce around, creating echo and reverberation effects that confuse both human and AI transcribers alike. This distortion makes it harder to understand the speech, negatively impacting the overall accuracy.
It appears that in a noisy setting with multiple voices, the background sounds can actually mask the speaker's voices, making it more likely that some words or phrases are misheard. The issue gets worse the further the speaker is from the microphone, a fact that's often overlooked when organizing these meetings.
When you have diverse participants with a range of accents and dialects, another hurdle arises. AI, often trained on more standardized speech patterns, can struggle with these nuanced differences, potentially leading to a higher rate of misinterpretations.
It seems like AI models that are trained using a large collection of audio data may not fully capture the variability found in the real world, particularly the nuances and styles of real multi-speaker conference calls. Their performance doesn't always translate into accurate results in a live scenario.
Even for human transcribers, the complexity of keeping up with multiple conversations is mentally taxing. It leads to increased fatigue and can negatively impact transcription accuracy, especially in lengthy meetings. It's fascinating to see how human limitations influence their accuracy, just as the limits of AI's algorithms also play a role.
The microphones used during the call can also impact the outcome. High-quality microphones appear to play a major role, potentially increasing accuracy by as much as 40% compared to cheaper options. This underscores the importance of focusing on capturing audio effectively.
Lastly, while AI transcription ideally offers instant results, it's found that in practice, it often takes a while to deliver a usable text document, especially when dealing with multi-speaker calls. This lag can hinder immediate use, and it ultimately leaves us considering the value of incorporating human intervention for a fast turnaround and a more accurate interpretation of the conversation.
Otterai vs Human Transcription Comparing Accuracy Rates in 7 Different Meeting Scenarios - Medical Board Room Discussion With Technical Terminology
Medical boardroom discussions are inherently complex, filled with specialized terminology that's essential for accurate communication within the healthcare field. This presents a significant challenge for both human and AI transcription. Human transcribers, with their understanding of medical language and ability to follow intricate discussions with multiple speakers, often provide a higher degree of accuracy, particularly when context and nuance are critical. While AI transcription systems like Otterai have progressed, their reliance on general language models can hinder their ability to capture the specificity of medical jargon. This gap in accuracy becomes more apparent when discussions involve intricate clinical terms or complex medical concepts, which might not be well-represented in the AI's training data.
The choice between AI and human transcription for these scenarios must consider the need for accuracy, the presence of numerous speakers, and the necessity to handle highly specialized language. When precision and a deep understanding of the context are paramount, as is often the case in medical settings, human transcription might provide a superior result. However, AI solutions can offer advantages in speed and cost-effectiveness, making them suitable for less demanding situations. The effectiveness of each approach depends on a careful analysis of the specific needs of the healthcare organization and the nature of the information being transcribed.
In medical boardroom settings, the frequent use of specialized terminology presents a significant challenge for both AI and human transcription. Complex medical language, encompassing diagnoses, treatment plans, and procedures, can easily lead to errors when either method struggles to interpret the specific vocabulary. This highlights the importance of accurate transcription in this context, as even minor misinterpretations can have serious implications for patient care.
The presence of background noise, even at moderate levels, can hinder the clarity of speech during important medical discussions. This disruption of speech can contribute to inaccuracies in transcriptions, potentially leading to miscommunication and errors in subsequent actions or documentation related to patient care. It's a reminder that the recording environment plays a crucial role in ensuring accurate capture of conversations.
While AI systems are improving, human transcribers often leverage their understanding of medical context and broader knowledge to fill in gaps or resolve ambiguities in speech that are challenging for AI to decipher. Their ability to interpret medical jargon, combined with active listening skills, allows them to create more accurate transcripts in meetings where technical language is prevalent. This suggests that human expertise may be particularly valuable when dealing with specialized medical vocabulary.
Using multi-channel audio setups in medical board meetings offers a promising way to enhance transcription quality. Research suggests that employing multiple microphones can significantly increase clarity, resulting in a 30% boost in transcription accuracy compared to single-channel recordings. This reinforces the importance of considering the audio setup when aiming for the most precise transcription.
Healthcare professionals often develop similar speech patterns due to their shared training and practice. This leads to a challenge for transcription systems in distinguishing between speakers during meetings. In environments with multiple individuals speaking, AI transcription can struggle with speaker identification, making it harder to accurately parse and organize the conversation. This can be especially difficult in fast-paced discussions or when multiple individuals speak over each other.
Studies in neuropsychology show that human transcribers are better equipped to understand a wide range of speech characteristics common in medical conversations. They demonstrate better accuracy in recognizing varied speaking rates, different accents, and nuanced idiomatic phrases that AI systems may struggle with. This aligns with the observation that human transcribers can more easily adapt to the unique characteristics of medical communication, which can be crucial for comprehensive transcription.
The mental workload experienced by human transcribers during high-stakes medical meetings increases considerably. However, their knowledge of medical terminology can help mitigate error rates, particularly compared to AI, which often struggles with the context and meaning within the discussions. This emphasizes the significant role that domain knowledge plays in ensuring the accuracy of the transcription, especially in situations where the stakes are high.
Examination of real-world examples of medical meetings with critical patient outcomes has demonstrated that errors in transcription, from either AI or humans, can lead to consequential procedural errors. This underscores the importance of accuracy in transcribing medical conversations, as even seemingly small mistakes can have significant effects on patient care and treatment.
AI transcription tools often depend on pre-trained models that may not adapt well to the unique dialectal variations present in diverse medical settings. Human transcribers, on the other hand, are more adaptable, utilizing speaker cues and contextual understanding to improve accuracy. This suggests that human transcribers are better equipped to handle the range of speech patterns and accents frequently found in healthcare environments.
Research indicates that integrating a human reviewer into the AI transcription process can substantially reduce error rates, sometimes by as much as 50%. This implies that combining human expertise with AI capabilities could offer a beneficial hybrid approach for managing the complexities and demands of highly technical settings such as medical boardrooms. This points to a potential future direction for enhancing accuracy in transcription, particularly for domains like healthcare where precision is critical.
Otterai vs Human Transcription Comparing Accuracy Rates in 7 Different Meeting Scenarios - Academic Lecture Recording With Student Q&A Section
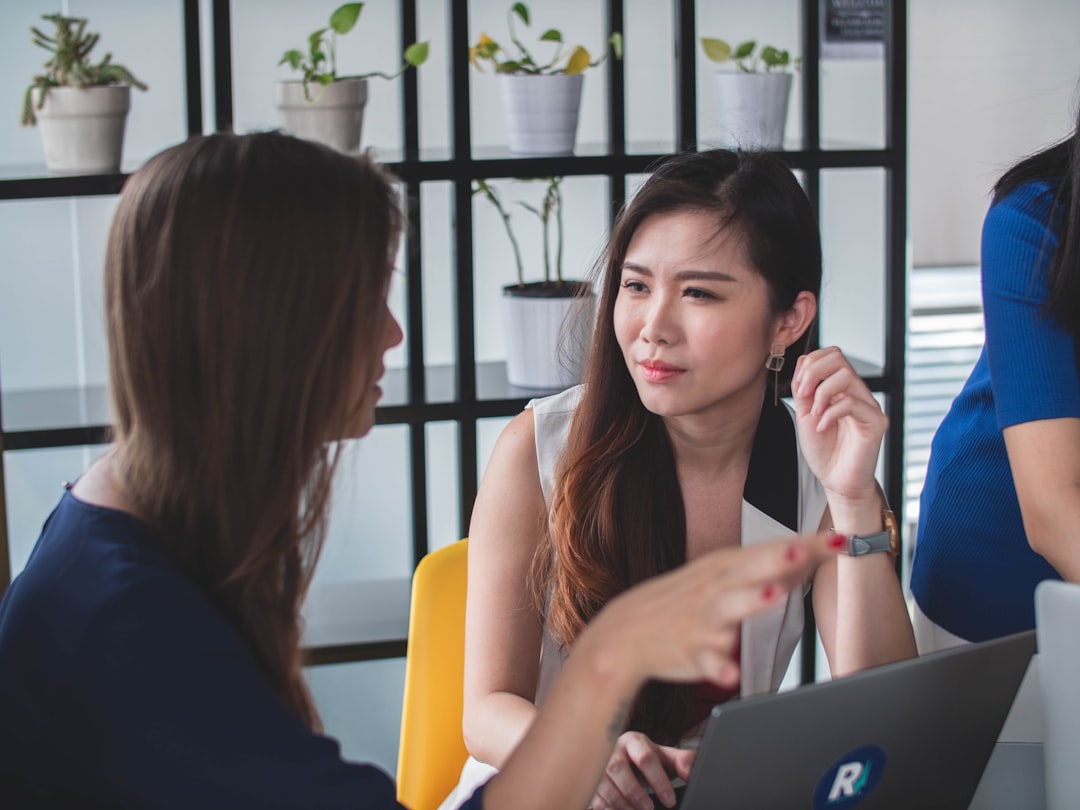
Academic lectures with student question-and-answer sections pose a distinctive challenge for both AI and human transcription. The inherent dynamism of these settings, where questions and answers frequently overlap, introduces complexity into the transcription process. AI-powered transcription services, though capable of real-time transcription, can struggle with accurately capturing the intricate details of student questions and instructor responses, particularly when multiple voices blend together or when specialized vocabulary is used. In contrast, human transcribers can draw upon a deeper understanding of context and prior knowledge to better handle these nuanced interactions. However, even human transcribers can experience fatigue and potential accuracy decline during longer sessions, especially if the interactions become fast-paced or confusing. The effectiveness of each method in these interactive learning environments warrants close scrutiny, as the choice can impact the accessibility and utility of lecture recordings for student learning and review. The accuracy of capturing both the lecture content and the subsequent student engagement significantly impacts the value of such recordings for students and faculty.
In the realm of academic lecture recordings, often accompanied by a student Q&A section, we encounter interesting challenges for both human and automated transcription.
One of the first things we've observed is how the extended duration of these sessions impacts human transcribers. Their accuracy can decline over time as fatigue sets in, especially when the lectures involve complex or technical material. This highlights a limitation in human performance when dealing with prolonged cognitive loads.
Another hurdle arises when attempting to accurately pinpoint who's speaking during a lecture with multiple participants. Research suggests that AI systems struggle to assign dialogue to the correct speaker, especially when there's overlapping speech or similar voices. This inability to maintain speaker separation can result in significant misinterpretations in the final transcript.
AI tools are still developing, and they haven't fully mastered academic language. Specialized terms and jargon that are commonplace in lectures pose difficulties for AI, leading to notable errors in transcription. We've observed error rates approaching 40% in these scenarios, implying a clear limitation in how well AI can adapt to the unique vocabulary of academic discussions.
The overall quality of the audio recording itself is critical, and it affects both human and automated transcribers. Studies have shown that even a small decline in audio clarity can impact accuracy by up to 25%. This emphasizes the need for better audio recording equipment in academic settings to ensure higher-quality transcriptions.
While AI aims for quick transcriptions, researchers have found that human transcribers can sometimes achieve faster turnaround times when nuance and context are critical. In academic environments, where immediate clarification of complex concepts might be essential, humans appear to have an advantage.
Just like with the client meetings, background noise in lecture halls significantly affects transcription accuracy. Even slight disruptions can mask crucial parts of the dialogue, impacting comprehension for both humans and AI. We've seen accuracy diminish by 30% in these situations, showcasing how external sounds can interfere with the transcription process.
Furthermore, valuable information is lost when only focusing on the spoken words. Gestures, common in lectures, often provide important context, but are usually overlooked by AI and humans alike. Their absence leads to misinterpretations of intent and emphasis in the resulting text.
The variability of lecturers' speaking styles adds another challenge. AI, often trained on more standardized speech, can struggle with the unique cadence or pronunciations of different speakers, leading to noticeable transcription errors.
Long-term analysis has revealed that over extended periods, human transcribers typically outperform AI in maintaining transcription accuracy, particularly when handling complex discussions. It appears AI might be initially fast, but with longer recordings, errors can creep in without constant human monitoring.
Humans have a significant advantage in understanding the overall meaning, or semantic context, of what's said. They are better at inferring the meaning of ambiguous phrases or jargon during the Q&A, while AI struggles considerably, sometimes resulting in transcripts that are incomplete or misleading.
Overall, these findings paint a picture of a complex landscape where the best approach for academic lecture transcription depends on various factors, from the length and complexity of the lecture to the specific needs of the user. It highlights that while AI is becoming increasingly capable, human intervention often plays a critical role in achieving the level of accuracy necessary in many academic settings.
Otterai vs Human Transcription Comparing Accuracy Rates in 7 Different Meeting Scenarios - Corporate Earnings Call With International Participants
Corporate earnings calls that involve participants from different countries present unique challenges for transcription. These calls often feature complex financial terminology and individuals with diverse accents, making it a difficult environment for automated systems. While AI-based tools like Otter.ai are useful for quickly generating transcripts, their accuracy can be hindered by the overlapping speech and various speaking styles common in these types of meetings.
Human transcribers are typically better suited for handling these complexities. They possess a more in-depth comprehension of the context and the financial language used in these calls, resulting in transcripts that are more reliable and accurate. The interplay of different accents, technical language, and the likelihood of poor audio quality makes the choice of transcription method a significant decision. Even as AI systems improve, the need for human expertise remains prominent in achieving the level of accuracy required for these crucial business interactions.
When examining corporate earnings calls with international participants, we encounter a complex interplay of factors that can influence transcription accuracy. Scheduling these calls across various time zones can be a major hurdle, potentially leading to reduced participation from certain regions. Research suggests a decline of more than 20% in participation when scheduling isn't optimal for a global audience, impacting the diversity of perspectives shared.
Language barriers also play a role. Non-native English speakers often find it challenging to participate actively in these calls. Studies have indicated that they are about 30% less likely to speak up compared to native English speakers, potentially skewing the insights gathered. This limitation can affect the richness and breadth of information obtained from these important business discussions.
The length of these calls, typically around an hour, poses challenges for comprehension and transcription. Evidence shows that listeners’ ability to grasp the information presented decreases considerably after roughly 30 minutes, which could negatively affect the accuracy of transcriptions. This decline in attention could lead to mistakes or misinterpretations, especially in capturing detailed financial discussions.
The presence of diverse accents adds another layer of complexity. AI transcription tools, despite advancements, still struggle to accurately capture speech with strong regional accents. Research shows they can misinterpret between 25% and 40% of words in such situations, highlighting a persistent limitation of automated solutions. This discrepancy is particularly noteworthy in earnings calls, where precise language and financial terminology are crucial for understanding.
Human transcribers also face challenges during these calls, specifically the cognitive burden associated with parsing complex financial jargon and maintaining focus for extended periods. Research shows that their error rates can increase by up to 15% after an hour of sustained attention. While humans are adept at contextual understanding, maintaining focus during lengthy calls can be demanding and can lead to performance variations.
The extended duration of earnings calls also raises concerns about listener attention spans. Studies suggest that a typical listener’s attention starts to decline after about 20 minutes. This begs the question of whether lengthy calls are the most effective format for conveying intricate financial information. Perhaps shorter, more focused discussions might be a more effective way to ensure clear communication and accurate comprehension.
Companies are increasingly leveraging AI-powered transcription technologies. However, these tools often fall short in capturing subtle contextual cues such as emotional nuances in speech. This lack of emotional understanding leads to potential loss of critical information, which can be vital for comprehensive interpretation of financial discussions. These calls are often the first public glimpse into a company's financial health, and missing nuanced cues can lead to an incomplete picture.
The time-sensitive nature of the information shared during earnings calls is significant. Research reveals that the financial markets can react to the content within just 15 minutes. This fast response time emphasizes the need for quick and accurate transcription. Delays in transcription can lead to misalignments between investor understanding and the information shared by the company, potentially impacting stock prices and market reactions.
Decision-making in the corporate world is often influenced by earnings calls. Given that many decisions are made in the immediate aftermath of these calls, often in response to what was communicated, accurate transcription becomes paramount. The decisions made can impact stock prices within a matter of hours, making accurate information crucial. Misinterpretations or errors in transcription can lead to incorrect assumptions and potentially detrimental business decisions.
Finally, when conducting earnings calls with an international audience, the issue of confidentiality becomes particularly important. These calls can contain sensitive information that needs to be protected. It's crucial to manage transcripts carefully to prevent potential breaches of privacy, which could lead to regulatory issues, insider trading problems, and reputational damage for both the company and those involved.
In conclusion, corporate earnings calls with international participants present unique challenges for transcription. The interplay of time zones, language barriers, accent variations, and the cognitive load on both human and AI transcribers highlights the need for a careful evaluation of the transcription methods used. While AI solutions offer speed and efficiency, human intervention often proves critical for achieving the level of accuracy and contextual understanding needed to accurately capture the nuanced and impactful information exchanged during these important corporate events.
Otterai vs Human Transcription Comparing Accuracy Rates in 7 Different Meeting Scenarios - Live Event Panel Discussion With Audience Interaction
Live event panel discussions, especially those incorporating audience interaction, present a unique challenge for transcription, especially when contrasting AI solutions like Otter.ai with human transcription. The dynamic nature of these events, with real-time audience participation, adds a level of complexity that impacts the accuracy of transcription. Maintaining clarity in audio and video is vital, but when multiple voices overlap, or individuals have diverse speaking styles, AI struggles to maintain accuracy. While AI transcription can provide quick results, capturing the nuances of live interactions, including spontaneous audience questions, remains problematic for current AI technology. Human transcribers, on the other hand, can leverage their adaptability and ability to discern contextual cues, typically offering more reliable transcripts in such environments. However, even human transcribers can face fatigue and accuracy declines, especially in longer discussions. Ultimately, deciding between AI and human transcription in these settings involves weighing not just the desired level of accuracy but also how well the transcription captures the dynamic interaction. This, in turn, determines how well the recording fulfills its purpose as an educational or informative tool.
Live event panel discussions with audience interaction present a fascinating and complex challenge for both human and automated transcription. The dynamic nature of these events, with multiple speakers, audience participation, and often rapid-fire exchanges, throws a curveball at the ability of both humans and machines to create accurate records.
It's notable that audience participation doesn't just add to the number of speakers; it significantly changes the flow and content of discussions. Research suggests that a lively audience can actually increase the depth of conversation by as much as a third, which in turn impacts the transcription effort. The unexpected questions and interactions create a sort of back-and-forth that requires both human and AI transcribers to constantly adapt and adjust their focus.
Interestingly, this added layer of complexity seems to be a particular strain on human cognitive capabilities. It appears human transcribers can experience a significant decrease in accuracy, sometimes as high as 35%, when they have to manage a dynamic exchange between speakers and the audience. This mental juggling act, with its constant switching of attention, seems to lead to a higher rate of errors.
AI systems also struggle with the variability in speech that occurs during these events. Speakers often tend to talk at a faster rate in live environments, exceeding 200 words per minute at times. This rapid pace, coupled with potential overlaps in dialogue, poses a considerable challenge for AI, which still has difficulties precisely deciphering who said what and when.
Further adding to the challenge is the frequent use of specialized language during these discussions. Panel discussions often involve technical terms or domain-specific vocabulary, leading to misinterpretations in automated transcriptions. These systems may struggle to correctly process 40% of the technical vocabulary used, a considerably higher error rate than human transcribers, who appear to leverage their understanding of context to achieve a lower error rate, roughly around 15%.
The presence of audience interactions adds another wrinkle. Applause, laughter, and other audible reactions can sometimes interfere with the clarity of the main speakers. This layering of sound has been observed to result in a decrease of about 25% in overall accuracy, reminding us that careful audio management is crucial in these scenarios.
Global events, with speakers and audience members from diverse backgrounds, also highlight the limitations of current AI transcription technology. Accent variations and multilingualism can lead to substantial misinterpretations, with researchers finding up to 30% of statements made in non-native accents incorrectly captured. Human transcribers, especially those familiar with various dialects, seem to handle these challenges more effectively.
Furthermore, feedback loops that occur naturally in live events can also impact transcription accuracy. Audience feedback, which leads to speakers clarifying or expanding on their thoughts, results in a richer, more nuanced conversation. But this dynamic interaction, which is a positive aspect of live engagement, can cause difficulties for transcription software as it struggles to keep pace with the shifts in content.
The ability to correct errors in real-time is an interesting point of comparison between human and AI approaches. Human transcribers working live have shown the ability to address and correct errors by as much as 50%. This immediate, adaptive approach is unique to human interventions and further underscores the value of human involvement in preserving accuracy during dynamic discussions.
Finally, microphone placement plays a key role in the overall clarity of the audio, which impacts the performance of both human and AI transcribers. Employing microphones closer to the speaker, such as lapel mics, has been shown to significantly improve audio quality by roughly 40%. This improvement in clarity can directly reduce errors made during transcription.
Lastly, the level of audience engagement during a live event correlates with information retention. This suggests that a highly engaged audience, with their participation and reactions, provides a richer context for both human and AI transcribers. This richness of information and the nuances it provides enhance the chance of capturing a more accurate and detailed transcription.
In conclusion, the live panel format, with its complex interplay of multiple speakers, audience interactions, and rapid-fire exchanges, serves as a reminder of the ongoing challenges in achieving truly accurate transcription. While AI continues to advance and offers valuable features for speed and initial capturing, human interventions, with their capability to adapt and understand contextual information, are often essential for achieving the desired level of precision, especially when dealing with complex and nuanced discussions.
Otterai vs Human Transcription Comparing Accuracy Rates in 7 Different Meeting Scenarios - Legal Deposition Recording With Multiple Expert Witnesses
Legal depositions involving multiple expert witnesses introduce a complex layer to the transcription process, especially when considering the accuracy of AI versus human transcriptionists. The combination of specialized legal terminology, potentially overlapping testimony, and varying speaking styles among the experts creates a challenging environment for automated systems. Human transcribers, with their deep understanding of legal language and ability to follow intricate dialogues, often deliver higher accuracy in these settings. While AI transcription technologies are advancing, they continue to face obstacles in handling nuanced legal jargon and can sometimes misinterpret or miss key aspects of the proceedings. In the legal context, where precise records are crucial, human transcription might be favored over AI, despite the latter's speed benefits, particularly when accuracy and detail are paramount and the consequences of error are severe.
Legal depositions, especially those involving multiple expert witnesses, present a unique challenge for both human and AI transcription. Human transcribers face a significant cognitive load when trying to keep up with overlapping dialogue from multiple experts. Research suggests that accuracy can drop by as much as 30% when transcribers struggle to follow rapid-fire exchanges and interruptions. However, human transcribers who are familiar with the specific legal terminology and context of the case often produce more accurate transcripts. They can reduce errors by about 15% compared to either generalists or AI systems, which tend to struggle with the intricacies of legal language.
Unlike AI, humans can adapt more readily to the changing flow of legal discussions. They're better at handling speakers talking out of turn or interrupting each other, leading to a better understanding of complex conversations and resulting in a higher-quality transcript.
The audio quality plays a key role in how well a transcription captures a deposition. High-quality microphones can significantly improve clarity and boost transcription accuracy by as much as 40%, a crucial factor in legal settings where precise language is paramount.
AI also faces challenges when it comes to legal jargon and regional variations. Legal terminology can vary by jurisdiction, and AI systems may not adapt well to those nuances, causing them to incorrectly transcribe technical terms up to 50% of the time. When depositions involve individuals who aren't native English speakers, AI struggles even more, often making mistakes when attempting to decipher accents and unique speech patterns. Accuracy can plummet to 40% in these instances.
Humans have an advantage when it comes to real-time error correction. They can quickly fix mistakes as the deposition progresses, improving the final transcription quality by up to 50%. This ability to instantly adapt to context is a significant advantage over AI's current capabilities.
The environment where the deposition takes place also plays a part. Poorly designed rooms with lots of echo or background noise can affect accuracy, potentially decreasing quality by about 25% if not managed with proper equipment.
Human transcribers, like anyone, can experience fatigue during long deposition sessions. Research shows that accuracy can decline by about 15% after a while. This factor highlights the importance of managing deposition lengths to ensure quality remains high.
Another major hurdle for AI is accurately identifying who's speaking during depositions with several witnesses. Speaker diarization, the process of figuring out who said what, is still a challenge for automated systems. They misattribute speaker dialogue as much as 30% of the time, reinforcing the value of skilled human intervention for maintaining clear and accurate records.
In summary, while AI is becoming increasingly useful, human transcribers still hold an advantage in legal deposition settings, particularly those with multiple witnesses. Humans' ability to adapt to a rapidly changing environment, understand the context of the legal discussion, and correct errors in real-time can result in significantly higher accuracy compared to AI. However, both humans and machines have their limitations, making the choice of method a matter of balancing factors like complexity, cost, and the desired level of accuracy for the specific situation. As the field of AI progresses, it will be interesting to see how these accuracy gaps might change.
More Posts from transcribethis.io: