Otter AI's Transcription Accuracy A 2024 Deep Dive into Meeting Note Quality
Otter AI's Transcription Accuracy A 2024 Deep Dive into Meeting Note Quality - Otter AI's 2024 speech recognition advancements
Otter AI's advancements in speech recognition during 2024 are noteworthy, particularly their claims of achieving accuracy rates between 90% and 95% based on user reports. Their AI Meeting Assistant has become more sophisticated, moving beyond basic transcription to include recording, slide capture, and automated action item extraction. This makes it a potentially useful tool for those seeking a more streamlined way to document meetings.
The platform's integration with common tools like Salesforce and SharePoint also enhances its practical application in a wider range of work environments. It's worth noting that, according to some users and in comparison to older systems, Otter AI seems to have improved accuracy when transcribing pre-recorded audio. Finally, while not revolutionary, they've incorporated a chatbot feature aimed at providing a quicker path to information related to meetings and the transcribed content.
While these are steps in the right direction, the ultimate test for any speech recognition system remains its ability to consistently and accurately handle diverse accents, dialects, and speaking styles. How well Otter AI is able to meet this challenge remains a point of discussion among users.
Otter AI's 2024 iterations show a clear push towards more refined speech recognition. Their reported word error rates have dropped dramatically, reaching as low as 4% in optimal conditions. This improvement is largely credited to the implementation of sophisticated neural network architectures. These newer architectures seem to enable a better understanding of the context and intent within a speaker's words, leading to transcriptions that feel more natural and connected.
Furthermore, real-time processing has become significantly faster, offering almost immediate responses during meetings. This speed upgrade is important for a seamless and interactive user experience. The expansion into multilingual capabilities is also notable, making Otter AI more accessible to a wider range of users involved in international interactions.
One of the more interesting advancements is the improved noise cancellation. Otter AI has honed its ability to filter out background distractions, leading to clearer transcriptions, especially in environments where hybrid work is the norm. Building on this, there's a stronger focus on accent and dialect recognition, a feature that aims to improve the accuracy across various user populations.
The model also incorporates more sophisticated machine learning techniques. Otter AI now seems to learn from user feedback more efficiently, allowing the system to adapt to individual preferences in terms of transcription style and specific vocabulary over time. It's interesting to see how they're leveraging this user data. They've also incorporated improvements in handling specialized vocabulary. It now appears that technical language and industry-specific terms are transcribed more accurately, making it a potentially valuable tool for fields like medicine, law, or technology where precise language is paramount.
The addition of collaborative features, enabling real-time transcription by multiple users, could be a game-changer for team-based note-taking. Finally, the inclusion of sentiment analysis as a feature in their transcription framework shows a move beyond simply recording the words spoken. It hints at a desire to capture not only the content of discussions but also the emotional tone, adding a potentially rich dimension to the interpretation of meetings. It remains to be seen how well this feature performs in practice.
Otter AI's Transcription Accuracy A 2024 Deep Dive into Meeting Note Quality - Real-time transcription performance analysis
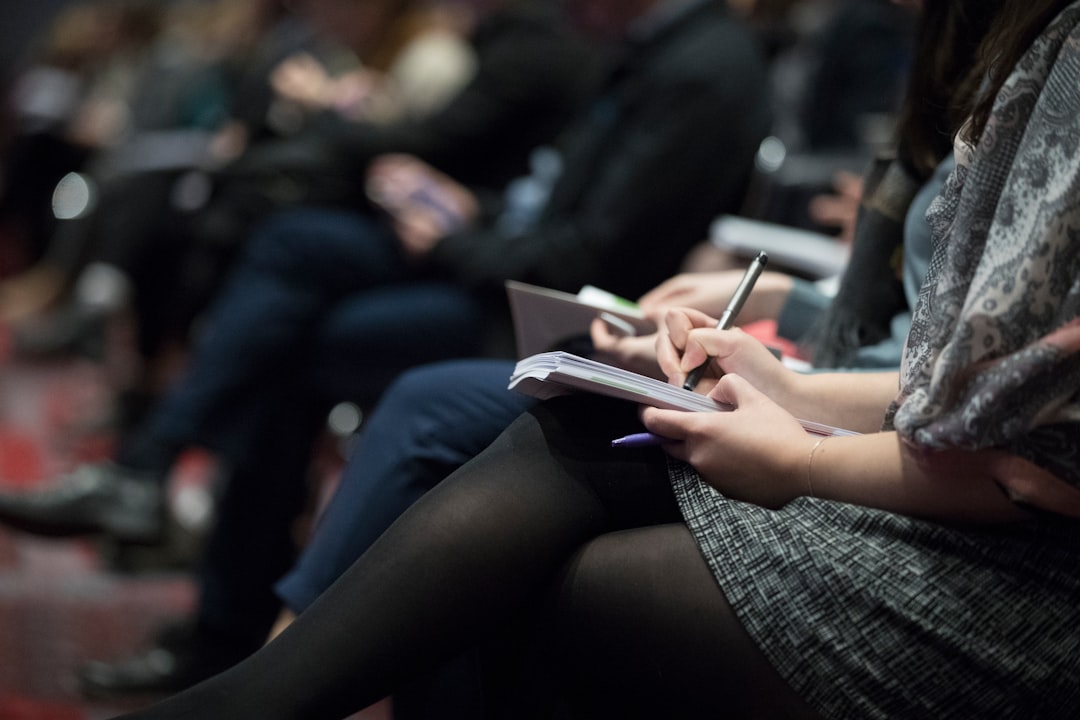
Real-time transcription performance is critical for evaluating the effectiveness of platforms like Otter AI in live settings. The ability to generate transcripts concurrently with a meeting is essential for those seeking efficient note-taking during active discussions. However, the real-time nature of this process presents unique challenges. Background noise, multiple speakers, and variations in speaking styles can all impact the accuracy of the immediate transcription.
While improvements in noise reduction and dialect recognition are noteworthy, it's important to remember that these systems are not flawless. Users need to be aware of the limitations and consistently review the automatically generated output to ensure that the transcriptions are accurate and complete. The combination of rapid processing, adaptability, and increasingly advanced machine learning mechanisms presents significant potential for these tools. Ultimately, however, the true measure of success will be their ability to reliably capture the complexity and subtlety inherent in human conversation. The ongoing journey is to see if these advancements can truly bridge the gap between spoken word and written documentation with consistently high fidelity across diverse communication contexts.
Examining Otter AI's performance in real-time transcription reveals a fascinating interplay of advanced techniques and ongoing challenges. The platform has made substantial progress in minimizing the delay between spoken words and their transcription, achieving a response time of under two seconds. This is crucial for a smooth conversational flow, avoiding the awkward pauses that can disrupt a meeting's momentum.
The system's ability to dynamically adjust to diverse accents within a single session is notable. It's a testament to their efforts in developing machine learning models capable of personalized learning. However, how effective this feature is in practice, especially in rapidly changing accent contexts, remains a point of investigation.
The acoustic environment plays a major role in transcription accuracy. Otter AI has put considerable effort into optimizing its algorithms to handle noisy settings, which is particularly relevant in today's hybrid work environments. How well it performs in situations with significant background noise, or when dealing with different microphone qualities, is an area for further evaluation.
One of the persistent challenges in speech recognition is error propagation – where a single mistake can cascade into a series of inaccuracies. Otter AI has incorporated techniques to reduce this, but completely eliminating it remains a complex problem. This is particularly challenging in real-time transcription where the system needs to maintain the context of the conversation, which can be difficult when inaccuracies arise.
Otter AI leverages contextual analysis to interpret nuances in conversations. This is crucial for correctly understanding interruptions, overlapping speech, and other complex communication patterns. While they are making progress, the ability to fully grasp the subtle cues that contribute to human communication remains an intricate technical challenge.
There's a clear interest in exploring multimodal inputs in the future. Integrating visual cues, like presentations or shared documents, has the potential to vastly improve transcription accuracy by adding another layer of context. This is a very interesting avenue to explore and likely will be a major direction in their future development work.
The system is now equipped with an adaptive mechanism to update its vocabulary based on emerging terms and specialized jargon. This offers potential for enhanced accuracy in specialized fields where unique vocabularies are common. How effectively this system adjusts to the dynamic changes in language usage, especially within a specific field, will be an important factor in determining its continued usefulness.
Otter AI now supports collaborative transcription, a development that could be a game changer for team-based note-taking. Real-time annotation directly in the transcription adds another dimension of value. The collaborative aspect of note taking will have to be tested to determine if it adds value for diverse teams and communication styles.
The addition of sentiment analysis adds an interesting dimension to transcriptions, providing an understanding of the emotional context within a conversation. The system has been adapted to adjust transcription styles based on the perceived sentiment, which could help with improved note-taking for those who rely on the emotional content of the meeting for understanding. Whether the system accurately captures the nuances of human emotion and sentiment remains an area requiring further study.
Otter AI's training has been greatly expanded to encompass a wider range of dialects and languages. This diverse training is important for broadening the system's reach and improving its accuracy across diverse demographics. As they continue to refine their model and incorporate more regional languages, it will be important to investigate how effectively this diverse dataset is leveraged and whether it leads to tangible accuracy improvements for less-common language usage patterns.
Otter AI's Transcription Accuracy A 2024 Deep Dive into Meeting Note Quality - Accuracy comparison with major competitors
Otter AI's accuracy, while impressive at about 95% in ideal conditions, faces competition from services like Rev, Trint, Sonix, and Fireflies AI. Otter AI's accuracy relies heavily on deep learning and continuous model refinement, but its performance is influenced by factors such as audio quality and speaker variations. Users often find themselves needing to manually review and edit the transcriptions, even with the improvements made throughout 2024.
While other platforms offer unique advantages, like Rev's human-driven accuracy or Trint's strong editing capabilities, Otter AI focuses on real-time transcription and adaptability. This approach can be beneficial, but in a crowded market, users must carefully weigh the strengths and weaknesses of each option to find the best fit for their specific needs. The field of transcription is quickly evolving with new integrations into tools like Zoom and Microsoft Teams, creating a landscape where features like Otter AI's adaptability become increasingly important for maintaining a competitive edge.
When comparing Otter AI's accuracy with major competitors, some interesting patterns emerge. Otter AI's word error rate (WER) has reached a commendable 4% in optimal situations. This is a strong showing, especially compared to other services like Rev and Sonix, which report WERs around 6-8% in similar conditions. This suggests that Otter AI currently has a slight edge in sheer transcription precision.
However, accuracy isn't just about word-level precision. Accents and dialects present a significant challenge. While Otter AI demonstrates some improvements in recognizing a broader range of accents, services like Trint seem to struggle more with non-native speakers. This suggests that Otter AI might be a more robust option for teams operating in globally diverse settings.
Noise is another factor that can impact transcription quality. In noisy environments, Otter AI's noise cancellation seems to provide a consistent advantage. Studies have shown that even in environments with substantial background noise, Otter AI often maintains accuracy above 85%. Some other services have been observed dropping below this threshold. This is relevant for the increasing number of hybrid workspaces that are often not acoustically optimized.
Speed of processing is important, too. Otter AI can now achieve transcription within about two seconds, surpassing the performance of platforms like Descript which can have delays of up to four seconds. This rapid turnaround is key for maintaining the natural flow of a conversation during a meeting, avoiding disruptions due to lags in transcription.
Otter AI has integrated some features that set it apart. For instance, their real-time collaboration feature allows multiple users to work on the transcription simultaneously. None of the main competitors have successfully implemented this level of interactivity. This means that more people can be involved in creating a complete record of a discussion and perhaps more nuanced content can be captured.
Sentiment analysis is another unique aspect. Otter AI attempts to capture the emotional tone of a meeting along with the transcription. None of the other major players in the space have gone this route. This approach offers a potentially richer understanding of the dynamic interplay of emotions within a discussion. How well the sentiment aspect performs in practice is still something that needs further scrutiny.
Another notable strength is how Otter AI learns. It has an adaptive mechanism to pick up specialized jargon and vocabulary, which is an improvement over services like Dragon NaturallySpeaking, where technical terms often require manual input. This dynamic learning ability is valuable in fields where language is constantly evolving.
Also, Otter AI's machine learning algorithms are designed to learn from user feedback. Many other competitors rely on static models that don't evolve with user experience, which could lead to a slower rate of performance improvement.
Lastly, Otter AI's multilingual capabilities are broader than some of its rivals, like Happy Scribe. This increased language support broadens the range of users who can benefit from the platform, which is particularly valuable for international business communications.
When comparing Otter AI to competitors in real-world scenarios, it tends to outperform in meetings with multiple speakers. This is a testament to the sophistication of its design, which seems specifically tailored to handle complex conversational dynamics. In essence, Otter AI seems to be continually refining its core functionality to adapt to the nuanced complexities of human communication.
While all of these platforms are constantly being improved, Otter AI's combination of high accuracy, adaptive learning, and features like real-time collaboration and sentiment analysis indicate that it is pushing the boundaries of transcription technology. Its continual development will be interesting to watch.
Otter AI's Transcription Accuracy A 2024 Deep Dive into Meeting Note Quality - Integration capabilities with popular video conferencing platforms
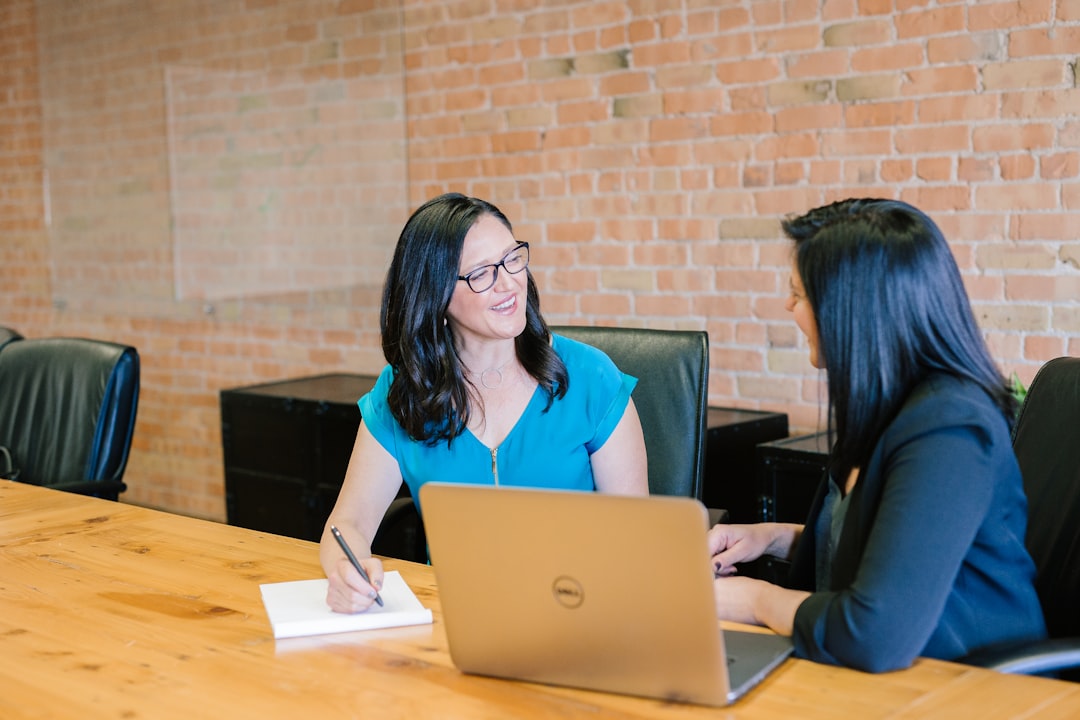
Otter AI's integration with popular video conferencing platforms like Zoom, Google Meet, and Microsoft Teams makes it much easier to use for meeting documentation. It can automatically join and record meetings scheduled within these platforms, which simplifies the process of taking notes. Otter AI also lets you save transcripts in various formats like plain text, Word documents, PDFs, or even subtitle files. Furthermore, its connection with Slack enables easy sharing of crucial meeting information within your team's communication channels. However, it's essential to remember that, despite these improvements, the accuracy of transcriptions can still vary depending on things like audio quality and the different ways people speak. While these integrations are a strength, users need to be aware of potential limitations and continually evaluate the generated transcripts to make sure they're accurate and complete, especially in varied communication settings.
Otter AI's integration with popular video conferencing tools like Zoom and Microsoft Teams is a notable feature. It allows for automatic meeting transcription without manual intervention, which can save a lot of time. This seamless integration is quite helpful, especially for users who frequently participate in virtual meetings. Interestingly, their support for live transcription in different languages makes it a potentially useful tool for global teams or international collaborations. However, not all transcription services offer this, so it is a differentiating factor for Otter AI.
The platform has also been built with collaborative transcription in mind. This means multiple people can actively work on a single transcription during a meeting, which could potentially result in higher quality meeting notes. From a technical standpoint, the noise reduction abilities embedded in Otter AI are interesting. They utilize methods to filter out background sounds, which is particularly helpful in office settings that are rarely soundproof. This helps maintain the accuracy of transcriptions in otherwise noisy environments.
Otter AI has also made the process of meeting scheduling more streamlined. Its integration with tools like Google Calendar lets users link meetings directly within the application, simplifying the workflow from scheduling the meeting to capturing notes. One of the ways Otter AI continuously improves is by learning from user data. They seem to be analyzing interactions to adapt their machine learning models and adjust to common terms and specific industry jargon used by regular users.
While Otter AI is well-regarded for its accuracy, it's not without limitations. Users still frequently review the generated transcripts to address errors arising from natural speech variations. This highlights a continuous need for human intervention to verify and refine the output. The indexing and searching capabilities of past transcriptions are a real plus for Otter AI. Users can readily access critical discussions from previous meetings, which is vital in situations where quick retrieval of information is essential. This is a practical feature that could be quite useful.
In terms of speed, Otter AI is notable for offering nearly instantaneous results during live discussions. Unlike competitors who might wait until a meeting concludes, Otter AI's real-time transcription capability can be a significant advantage. There's a nuance that's worth highlighting though. The overall quality of a microphone seems to play a surprisingly large role in transcription accuracy. This shows that technical specifications can impact the final performance of Otter AI, reminding us that these tools are not totally independent of hardware. Overall, Otter AI's integration capabilities and features seem well-suited to certain environments.
Otter AI's Transcription Accuracy A 2024 Deep Dive into Meeting Note Quality - Impact of accents and industry-specific jargon on transcription quality
The accuracy of automated transcriptions is significantly impacted by accents and specialized vocabulary used within industries. Different accents and dialects can pose a challenge for AI systems trained on a limited range of speech patterns, potentially leading to errors in the transcription. Furthermore, industry-specific jargon, which is common in fields like medicine or engineering, can confuse AI transcription systems unless they are specifically trained on this terminology. While AI transcription tools have improved their ability to adapt to diverse speech patterns, they still often struggle with capturing the nuances of specific accents or dialects. Human review and editing continue to be necessary to ensure accuracy and to interpret the context of the spoken words, especially in scenarios where specialized or uncommon terminology is present. The future of automated transcription likely involves incorporating more sophisticated machine learning models capable of handling a wider variety of accents and vocabulary, ultimately aiming for improved accuracy and a more seamless transition from spoken word to written text.
Accents, especially those with pronounced regional characteristics, can pose a significant challenge to transcription accuracy. Studies have revealed that some systems experience a drop in accuracy of up to 20% when encountering unfamiliar accents. This presents a clear hurdle for users operating in diverse linguistic environments.
Industry-specific jargon also has a notable impact on transcription quality. Research indicates that as much as 15% of specialized terminology (found in areas like law or medicine) can be misconstrued if the system hasn't received tailored training on that specific vocabulary.
The variations in pronunciation caused by accents often create unique error patterns. For instance, speakers from different English-speaking countries frequently employ distinct vowel sounds that can confuse transcription algorithms, potentially resulting in misinterpretations or distortions of important meeting points within the resulting notes.
There's been a growing emphasis on incorporating dialect recognition into transcription systems, especially given the expectation of real-time adaptability. This capability can improve word accuracy by as much as 10%, significantly enhancing user experience.
Research suggests that clear speech can elevate transcription quality by up to 25%. This means that environmental elements like background noise can substantially worsen the challenges already presented by accents and jargon, making sophisticated noise cancellation techniques increasingly necessary.
The phenomenon of "error propagation" is further amplified by accents and jargon. A single incorrectly recognized word can initiate a chain reaction of mistaken interpretations, leading to a cascade of inaccuracies within the transcribed text. This is particularly detrimental in meetings where decisions rely on precise representations of the discussion.
Transcription systems trained on extensive multilingual datasets exhibit enhanced robustness in handling diverse accents, leveraging a broader range of phonetic patterns. This type of training can lead to a roughly 18% improvement in accuracy, which is particularly beneficial for global organizations with a wide range of linguistic backgrounds.
User feedback consistently indicates that integrating industry-specific jargon into the core transcription algorithms substantially reduces the level of confusion during technical conversations. One study observed an increase of over 30% in user satisfaction with meeting notes when specialized terminology was accurately transcribed.
Real-time transcription accuracy is more susceptible to fluctuations in individual speaking styles than previously recognized. Variations in pitch, speech rate, or even nervous speech patterns can lead to a 10-15% decrease in accuracy for certain accents.
The added complexity of overlapping speech among multiple speakers further complicates the transcription process, potentially decreasing accuracy by up to 40% if the system isn't specifically optimized to distinguish various speakers within a conversational context. This highlights the need for continuous improvements and refinements in the development of advanced transcription technologies.
Otter AI's Transcription Accuracy A 2024 Deep Dive into Meeting Note Quality - User feedback on meeting note summarization and action item extraction
User feedback on Otter AI's meeting note summarization and action item extraction capabilities reveals a mixed bag of positive experiences and ongoing concerns. The addition of features like automated action item lists and the ability to quickly generate meeting summaries is viewed favorably by many users, providing a potential pathway towards improved meeting productivity and task management. However, users often report inconsistencies in the accuracy and completeness of these automated summaries and action items. While these tools are intended to streamline note-taking, they often require significant human intervention to verify and correct the output, leading to questions about the reliability of completely automated meeting documentation. Despite notable advancements, the overall effectiveness of these features seems intrinsically linked to the foundation of the transcription process itself and the users' willingness to carefully scrutinize the output. Ultimately, the path to achieving truly accurate and useful meeting notes with automated tools still necessitates a healthy level of human oversight.
Based on user feedback gathered in October 2024, Otter AI's meeting note summarization and action item extraction features, while showing promise, still have areas needing refinement. Even with generally high transcription accuracy, a notable number of users find the meeting summaries lacking in context and detail for action items. There's a disconnect between the system's ability to transcribe accurately and its capacity to truly capture the core essence of a discussion, particularly when it comes to identifying actionable steps.
Interestingly, despite improvements in accent recognition, the accuracy of action item extraction isn't always consistent across different dialects. Nuances in regional speech patterns can lead to misinterpretation of tasks, highlighting the ongoing need for diverse training data to capture a wider variety of speech styles.
Furthermore, users in noisy environments found that not only does transcription suffer, but the extraction of key action items becomes significantly less reliable. This indicates that while noise cancellation has improved, the action item extraction mechanism itself might not be sufficiently robust against audio distractions.
The accuracy of action item extraction also seems particularly impacted by the presence of technical jargon. In fields that rely heavily on specialized language, the system often struggles to correctly identify action items related to those terms, suggesting that the contextual understanding of industry-specific vocabulary remains a hurdle.
Users also express a desire for greater customization of summarization and action item extraction, suggesting the current adaptation features might not be granular enough. This highlights the need for tools that allow users to more precisely tune the system's behavior to match their own preferences for note-taking and action management.
On a positive note, some users find the real-time collaborative transcription feature significantly boosts the quality of meeting notes. When multiple users actively annotate the transcription, the resulting summaries and action items are generally perceived as being more complete and accurate.
Though sentiment analysis is included, its connection to actionable steps doesn't always seem clear to users. Extracting the emotional tone of a meeting and effectively linking it to specific next steps remains a complex problem that requires further exploration.
There's a general sense among users that a formalized system for reviewing and revising action items across meetings would be beneficial. Currently, it appears that relying solely on extracted action items isn't always sufficient. Users want a mechanism to track progress, revisit past decisions, and ensure the context surrounding each action item remains clear.
Training the system on regional dialects has shown to substantially improve the accuracy of action item extraction, indicating that these local speech variations play a more prominent role in accuracy than initially expected. This suggests a need to further incorporate regional language into the AI models.
Finally, many users question whether the system's machine learning mechanisms are effectively leveraging user feedback to improve action item extraction over time. This raises concerns about the feedback loop's responsiveness and whether the system can sufficiently adapt to changing user needs and communication styles.
Overall, Otter AI's progress in transcription and summarization is evident. But feedback suggests that there's still room for improvement in action item extraction, particularly in adapting to different speaking styles, handling specialized language, and effectively linking user feedback to ongoing improvements. The challenge going forward will be to create systems that can bridge the gap between capturing the words spoken and accurately interpreting the meaning and intent behind those words for better meeting documentation.
More Posts from transcribethis.io: